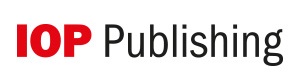

We apologize for the inconvenience...
To ensure we keep this website safe, please can you confirm you are a human by ticking the box below.
If you are unable to complete the above request please contact us using the below link, providing a screenshot of your experience.
https://ioppublishing.org/contacts/
9.1 How Microbes Grow
Learning objectives.
By the end of this section, you will be able to:
- Define the generation time for growth based on binary fission
- Identify and describe the activities of microorganisms undergoing typical phases of binary fission (simple cell division) in a growth curve
- Explain several laboratory methods used to determine viable and total cell counts in populations undergoing exponential growth
- Describe examples of cell division not involving binary fission, such as budding or fragmentation
- Describe the formation and characteristics of biofilms
- Identify health risks associated with biofilms and how they are addressed
- Describe quorum sensing and its role in cell-to-cell communication and coordination of cellular activities
Clinical Focus
Jeni, a 24-year-old pregnant woman in her second trimester, visits a clinic with complaints of high fever, 38.9 °C (102 °F), fatigue, and muscle aches—typical flu-like signs and symptoms. Jeni exercises regularly and follows a nutritious diet with emphasis on organic foods, including raw milk that she purchases from a local farmer’s market. All of her immunizations are up to date. However, the health-care provider who sees Jeni is concerned and orders a blood sample to be sent for testing by the microbiology laboratory.
- Why is the health-care provider concerned about Jeni’s signs and symptoms?
Jump to the next Clinical Focus box
The bacterial cell cycle involves the formation of new cells through the replication of DNA and partitioning of cellular components into two daughter cells. In prokaryotes, reproduction is always asexual, although extensive genetic recombination in the form of horizontal gene transfer takes place, as will be explored in a different chapter. Most bacteria have a single circular chromosome; however, some exceptions exist. For example, Borrelia burgdorferi , the causative agent of Lyme disease, has a linear chromosome.
Binary Fission
The most common mechanism of cell replication in bacteria is a process called binary fission , which is depicted in Figure 9.2 . Before dividing, the cell grows and increases its number of cellular components. Next, the replication of DNA starts at a location on the circular chromosome called the origin of replication, where the chromosome is attached to the inner cell membrane. Replication continues in opposite directions along the chromosome until the terminus is reached.
The center of the enlarged cell constricts until two daughter cells are formed, each offspring receiving a complete copy of the parental genome and a division of the cytoplasm (cytokinesis). This process of cytokinesis and cell division is directed by a protein called FtsZ . FtsZ assembles into a Z ring on the cytoplasmic membrane ( Figure 9.3 ). The Z ring is anchored by FtsZ-binding proteins and defines the division plane between the two daughter cells. Additional proteins required for cell division are added to the Z ring to form a structure called the divisome . The divisome activates to produce a peptidoglycan cell wall and build a septum that divides the two daughter cells. The daughter cells are separated by the division septum, where all of the cells’ outer layers (the cell wall and outer membranes, if present) must be remodeled to complete division. For example, we know that specific enzymes break bonds between the monomers in peptidoglycans and allow addition of new subunits along the division septum.
Check Your Understanding
- What is the name of the protein that assembles into a Z ring to initiate cytokinesis and cell division?
Generation Time
In eukaryotic organisms, the generation time is the time between the same points of the life cycle in two successive generations. For example, the typical generation time for the human population is 25 years. This definition is not practical for bacteria, which may reproduce rapidly or remain dormant for thousands of years. In prokaryotes (Bacteria and Archaea), the generation time is also called the doubling time and is defined as the time it takes for the population to double through one round of binary fission. Bacterial doubling times vary enormously. Whereas Escherichia coli can double in as little as 20 minutes under optimal growth conditions in the laboratory, bacteria of the same species may need several days to double in especially harsh environments. Most pathogens grow rapidly, like E. coli , but there are exceptions. For example, Mycobacterium tuberculosis , the causative agent of tuberculosis, has a generation time of between 15 and 20 hours. On the other hand, M. leprae , which causes Hansen’s disease (leprosy), grows much more slowly, with a doubling time of 14 days.
Micro Connections
Calculating number of cells.
It is possible to predict the number of cells in a population when they divide by binary fission at a constant rate. As an example, consider what happens if a single cell divides every 30 minutes for 24 hours. The diagram in Figure 9.4 shows the increase in cell numbers for the first three generations.
The number of cells increases exponentially and can be expressed as 2 n , where n is the number of generations. If cells divide every 30 minutes, after 24 hours, 48 divisions would have taken place. If we apply the formula 2 n , where n is equal to 48, the single cell would give rise to 2 48 or 281,474,976,710,656 cells at 48 generations (24 hours). When dealing with such huge numbers, it is more practical to use scientific notation. Therefore, we express the number of cells as 2.8 × 10 14 cells.
In our example, we used one cell as the initial number of cells. For any number of starting cells, the formula is adapted as follows:
N n is the number of cells at any generation n , N 0 is the initial number of cells, and n is the number of generations.
- With a doubling time of 30 minutes and a starting population size of 1 × 10 5 cells, how many cells will be present after 2 hours, assuming no cell death?
The Growth Curve
Microorganisms grown in closed culture (also known as a batch culture ), in which no nutrients are added and most waste is not removed, follow a reproducible growth pattern referred to as the growth curve . An example of a batch culture in nature is a pond in which a small number of cells grow in a closed environment. The culture density is defined as the number of cells per unit volume. In a closed environment, the culture density is also a measure of the number of cells in the population. Infections of the body do not always follow the growth curve, but correlations can exist depending upon the site and type of infection. When the number of live cells is plotted against time, distinct phases can be observed in the curve ( Figure 9.5 ).
The Lag Phase
The beginning of the growth curve represents a small number of cells, referred to as an inoculum , that are added to a fresh culture medium , a nutritional broth that supports growth. The initial phase of the growth curve is called the lag phase , during which cells are gearing up for the next phase of growth. The number of cells does not change during the lag phase; however, cells grow larger and are metabolically active, synthesizing proteins needed to grow within the medium. If any cells were damaged or shocked during the transfer to the new medium, repair takes place during the lag phase. The duration of the lag phase is determined by many factors, including the species and genetic make-up of the cells, the composition of the medium, and the size of the original inoculum.
The Log Phase
In the logarithmic (log) growth phase , sometimes called exponential growth phase , the cells are actively dividing by binary fission and their number increases exponentially. For any given bacterial species, the generation time under specific growth conditions (nutrients, temperature, pH, and so forth) is genetically determined, and this generation time is called the intrinsic growth rate . During the log phase, the relationship between time and number of cells is not linear but exponential; however, the growth curve is often plotted on a semilogarithmic graph, as shown in Figure 9.6 , which gives the appearance of a linear relationship.
Cells in the log phase show constant growth rate and uniform metabolic activity. For this reason, cells in the log phase are preferentially used for industrial applications and research work. The log phase is also the stage where bacteria are the most susceptible to the action of disinfectants and common antibiotics that affect protein, DNA, and cell-wall synthesis.
Stationary Phase
As the number of cells increases through the log phase, several factors contribute to a slowing of the growth rate. Waste products accumulate and nutrients are gradually used up. In addition, gradual depletion of oxygen begins to limit aerobic cell growth. This combination of unfavorable conditions slows and finally stalls population growth. The total number of live cells reaches a plateau referred to as the stationary phase ( Figure 9.5 ). In this phase, the number of new cells created by cell division is now equivalent to the number of cells dying; thus, the total population of living cells is relatively stagnant. The culture density in a stationary culture is constant. The culture’s carrying capacity, or maximum culture density, depends on the types of microorganisms in the culture and the specific conditions of the culture; however, carrying capacity is constant for a given organism grown under the same conditions.
During the stationary phase, cells switch to a survival mode of metabolism. As growth slows, so too does the synthesis of peptidoglycans, proteins, and nucleic-acids; thus, stationary cultures are less susceptible to antibiotics that disrupt these processes. In bacteria capable of producing endospores, many cells undergo sporulation during the stationary phase. Secondary metabolites, including antibiotics, are synthesized in the stationary phase. In certain pathogenic bacteria, the stationary phase is also associated with the expression of virulence factors, products that contribute to a microbe’s ability to survive, reproduce, and cause disease in a host organism. For example, quorum sensing in Staphylococcus aureus initiates the production of enzymes that can break down human tissue and cellular debris, clearing the way for bacteria to spread to new tissue where nutrients are more plentiful.
The Death Phase
As a culture medium accumulates toxic waste and nutrients are exhausted, cells die in greater and greater numbers. Soon, the number of dying cells exceeds the number of dividing cells, leading to an exponential decrease in the number of cells ( Figure 9.5 ). This is the aptly named death phase , sometimes called the decline phase. Many cells lyse and release nutrients into the medium, allowing surviving cells to maintain viability and form endospores. A few cells, the so-called persisters , are characterized by a slow metabolic rate. Persister cells are medically important because they are associated with certain chronic infections, such as tuberculosis, that do not respond to antibiotic treatment.
Sustaining Microbial Growth
The growth pattern shown in Figure 9.5 takes place in a closed environment; nutrients are not added and waste and dead cells are not removed. In many cases, though, it is advantageous to maintain cells in the logarithmic phase of growth. One example is in industries that harvest microbial products. A chemostat ( Figure 9.7 ) is used to maintain a continuous culture in which nutrients are supplied at a steady rate. A controlled amount of air is mixed in for aerobic processes. Bacterial suspension is removed at the same rate as nutrients flow in to maintain an optimal growth environment.
- During which phase does growth occur at the fastest rate?
- Name two factors that limit microbial growth.
Measurement of Bacterial Growth
Estimating the number of bacterial cells in a sample, known as a bacterial count, is a common task performed by microbiologists. The number of bacteria in a clinical sample serves as an indication of the extent of an infection. Quality control of drinking water, food, medication, and even cosmetics relies on estimates of bacterial counts to detect contamination and prevent the spread of disease. Two major approaches are used to measure cell number. The direct methods involve counting cells, whereas the indirect methods depend on the measurement of cell presence or activity without actually counting individual cells. Both direct and indirect methods have advantages and disadvantages for specific applications.
Direct Cell Count
Direct cell count refers to counting the cells in a liquid culture or colonies on a plate. It is a direct way of estimating how many organisms are present in a sample. Let’s look first at a simple and fast method that requires only a specialized slide and a compound microscope.
The simplest way to count bacteria is called the direct microscopic cell count , which involves transferring a known volume of a culture to a calibrated slide and counting the cells under a light microscope. The calibrated slide is called a Petroff-Hausser chamber ( Figure 9.8 ) and is similar to a hemocytometer used to count red blood cells. The central area of the counting chamber is etched into squares of various sizes. A sample of the culture suspension is added to the chamber under a coverslip that is placed at a specific height from the surface of the grid. It is possible to estimate the concentration of cells in the original sample by counting individual cells in a number of squares and determining the volume of the sample observed. The area of the squares and the height at which the coverslip is positioned are specified for the chamber. The concentration must be corrected for dilution if the sample was diluted before enumeration.
Cells in several small squares must be counted and the average taken to obtain a reliable measurement. The advantages of the chamber are that the method is easy to use, relatively fast, and inexpensive. On the downside, the counting chamber does not work well with dilute cultures because there may not be enough cells to count.
Using a counting chamber does not necessarily yield an accurate count of the number of live cells because it is not always possible to distinguish between live cells, dead cells, and debris of the same size under the microscope. However, newly developed fluorescence staining techniques make it possible to distinguish viable and dead bacteria. These viability stains (or live stains) bind to nucleic acids, but the primary and secondary stains differ in their ability to cross the cytoplasmic membrane. The primary stain, which fluoresces green, can penetrate intact cytoplasmic membranes, staining both live and dead cells. The secondary stain, which fluoresces red, can stain a cell only if the cytoplasmic membrane is considerably damaged. Thus, live cells fluoresce green because they only absorb the green stain, whereas dead cells appear red because the red stain displaces the green stain on their nucleic acids ( Figure 9.9 ).
Another technique uses an electronic cell counting device ( Coulter counter ) to detect and count the changes in electrical resistance in a saline solution. A glass tube with a small opening is immersed in an electrolyte solution. A first electrode is suspended in the glass tube. A second electrode is located outside of the tube. As cells are drawn through the small aperture in the glass tube, they briefly change the resistance measured between the two electrodes and the change is recorded by an electronic sensor ( Figure 9.10 ); each resistance change represents a cell. The method is rapid and accurate within a range of concentrations; however, if the culture is too concentrated, more than one cell may pass through the aperture at any given time and skew the results. This method also does not differentiate between live and dead cells.
Direct counts provide an estimate of the total number of cells in a sample. However, in many situations, it is important to know the number of live, or viable , cells. Counts of live cells are needed when assessing the extent of an infection, the effectiveness of antimicrobial compounds and medication, or contamination of food and water.
- Why would you count the number of cells in more than one square in the Petroff-Hausser chamber to estimate cell numbers?
- In the viability staining method, why do dead cells appear red?
Plate Count
The viable plate count , or simply plate count , is a count of viable or live cells. It is based on the principle that viable cells replicate and give rise to visible colonies when incubated under suitable conditions for the specimen. The results are usually expressed as colony-forming unit s per milliliter (CFU/mL) rather than cells per milliliter because more than one cell may have landed on the same spot to give rise to a single colony. Furthermore, samples of bacteria that grow in clusters or chains are difficult to disperse and a single colony may represent several cells. Some cells are described as viable but nonculturable and will not form colonies on solid media. For all these reasons, the viable plate count is considered a low estimate of the actual number of live cells. These limitations do not detract from the usefulness of the method, which provides estimates of live bacterial numbers.
Microbiologists typically count plates with 30–300 colonies. Samples with too few colonies (<30) do not give statistically reliable numbers, and overcrowded plates (>300 colonies) make it difficult to accurately count individual colonies. Also, counts in this range minimize occurrences of more than one bacterial cell forming a single colony. Thus, the calculated CFU is closer to the true number of live bacteria in the population.
There are two common approaches to inoculating plates for viable counts: the pour plate and the spread plate methods. Although the final inoculation procedure differs between these two methods, they both start with a serial dilution of the culture.
Serial Dilution
The serial dilution of a culture is an important first step before proceeding to either the pour plate or spread plate method. The goal of the serial dilution process is to obtain plates with CFUs in the range of 30–300, and the process usually involves several dilutions in multiples of 10 to simplify calculation. The number of serial dilutions is chosen according to a preliminary estimate of the culture density. Figure 9.11 illustrates the serial dilution method.
A fixed volume of the original culture, 1.0 mL, is added to and thoroughly mixed with the first dilution tube solution, which contains 9.0 mL of sterile broth. This step represents a dilution factor of 10, or 1:10, compared with the original culture. From this first dilution, the same volume, 1.0 mL, is withdrawn and mixed with a fresh tube of 9.0 mL of dilution solution. The dilution factor is now 1:100 compared with the original culture. This process continues until a series of dilutions is produced that will bracket the desired cell concentration for accurate counting. From each tube, a sample is plated on solid medium using either the pour plate method ( Figure 9.12 ) or the spread plate method ( Figure 9.13 ). The plates are incubated until colonies appear. Two to three plates are usually prepared from each dilution and the numbers of colonies counted on each plate are averaged. In all cases, thorough mixing of samples with the dilution medium (to ensure the cell distribution in the tube is random) is paramount to obtaining reliable results.
The dilution factor is used to calculate the number of cells in the original cell culture. In our example, an average of 50 colonies was counted on the plates obtained from the 1:10,000 dilution. Because only 0.1 mL of suspension was pipetted on the plate, the multiplier required to reconstitute the original concentration is 10 × 10,000. The number of CFU per mL is equal to 50 × 10 × 10,000 = 5,000,000. The number of bacteria in the culture is estimated as 5 million cells/mL. The colony count obtained from the 1:1000 dilution was 389, well below the expected 500 for a 10-fold difference in dilutions. This highlights the issue of inaccuracy when colony counts are greater than 300 and more than one bacterial cell grows into a single colony.
A very dilute sample—drinking water, for example—may not contain enough organisms to use either of the plate count methods described. In such cases, the original sample must be concentrated rather than diluted before plating. This can be accomplished using a modification of the plate count technique called the membrane filtration technique . Known volumes are vacuum-filtered aseptically through a membrane with a pore size small enough to trap microorganisms. The membrane is transferred to a Petri plate containing an appropriate growth medium. Colonies are counted after incubation. Calculation of the cell density is made by dividing the cell count by the volume of filtered liquid.
Link to Learning
Watch this video for demonstrations of serial dilutions and spread plate techniques.
The Most Probable Number
The number of microorganisms in dilute samples is usually too low to be detected by the plate count methods described thus far. For these specimens, microbiologists routinely use the most probable number (MPN) method , a statistical procedure for estimating of the number of viable microorganisms in a sample. Often used for water and food samples, the MPN method evaluates detectable growth by observing changes in turbidity or color due to metabolic activity.
A typical application of MPN method is the estimation of the number of coliforms in a sample of pond water. Coliforms are gram-negative rod bacteria that ferment lactose. The presence of coliforms in water is considered a sign of contamination by fecal matter. For the method illustrated in Figure 9.14 , a series of three dilutions of the water sample is tested by inoculating five lactose broth tubes with 10 mL of sample, five lactose broth tubes with 1 mL of sample, and five lactose broth tubes with 0.1 mL of sample. The lactose broth tubes contain a pH indicator that changes color from red to yellow when the lactose is fermented. After inoculation and incubation, the tubes are examined for an indication of coliform growth by a color change in media from red to yellow. The first set of tubes (10-mL sample) showed growth in all the tubes; the second set of tubes (1 mL) showed growth in two tubes out of five; in the third set of tubes, no growth is observed in any of the tubes (0.1-mL dilution). The numbers 5, 2, and 0 are compared with Figure B1 in Appendix B , which has been constructed using a probability model of the sampling procedure. From our reading of the table, we conclude that 49 is the most probable number of bacteria per 100 mL of pond water.no lo
- What is a colony-forming unit?
- What two methods are frequently used to estimate bacterial numbers in water samples?
Indirect Cell Counts
Besides direct methods of counting cells, other methods, based on an indirect detection of cell density, are commonly used to estimate and compare cell densities in a culture. The foremost approach is to measure the turbidity (cloudiness) of a sample of bacteria in a liquid suspension. The laboratory instrument used to measure turbidity is called a spectrophotometer ( Figure 9.15 ). In a spectrophotometer, a light beam is transmitted through a bacterial suspension, the light passing through the suspension is measured by a detector, and the amount of light passing through the sample and reaching the detector is converted to either percent transmission or a logarithmic value called absorbance (optical density). As the numbers of bacteria in a suspension increase, the turbidity also increases and causes less light to reach the detector. The decrease in light passing through the sample and reaching the detector is associated with a decrease in percent transmission and increase in absorbance measured by the spectrophotometer.
Measuring turbidity is a fast method to estimate cell density as long as there are enough cells in a sample to produce turbidity. It is possible to correlate turbidity readings to the actual number of cells by performing a viable plate count of samples taken from cultures having a range of absorbance values. Using these values, a calibration curve is generated by plotting turbidity as a function of cell density. Once the calibration curve has been produced, it can be used to estimate cell counts for all samples obtained or cultured under similar conditions and with densities within the range of values used to construct the curve.
Measuring dry weight of a culture sample is another indirect method of evaluating culture density without directly measuring cell counts. The cell suspension used for weighing must be concentrated by filtration or centrifugation, washed, and then dried before the measurements are taken. The degree of drying must be standardized to account for residual water content. This method is especially useful for filamentous microorganisms, which are difficult to enumerate by direct or viable plate count.
As we have seen, methods to estimate viable cell numbers can be labor intensive and take time because cells must be grown. Recently, indirect ways of measuring live cells have been developed that are both fast and easy to implement. These methods measure cell activity by following the production of metabolic products or disappearance of reactants. Adenosine triphosphate (ATP) formation, biosynthesis of proteins and nucleic acids, and consumption of oxygen can all be monitored to estimate the number of cells.
- What is the purpose of a calibration curve when estimating cell count from turbidity measurements?
- What are the newer indirect methods of counting live cells?
Alternative Patterns of Cell Division
Binary fission is the most common pattern of cell division in prokaryotes, but it is not the only one. Other mechanisms usually involve asymmetrical division (as in budding) or production of spores in aerial filaments.
In some cyanobacteria , many nucleoids may accumulate in an enlarged round cell or along a filament, leading to the generation of many new cells at once. The new cells often split from the parent filament and float away in a process called fragmentation ( Figure 9.16 ). Fragmentation is commonly observed in the Actinomycetes , a group of gram-positive, anaerobic bacteria commonly found in soil. Another curious example of cell division in prokaryotes, reminiscent of live birth in animals, is exhibited by the giant bacterium Epulopiscium . Several daughter cells grow fully in the parent cell, which eventually disintegrates, releasing the new cells to the environment. Other species may form a long narrow extension at one pole in a process called budding . The tip of the extension swells and forms a smaller cell, the bud that eventually detaches from the parent cell. Budding is most common in yeast ( Figure 9.16 ), but it is also observed in prosthecate bacteria and some cyanobacteria.
The soil bacteria Actinomyces grow in long filaments divided by septa, similar to the mycelia seen in fungi, resulting in long cells with multiple nucleoids. Environmental signals, probably related to low nutrient availability, lead to the formation of aerial filaments. Within these aerial filaments , elongated cells divide simultaneously. The new cells, which contain a single nucleoid, develop into spores that give rise to new colonies.
- Identify at least one difference between fragmentation and budding.
In nature, microorganisms grow mainly in biofilms , complex and dynamic ecosystems that form on a variety of environmental surfaces, from industrial conduits and water treatment pipelines to rocks in river beds. Biofilms are not restricted to solid surface substrates, however. Almost any surface in a liquid environment containing some minimal nutrients will eventually develop a biofilm. Microbial mats that float on water, for example, are biofilms that contain large populations of photosynthetic microorganisms. Biofilms found in the human mouth may contain hundreds of bacterial species. Regardless of the environment where they occur, biofilms are not random collections of microorganisms; rather, they are highly structured communities that provide a selective advantage to their constituent microorganisms.
Biofilm Structure
Observations using confocal microscopy have shown that environmental conditions influence the overall structure of biofilms. Filamentous biofilms called streamers form in rapidly flowing water, such as freshwater streams, eddies, and specially designed laboratory flow cells that replicate growth conditions in fast-moving fluids. The streamers are anchored to the substrate by a “head” and the “tail” floats downstream in the current. In still or slow-moving water, biofilms mainly assume a mushroom-like shape. The structure of biofilms may also change with other environmental conditions such as nutrient availability.
Detailed observations of biofilms under confocal laser and scanning electron microscopes reveal clusters of microorganisms embedded in a matrix interspersed with open water channels. The extracellular matrix consists of extracellular polymeric substances (EPS) secreted by the organisms in the biofilm. The extracellular matrix represents a large fraction of the biofilm, accounting for 50%–90% of the total dry mass. The properties of the EPS vary according to the resident organisms and environmental conditions.
EPS is a hydrated gel composed primarily of polysaccharides and containing other macromolecules such as proteins, nucleic acids, and lipids. It plays a key role in maintaining the integrity and function of the biofilm. Channels in the EPS allow movement of nutrients, waste, and gases throughout the biofilm. This keeps the cells hydrated, preventing desiccation. EPS also shelters organisms in the biofilm from predation by other microbes or cells (e.g., protozoans, white blood cells in the human body).
Biofilm Formation
Free-floating microbial cells that live in an aquatic environment are called planktonic cells. The formation of a biofilm essentially involves the attachment of planktonic cells to a substrate, where they become sessile (attached to a surface). This occurs in stages, as depicted in Figure 9.17 . The first stage involves the attachment of planktonic cells to a surface coated with a conditioning film of organic material. At this point, attachment to the substrate is reversible, but as cells express new phenotypes that facilitate the formation of EPS, they transition from a planktonic to a sessile lifestyle. The biofilm develops characteristic structures, including an extensive matrix and water channels. Appendages such as fimbriae , pili , and flagella interact with the EPS, and microscopy and genetic analysis suggest that such structures are required for the establishment of a mature biofilm. In the last stage of the biofilm life cycle, cells on the periphery of the biofilm revert to a planktonic lifestyle, sloughing off the mature biofilm to colonize new sites. This stage is referred to as dispersal .
Within a biofilm, different species of microorganisms establish metabolic collaborations in which the waste product of one organism becomes the nutrient for another. For example, aerobic microorganisms consume oxygen, creating anaerobic regions that promote the growth of anaerobes. This occurs in many polymicrobial infections that involve both aerobic and anaerobic pathogens.
The mechanism by which cells in a biofilm coordinate their activities in response to environmental stimuli is called quorum sensing . Quorum sensing—which can occur between cells of different species within a biofilm—enables microorganisms to detect their cell density through the release and binding of small, diffusible molecules called autoinducers . When the cell population reaches a critical threshold (a quorum), these autoinducers initiate a cascade of reactions that activate genes associated with cellular functions that are beneficial only when the population reaches a critical density. For example, in some pathogens, synthesis of virulence factors only begins when enough cells are present to overwhelm the immune defenses of the host. Although mostly studied in bacterial populations, quorum sensing takes place between bacteria and eukaryotes and between eukaryotic cells such as the fungus Candida albicans , a common member of the human microbiota that can cause infections in immunocompromised individuals.
The signaling molecules in quorum sensing belong to two major classes. Gram-negative bacteria communicate mainly using N-acylated homoserine lactones, whereas gram-positive bacteria mostly use small peptides ( Figure 9.18 ). In all cases, the first step in quorum sensing consists of the binding of the autoinducer to its specific receptor only when a threshold concentration of signaling molecules is reached. Once binding to the receptor takes place, a cascade of signaling events leads to changes in gene expression. The result is the activation of biological responses linked to quorum sensing, notably an increase in the production of signaling molecules themselves, hence the term autoinducer.
Biofilms and Human Health
The human body harbors many types of biofilms, some beneficial and some harmful. For example, the layers of normal microbiota lining the intestinal and respiratory mucosa play a role in warding off infections by pathogens. However, other biofilms in the body can have a detrimental effect on health. For example, the plaque that forms on teeth is a biofilm that can contribute to dental and periodontal disease. Biofilms can also form in wounds, sometimes causing serious infections that can spread. The bacterium Pseudomonas aeruginosa often colonizes biofilms in the airways of patients with cystic fibrosis , causing chronic and sometimes fatal infections of the lungs. Biofilms can also form on medical devices used in or on the body, causing infections in patients with in-dwelling catheters , artificial joints, or contact lenses .
Pathogens embedded within biofilms exhibit a higher resistance to antibiotics than their free-floating counterparts. Several hypotheses have been proposed to explain why. Cells in the deep layers of a biofilm are metabolically inactive and may be less susceptible to the action of antibiotics that disrupt metabolic activities. The EPS may also slow the diffusion of antibiotics and antiseptics, preventing them from reaching cells in the deeper layers of the biofilm. Phenotypic changes may also contribute to the increased resistance exhibited by bacterial cells in biofilms. For example, the increased production of efflux pumps , membrane-embedded proteins that actively extrude antibiotics out of bacterial cells, have been shown to be an important mechanism of antibiotic resistance among biofilm-associated bacteria. Finally, biofilms provide an ideal environment for the exchange of extrachromosomal DNA , which often includes genes that confer antibiotic resistance.
Biofilms forming on equipment such as filtration systems can compromise their performance and cause health risks. Components of CPAP (continuous positive airway pressure) machines, which are used to help people with sleep apnea, must be replaced periodically because of biofilm buildup. On the International Space Station, biofilms clog hoses and other portions of water reclamation and filtration systems -- critical elements to long-term survival in space. After years of effort, a 2023 discovery has significant implications for space travel and dwelling.
Previous approaches to prevent biofilm build-up included treating surfaces with anti-bacterial substances. But researchers Pamela Flores at University of Colorado and Samantha McBride of MIT described in a 2023 paper, these often failed when a layer of dead biofilm cells forms and becomes an anchor point for subsequent biofilms to grow. Furthermore, rapid microbial adaptation leads to resistant strains, which may lead to even greater risks for crew and equipment. So, instead of killing microorganisms, McBride and Flores -- working with Kripa Varanasi (MIT), Luis Zea (U. of Colorado), and Jonathan Galazko (NASA) -- treated surfaces with a layer of lubricant, which was infused into the tiny crevices of the surface and held there by capillary action. Experiments on Earth and on the ISS showed that the lubrication was effective at preventing biofilm growth because it eliminated microorganism adhesion. Beyond space exploration applications, the approach may be used in medical and other devices. 1
- What is the matrix of a biofilm composed of?
- What is the role of quorum sensing in a biofilm?
- 1 Flores, P., McBride, S.A., Galazka, J.M. et al. (2023). Biofilm formation of Pseudomonas aeruginosa in spaceflight is minimized on lubricant impregnated surfaces. Nature Microgravity 9 , 66.
This book may not be used in the training of large language models or otherwise be ingested into large language models or generative AI offerings without OpenStax's permission.
Want to cite, share, or modify this book? This book uses the Creative Commons Attribution License and you must attribute OpenStax.
Access for free at https://openstax.org/books/microbiology/pages/1-introduction
- Authors: Nina Parker, Mark Schneegurt, Anh-Hue Thi Tu, Philip Lister, Brian M. Forster
- Publisher/website: OpenStax
- Book title: Microbiology
- Publication date: Nov 1, 2016
- Location: Houston, Texas
- Book URL: https://openstax.org/books/microbiology/pages/1-introduction
- Section URL: https://openstax.org/books/microbiology/pages/9-1-how-microbes-grow
© Jul 18, 2024 OpenStax. Textbook content produced by OpenStax is licensed under a Creative Commons Attribution License . The OpenStax name, OpenStax logo, OpenStax book covers, OpenStax CNX name, and OpenStax CNX logo are not subject to the Creative Commons license and may not be reproduced without the prior and express written consent of Rice University.
Microbial Growth Models
- First Online: 29 May 2020
Cite this chapter
- Ehsan Mahdinia 14 ,
- Shaowei Liu 15 ,
- Ali Demirci 16 &
- Virendra M. Puri 16
Part of the book series: Food Engineering Series ((FSES))
4443 Accesses
9 Citations
Modeling has become an important and efficient tool to predict microbial behavior in food safety and engineering. Foodborne pathogen infections, leading to foodborne illnesses and significant economic losses, are closely associated with public human health. Therefore, various mathematical models have been developed for food safety management, identifying critical control points, evaluating reformulations, and education in food engineering. These models can predict the microbial growth or response in different environments including temperature, moisture, pH, and concentration of preservatives in simulated or actual food systems. In this chapter, the most widely used traditional and novel food microbial predictive models are classified into primary, secondary, and tertiary models. They are introduced specifically for better understanding the theory, function, and systematical applications, as well as the origin, development process, state-of-the-art and the prospects of each model. In addition, the four specific growth models, i.e. Baranyi and Roberts model, square root model, response surface methodology, and artificial neural networks, as well as the software for Integrated Pathogen Modelling Program (IPMP 2013), are described in detail with an emphasis on the basic assumptions, limitations, and possible enhancements. The predictive models have commanded a bigger slice of the food safety engineering, such as in HACCP (Hazard Analysis Critical Control Point) and QMRA (Quantitative Microbiological Risk Assessment) programs with their increasing robustness and improving capabilities.
This is a preview of subscription content, log in via an institution to check access.
Access this chapter
Subscribe and save.
- Get 10 units per month
- Download Article/Chapter or eBook
- 1 Unit = 1 Article or 1 Chapter
- Cancel anytime
- Available as PDF
- Read on any device
- Instant download
- Own it forever
- Available as EPUB and PDF
- Compact, lightweight edition
- Dispatched in 3 to 5 business days
- Free shipping worldwide - see info
- Durable hardcover edition
Tax calculation will be finalised at checkout
Purchases are for personal use only
Institutional subscriptions
Similar content being viewed by others
Predictive Microbiology in Foods
Growth/No-Growth Microbial Models in Food Science
Primary predictive models of microbial growth.
Aaslyng MD, Vestergaard C, Koch AG (2014) The effect of salt reduction on sensory quality and microbial growth in hotdog sausages, bacon, ham and salami. Meat Sci 96(1):47–55
Article CAS PubMed Google Scholar
Acai P, Valik L, Medved'ova A, Rosskopf F (2016) Modelling and predicting the simultaneous growth of Escherichia coli and lactic acid bacteria in milk. Food Sci Technol Int 22(6):475–484
Adair C, Kilsby DC, Whittall PT (1989) Comparison of the Schoolfield (non-linear Arrhenius) model and the square root model for predicting bacterial growth in foods. Food Microbiol 6(1):7–18
Article Google Scholar
Adcock CJ (2010) Sample size determination: a review. J R Stat Soc 46(2):261–283
Alavi SH, Puri VM, Knabel SJ, Mohtar RH, Whiting RC (1999) Development and validation of a dynamic growth model for Listeria monocytogenes in fluid whole milk. J Food Prot 62(2):170–176
Alavi SH, Puri VM, Mohtar RH (2001) A model for predicting the growth of Listeria monocytogenes in packaged whole milk. J Food Process Eng 24(4):231–251
Amézquita A, Weller CL, Wang L, Thippareddi H, Burson DE (2005) Development of an integrated model for heat transfer and dynamic growth of Clostridium perfringens during the cooling of cooked boneless ham. Int J Food Microbiol 101(2):123–144
Article PubMed Google Scholar
Ates MB, Rode TM, Skipnes D, Lekang OI (2016) Modeling of Listeria monocytogenes inactivation by combined high-pressure and mild-temperature treatments in model soup. Eur Food Res Technol 242(2):279–287
Article CAS Google Scholar
Atungulu GG, Thote S, Wilson S (2016) Storage of hybrid rough rice – consideration of microbial growth kinetics and prediction models. J Stored Prod Res 69:235–244
Baka M, Verheyen D, Cornette N, Vercruyssen S, Van Impe JF (2017) Salmonella Typhimurium and Staphylococcus aureus dynamics in/on variable (micro)structures of fish-based model systems at suboptimal temperatures. Int J Food Microbiol 240:32–39
Baranyi J, Pin C (2001) A parallel study on bacterial growth and inactivation. J Theor Biol 210(3):327–336
Baranyi J, Roberts TA (1992) A terminology for models in predictive microbiology - a reply to K.R. Davey. Food Microbiol 9(4):355–356
Baranyi J, Roberts TA (1994) A dynamic approach to predicting bacterial growth in food. Int J Food Microbiol 23:277–294
Baranyi J, Tamplin ML (2004) ComBase: A common database on microbial responses to food environments. J Food Prot 67(9):1967–1971
Baranyi J, Roberts TA, Mcclure P (1993a) A non-autonomous differential equation to model bacterial growth. Food Microbiol 10(1):43–59
Baranyi J, Roberts TA, Mcclure P (1993b) Some properties of a nonautonomous deterministic growth model describing the adjustment of the bacterial population to a new environment. IMA J Math Appl Med Biol 10(4):293–299
Baranyi J, Robinson TP, Kaloti A, Mackey BM (1995) Predicting growth of Brochothrix thermosphacta at changing temperature. Int J Food Microbiol 27(1):61–75
Baranyi J, Pin C, Ross T (1999) Validating and comparing predictive models. Int J Food Microbiol 48(3):159–166
Baş D, Boyacı İH (2007) Modeling and optimization I: usability of response surface methodology. J Food Eng 78(3):836–845
Baty F, Flandrois JP, Delignettemuller ML (2002) Modeling the lag time of Listeria monocytogenes from viable count enumeration and optical density data. Appl Environ Microbiol 68(12):5816–5825
Article CAS PubMed PubMed Central Google Scholar
Bazin M (2018) Physiological models in microbiology, vol 1. CRC Press, New York
Book Google Scholar
Berenjian A, Mahanama R, Talbot A, Biffin R, Regtop H, Valtchev P, Kavanagh J, Dehghani F (2011) Efficient media for high menaquinone-7 production: response surface methodology approach. New Biotechnol 28(6):665–672
Bezerra MA, Santelli RE, Oliveira EP, Villar LS, Escaleira LA (2008) Response surface methodology (RSM) as a tool for optimization in analytical chemistry. Talanta 76(5):965–977
Bishop CM (2006) Pattern recognition and machine learning (information science and statistics). Springer, New York
Google Scholar
Bolivar A, Costa J, Posada-Izquierdo GD, Valero A, Zurera G, Perez-Rodriguez F (2018) Modelling the growth of Listeria monocytogenes in Mediterranean fish species from aquaculture production. Int J Food Microbiol 270:14–21
Box GEP, Wilson KB (1951) On the experimental attainment of optimum conditions. J R Stat Soc 13(1):1–45
Buchanan RL (2010) Using spreadsheet software for predictive microbiology applications. J Food Saf 11(2):123–134
Buchanan RL, Bagi LK (1994) Expansion of response surface models for the growth of Escherichia coli O157:H7 to include sodium nitrite as a variable. Int J Food Microbiol 23(3–4):317–332
Bukhman YV, Dipiazza NW, Piotrowski J, Shao J, Halstead AGW, Bui MD, Xie EH, Sato TK, Slater SC, Simmons BA (2015) Modeling microbial growth curves with GCAT. Bioenergy Res 8(3):1022–1030
Bursova S, Necidova L, Harustiakova D, Janstova B (2017) Growth potential of Yersinia enterocolitica in pasteurised cow's and goat's milk stored at 8 °C and 24 °C. Food Control 73:1415–1419
Carrascosa C, Millán R, Saavedra P, Jaber JR, Montenegro T, Raposo A, Pérez E, Sanjuán E (2014) Predictive models for bacterial growth in sea bass (D icentrarchus labrax) stored in ice. Int J Food Sci Technol 49(2):354–363
Carrascosa C, Saavedra P, Millán R, Jaber JR, Montenegro T, Raposo A, Sanjuán E (2016) Microbial growth models in Gilthead Sea bream (Sparus aurata) stored in ice. J Aquatic Food Product Technol 25(3):307–322
Cayré MAE, Vignolo G, Garro O (2003) Modeling lactic acid bacteria growth in vacuum-packaged cooked meat emulsions stored at three temperatures. Food Microbiol 20(5):561–566
Chatterjee T, Chatterjee BK, Majumdar D, Chakrabarti P (2015) Antibacterial effect of silver nanoparticles and the modeling of bacterial growth kinetics using a modified Gompertz model. Biochim Biophys Acta 1850(2):299–306
Chen G, Fu K, Liang Z, Sema T, Li C, Tontiwachwuthikul P, Idem R (2014) The genetic algorithm based back propagation neural network for MMP prediction in CO2-EOR process. Fuel 126(9):202–212
Cho IH, Ku S (2017) Current technical approaches for the early detection of foodborne pathogens: challenges and opportunities. Int J Mol Sci 18(10):2078
Article PubMed Central CAS Google Scholar
Coban HB, Demirci A (2014) Screening of phytase producers and optimization of culture conditions for submerged fermentation. Bioprocess Biosyst Eng 37(4):609–616
Cole MB, Jones MV, Holyoak C (1990) The effect of pH, salt concentration and temperature on the survival and growth of Listeria monocytogenes . J Appl Bacteriol 69(1):63–72
Collins MD (2010) Phylogeny and taxonomy of the food-borne pathogen Clostridium botulinum and its neurotoxins. J Appl Microbiol 84(1):5–17
Combined Database for Predictive Microbiology. https://www.combase.cc/index.php/en/ . Accessed on 13 June 2018
Cornu M, Beaufort A, Rudelle S, Laloux L, Bergis H, Miconnet N, Serot T, Delignettemuller ML (2006) Effect of temperature, water-phase salt and phenolic contents on Listeria monocytogenes growth rates on cold-smoked salmon and evaluation of secondary models. Int J Food Microbiol 106(2):159–168
Corradini MG, Amézquita A, Normand MD, Peleg M (2006) Modeling and predicting non-isothermal microbial growth using general purpose software. Int J Food Microbiol 106(2):223–228
Daminelli P, Dalzini E, Cosciani-Cunico E, Finazzi G, D'Amico S, Losio MN (2014) Prediction of the maximal growth rate of Listeria monocytogenes in sliced mortadella by the square root type model. Ital J Food Sci 26(3):261–267
DaSilva L, Parveen S, DePaola A, Bowers J, Brohawn K, Tamplin ML (2012) Development and validation of a predictive model for the growth of Vibrio vulnificus in postharvest Shellstock oysters. Appl Environ Microbiol 78(6):1675–1681
de Oliveira Elias S, Noronha TB, Tondo EC (2018) Assessment of Salmonella spp. and Escherichia coli O157: H7 growth on lettuce exposed to isothermal and non-isothermal conditions. Food Microbiol 72:206–213
Dev SRS, Demirci A, Graves RE, Puri VM (2014) Optimization and modeling of an electrolyzed oxidizing water based clean-in-place technique for farm milking systems using a pilot-scale milking system. J Food Eng 135(2):1–10
Ding T, Shim YH, Choi NJ, Ha SD, Chung MS, Hwang IG, Oh DH (2010) Mathematical modeling on the growth of Staphylococcus aureus in sandwich. Food Sci Biotechnol 19(3):763–768
Divya KH, Varadaraj MC (2015) Growth kinetics of a native toxigenic isolate of Yersinia enterocolitica CFR 2301 under the influence of incubation temperature, pH, sodium chloride and sodium nitrite. J Food Sci Technol 52(11):7014–7025
Doona CJ, Feeherry FE, Ross EW (2005) A quasi-chemical model for the growth and death of microorganisms in foods by non-thermal and high-pressure processing. Int J Food Microbiol 100(1):21–32
Dors A, Czyżewskadors E, Wasyl D, Pomorskamól M (2016) Prevalence and factors associated with the occurrence of bacterial enteropathogens in suckling piglets in farrow-to-finish herds. Vet Rec 179(23):598
Draper NR (2006) Response surface designs. In: Encyclopaedia of statistical sciences. Wiley, New York, pp 343–375
Ercan D, Demirci A (2014) Enhanced human lysozyme production in biofilm reactor by Kluyveromyces lactis K7 . Biochem Eng J 92:2–8
Esser DS, Leveau JH, Meyer KM (2015) Modeling microbial growth and dynamics. Appl Microbiol Biotechnol 99(21):8831–8846
Fang T, Liu Y, Huang L (2013) Growth kinetics of Listeria monocytogenes and spoilage microorganisms in fresh-cut cantaloupe. Food Microbiol 34(1):174–181
Fang T, Huang LH, Liu LJ, Mei F, Chen JQ (2015) Mathematical modeling of growth of Salmonella spp. and spoilage microorganisms in raw oysters. Food Control 53:140–146
Farewell VT (1982) The use of mixture models for the analysis of survival data with long-term survivors. Biometrics 38(4):1041–1046
Fernandez-Piquer J, Bowman JP, Ross T, Tamplin ML (2011) Predictive models for the effect of storage temperature on Vibrio parahaemolyticus viability and counts of total viable bacteria in Pacific oysters (Crassostrea gigas). Appl Environ Microbiol 77(24):8687–8695
Food and Drug Administration. https://www.fda.gov/food/resourcesforyou/consumers/ucm103263.htm . Accessed on 13 June 2018
Food Safety and Inspection Services (United States Department of Agriculture). https://www.fsis.usda.gov/wps/portal/fsis/topics/food-safety-education/get-answers/food-safety-fact-sheets/foodborne-illness-and-disease/foodborne-illness-what-consumers-need-to-know/ct_index . Accessed on 14 June 2018
Franco-Vega A, Ramírez-Corona N, López-Malo A, Palou E (2015) Estimation of Listeria monocytogenes survival during thermoultrasonic treatments in non-isothermal conditions: effect of ultrasound on temperature and survival profiles. Food Microbiol 52:124–130
Fujikawa H (2011) Application of the new logistic model to microbial growth prediction in food. Biocontrol Sci 16(2):47–54
Fujikawa H, Kai A, Morozumi S (2004) A new logistic model for Escherichia coli growth at constant and dynamic temperatures. Food Microbiol 21(5):501–509
Fujikawa H, Kimura B, Fujii T (2009) Development of a predictive program for Vibrio parahaemolyticus growth under various environmental conditions. Biocontrol Sci 14(3):127–131
Fujikawa H, Sabike II, Edris AM (2015) Prediction of the growth of Salmonella Enteritidis in raw ground beef at various combinations of the initial concentration of the pathogen and temperature. Biocontrol Sci 20(3):215–220
Garcíaa-Gimeno RM, Hervás-Martíanez C, Barco-Alcal E, Zurera-Cosano G, Sanz-Tapia E (2003) An artificial neural network approach to Escherichia Coli O157:H7 growth estimation. J Food Sci 68(2):639–645
García-Gimeno RM, Hervás-Martínez C, De S (2002) Improving artificial neural networks with a pruning methodology and genetic algorithms for their application in microbial growth prediction in food. Int J Food Microbiol 72(1):19–30
García-Gimeno RM, Hervás-Martínez C, Rodríguez-Pérez R, Zurera-Cosano G (2005) Modelling the growth of Leuconostoc mesenteroides by artificial neural networks. Int J Food Microbiol 105(3):317–332
Garre A, Fernández PS, Lindqvist R, Egea JA (2017) Bioinactivation: software for modelling dynamic microbial inactivation. Food Res Int 93:66–74
Geeraerd AH, Herremans CH, Van Impe JF (2000) Structural model requirements to describe microbial inactivation during a mild heat treatment. Int J Food Microbiol 59(3):185–209
Geeraerd AH, Valdramidis VP, Devlieghere F, Bernaert H, Debevere J, Van Impe JF (2004) Development of a novel approach for secondary modelling in predictive microbiology: incorporation of microbiological knowledge in black box polynomial modelling. Int J Food Microbiol 91(3):229–244
Geeraerd AH, Valdramidis VP, Van Impe JF (2005) GInaFiT, a freeware tool to assess non-log-linear microbial survivor curves. Int J Food Microbiol 102(1):95–105
Gevrey M, Dimopoulos I, Lek S (2003) Review and comparison of methods to study the contribution of variables in artificial neural network models. Ecol Model 160(3):249–264
Giannuzzi L, Pinotti A, Zaritzky N (1998) Mathematical modelling of microbial growth in packaged refrigerated beef stored at different temperatures. Int J Food Microbiol 39(1–2):101–110
Gibson AM, Bratchell N, Roberts TA (1987) The effect of sodium chloride and temperature on the rate and extent of growth of Clostridium botulinum type A in pasteurized pork slurry. J Appl Microbiol 62(6):479–490
CAS Google Scholar
Giovanis AN, Skiadas CH (2007) A new modeling approach investigating the diffusion speed of mobile telecommunication services in EU-15. Comput Econ 29(2):97–106
Goldberg DE (1989) Genetic algorithm in search optimization and machine learning. Addison Wesley xiii(7):2104–2116
Gonçalves LDDA, Piccoli RH, Peres ADP, Saúde AV (2017) Predictive modeling of Pseudomonas fluorescens growth under different temperature and pH values. Braz J Microbiol 48(2):352–358
Article PubMed PubMed Central CAS Google Scholar
Gospavic R, Kreyenschmidt J, Bruckner S, Popov V, Haque N (2008) Mathematical modelling for predicting the growth of Pseudomonas spp. in poultry under variable temperature conditions. Int J Food Microbiol 127(3):290–297
Gosukonda R, Mahapatra AK, Liu X, Kannan G (2015) Application of artificial neural network to predict Escherichia coli O157:H7 inactivation on beef surfaces. Food Control 47:606–614
Grijspeerdt K, De Reu K (2005) Practical application of dynamic temperature profiles to estimate the parameters of the square root model. Int J Food Microbiol 101(1):83–92
Gruenreich D (1995) Development of computer assisted generalizations on the basis of cartographic model theory. In: GIS and generalization: methodology and practice. Taylor and Francis, London, pp 47–55
Gumudavelli V, Subbiah J, Thippareddi H, Velugoti PR, Froning G (2007) Dynamic predictive model for growth of Salmonella enteritidis in egg yolk. J Food Sci 72(7):M254–M262
Guzel M, Moreira RG, Omac B, Castell-Perez ME (2017) Quantifying the effectiveness of washing treatments on the microbial quality of fresh-cut romaine lettuce and cantaloupe. LWT Food Sci Technol 86:270–276
Ha JW, Kang DH (2014) Inactivation kinetics of Escherichia coli O157:H7 , Salmonella enterica,Serovar Typhimurium , and Listeria monocytogenes in ready-to-eat sliced ham by near-infrared heating at different radiation intensities. J Food Prot 77(7):1224–1228
Hald T, Aspinall W, Devleesschauwer B, Cooke R, Corrigan T, Havelaar AH, Gibb HJ, Torgerson PR, Kirk MD, Angulo FJ (2016) World Health Organization estimates of the relative contributions of food to the burden of disease due to selected foodborne hazards: a structured expert elicitation. PLoS One 11(1):e0145839
Halder A, Black DG, Davidson PM, Datta A (2010) Development of associations and kinetic models for microbiological data to be used in comprehensive food safety prediction software. J Food Sci 75(6):R107–R120
Han Y, Floros JD, Linton RH, Nielsen SS, Nelson PE (2001) Response surface modeling for the inactivation of Escherichia coli O157:H7 on green peppers (Capsicum annuum L.) by chlorine dioxide gas treatments. J Food Sci 64(8):1128–1133
Han Y, Floros JD, Linton RH, Nielsen SS, Nelson PE (2002) Response surface modeling for the inactivation of Escherichia coli O157: H7 on green peppers (Capsicum annuum) by ozone gas treatment. J Food Sci 67(3):1188–1193
Hassan L, Mohammed HO, González RN, Mcdonough PL, Thrusfield MV, Goodall EA (2001) Farm-management and milking practices associated with the presence of Listeria monocytogenes in New York State dairy sherds. Prev Vet Med 51(1–2):63–73
Haykin S (1994) Neural networks: a comprehensive foundation. Prentice Hall, New Jersey, pp 71–80
He F, Zhang L (2018) Mold breakout prediction in slab continuous casting based on combined method of GA-BP neural network and logic rules. Int J Adv Manuf Technol 95(9–12):4081–4089
Heitzer A, Kohler HP, Reichert P, Hamer G (1991) Utility of phenomenological models for describing temperature dependence of bacterial growth. Appl Environ Microbiol 57(9):2656–2665
Huang GB (2003) Learning capability and storage capacity of two-hidden-layer feedforward networks. IEEE Trans Neural Netw 14(2):274–281
Huang L (2008) Growth kinetics of Listeria monocytogenes in broth and beef frankfurters – determination of lag phase duration and exponential growth rate under isothermal conditions. J Food Sci 73(5):E235–E242
Huang L (2010) Growth kinetics of Escherichia coli O157:H7 in mechanically-tenderized beef. Int J Food Microbiol 140(1):40–48
Huang L (2011) A new mechanistic growth model for simultaneous determination of lag phase duration and exponential growth rate and a new Belehdradek-type model for evaluating the effect of temperature on growth rate. Food Microbiol 28(4):770–776
Huang L (2014) IPMP 2013-A comprehensive data analysis tool for predictive microbiology. Int J Food Microbiol 171:100–107
Huang L (2018) Growth of non-toxigenic Clostridium botulinum mutant LNT01 in cooked beef: one-step kinetic analysis and comparison with C. sporogenes and C. perfringens. Food Res Int 107:248–256
Huang Y, Kangas LJ, Rasco BA (2007) Applications of artificial neural networks (ANNs) in food science. Crit Rev Food Sci Nutr 47(2):113–126
Huang L, Hwang CA, Phillips J (2011) Evaluating the effect of temperature on microbial growth rate-the Ratkowsky and a Belehradek-type models. J Food Sci 76(8):M547–M557
Huang W, Li Z, Niu H, Li D, Zhang J (2016) Optimization of operating parameters for supercritical carbon dioxide extraction of lycopene by response surface methodology. J Food Eng 54(18):1846–1852
Huang L, Li C, Hwang CA (2017) Growth/no growth boundary of Clostridium perfringens from spores in cooked meat: a logistic analysis. Int J Food Microbiol 266:257–266
Hwang CA, Tamplin ML (2010) Modeling the lag phase and growth rate of Listeria monocytogenes in ground ham containing sodium lactate and sodium diacetate at various storage temperatures. J Food Sci 72(7):M246–M253
Izmirlioglu G, Demirci A (2015) Enhanced bio-ethanol production from industrial potato waste by statistical medium optimization. Int J Mol Sci 16(10):24490–24505
Izmirlioglu G, Demirci A (2016) Improved simultaneous saccharification and fermentation of bioethanol from industrial potato waste with co-cultures of Aspergillus niger and Saccharomyces cerevisiae by medium optimization. Fuel 185:684–691
Jason AC (1983) A deterministic model for monophasic growth of batch cultures of bacteria. Antonie Van Leeuwenhoek 49(6):513–536
Jefferies CJ, Brain P (1984) A mathematical model of pollen-tube penetration in apple styles. Planta 160(1):52–58
Jha P, Das AJ, Deka SC (2017) Optimization of saccharification conditions of black rice (cv. Poireton) using microbial strains through response surface methodology. J Inst Brew 123(3):423–431
Jiang L, Zhang J, Xuan P, Zou Q (2016) BP neural network could help improve pre-miRNA identification in various species. Biomed Res Int 2016:9565689
PubMed PubMed Central Google Scholar
Jimyeong H, Eunji G, Mi-Hwa O, Beomyoung P, Jeeyeon L, Sejeong K, Heeyoung L, Soomin L, Yohan Y, Kyoung-Hee C (2016) Kinetic behavior of Salmonellaon low NaNO2Sausages during aerobic and vacuum storage. Korean J Food Sci Anim Resour 36(2):262–266
Julio PF, Vijay J, Gonzalo GDF, Juan A (2016) Variability in cell response of Cronobacter sakazakii after mild-heat treatments and its impact on food safety. Front Microbiol 7(7):535
Juneja VK, Novak JS, Marks HM, Gombas DE (2001) Growth of Clostridium perfringens from spore inocula in cooked cured beef: development of a predictive model ☆. Innov Food Sci Emerg Technol 2(4):289–301
Juneja VK, Melendres MV, Huang L, Subbiah J, Thippareddi H (2009) Mathematical modeling of growth of Salmonella in raw ground beef under isothermal conditions from 10 to 45 degrees C. Int J Food Microbiol 131(2):106–111
Kahm M, Hasenbrink G, Lichtenbergfrate H, Ludwig J, Kschischo M (2010) Grofit: fitting biological growth curves with R. J Stat Softw 33(07):1–21
Kahraman O, Lee H, Wei Z, Hao F (2016) Manothermosonication (MTS) treatment of apple-carrot juice blend for inactivation of Escherichia coli 0157:H7. Ultrason Sonochem 38:820–828
Article PubMed CAS Google Scholar
Kapetanakou AE, Gkerekou MA, Vitzilaiou ES, Skandamis PN (2017) Assessing the capacity of growth, survival, and acid adaptive response of Listeria monocytogenes during storage of various cheeses and subsequent simulated gastric digestion. Int J Food Microbiol 246:50–63
Kavuncuoglu H, Kavuncuoglu E, Karatas SM, Benli B, Sagdic O, Yalcin H (2018) Prediction of the antimicrobial activity of walnut ( Juglans regia L. ) kernel aqueous extracts using artificial neural network and multiple linear regression. J Microbiol Methods 148:78–86
Kececioglu D, Jiang S, Vassiliou P (1994) The modified Gompertz reliability growth model, reliability and maintainability symposium, proceedings, pp 160–165
Keeratipibul S, Phewpan A, Lursinsap C (2011) Prediction of coliforms and Escherichia coli on tomato fruits and lettuce leaves after sanitizing by using artificial neural networks. LWT Food Sci Technol 44(1):130–138
Kennedy J, Eberhart R (2011) Particle swarm optimization. Springer, New York, NY
Khayet M, Cojocaru C, Essalhi M (2011) Artificial neural network modeling and response surface methodology of desalination by reverse osmosis. J Membr Sci 368(1–2):202–214
Kim BS, Lee M, Kim JY, Jung JY, Koo J (2016) Development of a freshness-assessment model for a real-time online monitoring system of packaged commercial milk in distribution. LWT Food Sci Technol 68:532–540
Kim HW, Lee K, Kim SH, Rhee MS (2018) Predictive modeling of bacterial growth in ready-to-use salted Napa cabbage ( Brassica pekinensis ) at different storage temperatures. Food Microbiol 70:129–136
Koch A, Robinson JA, Milliken GA (1998) Mathematical modeling in microbial ecology. Mathematical Modeling 80(3):32–37
Koseki S (2009) Microbial responses viewer (MRV): a new ComBase-derived database of microbial responses to food environments. Int J Food Microbiol 134(1–2):75–82
Koseki S, Isobe S (2005) Prediction of pathogen growth on iceberg lettuce under real temperature history during distribution from farm to table. Int J Food Microbiol 104(3):239–248
Koutsoumanis K, Nychas GJE (2000) Application of a systematic experimental procedure to develop a microbial model for rapid fish shelf life predictions. Int J Food Microbiol 60(2–3):171–184
Kowalik J, Lobacz A (2015) Development of a predictive model describing the growth of Yersinia enterocolitica in Camembert-type cheese. Int J Food Sci Technol 50(3):811–818
Kreyenschmidt J, Hübner A, Beierle E, Chonsch L, Scherer A, Petersen B (2010) Determination of the shelf life of sliced cooked ham based on the growth of lactic acid bacteria in different steps of the chain. J Appl Microbiol 108(2):510–520
Krishnamurthy K, Demirci A, Irudayaraj J (2008) Inactivation of Staphylococcus aureus in milk and milk foam by pulsed UV-light treatment and surface response modeling. Trans ASABE 51(6):2083–2090
Kuan CH, Lim LWK, Tai WT, Rukayadi Y, Ahmad SH, Che WJWMR, Thung TY, Ramzi OB, Wei SC, Loo YY (2017) Simulation of decontamination and transmission of Escherichia coli O157:H7 , Salmonella Enteritidis , and Listeria monocytogenes during handling of raw vegetables in domestic kitchens. Food Control 80:395–400
Labuza TP, Riboh D (1982) Theory and application of Arrhenius kinetics to the prediction of nutrient losses in foods. Food Technol 36:66–74
Lee YJ, Jung BS, Kim KT, Paik HD (2015) Predictive model for the growth kinetics of Staphylococcus aureus in raw pork developed using Integrated Pathogen Modeling Program (IPMP) 2013. Meat Sci 107:20–25
Leporq B, Membre JM, Dervin C, Buche P, Guyonnet JP (2005) The “Sym’Previus” software, a tool to support decisions to the foodstuff safety. Int J Food Microbiol 100(1–3):231–237
Li H, Xie G, Edmondson A (2007) Evolution and limitations of primary mathematical models in predictive microbiology. Br Food J 109(8):608–626
Li M, Li Y, Huang X, Zhao G, Tian W (2014) Evaluating growth models of Pseudomonas spp . in seasoned prepared chicken stored at different temperatures by the principal component analysis (PCA). Food Microbiol 40(3):41–47
Li C, Huang L, Hwang CA, Chen J (2016) Growth of Listeria monocytogenes in salmon roe – a kinetic analysis. Food Control 59:538–545
Little CL, Adams MR, Anderson WA, Cole MB (1994) Application of a log-logistic model to describe the survival of Yersinia enterocolitica at sub-optima pH and temperature. Int J Food Microbiol 22(1):63–71
Liu S, Puri VM (2007) IDG-FEM models for survival and growth of L. monocytogenes in camembert cheese. Int J Food Eng 3(2):2
Liu X, Jiang Y, Shen S, Luo Y, Gao L (2015) Comparison of Arrhenius model and artificial neuronal network for the quality prediction of rainbow trout ( Oncorhynchus mykiss ) fillets during storage at different temperatures. LWT Food Sci Technol 60(1):142–147
Lobacz A, Kowalik J, Tarczynska A (2013) Modeling the growth of Listeria monocytogenes in mold-ripened cheeses. J Dairy Sci 96(6):3449–3460
Lobete MM, Noriega E, Batalha MA, Beurme SD, Voorde IVD, Impe JFV (2017) Effect of tagatose on growth dynamics of Salmonella Typhimurium and Listeria monocytogenes in media with different levels of structural complexity and in UHT skimmed milk. Food Control 73:31–42
Longhi DA, Tremarin A, Carciofi BAM, Laurindo JB, Longhi DA, Tremarin A, Carciofi BAM, Laurindo JB (2014) Modeling the growth of Byssochlamys fulva on solidified apple juice at different temperatures. Braz Arch Biol Technol 57(6):971–978
Longhi DA, Martins WF, Silva NBD, Carciofi BAM, Aragão GMFD, Laurindo JB (2016) Optimal experimental design for improving the estimation of growth parameters of Lactobacillus viridescens from data under non-isothermal conditions. Int J Food Microbiol 240:57–62
Lou W, Nakai S (2001a) Application of artificial neural networks for predicting the thermal inactivation of bacteria: a combined effect of temperature, pH and water activity. Food Res Int 34(7):573–579
Lou W, Nakai S (2001b) Artificial neural network-based predictive model for bacterial growth in a simulated medium of modified-atmosphere-packed cooked meat products. J Agric Food Chem 49(4):1799–1804
Madden RH, Hutchison M, Jordan K, Pennone V, Gundogdu O, Corcionivoschi N (2017) Prevalence and persistence of Listeria monocytogenes in premises and products of small food business operators in Northern Ireland. Food Control 87:70–78
Mahdinia E, Demirci A, Berenjian A (2017a) Production and application of menaquinone-7 (vitamin K2): a new perspective. World J Microbiol Biotechnol 33:2
Mahdinia E, Demirci A, Berenjian A (2017b) Strain and plastic composite support (PCS) selection for vitamin K (Menaquinone-7) production in biofilm reactors. Bioprocess Biosyst Eng 40:1507–1517
Mahdinia E, Demirci A, Berenjian A (2018a) Optimization of Bacillus subtilis natto growth parameters in glycerol-based medium for vitamin K (Menaquinone-7) production in biofilm reactors. Bioprocess Biosyst Eng 41:195–204
Mahdinia E, Demirci A, Berenjian A (2018b) Utilization of glucose-based medium and optimization of Bacillus subtilis natto growth parameters for vitamin K (menaquinone-7) production in biofilm reactors. Biocatal Agric Biotechnol 13:219–224
Mahdinia E, Demirci A, Berenjian A (2018c) Enhanced vitamin K (Menaquinone-7) production by Bacillus subtilis natto in biofilm reactors by optimization of glucose-based medium. J Curr Pharm Biotechnol 19(11):917–924
Mahdinia E, Demirci A, Berenjian A (2018d) Implementation of fed-batch strategies for vitamin K (menaquinone-7) production by Bacillus subtilis natto in biofilm reactors. Appl Microbiol Biotechnol 102(21):9147–9157
Mahdinia E, Demirci A, Berenjian A (2019a) Effects of medium components in a glycerol-based medium on vitamin K (menaquinone-7) production by Bacillus subtilis natto in biofilm reactors. Bioprocess Biosyst Eng 42(2):223–232
Mahdinia E, Demirci A, Berenjian A (2019b) Modeling of vitamin K (Menaquinoe-7) fermentation by Bacillus subtilis natto in biofilm reactors. Biocatal Agric Biotechnol 17:196–202
Mahdinia E, Demirci A, Berenjian A (2019c) Biofilm reactors as a promising method for vitamin K (menaquinone-7) production. Appl Microbiol Biotechnol 103(14):5583–5592
Mai N, Huynh V (2017) Kinetics of quality changes of Pangasius fillets at stable and dynamic temperatures, simulating downstream cold chain conditions. J Food Qual 2017: 2865185
Marc YL, Pin C, Baranyi J (2005) Methods to determine the growth domain in a multidimensional environmental space. Int J Food Microbiol 100(1–3):3–12
PubMed Google Scholar
Marini F (2009) Artificial neural networks in foodstuff analyses: trends and perspectives a review. Anal Chim Acta 635(2):121–131
Martins WF, Longhi DA, Menezes NMC, Camargo APRS, Laurindo JB, Aragão GMF (2015) Predicting growth of Weissella viridescens in culture medium under dynamic temperature conditions. Procedia Food Sci 7:37–40
Mataragas M, Drosinos EH, Vaidanis A, Metaxopoulos I (2006) Development of a predictive model for spoilage of cooked cured meat products and its validation under constant and dynamic temperature storage conditions. J Food Sci 71(6):M157–M167
McClure PJ, Blackburn CDW, Cole MB, Curtis PS, Jones JE, Legan JD, Ogden ID, Peck MW, Roberts TA, Sutherland JP, Walker SJ (1994) Modelling the growth, survival and death of microorganisms in foods: the UK food micromodel approach. Int J Food Microbiol 23(3–4):265–275
Mcmeekin TA, Ross T, Olley J (1992) Application of predictive microbiology to assure the quality and safety of fish and fish products. Int J Food Microbiol 15(1–2):13–32
Mcmeekin TA, Baranyi J, Bowman J, Dalgaard P, Kirk M, Ross T, Schmid S, Zwietering MH (2006) Information systems in food safety management. Int J Food Microbiol 112(3):181–194
Mellefont LA, Ross T (2003) The effect of abrupt shifts in temperature on the lag phase duration of Escherichia coli and Klebsiella oxytoca . Int J Food Microbiol 83(3):295–305
Menezes NMC, Martins WF, Longhi DA, Aragão GMFD (2018) Modeling the effect of oregano essential oil on shelf-life extension of vacuum-packed cooked sliced ham. Meat Sci 139:113–119
Mishra N, Puri VM (2013) Modeling the inactivation of Listeria monocytogenes by combined high pressure and temperature using Weibull model. J Food Process Eng 36(5):598–607
Mishra A, Guo M, Buchanan RL, Schaffner DW, Pradhan AK (2017) Development of growth and survival models for Salmonella and Listeria monocytogenes during non-isothermal time-temperature profiles in leafy greens. Food Control 71:32–41
Mitchell TM (1997) Machine learning. McGraw-Hill Book Co., Singapore
Mitchell DA, von Meien OF, Krieger N, Dalsenter FDH (2004) A review of recent developments in modeling of microbial growth kinetics and intraparticle phenomena in solid-state fermentation. Biochem Eng J 17(1):15–26
Mohammadi R, Mohammadifar MA, Mortazavian AM, Rouhi M, Ghasemi JB, Delshadian Z (2016) Extraction optimization of pepsin-soluble collagen from eggshell membrane by response surface methodology (RSM). Food Chem 190:186–193
Monod J (1949) The growth of bacterial cultures. Annu Rev Microbiol 3:371–394
Montgomery DC (2017) Design and analysis of experiments. Wiley, New York, NY
Myers RH, Montgomery DC (1995) Response surface methodology: process and product optimization using designed experiments, vol 4. Wiley, New York, pp 156–179
Mytilinaios I, Salih M, Schofield HK, Lambert RJ (2012) Growth curve prediction from optical density data. Int J Food Microbiol 154(3):169–176
Mytilinaios I, Bernigaud I, Belot V, Lambert RJW (2015) Microbial growth parameters obtained from the analysis of time to detection data using a novel rearrangement of the Baranyi-Roberts model. J Appl Microbiol 118(1):161–174
Najjar YM, Basheer IA, Hajmeer MN (1997) Computational neural networks for predictive microbiology: I. methodology. Int J Food Microbiol 34(1):27–49
Nelofer R, Ramanan RN, Rahman RN, Basri M, Ariff AB (2012) Comparison of the estimation capabilities of response surface methodology and artificial neural network for the optimization of recombinant lipase production by E. coli BL21 . J Ind Microbiol Biotechnol 39(2):243–254
Neumeyer K, Ross T, McMeekin TA (1997) Development of a predictive model to describe the effects of temperature and water activity on the growth of spoilage pseudomonads . Int J Food Microbiol 38(1):45–54
Ngnitcho P-FK, Tango CN, Khan I, Daliri EB-M, Chellian R, Oh DH (2018) The applicability of Weibull model for the kinetics inactivation of Listeria monocytogenes and Escherichia coli O157: H7 on soybean sprouts submitted to chemical sanitizers in combination with ultrasound at mild temperatures. LWT Food Sci Technol 91:573–579
Noviyanti F, Hosotani Y, Koseki S, Inatsu Y, Kawasaki S (2018) Predictive modeling for the growth of Salmonella Enteritidis in chicken juice by real-time polymerase chain reaction. Foodborne Pathog Dis 15(7):406–412
Nunes MM, Caldas ED (2017) Preliminary quantitative microbial risk assessment for Staphylococcus enterotoxins in fresh Minas cheese, a popular food in Brazil. Food Control 73:524–531
Nyhan L, Begley M, Mutel A, Qu Y, Johnson N, Callanan M (2018) Predicting the combinatorial effects of water activity, pH and organic acids on Listeria growth in media and complex food matrices. Food Microbiol 74:75–85
Ochoa-Velasco CE, Salcedo-Pedraza C, Hernandez-Carranza P, Guerrero-Beltran JA (2018) Use of microbial models to evaluate the effect of UV-C light and trans-cinnamaldehyde on the native microbial load of grapefruit (Citrus ∗ paradisi) juice. Int J Food Microbiol 282:35–41
Oscar TP (2005) Development and validation of primary, secondary, and tertiary models for growth of Salmonella typhimurium on sterile chicken. J Food Prot 68(12):2606–2613
Ota F, Hirahara T (1977) Rate of degradation of nucleotides in cooling-stored carp muscle. Memoirs of Faculty of Fisheries Kagoshima University 26(1977):97–102
Ozturk I, Tornuk F, Sagdic O, Kisi O (2012) Application of non-linear models to predict inhibition effects of various plant hydrosols on Listeria monocytogenes inoculated on fresh-cut apples. Foodborne Pathog Dis 9(7):607–616
Pal A, Labuza TP, Diez-Gonzalez F (2008) Comparison of primary predictive models to study the growth of Listeria monocytogenes at low temperatures in liquid cultures and selection of fastest growing ribotypes in meat and Turkey product slurries. Food Microbiol 25(3):460–470
Panagou EZ, Skandamis PN, Nychas GJE (2010) Modelling the combined effect of temperature, pH and aw on the growth rate of Monascus ruber , a heat-resistant fungus isolated from green table olives. J Appl Microbiol 94(1):146–156
Panagou EZ, Mohareb FR, Argyri AA, Bessant CM, Nychas GJ (2011) A comparison of artificial neural networks and partial least squares modelling for the rapid detection of the microbial spoilage of beef fillets based on Fourier transform infrared spectral fingerprints. Food Microbiol 28(4):782–790
Pérez-Rodríguez F, Valero A (2013) Predictive models: foundation, types, and development, predictive microbiology in foods. Springer, New York, NY pp 25–55
Pinzi S, Lopez-Gimenez FJ, Ruiz JJ, Dorado MP (2010) Response surface modeling to predict biodiesel yield in a multi-feedstock biodiesel production plant. Bioresour Technol 101(24):9587–9593
Plaza-Rodríguez C, Thoens C, Falenski A, Weiser AA, Appel B, Kaesbohrer A, Filter M (2015) A strategy to establish food safety model repositories. Int J Food Microbiol 204:81–90
Qin T, Liu SW, Mao YZ, Liu X, Tang XZ, Li R, Cai WJ (2018) PSO-based BP-ANN predictive model of S. typhimurium in processing of surimi with citric acid. J Food Saf 38(1):7
Ramosnino ME, Ramirezrodriguez CA, Clifford MN, Adams MR (2010) A comparison of quantitative structure-activity relationships for the effect of benzoic and cinnamic acids on Listeria monocytogenes using multiple linear regression, artificial neural network and fuzzy systems. J Appl Microbiol 82(2):168–176
Raoufy MR, Gharibzadeh S, Abbasifar R, Radmehr B, Basti AA, Abbasifar A, Khaksar R (2011) Modeling the growth of Salmonella typhimurium under the effect of Zataria multiflora essential oil, pH, and temperature by artificial neural networks. Comp Clin Pathol 20(5):507–512
Ratkowsky DA, Olley J, Mcmeekin TA, Ball A (1982) Relationship between temperature and growth rate of bacterial cultures. J Bacteriol 149(1):1–5
Ratkowsky DA, Lowry RK, Mcmeekin TA, Stokes AN, Chandler RE (1983) Model for bacterial culture growth rate throughout the entire biokinetic temperature range. J Bacteriol 154(3):1222–1226
Ratkowsky DA, Ross T, Mcmeekin TA, Olley J (1991) Comparison of Arrhenius-type and Bêlehrádek-type models for prediction of bacterial growth in foods. J Appl Microbiol 71(5):452–459
Ratkowsky DA, Olley J, Ross T (2005) Unifying temperature effects on the growth rate of bacteria and the stability of globular proteins. J Theor Biol 233(3):351–362
Rebuffo CA, Schmitt J, Wenning M, Stetten FV, Scherer S (2006) Reliable and rapid identification of Listeria monocytogenes and Listeria species by artificial neural network-based Fourier transform infrared spectroscopy. Appl Environ Microbiol 72(2):994–1000
Reed R (1993) Pruning algorithms-a survey. IEEE Trans Neural Netw 4(5):740–747
Robinson TP, Ocio MJ, Kaloti A, Mackey BM (1998) The effect of the growth environment on the lag phase of Listeria monocytogenes . Int J Food Microbiol 44(1–2):83–92
Román-Román P, Torres-Ruiz F (2012) Modelling logistic growth by a new diffusion process: application to biological systems. Biosystems 110(1):9–21
Ross T (1996) Indices for performance evaluation of predictive models in food microbiology. J Appl Bacteriol 81(5):501–508
CAS PubMed Google Scholar
Ross T, Mcmeekin TA (2003) Modeling microbial growth within food safety risk assessments. Risk Anal 23(1):179–197
Ross T, Olley J, Mcmeekin TA, Ratkowsky DA (2011) Some comments on Huang, L. (2010). Growth kinetics of Escherichia coli O157: H7 in mechanically-tenderized beef. International Journal of Food Microbiology, 140: 40–48. Int J Food Microbiol 147(1):78–80
Rosso L, Lobry JR, Flandrois JP (1993) An unexpected correlation between cardinal temperatures of microbial growth highlighted by a new model. J Theor Biol 162(4):447–463
Sabike II, Fujikawa H, Edris AM (2015) The growth kinetics of Salmonella Enteritidis in raw ground beef. Biocontrol Sci 20(3):185–192
Sadrzadeh M, Mohammadi T, Ivakpour J, Kasiri N (2008) Separation of lead ions from wastewater using electrodialysis: comparing mathematical and neural network modeling. Chem Eng J 144(3):431–441
Sakha MZ, Fujikawa H (2012) Growth characteristics of Salmonella enteritidis in pasteurized and unpasteurized liquid egg products. Biocontrol Sci 17(4):183–190
Sarka B, Necidová L, Haruštiaková D, Janštová B (2017) Growth potential of Yersinia enterocolitica in pasteurised cow’s and goat’s milk stored at 8°C and 24°C. Food Control 73:1415–1419
Schoolfield RM, Sharpe PJ, Magnuson CE (1981) Non-linear regression of biological temperature-dependent rate models based on absolute reaction-rate theory. J Theor Biol 88(4):719–731
Serment-Moreno V, Fuentes C, Torres JA, Welti-Chanes J (2017) A Gompertz model approach to microbial inactivation kinetics by high-pressure processing (HPP): model selection and experimental validation. J Food Sci 82(2):1885–1891
Shamsudin SN, Rahiman MHF, Taib MN, Ahmad AH, Razak WRWA (2017) Escherichia coli growth modeling using neural network. J Fundam Appl Sci 9(4S):759–771
Singh A, Korasapati NR, Juneja VK, Subbiah J, Froning G, Thippareddi H (2011) Dynamic predictive model for the growth of Salmonella spp. in liquid whole rgg. J Food Sci 76(3):M225–M232
Siripatrawan U, Linz JE, Harte BR (2006) Electronic sensor array coupled with artificial neural network for detection of Salmonella Typhimurium . Sens Actuators B Chem 119(1):64–69
Skandamis PN, Nychas GJE (2000) Development and evaluation of a model predicting the survival of Escherichia coli O157: H7 NCTC 12900 in homemade eggplant salad at various temperatures, pHs, and oregano essential oil concentrations. Appl Environ Microbiol 66(4):1646–1653
Skinner GE, Larkin JW, Rhodehamel EJ (1994) Mathematical modeling of microbial growth: A review. J Food Saf 14(3):175–217
Sojung K, Dongsun L (2015) Simple microbial growth model applicable to dynamic temperature conditions: evaluation of a nondimensional model. J Food Agric Environ 7(3/4):192–196
Sommers C, Huang CY, Sheen LY, Sheen S, Huang L (2018) Growth modeling of Uropathogenic Escherichia coli in ground chicken meat. Food Control 86:397–402
Srivastava AK, Volesky B (1990) Characterization of transient cultures of Clostridium acetobutylicum . Biotechnol Prog 6(6):408–420
Stannard CJ, Williams AP, Gibbs PA (1985) Temperature/growth relationships for psychrotrophic food-spoilage bacteria. Food Microbiol 2(2):115–122
Stern NJ, Pierson MD (2010) Yersinia enterocolitica: a review of the psychrotrophic water and foodborne pathogen. J Food Sci 44(6):1736–1742
Stern NJ, Pierson MD, Kotula AW (2010) Growth and competitive nature of Yersinia enterocolitica in whole milk. J Food Sci 45(4):972–974
Sun YS, Zhang Q (2018) Optimization design and reality of the virtual cutting process for the boring bar based on PSO-BP neural networks. Neural Comput Applic 29(5):1357–1367
Sutherland JP, Bayliss AJ, Roberts TA (1994) Predictive modelling of growth of Staphylococcus aureus : the effects of temperature, pH and sodium chloride. Int J Food Microbiol 21(3):217–236
Swinnen IAM, Bernaerts K, Dens EJJ, Geeraerd AH, Impe JFV (2004) Predictive modelling of the microbial lag phase: a review. Int J Food Microbiol 94(2):137–159
Tarlak F, Ozdemir M, Melikoglu M (2018) Mathematical modelling of temperature effect on growth kinetics of Pseudomonas spp. on sliced mushroom (Agaricus bisporus). Int J Food Microbiol 266:274–281
te Giffel MC, Zwietering MH (1999) Validation of predictive models describing the growth of Listeria monocytogenes . Int J Food Microbiol 46(2):135–149
Tsoularis A, Wallace J (2002) Analysis of logistic growth models. Math Biosci 179(1):21–55
USDA Integrated Pathogen Modeling Program. https://www.ars.usda.gov/northeast-area/wyndmoor-pa/eastern-regional-research-center/docs/ipmp-2013/ . Accessed on 7 July 2018
Vadasz P, Vadasz AS (2007) Biological implications from an autonomous version of Baranyi and Roberts growth model. Int J Food Microbiol 114(3):357–365
Van Houdt R, Kokkonen E, Lehtimäki M, Pasanen P, Leys N, Kulmala I (2018) Requirements for modeling airborne microbial contamination in space stations. Acta Astronaut 144:380–387
Vanier MC, Bower JM (1999) A comparative survey of automated parameter-search methods for compartmental neural models. J Comput Neurosci 7(2):149–171
Velugoti PR, Bohra LK, Juneja VK, Huang L, Wesseling AL, Subbiah J, Thippareddi H (2011) Dynamic model for predicting growth of Salmonella spp . in ground sterile pork. Food Microbiol 28(4):796–803
Verhulst PF (1845) Recherces mathematiques sur la loi d’accroissement de la population. Nouv Mém 18:1–41
Volterra V (1928) Variations and fluctuations of the numbers of individuals in animal species living together. J Conseil 3(1):3–51
Wachenheim DE, Patterson JA, Ladisch MR (2003) Analysis of the logistic function model: derivation and applications specific to batch cultured microorganisms. Bioresour Technol 86(2):157–164
Walter L, Knight G, Ng SY, Buckow R (2016) Kinetic models for pulsed electric field and thermal inactivation of Escherichia coli and Pseudomonas fluorescens in whole milk. Int Dairy J 57:7–14
Wang H, Kong C, Li D, Qin N, Fan H, Hong H, Luo Y (2015) Modeling quality changes in brined bream (Megalobrama amblycephala) fillets during storage: comparison of the Arrhenius model, BP, and RBF neural network. Food Bioprocess Technol 8(12):2429–2443
Wang J, Shi P, Jiang P, Hu J, Qu S, Chen X, Chen Y, Dai Y, Xiao Z (2017a) Application of BP neural network algorithm in traditional hydrological model for flood forecasting. Water 9(1):48
Wang W, Li M, Hassanien RHE, Ji ME, Feng Z (2017b) Optimization of thermal performance of the parabolic trough solar collector systems based on GA-BP neural network model. Int J Green Energy 14(10):819–830
Whiting RC (1995) Microbial modeling in foods. Crit Rev Food Sci Nutr 35(6):467–494
Whiting RC, Buchanan R (1993) A classification of models for predictive microbiology. Food Microbiol 10(12):175–177
Widder S, Allen RJ, Pfeiffer T, Curtis TP, Wiuf C, Sloan WT, Cordero OX, Brown SP, Momeni B, Shou W, Kettle H (2016) Challenges in microbial ecology: building predictive understanding of community function and dynamics. ISME J 10(11):2557–2568
Article PubMed PubMed Central Google Scholar
Xu G, Liang C, Huang P, Liu Q, Xu Y, Ding C, Li T (2016) Optimization of rice lipid production from ultrasound-assisted extraction by response surface methodology. J Cereal Sci 70:23–28
Ye K, Wang H, Zhang X, Xu X, Zhou G (2013) Development and validation of a molecular predictive model to describe;the growth of Listeria monocytogenes in vacuum-packaged chilled pork. Food Control 32(1):246–254
Yilmaz MT (2011) Identifiability of Baranyi model and comparison with empirical models in predicting effect of essential oils on growth of Salmonella Typhimurium in rainbow trout stored under aerobic, modified atmosphere and vacuum packed conditions. Afr J Biotechnol 10(38):7468–7479
Yolmeh M, Jafari SM (2017) Applications of response surface methodology in the food industry processes. Food Bioprocess Technol 10(3):413–433
Yoon JH, Bae YM, Jung SY, Cha MH, Ryu K, Park KH, Lee SY (2014) Predictive modeling for the growth of Listeria monocytogenes and Salmonella Typhimurium on fresh-cut cabbage at various temperatures. J Korean Soc Appl Biol Chem 57(5):631–638
Zhang E, Hou L, Shen C, Shi Y, Zhang Y (2016) Sound quality prediction of vehicle interior noise and mathematical modeling using a back propagation neural network (BPNN) based on particle swarm optimization (PSO). Meas Sci Technol 27(1):015801
Zheng ZY, Guo XN, Zhu KX, Peng W, Zhou HM (2017) Artificial neural network – genetic algorithm to optimize wheat germ fermentation condition: application to the production of two anti-tumor benzoquinones. Food Chem 227:264–270
Zhou K, George SM, Métris A, Li PL, Baranyi J (2011) Lag phase of Salmonella enterica under osmotic stress conditions. Appl Environ Microbiol 77(5):1758–1762
Zhu S, Chen G (2015) Numerical solution of a microbial growth model applied to dynamic environments. J Microbiol Methods 112:76–82
Zhu X, Zhao L, Liu S, Huang W, Zhao S (2011) Experimental study and modeling NaCl mass transfer during feta cheese ripening. Int J Food Eng 7(4):457–461
Zupan J, Gasteiger J (1991) Neural networks: a new method for solving chemical problems or just a passing phase? Anal Chim Acta 248(1):1–30
Zwietering MH, Jongenburger I, Rombouts FM, Van't Riet K (1990) Modeling of the bacterial growth curve. Appl Environ Microbiol 56(6):1875–1881
Zwietering MH, de Koos JT, Hasenack BE, de Witt JC, Van'T RK (1991) Modeling of bacterial growth as a function of temperature. Appl Environ Microbiol 57(4):1094–1101
Zwietering MH, de Wit JC, Notermans S (1996) Application of predictive microbiology to estimate the number of Bacillus cereus in pasteurised milk at the point of consumption. Int J Food Microbiol 30(1–2):55–70
Download references
Author information
Authors and affiliations.
Department of Food Science, Pennsylvania State University, University Park, PA, USA
Ehsan Mahdinia
Department of Food Science and Technology, East China University of Science and Technology, Shanghai, China
Shaowei Liu
Department of Agricultural and Biological Engineering, Pennsylvania State University, University Park, PA, USA
Ali Demirci & Virendra M. Puri
You can also search for this author in PubMed Google Scholar
Corresponding author
Correspondence to Virendra M. Puri .
Editor information
Editors and affiliations.
Ali Demirci
Department of Food Science and Human Nutrition, University of Illinois Urbana-Champaign, Urbana, IL, USA
Department of Food Science and Nutrition, Institute for Food Safety and Health, Illinois Institute of Technology, Chicago, IL, USA
Kathiravan Krishnamurthy
Rights and permissions
Reprints and permissions
Copyright information
© 2020 Springer Nature Switzerland AG
About this chapter
Mahdinia, E., Liu, S., Demirci, A., Puri, V.M. (2020). Microbial Growth Models. In: Demirci, A., Feng, H., Krishnamurthy, K. (eds) Food Safety Engineering. Food Engineering Series. Springer, Cham. https://doi.org/10.1007/978-3-030-42660-6_14
Download citation
DOI : https://doi.org/10.1007/978-3-030-42660-6_14
Published : 29 May 2020
Publisher Name : Springer, Cham
Print ISBN : 978-3-030-42659-0
Online ISBN : 978-3-030-42660-6
eBook Packages : Chemistry and Materials Science Chemistry and Material Science (R0)
Share this chapter
Anyone you share the following link with will be able to read this content:
Sorry, a shareable link is not currently available for this article.
Provided by the Springer Nature SharedIt content-sharing initiative
- Publish with us
Policies and ethics
- Find a journal
- Track your research
Information
- Author Services
Initiatives
You are accessing a machine-readable page. In order to be human-readable, please install an RSS reader.
All articles published by MDPI are made immediately available worldwide under an open access license. No special permission is required to reuse all or part of the article published by MDPI, including figures and tables. For articles published under an open access Creative Common CC BY license, any part of the article may be reused without permission provided that the original article is clearly cited. For more information, please refer to https://www.mdpi.com/openaccess .
Feature papers represent the most advanced research with significant potential for high impact in the field. A Feature Paper should be a substantial original Article that involves several techniques or approaches, provides an outlook for future research directions and describes possible research applications.
Feature papers are submitted upon individual invitation or recommendation by the scientific editors and must receive positive feedback from the reviewers.
Editor’s Choice articles are based on recommendations by the scientific editors of MDPI journals from around the world. Editors select a small number of articles recently published in the journal that they believe will be particularly interesting to readers, or important in the respective research area. The aim is to provide a snapshot of some of the most exciting work published in the various research areas of the journal.
Original Submission Date Received: .
- Active Journals
- Find a Journal
- Proceedings Series
- For Authors
- For Reviewers
- For Editors
- For Librarians
- For Publishers
- For Societies
- For Conference Organizers
- Open Access Policy
- Institutional Open Access Program
- Special Issues Guidelines
- Editorial Process
- Research and Publication Ethics
- Article Processing Charges
- Testimonials
- Preprints.org
- SciProfiles
- Encyclopedia
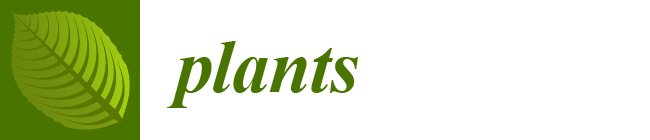
Article Menu

- Subscribe SciFeed
- Recommended Articles
- Google Scholar
- on Google Scholar
- Table of Contents
Find support for a specific problem in the support section of our website.
Please let us know what you think of our products and services.
Visit our dedicated information section to learn more about MDPI.
JSmol Viewer
How do plant growth-promoting bacteria use plant hormones to regulate stress reactions.

1. Introduction
2. pgpb and phytohormones in the rhizosphere, 2.1. auxins, 2.2. cytokinins, 2.3. gibberellins, 2.4. salicylic acid, 2.5. abscisic acid, 2.6. volatile organic compounds, 2.7. ethylene and acc deaminase, 3. synergistic effects of pgpb on plant growth through the interaction of multiple pathways, 3.1. effect of iaa on acc deaminase and ethylene synthesis, 3.2. interactions among phytohormones, 4. strategies for assessing the ability of pgpb to synthesize phytohormones, 4.1. determination of the potential for iaa synthesis, 4.2. detection of acc deaminase activity, 5. conclusions, author contributions, data availability statement, conflicts of interest.
- Cui, M.; Guo, Y.; Chen, J. Influence of Transfer Plot Area and Location on Chemical Input Reduction in Agricultural Production: Evidence from China. Agriculture 2023 , 13 , 1794. [ Google Scholar ] [ CrossRef ]
- Devi, P.I.; Manjula, M.; Bhavani, R.V. Agrochemicals, Environment, and Human Health. Annu. Rev. Environ. Resour. 2022 , 47 , 399–421. [ Google Scholar ] [ CrossRef ]
- Akinnawo, S.O. Eutrophication: Causes, Consequences, Physical, Chemical and Biological Techniques for Mitigation Strategies. Environ. Chall. 2023 , 12 , 100733. [ Google Scholar ] [ CrossRef ]
- Chataut, G.; Bhatta, B.; Joshi, D.; Subedi, K.; Kafle, K. Greenhouse Gases Emission from Agricultural Soil: A Review. J. Agric. Food Res. 2023 , 11 , 100533. [ Google Scholar ] [ CrossRef ]
- Ortiz-Monasterio, J.I.; Raun, W. Paper Presented At International Workshop On Increasing Wheat Yield Potential, Cimmyt, Obregon, Mexico, 20–24 MARCH 2006 Reduced Nitrogen and Improved Farm Income for Irrigated Spring Wheat in the Yaqui Valley, Mexico, Using Sensor Based Nitrogen Manageme. J. Agric. Sci. 2007 , 145 , 215–222. [ Google Scholar ] [ CrossRef ]
- Majeed, A.; Abbasi, M.K.; Hameed, S.; Imran, A.; Rahim, N. Isolation and Characterization of Plant Growth-Promoting Rhizobacteria from Wheat Rhizosphere and Their Effect on Plant Growth Promotion. Front. Microbiol. 2015 , 6 , 198. [ Google Scholar ] [ CrossRef ]
- Timofeeva, A.M.; Galyamova, M.R.; Sedykh, S.E. Plant Growth-Promoting Soil Bacteria: Nitrogen Fixation, Phosphate Solubilization, Siderophore Production, and Other Biological Activities. Plants 2023 , 12 , 4074. [ Google Scholar ] [ CrossRef ]
- Montoya-Martínez, A.C.; Parra-Cota, F.I.; de los Santos-Villalobos, S. Beneficial Microorganisms in Sustainable Agriculture: Harnessing Microbes’ Potential to Help Feed the World. Plants 2022 , 11 , 372. [ Google Scholar ] [ CrossRef ]
- Timofeeva, A.M.; Galyamova, M.R.; Sedykh, S.E. Bacterial Siderophores: Classification, Biosynthesis, Perspectives of Use in Agriculture. Plants 2022 , 11 , 3065. [ Google Scholar ] [ CrossRef ]
- Timofeeva, A.; Galyamova, M.; Sedykh, S. Prospects for Using Phosphate-Solubilizing Microorganisms as Natural Fertilizers in Agriculture. Plants 2022 , 11 , 2119. [ Google Scholar ] [ CrossRef ]
- Gurska, J.; Glick, B.R.; Greenberg, B.M. Gene Expression of Secale Cereale (Fall Rye) Grown in Petroleum Hydrocarbon (PHC) Impacted Soil With and Without Plant Growth-Promoting Rhizobacteria (PGPR), Pseudomonas putida . Water Air Soil Pollut. 2015 , 226 , 308. [ Google Scholar ] [ CrossRef ]
- Chet, I.; Inbar, J. Biological Control of Fungal Pathogens. Appl. Biochem. Biotechnol. 1994 , 48 , 37–43. [ Google Scholar ] [ CrossRef ] [ PubMed ]
- Penrose, D.M.; Glick, B.R. Methods for Isolating and Characterizing ACC Deaminase-containing Plant Growth-promoting Rhizobacteria. Physiol. Plant. 2003 , 118 , 10–15. [ Google Scholar ] [ CrossRef ] [ PubMed ]
- Asif, R.; Yasmin, R.; Mustafa, M.; Ambreen, A.; Mazhar, M.; Rehman, A.; Umbreen, S.; Ahmad, M. Phytohormones as Plant Growth Regulators and Safe Protectors against Biotic and Abiotic Stress. In Plant Hormones—Recent Advances, New Perspectives and Applications ; IntechOpen: Rijeka, Croatia, 2022. [ Google Scholar ]
- Egamberdieva, D.; Wirth, S.J.; Alqarawi, A.A.; Abd_Allah, E.F.; Hashem, A. Phytohormones and Beneficial Microbes: Essential Components for Plants to Balance Stress and Fitness. Front. Microbiol. 2017 , 8 , 2104. [ Google Scholar ] [ CrossRef ] [ PubMed ]
- Santoro, M.V.; Zygadlo, J.; Giordano, W.; Banchio, E. Volatile Organic Compounds from Rhizobacteria Increase Biosynthesis of Essential Oils and Growth Parameters in Peppermint ( Mentha piperita ). Plant Physiol. Biochem. 2011 , 49 , 1177–1182. [ Google Scholar ] [ CrossRef ]
- Nascimento, F.X.; Glick, B.R.; Rossi, M.J. Multiple Plant Hormone Catabolism Activities: An Adaptation to a Plant-associated Lifestyle by Achromobacter Spp. Environ. Microbiol. Rep. 2021 , 13 , 533–539. [ Google Scholar ] [ CrossRef ]
- Rashid, M.I.; Mujawar, L.H.; Shahzad, T.; Almeelbi, T.; Ismail, I.M.I.; Oves, M. Bacteria and Fungi Can Contribute to Nutrients Bioavailability and Aggregate Formation in Degraded Soils. Microbiol. Res. 2016 , 183 , 26–41. [ Google Scholar ] [ CrossRef ]
- Novo, L.A.B.; Mahler, C.F.; González, L. Plants to Harvest Rhenium: Scientific and Economic Viability. Environ. Chem. Lett. 2015 , 13 , 439–445. [ Google Scholar ] [ CrossRef ]
- Goswami, D.; Thakker, J.N.; Dhandhukia, P.C. Portraying Mechanics of Plant Growth Promoting Rhizobacteria (PGPR): A Review. Cogent Food Agric. 2016 , 2 , 1127500. [ Google Scholar ] [ CrossRef ]
- Arkhipova, T.N.; Evseeva, N.V.; Tkachenko, O.V.; Burygin, G.L.; Vysotskaya, L.B.; Akhtyamova, Z.A.; Kudoyarova, G.R. Rhizobacteria Inoculation Effects on Phytohormone Status of Potato Microclones Cultivated In Vitro under Osmotic Stress. Biomolecules 2020 , 10 , 1231. [ Google Scholar ] [ CrossRef ]
- Pankievicz, V.C.S.; do Amaral, F.P.; Ané, J.-M.; Stacey, G. Diazotrophic Bacteria and Their Mechanisms to Interact and Benefit Cereals. Mol. Plant-Microbe Interact. 2021 , 34 , 491–498. [ Google Scholar ] [ CrossRef ] [ PubMed ]
- Ma, Y.; Zhang, C.; Oliveira, R.S.; Freitas, H.; Luo, Y. Bioaugmentation with Endophytic Bacterium E6S Homologous to Achromobacter piechaudii Enhances Metal Rhizoaccumulation in Host Sedum plumbizincicola . Front. Plant Sci. 2016 , 7 , 75. [ Google Scholar ] [ CrossRef ] [ PubMed ]
- Iqbal, N.; Khan, N.A.; Ferrante, A.; Trivellini, A.; Francini, A.; Khan, M.I.R. Ethylene Role in Plant Growth, Development and Senescence: Interaction with Other Phytohormones. Front. Plant Sci. 2017 , 8 , 475. [ Google Scholar ] [ CrossRef ] [ PubMed ]
- Ma, Y.; Oliveira, R.S.; Freitas, H.; Zhang, C. Biochemical and Molecular Mechanisms of Plant-Microbe-Metal Interactions: Relevance for Phytoremediation. Front. Plant Sci. 2016 , 7 , 918. [ Google Scholar ] [ CrossRef ]
- Spence, C.; Bais, H. Role of Plant Growth Regulators as Chemical Signals in Plant–Microbe Interactions: A Double Edged Sword. Curr. Opin. Plant Biol. 2015 , 27 , 52–58. [ Google Scholar ] [ CrossRef ]
- Dodd, I.C.; Zinovkina, N.Y.; Safronova, V.I.; Belimov, A.A. Rhizobacterial Mediation of Plant Hormone Status. Ann. Appl. Biol. 2010 , 157 , 361–379. [ Google Scholar ] [ CrossRef ]
- Taylor, J.L.; Zaharia, L.I.; Chen, H.; Anderson, E.; Abrams, S.R. Biotransformation of Adenine and Cytokinins by the Rhizobacterium Serratia proteamaculans . Phytochemistry 2006 , 67 , 1887–1894. [ Google Scholar ] [ CrossRef ]
- Ullah, A.; Bano, A.; Khan, N. Climate Change and Salinity Effects on Crops and Chemical Communication Between Plants and Plant Growth-Promoting Microorganisms Under Stress. Front. Sustain. Food Syst. 2021 , 5 , 618092. [ Google Scholar ] [ CrossRef ]
- Yen, K.-M.; Serdar, C.M.; Gunsalus, I.C. Genetics of Naphthalene Catabolism in Pseudomonads. CRC Crit. Rev. Microbiol. 1988 , 15 , 247–268. [ Google Scholar ] [ CrossRef ]
- Sazonova, O.I.; Izmalkova, T.Y.; Kosheleva, I.A.; Boronin, A.M. Salicylate Degradation by Pseudomonas putida Strains Not Involving the “Classical” Nah2 Operon. Microbiology 2008 , 77 , 710–716. [ Google Scholar ] [ CrossRef ]
- Nascimento, F.X.; Glick, B.R.; Rossi, M.J. Isolation and Characterization of Novel Soil- and Plant-Associated Bacteria with Multiple Phytohormone-Degrading Activities Using a Targeted Methodology. Access Microbiol. 2019 , 1 , e000053. [ Google Scholar ] [ CrossRef ] [ PubMed ]
- Fukami, J.; Nogueira, M.A.; Araujo, R.S.; Hungria, M. Accessing Inoculation Methods of Maize and Wheat with Azospirillum brasilense . AMB Express 2016 , 6 , 3. [ Google Scholar ] [ CrossRef ]
- Sahoo, R.K.; Ansari, M.W.; Pradhan, M.; Dangar, T.K.; Mohanty, S.; Tuteja, N. Phenotypic and Molecular Characterization of Native Azospirillum Strains from Rice Fields to Improve Crop Productivity. Protoplasma 2014 , 251 , 943–953. [ Google Scholar ] [ CrossRef ]
- Fukami, J.; Cerezini, P.; Hungria, M. Azospirillum : Benefits That Go Far beyond Biological Nitrogen Fixation. AMB Express 2018 , 8 , 73. [ Google Scholar ] [ CrossRef ] [ PubMed ]
- Cohen, A.C.; Bottini, R.; Piccoli, P.N. Azospirillum brasilense Sp 245 Produces ABA in Chemically-Defined Culture Medium and Increases ABA Content in Arabidopsis Plants. Plant Growth Regul. 2008 , 54 , 97–103. [ Google Scholar ] [ CrossRef ]
- Weijers, D.; Wagner, D. Transcriptional Responses to the Auxin Hormone. Annu. Rev. Plant Biol. 2016 , 67 , 539–574. [ Google Scholar ] [ CrossRef ] [ PubMed ]
- Spaepen, S.; Vanderleyden, J.; Remans, R. Indole-3-Acetic Acid in Microbial and Microorganism-Plant Signaling. FEMS Microbiol. Rev. 2007 , 31 , 425–448. [ Google Scholar ] [ CrossRef ] [ PubMed ]
- Korasick, D.A.; Enders, T.A.; Strader, L.C. Auxin Biosynthesis and Storage Forms. J. Exp. Bot. 2013 , 64 , 2541–2555. [ Google Scholar ] [ CrossRef ]
- Patten, C.L.; Glick, B.R. Bacterial Biosynthesis of Indole-3-Acetic Acid. Can. J. Microbiol. 1996 , 42 , 207–220. [ Google Scholar ] [ CrossRef ]
- Khalid, A.; Tahir, S.; Arshad, M.; Zahir, Z.A. Relative Efficiency of Rhizobacteria for Auxin Biosynthesis in Rhizosphere and Non-Rhizosphere Soils. Soil Res. 2004 , 42 , 921. [ Google Scholar ] [ CrossRef ]
- Cassán, F.; Coniglio, A.; López, G.; Molina, R.; Nievas, S.; de Carlan, C.L.N.; Donadio, F.; Torres, D.; Rosas, S.; Pedrosa, F.O.; et al. Everything You Must Know about Azospirillum and Its Impact on Agriculture and Beyond. Biol. Fertil. Soils 2020 , 56 , 461–479. [ Google Scholar ] [ CrossRef ]
- Spaepen, S.; Vanderleyden, J. Auxin and Plant-Microbe Interactions. Cold Spring Harb. Perspect. Biol. 2011 , 3 , a001438. [ Google Scholar ] [ CrossRef ]
- Zúñiga, A.; Poupin, M.J.; Donoso, R.; Ledger, T.; Guiliani, N.; Gutiérrez, R.A.; González, B. Quorum Sensing and Indole-3-Acetic Acid Degradation Play a Role in Colonization and Plant Growth Promotion of Arabidopsis thaliana by Burkholderia Phytofirmans PsJN. Mol. Plant-Microbe Interact. 2013 , 26 , 546–553. [ Google Scholar ] [ CrossRef ] [ PubMed ]
- Leveau, J.H.J.; Gerards, S. Discovery of a Bacterial Gene Cluster for Catabolism of the Plant Hormone Indole 3-Acetic Acid. FEMS Microbiol. Ecol. 2008 , 65 , 238–250. [ Google Scholar ] [ CrossRef ] [ PubMed ]
- Calatrava, V.; Hom, E.F.Y.; Guan, Q.; Llamas, A.; Fernández, E.; Galván, A. Genetic Evidence for Algal Auxin Production in Chlamydomonas and Its Role in Algal-Bacterial Mutualism. iScience 2024 , 27 , 108762. [ Google Scholar ] [ CrossRef ] [ PubMed ]
- Calatrava, V.; Hom, E.F.Y.; Llamas, Á.; Fernández, E.; Galván, A. OK, Thanks! A New Mutualism between Chlamydomonas and Methylobacteria Facilitates Growth on Amino Acids and Peptides. FEMS Microbiol. Lett. 2018 , 365 , fny021. [ Google Scholar ] [ CrossRef ]
- Olesen, M.R.; Jochimsen, B.U. Identification of Enzymes Involved in Indole-3-Acetic Acid Degradation. Plant Soil 1996 , 186 , 143–149. [ Google Scholar ] [ CrossRef ]
- Mole, B.M.; Baltrus, D.A.; Dangl, J.L.; Grant, S.R. Global Virulence Regulation Networks in Phytopathogenic Bacteria. Trends Microbiol. 2007 , 15 , 363–371. [ Google Scholar ] [ CrossRef ]
- Duca, D.; Lorv, J.; Patten, C.L.; Rose, D.; Glick, B.R. Indole-3-Acetic Acid in Plant–Microbe Interactions. Antonie Van Leeuwenhoek 2014 , 106 , 85–125. [ Google Scholar ] [ CrossRef ]
- Kudoyarova, G.; Arkhipova, T.; Korshunova, T.; Bakaeva, M.; Loginov, O.; Dodd, I.C. Phytohormone Mediation of Interactions Between Plants and Non-Symbiotic Growth Promoting Bacteria Under Edaphic Stresses. Front. Plant Sci. 2019 , 10 , 1368. [ Google Scholar ] [ CrossRef ]
- Keswani, C.; Singh, S.P.; García-Estrada, C.; Mezaache-Aichour, S.; Glare, T.R.; Borriss, R.; Rajput, V.D.; Minkina, T.M.; Ortiz, A.; Sansinenea, E. Biosynthesis and Beneficial Effects of Microbial Gibberellins on Crops for Sustainable Agriculture. J. Appl. Microbiol. 2022 , 132 , 1597–1615. [ Google Scholar ] [ CrossRef ] [ PubMed ]
- Morales-Cedeño, L.R.; Orozco-Mosqueda, M.d.C.; Loeza-Lara, P.D.; Parra-Cota, F.I.; de los Santos-Villalobos, S.; Santoyo, G. Plant Growth-Promoting Bacterial Endophytes as Biocontrol Agents of Pre- and Post-Harvest Diseases: Fundamentals, Methods of Application and Future Perspectives. Microbiol. Res. 2021 , 242 , 126612. [ Google Scholar ] [ CrossRef ] [ PubMed ]
- Bianco, C.; Defez, R. Medicago truncatula Improves Salt Tolerance When Nodulated by an Indole-3-Acetic Acid-Overproducing Sinorhizobium meliloti Strain. J. Exp. Bot. 2009 , 60 , 3097–3107. [ Google Scholar ] [ CrossRef ]
- Pii, Y.; Crimi, M.; Cremonese, G.; Spena, A.; Pandolfini, T. Auxin and Nitric Oxide Control Indeterminate Nodule Formation. BMC Plant Biol. 2007 , 7 , 21. [ Google Scholar ] [ CrossRef ]
- Camerini, S.; Senatore, B.; Lonardo, E.; Imperlini, E.; Bianco, C.; Moschetti, G.; Rotino, G.L.; Campion, B.; Defez, R. Introduction of a Novel Pathway for IAA Biosynthesis to Rhizobia Alters Vetch Root Nodule Development. Arch. Microbiol. 2008 , 190 , 67–77. [ Google Scholar ] [ CrossRef ] [ PubMed ]
- Baudoin, E.; Lerner, A.; Mirza, M.S.; El Zemrany, H.; Prigent-Combaret, C.; Jurkevich, E.; Spaepen, S.; Vanderleyden, J.; Nazaret, S.; Okon, Y.; et al. Effects of Azospirillum brasilense with Genetically Modified Auxin Biosynthesis Gene IpdC upon the Diversity of the Indigenous Microbiota of the Wheat Rhizosphere. Res. Microbiol. 2010 , 161 , 219–226. [ Google Scholar ] [ CrossRef ]
- Salomé, P.A.; Merchant, S.S. A Series of Fortunate Events: Introducing Chlamydomonas as a Reference Organism. Plant Cell 2019 , 31 , 1682–1707. [ Google Scholar ] [ CrossRef ]
- Zhang, C.; Wang, M.-Y.; Khan, N.; Tan, L.-L.; Yang, S. Potentials, Utilization, and Bioengineering of Plant Growth-Promoting Methylobacterium for Sustainable Agriculture. Sustainability 2021 , 13 , 3941. [ Google Scholar ] [ CrossRef ]
- Calatrava, V.; Hom, E.F.Y.; Llamas, Á.; Fernández, E.; Galván, A. Nitrogen Scavenging from Amino Acids and Peptides in the Model Alga Chlamydomonas reinhardtii . The Role of Extracellular l-Amino Oxidase. Algal Res. 2019 , 38 , 101395. [ Google Scholar ] [ CrossRef ]
- Zhao, Y. Auxin Biosynthesis: A Simple Two-Step Pathway Converts Tryptophan to Indole-3-Acetic Acid in Plants. Mol. Plant 2012 , 5 , 334–338. [ Google Scholar ] [ CrossRef ]
- Wang, X.; Zeng, L.; Liao, Y.; Zhou, Y.; Xu, X.; Dong, F.; Yang, Z. An Alternative Pathway for the Formation of Aromatic Aroma Compounds Derived from L-Phenylalanine via Phenylpyruvic Acid in Tea ( Camellia sinensis (L.) O. Kuntze) Leaves. Food Chem. 2019 , 270 , 17–24. [ Google Scholar ] [ CrossRef ] [ PubMed ]
- Osugi, A.; Sakakibara, H. Q&A: How Do Plants Respond to Cytokinins and What Is Their Importance? BMC Biol. 2015 , 13 , 102. [ Google Scholar ] [ CrossRef ]
- Kieber, J.J.; Schaller, G.E. Cytokinin Signaling in Plant Development. Development 2018 , 145 , dev149344. [ Google Scholar ] [ CrossRef ] [ PubMed ]
- Kieber, J.J.; Schaller, G.E. The Perception of Cytokinin: A Story 50 Years in the Making. Plant Physiol. 2010 , 154 , 487–492. [ Google Scholar ] [ CrossRef ] [ PubMed ]
- Orozco-Mosqueda, M.d.C.; Santoyo, G.; Glick, B.R. Recent Advances in the Bacterial Phytohormone Modulation of Plant Growth. Plants 2023 , 12 , 606. [ Google Scholar ] [ CrossRef ]
- Auer, C.A. Cytokinin Inhibition of Arabidopsis Root Growth: An Examination of Genotype, Cytokinin Activity, and N6-Benzyladenine Metabolism. J. Plant Growth Regul. 1996 , 15 , 201–206. [ Google Scholar ] [ CrossRef ]
- Strnad, M. The Aromatic Cytokinins. Physiol. Plant. 1997 , 101 , 674–688. [ Google Scholar ] [ CrossRef ]
- Tarkowská, D.; Doležal, K.; Tarkowski, P.; Åstot, C.; Holub, J.; Fuksová, K.; Schmülling, T.; Sandberg, G.; Strnad, M. Identification of New Aromatic Cytokinins in Arabidopsis thaliana and Populus × Canadensis Leaves by LC-(+)ESI-MS and Capillary Liquid Chromatography/Frit–Fast Atom Bombardment Mass Spectrometry. Physiol. Plant. 2003 , 117 , 579–590. [ Google Scholar ] [ CrossRef ]
- Spíchal, L.; Rakova, N.Y.; Riefler, M.; Mizuno, T.; Romanov, G.A.; Strnad, M.; Schmülling, T. Two Cytokinin Receptors of Arabidopsis thaliana , CRE1/AHK4 and AHK3, Differ in Their Ligand Specificity in a Bacterial Assay. Plant Cell Physiol. 2004 , 45 , 1299–1305. [ Google Scholar ] [ CrossRef ]
- Hönig, M.; Plíhalová, L.; Husičková, A.; Nisler, J.; Doležal, K. Role of Cytokinins in Senescence, Antioxidant Defence and Photosynthesis. Int. J. Mol. Sci. 2018 , 19 , 4045. [ Google Scholar ] [ CrossRef ]
- Mok, D.W.; Mok, M.C. CYTOKININ METABOLISM AND ACTION. Annu. Rev. Plant Physiol. Plant Mol. Biol. 2001 , 52 , 89–118. [ Google Scholar ] [ CrossRef ] [ PubMed ]
- Lomin, S.N.; Krivosheev, D.M.; Steklov, M.Y.; Arkhipov, D.V.; Osolodkin, D.I.; Schmülling, T.; Romanov, G.A. Plant Membrane Assays with Cytokinin Receptors Underpin the Unique Role of Free Cytokinin Bases as Biologically Active Ligands. J. Exp. Bot. 2015 , 66 , 1851–1863. [ Google Scholar ] [ CrossRef ]
- Li, Y.; Baldauf, S.; Lim, E.-K.; Bowles, D.J. Phylogenetic Analysis of the UDP-Glycosyltransferase Multigene Family of Arabidopsis thaliana . J. Biol. Chem. 2001 , 276 , 4338–4343. [ Google Scholar ] [ CrossRef ] [ PubMed ]
- Bajguz, A.; Piotrowska, A. Conjugates of Auxin and Cytokinin. Phytochemistry 2009 , 70 , 957–969. [ Google Scholar ] [ CrossRef ] [ PubMed ]
- Hluska, T.; Hlusková, L.; Emery, R.J.N. The Hulks and the Deadpools of the Cytokinin Universe: A Dual Strategy for Cytokinin Production, Translocation, and Signal Transduction. Biomolecules 2021 , 11 , 209. [ Google Scholar ] [ CrossRef ] [ PubMed ]
- Frebort, I.; Kowalska, M.; Hluska, T.; Frebortova, J.; Galuszka, P. Evolution of Cytokinin Biosynthesis and Degradation. J. Exp. Bot. 2011 , 62 , 2431–2452. [ Google Scholar ] [ CrossRef ]
- Wei, X.; Moreno-Hagelsieb, G.; Glick, B.R.; Doxey, A.C. Comparative Analysis of Adenylate Isopentenyl Transferase Genes in Plant Growth-Promoting Bacteria and Plant Pathogenic Bacteria. Heliyon 2023 , 9 , e13955. [ Google Scholar ] [ CrossRef ]
- Nester, E.W.; Gordon, M.P.; Amasino, R.M.; Yanofsky, M.F. Crown Gall: A Molecular and Physiological Analysis. Annu. Rev. Plant Physiol. 1984 , 35 , 387–413. [ Google Scholar ] [ CrossRef ]
- Zboralski, A.; Filion, M. Pseudomonas Spp. Can Help Plants Face Climate Change. Front. Microbiol. 2023 , 14 , 1198131. [ Google Scholar ] [ CrossRef ]
- Frébortová, J.; Greplová, M.; Seidl, M.F.; Heyl, A.; Frébort, I. Biochemical Characterization of Putative Adenylate Dimethylallyltransferase and Cytokinin Dehydrogenase from Nostoc Sp. PCC 7120. PLoS ONE 2015 , 10 , e0138468. [ Google Scholar ] [ CrossRef ]
- Arkhipova, T.N.; Prinsen, E.; Veselov, S.U.; Martinenko, E.V.; Melentiev, A.I.; Kudoyarova, G.R. Cytokinin Producing Bacteria Enhance Plant Growth in Drying Soil. Plant Soil 2007 , 292 , 305–315. [ Google Scholar ] [ CrossRef ]
- Großkinsky, D.K.; Tafner, R.; Moreno, M.V.; Stenglein, S.A.; García de Salamone, I.E.; Nelson, L.M.; Novák, O.; Strnad, M.; van der Graaff, E.; Roitsch, T. Cytokinin Production by Pseudomonas fluorescens G20-18 Determines Biocontrol Activity against Pseudomonas syringae in Arabidopsis . Sci. Rep. 2016 , 6 , 23310. [ Google Scholar ] [ CrossRef ]
- Akhtar, S.S.; Mekureyaw, M.F.; Pandey, C.; Roitsch, T. Role of Cytokinins for Interactions of Plants With Microbial Pathogens and Pest Insects. Front. Plant Sci. 2020 , 10 , 1777. [ Google Scholar ] [ CrossRef ]
- De Rybel, B.; Mähönen, A.P.; Helariutta, Y.; Weijers, D. Plant Vascular Development: From Early Specification to Differentiation. Nat. Rev. Mol. Cell Biol. 2016 , 17 , 30–40. [ Google Scholar ] [ CrossRef ] [ PubMed ]
- Brenner, W.G.; Ramireddy, E.; Heyl, A.; Schmülling, T. Gene Regulation by Cytokinin in Arabidopsis . Front. Plant Sci. 2012 , 3 , 8. [ Google Scholar ] [ CrossRef ]
- Aremu, A.O.; Fawole, O.A.; Makunga, N.P.; Masondo, N.A.; Moyo, M.; Buthelezi, N.M.D.; Amoo, S.O.; Spíchal, L.; Doležal, K. Applications of Cytokinins in Horticultural Fruit Crops: Trends and Future Prospects. Biomolecules 2020 , 10 , 1222. [ Google Scholar ] [ CrossRef ]
- AlAli, H.A.; Khalifa, A.; Almalki, M. Plant Growth-Promoting Rhizobacteria from Ocimum basilicum Improve Growth of Phaseolus vulgaris and Abelmoschus esculentus . South African J. Bot. 2021 , 139 , 200–209. [ Google Scholar ] [ CrossRef ]
- Al-Tammar, F.K.; Khalifa, A.Y.Z. Plant Growth Promoting Bacteria Drive Food Security. Brazilian J. Biol. 2022 , 82 , e267257. [ Google Scholar ] [ CrossRef ] [ PubMed ]
- Forni, C.; Duca, D.; Glick, B.R. Mechanisms of Plant Response to Salt and Drought Stress and Their Alteration by Rhizobacteria . Plant Soil 2017 , 410 , 335–356. [ Google Scholar ] [ CrossRef ]
- Arkhipova, T.N.; Veselov, S.U.; Melentiev, A.I.; Martynenko, E.V.; Kudoyarova, G.R. Ability of Bacterium Bacillus subtilis to Produce Cytokinins and to Influence the Growth and Endogenous Hormone Content of Lettuce Plants. Plant Soil 2005 , 272 , 201–209. [ Google Scholar ] [ CrossRef ]
- Xu, J.; Li, X.-L.; Luo, L. Effects of Engineered Sinorhizobium meliloti on Cytokinin Synthesis and Tolerance of Alfalfa to Extreme Drought Stress. Appl. Environ. Microbiol. 2012 , 78 , 8056–8061. [ Google Scholar ] [ CrossRef ]
- Palberg, D.; Kisiała, A.; Jorge, G.L.; Emery, R.J.N. A Survey of Methylobacterium Species and Strains Reveals Widespread Production and Varying Profiles of Cytokinin Phytohormones. BMC Microbiol. 2022 , 22 , 49. [ Google Scholar ] [ CrossRef ] [ PubMed ]
- Mekureyaw, M.F.; Pandey, C.; Hennessy, R.C.; Nicolaisen, M.H.; Liu, F.; Nybroe, O.; Roitsch, T. The Cytokinin-Producing Plant Beneficial Bacterium Pseudomonas fluorescens G20-18 Primes Tomato ( Solanum lycopersicum ) for Enhanced Drought Stress Responses. J. Plant Physiol. 2022 , 270 , 153629. [ Google Scholar ] [ CrossRef ] [ PubMed ]
- Van De Velde, K.; Ruelens, P.; Geuten, K.; Rohde, A.; Van Der Straeten, D. Exploiting DELLA Signaling in Cereals. Trends Plant Sci. 2017 , 22 , 880–893. [ Google Scholar ] [ CrossRef ]
- Zaidi, A.; Ahmad, E.; Khan, M.S.; Saif, S.; Rizvi, A. Role of Plant Growth Promoting Rhizobacteria in Sustainable Production of Vegetables: Current Perspective. Sci. Hortic. (Amsterdam). 2015 , 193 , 231–239. [ Google Scholar ] [ CrossRef ]
- Khan, A.L.; Waqas, M.; Hussain, J.; Al-Harrasi, A.; Hamayun, M.; Lee, I.-J. Phytohormones Enabled Endophytic Fungal Symbiosis Improve Aluminum Phytoextraction in Tolerant Solanum lycopersicum : An Examples of Penicillium janthinellum LK5 and Comparison with Exogenous GA3. J. Hazard. Mater. 2015 , 295 , 70–78. [ Google Scholar ] [ CrossRef ]
- You, Y.-H. Fungal Diversity and Plant Growth Promotion of Endophytic Fungi from Six Halophytes in Suncheon Bay. J. Microbiol. Biotechnol. 2012 , 22 , 1549–1556. [ Google Scholar ] [ CrossRef ]
- Kozaki, A.; Aoyanagi, T. Molecular Aspects of Seed Development Controlled by Gibberellins and Abscisic Acids. Int. J. Mol. Sci. 2022 , 23 , 1876. [ Google Scholar ] [ CrossRef ] [ PubMed ]
- Hedden, P.; Thomas, S.G. Gibberellin Biosynthesis and Its Regulation. Biochem. J. 2012 , 444 , 11–25. [ Google Scholar ] [ CrossRef ]
- Olanrewaju, O.S.; Glick, B.R.; Babalola, O.O. Mechanisms of Action of Plant Growth Promoting Bacteria. World J. Microbiol. Biotechnol. 2017 , 33 , 197. [ Google Scholar ] [ CrossRef ]
- Nelson, S.K.; Steber, C.M. Gibberellin Hormone Signal Perception: Down-regulating DELLA Repressors of Plant Growth and Development. In Annual Plant Reviews ; Wiley: Hoboken, NJ, USA, 2016; Volume 49, pp. 153–188. [ Google Scholar ]
- Yamaguchi, S. Gibberellin Metabolism and Its Regulation. Annu. Rev. Plant Biol. 2008 , 59 , 225–251. [ Google Scholar ] [ CrossRef ]
- Liu, J.; Qiu, G.; Liu, C.; Li, H.; Chen, X.; Fu, Q.; Lin, Y.; Guo, B. Salicylic Acid, a Multifaceted Hormone, Combats Abiotic Stresses in Plants. Life 2022 , 12 , 886. [ Google Scholar ] [ CrossRef ] [ PubMed ]
- Koo, Y.M.; Heo, A.Y.; Choi, H.W. Salicylic Acid as a Safe Plant Protector and Growth Regulator. Plant Pathol. J. 2020 , 36 , 1–10. [ Google Scholar ] [ CrossRef ] [ PubMed ]
- Riaz, A.; Rafique, M.; Aftab, M.; Qureshi, M.A.; Javed, H.; Mujeeb, F.; Akhtar, S. Mitigation of Salinity in Chickpea by Plant Growth Promoting Rhizobacteria and Salicylic Acid. EURASIAN J. SOIL Sci. 2019 , 8 , 221–228. [ Google Scholar ] [ CrossRef ]
- Mehrasa, H.; Farnia, A.; Kenarsari, M.J.; Nakhjavan, S. Endophytic Bacteria and SA Application Improve Growth, Biochemical Properties, and Nutrient Uptake in White Beans Under Drought Stress. J. Soil Sci. Plant Nutr. 2022 , 22 , 3268–3279. [ Google Scholar ] [ CrossRef ]
- de Andrade, W.L.; de Melo, A.S.; Melo, Y.L.; da Silva Sá, F.V.; Rocha, M.M.; da Silva Oliveira, A.P.; Fernandes Júnior, P.I. Bradyrhizobium Inoculation Plus Foliar Application of Salicylic Acid Mitigates Water Deficit Effects on Cowpea. J. Plant Growth Regul. 2021 , 40 , 656–667. [ Google Scholar ] [ CrossRef ]
- Lefevere, H.; Bauters, L.; Gheysen, G. Salicylic Acid Biosynthesis in Plants. Front. Plant Sci. 2020 , 11 , 338. [ Google Scholar ] [ CrossRef ]
- Torrens-Spence, M.P.; Bobokalonova, A.; Carballo, V.; Glinkerman, C.M.; Pluskal, T.; Shen, A.; Weng, J.-K. PBS3 and EPS1 Complete Salicylic Acid Biosynthesis from Isochorismate in Arabidopsis . Mol. Plant 2019 , 12 , 1577–1586. [ Google Scholar ] [ CrossRef ]
- Rekhter, D.; Lüdke, D.; Ding, Y.; Feussner, K.; Zienkiewicz, K.; Lipka, V.; Wiermer, M.; Zhang, Y.; Feussner, I. Isochorismate-Derived Biosynthesis of the Plant Stress Hormone Salicylic Acid. Science (80-.). 2019 , 365 , 498–502. [ Google Scholar ] [ CrossRef ]
- Grobelak, A.; Hiller, J. Bacterial Siderophores Promote Plant Growth: Screening of Catechol and Hydroxamate Siderophores. Int. J. Phytoremediation 2017 , 19 , 825–833. [ Google Scholar ] [ CrossRef ]
- Kjærbølling, I.; Mortensen, U.H.; Vesth, T.; Andersen, M.R. Strategies to Establish the Link between Biosynthetic Gene Clusters and Secondary Metabolites. Fungal Genet. Biol. 2019 , 130 , 107–121. [ Google Scholar ] [ CrossRef ] [ PubMed ]
- Mishra, A.; Baek, K.-H. Salicylic Acid Biosynthesis and Metabolism: A Divergent Pathway for Plants and Bacteria. Biomolecules 2021 , 11 , 705. [ Google Scholar ] [ CrossRef ] [ PubMed ]
- Weber, T.; Kim, H.U. The Secondary Metabolite Bioinformatics Portal: Computational Tools to Facilitate Synthetic Biology of Secondary Metabolite Production. Synth. Syst. Biotechnol. 2016 , 1 , 69–79. [ Google Scholar ] [ CrossRef ] [ PubMed ]
- Zeevaart, J.A.D.; Creelman, R.A. Metabolism and Physiology of Abscisic Acid. Annu. Rev. Plant Physiol. Plant Mol. Biol. 1988 , 39 , 439–473. [ Google Scholar ] [ CrossRef ]
- Melcher, K.; Xu, Y.; Ng, L.-M.; Zhou, X.E.; Soon, F.-F.; Chinnusamy, V.; Suino-Powell, K.M.; Kovach, A.; Tham, F.S.; Cutler, S.R.; et al. Identification and Mechanism of ABA Receptor Antagonism. Nat. Struct. Mol. Biol. 2010 , 17 , 1102–1108. [ Google Scholar ] [ CrossRef ]
- Liu, S.; Lv, Z.; Liu, Y.; Li, L.; Zhang, L. Network Analysis of ABA-Dependent and ABA-Independent Drought Responsive Genes in Arabidopsis thaliana . Genet. Mol. Biol. 2018 , 41 , 624–637. [ Google Scholar ] [ CrossRef ]
- Nambara, E.; Marion-Poll, A. ABSCISIC ACID BIOSYNTHESIS AND CATABOLISM. Annu. Rev. Plant Biol. 2005 , 56 , 165–185. [ Google Scholar ] [ CrossRef ]
- Chen, K.; Li, G.; Bressan, R.A.; Song, C.; Zhu, J.; Zhao, Y. Abscisic Acid Dynamics, Signaling, and Functions in Plants. J. Integr. Plant Biol. 2020 , 62 , 25–54. [ Google Scholar ] [ CrossRef ]
- Hartung, W.; Sauter, A.; Turner, N.C.; Fillery, I.; Heilmeier, H. Abscisic Acid in Soils: What Is Its Function and Which Factors and Mechanisms Influence Its Concentration? Plant Soil 1996 , 184 , 105–110. [ Google Scholar ] [ CrossRef ]
- Forchetti, G.; Masciarelli, O.; Alemano, S.; Alvarez, D.; Abdala, G. Endophytic Bacteria in Sunflower ( Helianthus annuus L.): Isolation, Characterization, and Production of Jasmonates and Abscisic Acid in Culture Medium. Appl. Microbiol. Biotechnol. 2007 , 76 , 1145–1152. [ Google Scholar ] [ CrossRef ]
- Cohen, A.C.; Travaglia, C.N.; Bottini, R.; Piccoli, P.N. Participation of Abscisic Acid and Gibberellins Produced by Endophytic Azospirillum in the Alleviation of Drought Effects in Maize. Botany 2009 , 87 , 455–462. [ Google Scholar ] [ CrossRef ]
- Sgroy, V.; Cassán, F.; Masciarelli, O.; Del Papa, M.F.; Lagares, A.; Luna, V. Isolation and Characterization of Endophytic Plant Growth-Promoting (PGPB) or Stress Homeostasis-Regulating (PSHB) Bacteria Associated to the Halophyte Prosopis Strombulifera. Appl. Microbiol. Biotechnol. 2009 , 85 , 371–381. [ Google Scholar ] [ CrossRef ] [ PubMed ]
- Hartung, W. The Evolution of Abscisic Acid (ABA) and ABA Function in Lower Plants, Fungi and Lichen. Funct. Plant Biol. 2010 , 37 , 806. [ Google Scholar ] [ CrossRef ]
- Crocoll, C.; Kettner, J.; Dörffling, K. Abscisic Acid in Saprophytic and Parasitic Species of Fungi. Phytochemistry 1991 , 30 , 1059–1060. [ Google Scholar ] [ CrossRef ]
- Belimov, A.A.; Dodd, I.C.; Safronova, V.I.; Dumova, V.A.; Shaposhnikov, A.I.; Ladatko, A.G.; Davies, W.J. Abscisic Acid Metabolizing Rhizobacteria Decrease ABA Concentrations in Planta and Alter Plant Growth. Plant Physiol. Biochem. 2014 , 74 , 84–91. [ Google Scholar ] [ CrossRef ]
- Zhang, J.; Schurr, U.; Davies, W.J. Control of Stomatal Behaviour by Abscisic Acid Which Apparently Originates in the Roots. J. Exp. Bot. 1987 , 38 , 1174–1181. [ Google Scholar ] [ CrossRef ]
- Vacheron, J.; Desbrosses, G.; Bouffaud, M.-L.; Touraine, B.; Moënne-Loccoz, Y.; Muller, D.; Legendre, L.; Wisniewski-Dyé, F.; Prigent-Combaret, C. Plant Growth-Promoting Rhizobacteria and Root System Functioning. Front. Plant Sci. 2013 , 4 , 356. [ Google Scholar ] [ CrossRef ] [ PubMed ]
- Zhou, S.; Hu, W.; Deng, X.; Ma, Z.; Chen, L.; Huang, C.; Wang, C.; Wang, J.; He, Y.; Yang, G.; et al. Overexpression of the Wheat Aquaporin Gene, TaAQP7, Enhances Drought Tolerance in Transgenic Tobacco. PLoS ONE 2012 , 7 , e52439. [ Google Scholar ] [ CrossRef ] [ PubMed ]
- Hasegawa, S.; Poling, S.M.; Maier, V.P.; Bennett, R.D. Metabolism of Abscisic Acid: Bacterial Conversion to Dehydrovomifoliol and Vomifoliol Dehydrogenase Activity. Phytochemistry 1984 , 23 , 2769–2771. [ Google Scholar ] [ CrossRef ]
- Montes-Osuna, N.; Cernava, T.; Gómez-Lama Cabanás, C.; Berg, G.; Mercado-Blanco, J. Identification of Volatile Organic Compounds Emitted by Two Beneficial Endophytic Pseudomonas Strains from Olive Roots. Plants 2022 , 11 , 318. [ Google Scholar ] [ CrossRef ]
- Kloepper, J.W.; Ryu, C.-M.; Zhang, S. Induced Systemic Resistance and Promotion of Plant Growth by Bacillus Spp. Phytopathology® 2004 , 94 , 1259–1266. [ Google Scholar ] [ CrossRef ] [ PubMed ]
- Kai, M.; Effmert, U.; Piechulla, B. Bacterial-Plant-Interactions: Approaches to Unravel the Biological Function of Bacterial Volatiles in the Rhizosphere. Front. Microbiol. 2016 , 7 , 108. [ Google Scholar ] [ CrossRef ] [ PubMed ]
- Choub, V.; Won, S.-J.; Ajuna, H.B.; Moon, J.-H.; Choi, S.-I.; Lim, H.-I.; Ahn, Y.S. Antifungal Activity of Volatile Organic Compounds from Bacillus velezensis CE 100 against Colletotrichum Gloeosporioides. Horticulturae 2022 , 8 , 557. [ Google Scholar ] [ CrossRef ]
- Tahir, H.A.S.; Gu, Q.; Wu, H.; Raza, W.; Hanif, A.; Wu, L.; Colman, M.V.; Gao, X. Plant Growth Promotion by Volatile Organic Compounds Produced by Bacillus subtilis SYST2. Front. Microbiol. 2017 , 8 , 171. [ Google Scholar ] [ CrossRef ] [ PubMed ]
- Venneman, J.; Vandermeersch, L.; Walgraeve, C.; Audenaert, K.; Ameye, M.; Verwaeren, J.; Steppe, K.; Van Langenhove, H.; Haesaert, G.; Vereecke, D. Respiratory CO 2 Combined With a Blend of Volatiles Emitted by Endophytic Serendipita Strains Strongly Stimulate Growth of Arabidopsis Implicating Auxin and Cytokinin Signaling. Front. Plant Sci. 2020 , 11 , 544435. [ Google Scholar ] [ CrossRef ]
- Sharifi, R.; Ryu, C.-M. Revisiting Bacterial Volatile-Mediated Plant Growth Promotion: Lessons from the Past and Objectives for the Future. Ann. Bot. 2018 , 122 , 349–358. [ Google Scholar ] [ CrossRef ]
- del Carmen Orozco-Mosqueda, M.; Macías-Rodríguez, L.I.; Santoyo, G.; Farías-Rodríguez, R.; Valencia-Cantero, E. Medicago truncatula Increases Its Iron-Uptake Mechanisms in Response to Volatile Organic Compounds Produced by Sinorhizobium meliloti . Folia Microbiol. (Praha) 2013 , 58 , 579–585. [ Google Scholar ] [ CrossRef ]
- Lemfack, M.C.; Nickel, J.; Dunkel, M.; Preissner, R.; Piechulla, B. MVOC: A Database of Microbial Volatiles. Nucleic Acids Res. 2014 , 42 , D744–D748. [ Google Scholar ] [ CrossRef ]
- Yasmin, H.; Rashid, U.; Hassan, M.N.; Nosheen, A.; Naz, R.; Ilyas, N.; Sajjad, M.; Azmat, A.; Alyemeni, M.N. Volatile Organic Compounds Produced by Pseudomonas pseudoalcaligenes Alleviated Drought Stress by Modulating Defense System in Maize ( Zea mays L.). Physiol. Plant. 2021 , 172 , 896–911. [ Google Scholar ] [ CrossRef ]
- Sudha, A.; Durgadevi, D.; Archana, S.; Muthukumar, A.; Suthin Raj, T.; Nakkeeran, S.; Poczai, P.; Nasif, O.; Ansari, M.J.; Sayyed, R.Z. Unraveling the Tripartite Interaction of Volatile Compounds of Streptomyces rochei with Grain Mold Pathogens Infecting Sorghum. Front. Microbiol. 2022 , 13 , 923360. [ Google Scholar ] [ CrossRef ]
- Poveda, J. Beneficial Effects of Microbial Volatile Organic Compounds (MVOCs) in Plants. Appl. Soil Ecol. 2021 , 168 , 104118. [ Google Scholar ] [ CrossRef ]
- Lemfack, M.C.; Gohlke, B.-O.; Toguem, S.M.T.; Preissner, S.; Piechulla, B.; Preissner, R. MVOC 2.0: A Database of Microbial Volatiles. Nucleic Acids Res. 2018 , 46 , D1261–D1265. [ Google Scholar ] [ CrossRef ] [ PubMed ]
- Giorgio, A.; De Stradis, A.; Lo Cantore, P.; Iacobellis, N.S. Biocide Effects of Volatile Organic Compounds Produced by Potential Biocontrol Rhizobacteria on Sclerotinia sclerotiorum . Front. Microbiol. 2015 , 6 , 1056. [ Google Scholar ] [ CrossRef ] [ PubMed ]
- Rybakova, D.; Rack-Wetzlinger, U.; Cernava, T.; Schaefer, A.; Schmuck, M.; Berg, G. Aerial Warfare: A Volatile Dialogue between the Plant Pathogen Verticillium longisporum and Its Antagonist Paenibacillus polymyxa . Front. Plant Sci. 2017 , 8 , 1294. [ Google Scholar ] [ CrossRef ]
- Kai, M.; Effmert, U.; Berg, G.; Piechulla, B. Volatiles of Bacterial Antagonists Inhibit Mycelial Growth of the Plant Pathogen Rhizoctonia solani . Arch. Microbiol. 2007 , 187 , 351–360. [ Google Scholar ] [ CrossRef ]
- Rojas-Solís, D.; Zetter-Salmón, E.; Contreras-Pérez, M.; Rocha-Granados, M.d.C.; Macías-Rodríguez, L.; Santoyo, G. Pseudomonas stutzeri E25 and Stenotrophomonas maltophilia CR71 Endophytes Produce Antifungal Volatile Organic Compounds and Exhibit Additive Plant Growth-Promoting Effects. Biocatal. Agric. Biotechnol. 2018 , 13 , 46–52. [ Google Scholar ] [ CrossRef ]
- Riyazuddin, R.; Verma, R.; Singh, K.; Nisha, N.; Keisham, M.; Bhati, K.K.; Kim, S.T.; Gupta, R. Ethylene: A Master Regulator of Salinity Stress Tolerance in Plants. Biomolecules 2020 , 10 , 959. [ Google Scholar ] [ CrossRef ]
- Burg, S.P.; Burg, E.A. Molecular Requirements for the Biological Activity of Ethylene. Plant Physiol. 1967 , 42 , 144–152. [ Google Scholar ] [ CrossRef ]
- Guzmán, P.; Ecker, J.R. Exploiting the Triple Response of Arabidopsis to Identify Ethylene-Related Mutants. Plant Cell 1990 , 2 , 513–523. [ Google Scholar ] [ CrossRef ]
- Ali, S.; Charles, T.C.; Glick, B.R. Amelioration of High Salinity Stress Damage by Plant Growth-Promoting Bacterial Endophytes That Contain ACC Deaminase. Plant Physiol. Biochem. 2014 , 80 , 160–167. [ Google Scholar ] [ CrossRef ]
- Gómez-Cadenas, A.; Tadeo, F.R.; Primo-Millo, E.; Talon, M. Involvement of Abscisic Acid and Ethylene in the Responses of Citrus Seedlings to Salt Shock. Physiol. Plant. 1998 , 103 , 475–484. [ Google Scholar ] [ CrossRef ]
- Arraes, F.B.M.; Beneventi, M.A.; Lisei de Sa, M.E.; Paixao, J.F.R.; Albuquerque, E.V.S.; Marin, S.R.R.; Purgatto, E.; Nepomuceno, A.L.; Grossi-de-Sa, M.F. Implications of Ethylene Biosynthesis and Signaling in Soybean Drought Stress Tolerance. BMC Plant Biol. 2015 , 15 , 213. [ Google Scholar ] [ CrossRef ] [ PubMed ]
- Gupta, R.; Min, C.W.; Kim, S.W.; Yoo, J.S.; Moon, A.-R.; Shin, A.-Y.; Kwon, S.-Y.; Kim, S.T. A TMT-Based Quantitative Proteome Analysis to Elucidate the TSWV Induced Signaling Cascade in Susceptible and Resistant Cultivars of Solanum lycopersicum . Plants 2020 , 9 , 290. [ Google Scholar ] [ CrossRef ] [ PubMed ]
- Gupta, R.; Min, C.W.; Kim, Y.-J.; Kim, S.T. Identification of Msp1-Induced Signaling Components in Rice Leaves by Integrated Proteomic and Phosphoproteomic Analysis. Int. J. Mol. Sci. 2019 , 20 , 4135. [ Google Scholar ] [ CrossRef ] [ PubMed ]
- Glick, B.R. Modulation of Plant Ethylene Levels by the Bacterial Enzyme ACC Deaminase. FEMS Microbiol. Lett. 2005 , 251 , 1–7. [ Google Scholar ] [ CrossRef ]
- Munns, R.; Tester, M. Mechanisms of Salinity Tolerance. Annu. Rev. Plant Biol. 2008 , 59 , 651–681. [ Google Scholar ] [ CrossRef ]
- Siddikee, M.A.; Chauhan, P.S.; Sa, T. Regulation of Ethylene Biosynthesis Under Salt Stress in Red Pepper ( Capsicum annuum L.) by 1-Aminocyclopropane-1-Carboxylic Acid (ACC) Deaminase-Producing Halotolerant Bacteria. J. Plant Growth Regul. 2012 , 31 , 265–272. [ Google Scholar ] [ CrossRef ]
- Ma, W.; Charles, T.C.; Glick, B.R. Expression of an Exogenous 1-Aminocyclopropane-1-Carboxylate Deaminase Gene in Sinorhizobium Meliloti Increases Its Ability To Nodulate Alfalfa. Appl. Environ. Microbiol. 2004 , 70 , 5891–5897. [ Google Scholar ] [ CrossRef ]
- Nascimento, F.X.; Brígido, C.; Glick, B.R.; Oliveira, S. ACC Deaminase Genes Are Conserved among Mesorhizobium Species Able to Nodulate the Same Host Plant. FEMS Microbiol. Lett. 2012 , 336 , 26–37. [ Google Scholar ] [ CrossRef ]
- Ma, W.; Sebestianova, S.B.; Sebestian, J.; Burd, G.I.; Guinel, F.C.; Glick, B.R. Prevalence of 1-Aminocyclopropane-1-Carboxylate Deaminase in Rhizobium Spp. Antonie Van Leeuwenhoek 2003 , 83 , 285–291. [ Google Scholar ] [ CrossRef ]
- Sun, Y.; Cheng, Z.; Glick, B.R. The Presence of a 1-Aminocyclopropane-1-Carboxylate (ACC) Deaminase Deletion Mutation Alters the Physiology of the Endophytic Plant Growth-Promoting Bacterium Burkholderia Phytofirmans PsJN. FEMS Microbiol. Lett. 2009 , 296 , 131–136. [ Google Scholar ] [ CrossRef ] [ PubMed ]
- Ali, S.; Charles, T.C.; Glick, B.R. Delay of Flower Senescence by Bacterial Endophytes Expressing 1-Aminocyclopropane-1-Carboxylate Deaminase. J. Appl. Microbiol. 2012 , 113 , 1139–1144. [ Google Scholar ] [ CrossRef ] [ PubMed ]
- Nascimento, F.X.; Rossi, M.J.; Soares, C.R.F.S.; McConkey, B.J.; Glick, B.R. New Insights into 1-Aminocyclopropane-1-Carboxylate (ACC) Deaminase Phylogeny, Evolution and Ecological Significance. PLoS ONE 2014 , 9 , e99168. [ Google Scholar ] [ CrossRef ] [ PubMed ]
- Glick, B.R. Bacteria with ACC Deaminase Can Promote Plant Growth and Help to Feed the World. Microbiol. Res. 2014 , 169 , 30–39. [ Google Scholar ] [ CrossRef ]
- Sheehy, R.E.; Honma, M.; Yamada, M.; Sasaki, T.; Martineau, B.; Hiatt, W.R. Isolation, Sequence, and Expression in Escherichia coli of the Pseudomonas Sp. Strain ACP Gene Encoding 1-Aminocyclopropane-1-Carboxylate Deaminase. J. Bacteriol. 1991 , 173 , 5260–5265. [ Google Scholar ] [ CrossRef ]
- Jacobson, C.B.; Pasternak, J.J.; Glick, B.R. Partial Purification and Characterization of 1-Aminocyclopropane-1-Carboxylate Deaminase from the Plant Growth Promoting Rhizobacterium Pseudomonas putida GR12-2. Can. J. Microbiol. 1994 , 40 , 1019–1025. [ Google Scholar ] [ CrossRef ]
- Hontzeas, N.; Zoidakis, J.; Glick, B.R.; Abu-Omar, M.M. Expression and Characterization of 1-Aminocyclopropane-1-Carboxylate Deaminase from the Rhizobacterium Pseudomonas putida UW4: A Key Enzyme in Bacterial Plant Growth Promotion. Biochim. Biophys. Acta Proteins Proteomics 2004 , 1703 , 11–19. [ Google Scholar ] [ CrossRef ]
- Khalifa, A. ACC Deaminase-Containing Rhizobacteria from Rhizosphere of Zygophyllum coccineum Alleviate Salt Stress Impact on Wheat ( Triticum aestivum L.). Basic Appl. Sci. Sci. J. King Faisal Univ. 2020 , 21 , 89–101. [ Google Scholar ] [ CrossRef ]
- Ali, S.; Glick, B.R. Plant–Bacterial Interactions in Management of Plant Growth under Abiotic Stresses. In New and Future Developments in Microbial Biotechnology and Bioengineering ; Elsevier: Amsterdam, The Netherlands, 2019; pp. 21–45. [ Google Scholar ]
- Araya, M.A.; Valenzuela, T.; Inostroza, N.G.; Maruyama, F.; Jorquera, M.A.; Acuña, J.J. Isolation and Characterization of Cold-Tolerant Hyper-ACC-Degrading Bacteria from the Rhizosphere, Endosphere, and Phyllosphere of Antarctic Vascular Plants. Microorganisms 2020 , 8 , 1788. [ Google Scholar ] [ CrossRef ]
- Bal, H.B.; Adhya, T.K. Alleviation of Submergence Stress in Rice Seedlings by Plant Growth-Promoting Rhizobacteria With ACC Deaminase Activity. Front. Sustain. Food Syst. 2021 , 5 , 606158. [ Google Scholar ] [ CrossRef ]
- Cheng, Z.; Park, E.; Glick, B.R. 1-Aminocyclopropane-1-Carboxylate Deaminase from Pseudomonas putida UW4 Facilitates the Growth of Canola in the Presence of Salt. Can. J. Microbiol. 2007 , 53 , 912–918. [ Google Scholar ] [ CrossRef ] [ PubMed ]
- Belimov, A.A.; Dodd, I.C.; Hontzeas, N.; Theobald, J.C.; Safronova, V.I.; Davies, W.J. Rhizosphere Bacteria Containing 1-aminocyclopropane-1-carboxylate Deaminase Increase Yield of Plants Grown in Drying Soil via Both Local and Systemic Hormone Signalling. New Phytol. 2009 , 181 , 413–423. [ Google Scholar ] [ CrossRef ] [ PubMed ]
- Moon, Y.S.; Ali, S. Possible Mechanisms for the Equilibrium of ACC and Role of ACC Deaminase-Producing Bacteria. Appl. Microbiol. Biotechnol. 2022 , 106 , 877–887. [ Google Scholar ] [ CrossRef ] [ PubMed ]
- Ma, W.; Guinel, F.C.; Glick, B.R. Rhizobium Leguminosarum Biovar Viciae 1-Aminocyclopropane-1-Carboxylate Deaminase Promotes Nodulation of Pea Plants. Appl. Environ. Microbiol. 2003 , 69 , 4396–4402. [ Google Scholar ] [ CrossRef ]
- Nukui, N.; Minamisawa, K.; Ayabe, S.-I.; Aoki, T. Expression of the 1-Aminocyclopropane-1-Carboxylic Acid Deaminase Gene Requires Symbiotic Nitrogen-Fixing Regulator Gene NifA2 in Mesorhizobium Loti MAFF303099. Appl. Environ. Microbiol. 2006 , 72 , 4964–4969. [ Google Scholar ] [ CrossRef ]
- Alemneh, A.A.; Zhou, Y.; Ryder, M.H.; Denton, M.D. Is Phosphate Solubilizing Ability in Plant Growth-promoting Rhizobacteria Isolated from Chickpea Linked to Their Ability to Produce ACC Deaminase? J. Appl. Microbiol. 2021 , 131 , 2416–2432. [ Google Scholar ] [ CrossRef ]
- Gamalero, E.; Glick, B.R. Bacterial Modulation of Plant Ethylene Levels. Plant Physiol. 2015 , 169 , 13–22. [ Google Scholar ] [ CrossRef ]
- Barnawal, D.; Bharti, N.; Maji, D.; Chanotiya, C.S.; Kalra, A. 1-Aminocyclopropane-1-Carboxylic Acid (ACC) Deaminase-Containing Rhizobacteria Protect Ocimum sanctum Plants during Waterlogging Stress via Reduced Ethylene Generation. Plant Physiol. Biochem. 2012 , 58 , 227–235. [ Google Scholar ] [ CrossRef ]
- Glick, B.R.; Cheng, Z.; Czarny, J.; Duan, J. Promotion of Plant Growth by ACC Deaminase-Producing Soil Bacteria. In New Perspectives and Approaches in Plant Growth-Promoting Rhizobacteria Research ; Springer Netherlands: Dordrecht, The Netherlands, 2007; pp. 329–339. [ Google Scholar ]
- Pierik, R.; Tholen, D.; Poorter, H.; Visser, E.J.W.; Voesenek, L.A.C.J. The Janus Face of Ethylene: Growth Inhibition and Stimulation. Trends Plant Sci. 2006 , 11 , 176–183. [ Google Scholar ] [ CrossRef ]
- van Loon, L.C.; Geraats, B.P.J.; Linthorst, H.J.M. Ethylene as a Modulator of Disease Resistance in Plants. Trends Plant Sci. 2006 , 11 , 184–191. [ Google Scholar ] [ CrossRef ]
- Robison, M.M.; Griffith, M.; Pauls, K.P.; Glick, B.R. Dual Role for Ethylene in Susceptibility of Tomato to Verticillium Wilt. J. Phytopathol. 2001 , 149 , 385–388. [ Google Scholar ] [ CrossRef ]
- Glick, B.R.; Penrose, D.M.; Li, J. A Model For the Lowering of Plant Ethylene Concentrations by Plant Growth-Promoting Bacteria. J. Theor. Biol. 1998 , 190 , 63–68. [ Google Scholar ] [ CrossRef ]
- Prayitno, J.; Rolfe, B.G.; Mathesius, U. The Ethylene-Insensitive Sickle Mutant of Medicago Truncatula Shows Altered Auxin Transport Regulation during Nodulation. Plant Physiol. 2006 , 142 , 168–180. [ Google Scholar ] [ CrossRef ] [ PubMed ]
- Stearns, J.C.; Woody, O.Z.; McConkey, B.J.; Glick, B.R. Effects of Bacterial ACC Deaminase on Brassica Napus Gene Expression. Mol. Plant-Microbe Interact. 2012 , 25 , 668–676. [ Google Scholar ] [ CrossRef ] [ PubMed ]
- Adie, B.; Chico, J.M.; Rubio-Somoza, I.; Solano, R. Modulation of Plant Defenses by Ethylene. J. Plant Growth Regul. 2007 , 26 , 160–177. [ Google Scholar ] [ CrossRef ]
- Robert-Seilaniantz, A.; Navarro, L.; Bari, R.; Jones, J.D. Pathological Hormone Imbalances. Curr. Opin. Plant Biol. 2007 , 10 , 372–379. [ Google Scholar ] [ CrossRef ]
- Cao, H.; Li, X.; Dong, X. Generation of Broad-Spectrum Disease Resistance by Overexpression of an Essential Regulatory Gene in Systemic Acquired Resistance. Proc. Natl. Acad. Sci. USA 1998 , 95 , 6531–6536. [ Google Scholar ] [ CrossRef ]
- Koornneef, A.; Pieterse, C.M.J. Cross Talk in Defense Signaling. Plant Physiol. 2008 , 146 , 839–844. [ Google Scholar ] [ CrossRef ]
- Grant, M.; Lamb, C. Systemic Immunity. Curr. Opin. Plant Biol. 2006 , 9 , 414–420. [ Google Scholar ] [ CrossRef ]
- Pieterse, C.M.J.; Zamioudis, C.; Berendsen, R.L.; Weller, D.M.; Van Wees, S.C.M.; Bakker, P.A.H.M. Induced Systemic Resistance by Beneficial Microbes. Annu. Rev. Phytopathol. 2014 , 52 , 347–375. [ Google Scholar ] [ CrossRef ]
- van Wees, S.C.; Luijendijk, M.; Smoorenburg, I.; van Loon, L.C.; Pieterse, C.M. Rhizobacteria-Mediated Induced Systemic Resistance (ISR) in Arabidopsis Is Not Associated with a Direct Effect on Expression of Known Defense-Related Genes but Stimulates the Expression of the Jasmonate-Inducible Gene Atvsp upon Challenge. Plant Mol. Biol. 1999 , 41 , 537–549. [ Google Scholar ] [ CrossRef ] [ PubMed ]
- Pieterse, C.M.J.; van Wees, S.C.M.; van Pelt, J.A.; Knoester, M.; Laan, R.; Gerrits, H.; Weisbeek, P.J.; van Loon, L.C. A Novel Signaling Pathway Controlling Induced Systemic Resistance in Arabidopsis . Plant Cell 1998 , 10 , 1571–1580. [ Google Scholar ] [ CrossRef ] [ PubMed ]
- Ahn, I.-P.; Lee, S.-W.; Suh, S.-C. Rhizobacteria-Induced Priming in Arabidopsis Is Dependent on Ethylene, Jasmonic Acid, and NPR1. Mol. Plant-Microbe Interact. 2007 , 20 , 759–768. [ Google Scholar ] [ CrossRef ]
- Tran, H.; Ficke, A.; Asiimwe, T.; Höfte, M.; Raaijmakers, J.M. Role of the Cyclic Lipopeptide Massetolide A in Biological Control of Phytophthora Infestans and in Colonization of Tomato Plants by Pseudomonas fluorescens . New Phytol. 2007 , 175 , 731–742. [ Google Scholar ] [ CrossRef ]
- Verhagen, B.W.M.; Glazebrook, J.; Zhu, T.; Chang, H.-S.; van Loon, L.C.; Pieterse, C.M.J. The Transcriptome of Rhizobacteria-Induced Systemic Resistance in Arabidopsis . Mol. Plant-Microbe Interact. 2004 , 17 , 895–908. [ Google Scholar ] [ CrossRef ]
- Cartieaux, F.; Contesto, C.; Gallou, A.; Desbrosses, G.; Kopka, J.; Taconnat, L.; Renou, J.-P.; Touraine, B. Simultaneous Interaction of Arabidopsis thaliana with Bradyrhizobium Sp. Strain ORS278 and Pseudomonas syringae Pv. Tomato DC3000 Leads to Complex Transcriptome Changes. Mol. Plant-Microbe Interact. 2008 , 21 , 244–259. [ Google Scholar ] [ CrossRef ] [ PubMed ]
- Yan, Z.; Reddy, M.S.; Ryu, C.-M.; McInroy, J.A.; Wilson, M.; Kloepper, J.W. Induced Systemic Protection Against Tomato Late Blight Elicited by Plant Growth-Promoting Rhizobacteria. Phytopathology® 2002 , 92 , 1329–1333. [ Google Scholar ] [ CrossRef ]
- De Vleesschauwer, D.; Djavaheri, M.; Bakker, P.A.H.M.; Höfte, M. Pseudomonas Fluorescens WCS374r-Induced Systemic Resistance in Rice against Magnaporthe Oryzae Is Based on Pseudobactin-Mediated Priming for a Salicylic Acid-Repressible Multifaceted Defense Response. Plant Physiol. 2008 , 148 , 1996–2012. [ Google Scholar ] [ CrossRef ]
- Segarra, G.; Van der Ent, S.; Trillas, I.; Pieterse, C.M.J. MYB72, a Node of Convergence in Induced Systemic Resistance Triggered by a Fungal and a Bacterial Beneficial Microbe. Plant Biol. 2009 , 11 , 90–96. [ Google Scholar ] [ CrossRef ]
- Glazebrook, J. Contrasting Mechanisms of Defense Against Biotrophic and Necrotrophic Pathogens. Annu. Rev. Phytopathol. 2005 , 43 , 205–227. [ Google Scholar ] [ CrossRef ]
- Van der Ent, S.; Van Wees, S.C.M.; Pieterse, C.M.J. Jasmonate Signaling in Plant Interactions with Resistance-Inducing Beneficial Microbes. Phytochemistry 2009 , 70 , 1581–1588. [ Google Scholar ] [ CrossRef ]
- Glick, B.R.; Jacobson, C.B.; Schwarze, M.M.K.; Pasternak, J.J. 1-Aminocyclopropane-1-Carboxylic Acid Deaminase Mutants of the Plant Growth Promoting Rhizobacterium Pseudomonas putida GR12-2 Do Not Stimulate Canola Root Elongation. Can. J. Microbiol. 1994 , 40 , 911–915. [ Google Scholar ] [ CrossRef ]
- Cheng, Z.; Duncker, B.P.; McConkey, B.J.; Glick, B.R. Transcriptional Regulation of ACC Deaminase Gene Expression in Pseudomonas putida UW4. Can. J. Microbiol. 2008 , 54 , 128–136. [ Google Scholar ] [ CrossRef ] [ PubMed ]
- Duan, J.; Müller, K.M.; Charles, T.C.; Vesely, S.; Glick, B.R. 1-Aminocyclopropane-1-Carboxylate (ACC) Deaminase Genes in Rhizobia from Southern Saskatchewan. Microb. Ecol. 2009 , 57 , 423–436. [ Google Scholar ] [ CrossRef ] [ PubMed ]
- Bouffaud, M.-L.; Renoud, S.; Dubost, A.; Moënne-Loccoz, Y.; Muller, D. 1-Aminocyclopropane-1-Carboxylate Deaminase Producers Associated to Maize and Other Poaceae Species. Microbiome 2018 , 6 , 114. [ Google Scholar ] [ CrossRef ]
- Jha, B.; Gontia, I.; Hartmann, A. The Roots of the Halophyte Salicornia brachiata Are a Source of New Halotolerant Diazotrophic Bacteria with Plant Growth-Promoting Potential. Plant Soil 2012 , 356 , 265–277. [ Google Scholar ] [ CrossRef ]
- Sorty, A.M.; Ntana, F.; Hansen, M.; Stougaard, P. Plant-Root Exudate Analogues Influence Activity of the 1-Aminocyclopropane-1-Carboxylate (ACC) Deaminase Gene in Pseudomonas hormoni G20-18T. Microorganisms 2023 , 11 , 2504. [ Google Scholar ] [ CrossRef ]
- Vega-Celedón, P.; Bravo, G.; Velásquez, A.; Cid, F.P.; Valenzuela, M.; Ramírez, I.; Vasconez, I.-N.; Álvarez, I.; Jorquera, M.A.; Seeger, M. Microbial Diversity of Psychrotolerant Bacteria Isolated from Wild Flora of Andes Mountains and Patagonia of Chile towards the Selection of Plant Growth-Promoting Bacterial Consortia to Alleviate Cold Stress in Plants. Microorganisms 2021 , 9 , 538. [ Google Scholar ] [ CrossRef ]
- Heydarian, Z.; Gruber, M.; Coutu, C.; Glick, B.R.; Hegedus, D.D. Gene Expression Patterns in Shoots of Camelina Sativa with Enhanced Salinity Tolerance Provided by Plant Growth Promoting Bacteria Producing 1-Aminocyclopropane-1-Carboxylate Deaminase or Expression of the Corresponding AcdS Gene. Sci. Rep. 2021 , 11 , 4260. [ Google Scholar ] [ CrossRef ]
- Guo, D.-J.; Singh, R.K.; Singh, P.; Li, D.-P.; Sharma, A.; Xing, Y.-X.; Song, X.-P.; Yang, L.-T.; Li, Y.-R. Complete Genome Sequence of Enterobacter roggenkampii ED5, a Nitrogen Fixing Plant Growth Promoting Endophytic Bacterium With Biocontrol and Stress Tolerance Properties, Isolated From Sugarcane Root. Front. Microbiol. 2020 , 11 , 580081. [ Google Scholar ] [ CrossRef ]
- Fernández-Llamosas, H.; Ibero, J.; Thijs, S.; Imperato, V.; Vangronsveld, J.; Díaz, E.; Carmona, M. Enhancing the Rice Seedlings Growth Promotion Abilities of Azoarcus Sp. CIB by Heterologous Expression of ACC Deaminase to Improve Performance of Plants Exposed to Cadmium Stress. Microorganisms 2020 , 8 , 1453. [ Google Scholar ] [ CrossRef ] [ PubMed ]
- Goswami, D.; Thakker, J.N.; Dhandhukia, P.C. Simultaneous Detection and Quantification of Indole-3-Acetic Acid (IAA) and Indole-3-Butyric Acid (IBA) Produced by Rhizobacteria from l-Tryptophan (Trp) Using HPTLC. J. Microbiol. Methods 2015 , 110 , 7–14. [ Google Scholar ] [ CrossRef ] [ PubMed ]
- Arund, J.; Luman, M.; Uhlin, F.; Tanner, R.; Fridolin, I. Is Fluorescence Valid to Monitor Removal of Protein Bound Uremic Solutes in Dialysis? PLoS ONE 2016 , 11 , e0156541. [ Google Scholar ] [ CrossRef ] [ PubMed ]
- Dworkin, M.; Foster, J.W. EXPERIMENTS WITH SOME MICROORGANISMS WHICH UTILIZE ETHANE AND HYDROGEN. J. Bacteriol. 1958 , 75 , 592–603. [ Google Scholar ] [ CrossRef ]
- Honma, M.; Shimomura, T. Metabolism of 1-Aminocyclopropane-1-Carboxylic Acid. Agric. Biol. Chem. 1978 , 42 , 1825–1831. [ Google Scholar ] [ CrossRef ]
Click here to enlarge figure
The statements, opinions and data contained in all publications are solely those of the individual author(s) and contributor(s) and not of MDPI and/or the editor(s). MDPI and/or the editor(s) disclaim responsibility for any injury to people or property resulting from any ideas, methods, instructions or products referred to in the content. |
Share and Cite
Timofeeva, A.M.; Galyamova, M.R.; Sedykh, S.E. How Do Plant Growth-Promoting Bacteria Use Plant Hormones to Regulate Stress Reactions? Plants 2024 , 13 , 2371. https://doi.org/10.3390/plants13172371
Timofeeva AM, Galyamova MR, Sedykh SE. How Do Plant Growth-Promoting Bacteria Use Plant Hormones to Regulate Stress Reactions? Plants . 2024; 13(17):2371. https://doi.org/10.3390/plants13172371
Timofeeva, Anna M., Maria R. Galyamova, and Sergey E. Sedykh. 2024. "How Do Plant Growth-Promoting Bacteria Use Plant Hormones to Regulate Stress Reactions?" Plants 13, no. 17: 2371. https://doi.org/10.3390/plants13172371
Article Metrics
Article access statistics, further information, mdpi initiatives, follow mdpi.
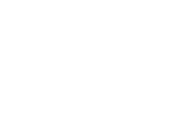
Subscribe to receive issue release notifications and newsletters from MDPI journals
Thank you for visiting nature.com. You are using a browser version with limited support for CSS. To obtain the best experience, we recommend you use a more up to date browser (or turn off compatibility mode in Internet Explorer). In the meantime, to ensure continued support, we are displaying the site without styles and JavaScript.
- View all journals
- Explore content
- About the journal
- Publish with us
- Sign up for alerts
- Original Article
- Published: 04 April 2017
The evolution of bacterial cell size: the internal diffusion-constraint hypothesis
- Romain Gallet 1 , 2 na1 ,
- Cyrille Violle 1 na1 ,
- Nathalie Fromin 1 ,
- Roula Jabbour-Zahab 1 ,
- Brian J Enquist 3 , 4 &
- Thomas Lenormand 1
The ISME Journal volume 11 , pages 1559–1568 ( 2017 ) Cite this article
5808 Accesses
27 Citations
17 Altmetric
Metrics details
- Theoretical ecology
Size is one of the most important biological traits influencing organismal ecology and evolution. However, we know little about the drivers of body size evolution in unicellulars. A long-term evolution experiment (Lenski’s LTEE) in which Escherichia coli adapts to a simple glucose medium has shown that not only the growth rate and the fitness of the bacterium increase over time but also its cell size. This increase in size contradicts prominent ‘external diffusion’ theory (EDC) predicting that cell size should have evolved toward smaller cells. Among several scenarios, we propose and test an alternative ‘internal diffusion-constraint’ (IDC) hypothesis for cell size evolution. A change in cell volume affects metabolite concentrations in the cytoplasm. The IDC states that a higher metabolism can be achieved by a reduction in the molecular traffic time inside of the cell, by increasing its volume. To test this hypothesis, we studied a population from the LTEE. We show that bigger cells with greater growth and CO 2 production rates and lower mass-to-volume ratio were selected over time in the LTEE. These results are consistent with the IDC hypothesis. This novel hypothesis offers a promising approach for understanding the evolutionary constraints on cell size.
Similar content being viewed by others
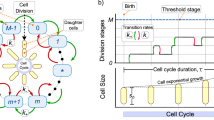
Mechanisms of cell size regulation in slow-growing Escherichia coli cells: discriminating models beyond the adder
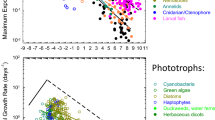
Evolutionary scaling of maximum growth rate with organism size
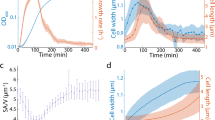
Precise regulation of the relative rates of surface area and volume synthesis in bacterial cells growing in dynamic environments
Introduction.
Constraints on evolution can have multiple causes, ranging from specific developmental mechanisms ( Maynard-Smith et al., 1985 ; Brakefield, 2006 ) to broad biophysical processes ( Haldane, 1926 ; LaBarbera, 1990 ; Barton and Partridge, 2000 ). The impact of biophysical constraints on evolution is particularly intriguing as they can shape the phenotypic diversity of life at very broad taxonomic scales, as shown in prominent ecological theories linking morphology and metabolism in metazoans ( West et al., 1997 , 1999 ; Enquist et al., 1999 ). Basic physical traits such as the mass and size of an organism play an important role in these theories. They influence how most biological structures, processes and dynamics covary with each other ( Peters, 1983 ). Here, we focus on the evolution of size in the bacteria Escherichia coli and on how the interplay of different biophysical constraints can drive its evolutionary trajectory.
Studying the allometric scaling between traits across species provides helpful information regarding the origin of biophysical constraint and their impact on the diversification of phenotypes ( Enquist et al., 2007 ). The dependence of a given biological trait, Y , on organismal size, M , is known as allometry. Allometric relationships are often characterized by power laws of the form, Y = Y 0 M b , where b is the scaling exponent and Y 0 is a normalization constant that may be characteristic of a given taxon ( Moses et al., 2008 ). Identifying allometric relationships has been key to elucidate the evolution of body size across the tree of life ( Okie et al., 2013 ). One of the most debated allometric relationships is between metabolic rate, B , and body size, M . For example, if the scaling of metabolic rate is governed by b <1, increases in size would then decrease the mass-specific metabolism where B / M ~ M 1− b . In many organisms, nutrients cannot simply diffuse through the body, there are some constraints limiting the diffusion and transport of nutrients. Metabolic Scaling Theory hypothesizes that the origin of the allometric exponent b results from selection to maximize resource delivery rates which is constrained by diffusion and transport constraints across hierarchical vascular networks ( West et al., 1997 , 1999 ). An open question is if Metabolic Scaling Theory can (i) apply to small, non-vascularized organisms like prokaryotes; and (ii) shed light on the evolution of size in unicellulars.
DeLong et al. (2010) highlighted that metabolic rates and growth rate ( Kempes et al., 2012 ; Kirchman, 2015 ) appear to have strongly covaried with cell size across prokaryote evolution, but the cause of why these scaling relationships appear to have varied have remained elusive. Two biophysical hypotheses for cell size evolution in bacteria were advanced. First, the ‘external diffusion constraints’ (EDC) hypothesis states that metabolic rates are limited by the rate of nutrient diffusion from the environment to the organism’s body. To increase this diffusion rate, selection will act primarily via changes in the cellular surface-to-volume ratio or cell size ( Koch, 1996 ; Schulz and Jorgensen, 2001 ; Young, 2006 ). The growth rate is often limited by the metabolic rate. Hence, higher metabolic rates are expected to be favored by natural selection whenever ecological conditions favors fast growth and fast resource depletion. If the diffusion of nutrients from the environment to the cell is the limiting step (EDC), then a bigger exchange surface (per unit volume of cytosol, that is, a higher surface-to-volume ratio) should be selected. Long-term evolution experiments (LTEEs) performed on microorganisms under various conditions indeed observed an increase in the growth rate associated with a reduction of cell size, resulting in a higher surface-to-volume ratio ( Spor et al., 2014 ; Gounand et al., 2016 ). Second, the ‘genome size’ hypothesis assumes (1) that bigger cells are required to pack more DNA and (2) that more DNA correlates with higher metabolic or division rates. To explain (2), Delong et al. (2010) hypothesize that bacteria with bigger genomes have more genes and thus supposedly larger biochemical networks, enabling the organism to metabolize a greater diversity of substrates. Another possibility to explain (2) is that the growth rate could be transcription limited. In that case, increasing the number of copies of the genome could favor a higher growth rate. Finally, (2) might occur because cell division rate might increase in cells with active replication forks and during times of DNA replication ( Weart et al., 2007 ).
In their LTEE, Lenski et al. (1991) and Lenski (2001) cultivated E. coli populations for more than 60 000 generations in a simple glucose medium. Along with an increase in fitness and growth rate, a spectacular evolutionary increase in cell size was observed ( Lenski and Travisano, 1994 ). Compared with the ancestral strain, evolved populations were composed of bigger but fewer cells, showing an overall higher total biomass ( Vasi et al., 1994 ). Contrary to the EDC hypothesis, a lower surface-to-volume ratio was selected for. Contrary to the ‘genome size’ hypothesis, changes in cell volume in the LTEE were not accompanied by any substantial change in the genome size or composition ( Barrick et al., 2009 ). Whether bigger cells from the LTEE contained more copies of the genome was not investigated at that time. The difficulty of explaining the evolution of cell size in the longest ongoing evolutionary experiment can be due to: (i) a focus on the evolution of cell volume whereas selection applies on several—probably interacting—traits (for example, cell mass, cell volume, molecular traffic time in the cell); (ii) unidentified constraints acting upon these traits, and (iii) the interplay of different, sometimes opposing, biophysical constraints.
Diffusion time is considered a pivotal constraint that governs the evolution of body size in metazoans. In this paper, we recast theoretical predictions regarding the evolution of cell size and mass in bacteria, introducing inside-to-the-cell diffusion constraints. Based on these predictions, we tested which combinations of biophysical and ecophysiological constraints are at play by specifically tracking the concomitant evolutionary change in per-capita dry mass and volume in one population from the LTEE, sampled at seven time points (between 0 and 40 000 generations). We re-measured relative fitness, maximum growth rate and cell volume (previously measured by Lenski et al. ) and measured additional size-related traits (dry mass, dry mass-to-volume ratio, DNA content per cell and volume-to-DNA content ratio) as well as metabolic rates (per-capita CO 2 production rate and energetic efficiency). These results are consistent with the internal diffusion constraint (IDC), highlighting that specific biophysical constraints may impact the evolution of cell size in unicellulars.
Materials and methods
Bacterial strains and medium.
The E. coli strains REL606, REL1164A, REL2179A, REL4536A, REL7177A, REL8593A and REL10938A used in this study correspond to clones from a single population (Ara-1) in Lenski’s E. coli LTEE ( Lenski et al., 1991 ) sampled at generation 0, 2000, 5000, 10 000, 15 000, 20 000, 40 000 respectively. In order to perform fitness measures, we introduced the cyan fluorescent protein marker (and yellow fluorescent protein for the ancestral strain REL606) at the RhaA locus in each of these seven strains as detailed in Gallet et al. (2012) .
All cultures were performed in DM250, that is, Davis minimal medium supplemented with 250 μg ml −1 glucose (7 g K 2 HPO 4 , 2 g KH 2 PO 4 , 1 g ammonium sulfate, 0.5 g sodium citrate; qsp 1000 ml H 2 O; pH was adjusted to 7.0 with HCl as necessary, 806 μl of MgSO 4 2− (1 m) , 1 ml of Thiamine 0.2% and 1.25 ml of glucose 20% were added after autoclaving). DM250 is equivalent to DM25 used by Lenski et al. (1991) , but with 10 times more glucose. DM250 was used to increase cell density in the cultures, in order to perform the following measurements with more precision. LB agar plates (10 g tryptone, 5 g yeast extract, 10 g NaCl and 15 g agar per liter of H 2 O) were used for cell concentration estimation.
Growth rate measurement
In the morning, for each of the seven strains, a colony was inoculated in a 50-ml Falcon tube containing 5 ml of DM250 and incubated at 37 °C with 200 r.p.m. agitation. In the late afternoon, 2 microliters of each culture was inoculated independently in one of the 60 wells at the center of a black 96-well plate (96F—not treated—Nunc), filled with 200 μl of DM250 (four to nine replicated wells/strain/plate). The 96-well plate was incubated at 37 °C in a TECAN Infinite 200 microtiter plate reader (Tecan, Männedorf, Switzerland), and fluorescence measured every 15 min. Maximal growth rate ( r max ) were obtained by fitting growth curves with the R grofit package. The whole experiment was replicated three times independently. Our measures of relative r max were well correlated ( r =0.94) to those performed on the 12 populations of the LTEE and reported in Cooper et al. (2001) .
Relative fitness measures
The relative fitness of each mutant was estimated by measuring the change in its relative frequency in competition experiments against the ancestor. After an overnight pre-culture, competitions were performed in at least eight independent replicated wells in a 96-well microtiter plate in DM250 at 37 °C. The relative frequency of each competitor was estimated by measuring both cyan fluorescent protein and yellow fluorescent protein fluorescence at the beginning and the end of the assay. For an extended description of this fitness measure, see the Electronic Supplementary Material .
Cell volume measurement
Cell size was estimated by flow cytometry. All strains were first grown overnight in DM250 at 37 °C under 240 r.p.m. The next day, 50 μl of each culture were used to inoculate 5 ml of DM250 in 50 ml falcon tubes. After 4 h incubation at 37 °C under 240 r.p.m., 950 μl of each culture were taken and mixed to 55 μl of formaldehyde 36% (final concentration=2%). The volume of fixed cells was then estimated by their forward scatter ( Volkmer and Heinemann, 2011 ) using a Gallios Beckman Coulter flow cytometer (Brea, CA, USA). In order to minimize potential skewing effects, the mode of forward scatter distributions was used as a descriptive measure of these distributions. The experiment was repeated six times independently. We converted forward scatter values to cell volumes by using three calibration polystyrene beads of volumes: 0.06, 4.19 and 20.6 μm 3 .
Cell DNA content measurement
The DNA content of individual cells was measured by flow cytometry. Cells in the exponential phase were fixed with ethanol (75% final concentration) and stained with propidium iodide. The average fluorescence of 10 000 single cells was measured. Cell size was also measured concomitantly in order to estimate the volume-to-DNA content ratio. Bacterial cells fixation and staining could have affected the estimation of cell size, compared with the previous estimation of this parameter. Supplementary Figure S1 shows that cell size measurements are strongly correlated.
Cell mass measurement
All strains were grown overnight in 1 l Erlenmeyer flasks containing 110 ml of DM250 at 37 °C under 240 r.p.m. agitation. A hundred milliliter of each culture were placed in two 50-ml Falcon tubes and centrifuged for 25 min at 7830 r.p.m. in an Eppendorf 5430R centrifuge (Hauppauge, NY, USA) at 7 °C. Pellets were re-suspended and placed in 1.5 ml microtubes for an extra centrifugation at 14 680 r.p.m. for 1 min in an Eppendorf 5424 centrifuge. Finally, pellets were re-suspended again in DM250, placed in 10.5 × 9 mm pressed tin capsules (Elemental Microanalysis D1104) and let to dry for at least 48 h at 70 °C. Tin capsules were weighed before and after adding the pellet on a Sartorius MC5 microbalance (1 μg readability). Dried pellet weights (total population biomass) corresponded to our measure of energetic efficiency, that is, the efficiency at which nutrients were converted into biomass. To estimate cell weight, dried pellet weights were divided by the cell number in 100 ml of cultures, which was estimated by optical density (OD) measurement (ODs were converted into cell number with standard curves—one standard curve per strain). The whole experiment was repeated three times independently. An independent experiment was run in order to estimate the number of cells per OD unit in DM250 for each strain (seven independent replicates per strain).
CO 2 production rate measurement
The per-capita CO 2 production rate (metabolic rate) was also estimated in this experiment. Bacteria were grown overnight in 50 ml Falcon tubes containing 5 ml DM250. The next day, ODs of all seven cultures were measured and 200 μl of each were inoculated in three independent sealed 150 ml plasma flasks containing 20 ml DM250 and closed by a cap. The flasks were incubated in a water bath at 37 °C. Air samples from the headspace of each flask were analyzed after 1 and 3 h incubation to quantify CO 2 concentration with a gas chromatograph using a catharometer (VARIAN GC 4900; Walnut Creek, CA, USA). OD was measured just after CO 2 measurements to ultimately assess the final number of cells for each sample. The amount of CO 2 released during this time allowed calculating CO 2 production rate per unit of OD, then per cell, expressed in mol CO 2 per cell per second.
Statistical analyses
All analyses were conducted in R ( http://www.r-project.org ). We analyzed scaling relationships between size-related traits and metabolic rates using log–log relationships to make them comparable with previously reported relationships ( DeLong et al., 2010 ) and more broadly, to infer metabolic hypotheses about the slope of these relationships ( West et al., 1997 , 2002 ). Standardized major axis regressions were performed with the R smatr package ( Warton et al., 2006 , 2012 ).
Recasting hypotheses for the evolution of bacterial cell size
Let us consider the scaling of metabolic rate M in a bacteria cell of size z . Let us consider that the metabolic rate is limited by glucose processing. We have
Indeed, this rate M has to be proportional to the number of glucose molecules that get in. This uptake can scale or not with the cell surface, depending on the mechanism. If it depends on transporters and if increasing the number of these transporters can be done without increasing cell surface, it is simply proportional to the number of transporters and independent of cell size. If on the contrary, it is necessary to increase cell surface to pack more transporters, or if only diffusion matters, then the uptake is proportional to the surface z 2 times a constant c 1 (uptake efficiency per unit surface), which provides a mechanism by which increased cell size could be favored. Since, we aim at explaining how larger cells could be favored, we follow the reasoning with this best-case scenario. Then, the rate M must depend on the number of metabolic effectors E that can process glucose (with a constant c 2 measuring enzymatic efficiency), times the rate of ‘encounter’ between glucose and effectors. We ignore here the time to produce and maintain these effectors as well as the nutrient uptake necessary to produce them, which is justified since the half-life of those enzymes is much longer than half-life of glucose. The rate of encounter between glucose and effectors can be measured by the inverse of travel time T of the glucose molecule to the enzyme. This travel time depends on the distance that needs to be traveled between the membrane and the effector that will process it. This distance necessarily scales with cell size z : all distances increase in a larger cell. For instance, the mean distance to the membrane of effectors uniformly spread in a spherical cell of radius z is z /4. The mean distance between E uniformly spread effectors is proportional to ( z 3 / E ) 1/3 = zE −1/3 . Hence, we note this distance fz , where f represents a size-independent variable that depends on the number and repartition of effectors. As long established (for example, Edelstein-Keshet, 1989 ), the distance traveled by a diffusive particle scales with the square root of time times a constant c 3 (that depends on the diffusion coefficient and the dimensionality), we thus have
Hence metabolic rate is simply
where C is size independent and equal to c 1 c 2 c 3 / f 2 . Note however that f may include power of E since travel time in the cytosol is likely to decrease with the number of effectors, so that the overall power of E in Equation (3) may be larger than one. This equation indicates that the metabolic rate does not directly depend on cell size since the increased input of glucose that increases with surface (size squared) is offset by the travel time of glucose which is also increasing with the square of size. This is the best-case scenario. When more glucose transporters can be added without the requirement of a larger cell surface, this rate would scale with the inverse of cell surface.
Hence, in the best-case scenario, increased M can be either achieved by a larger constant C or by increasing the number of effectors E , which is expected to increase weight. If there is a cost to build and maintain the membrane (pores, membrane transporters, etc.), it must increase with the surface of the membrane and hence the square of size. As a consequence, without considering variation in C , the expectation is that selection pressures to increase metabolic rates and/or the growth rate would consist in decreasing size (to reduce costs related to the membrane, while achieving the same metabolic rates) and increasing weight (that is, E , the number of effectors), until the marginal fitness benefit of increasing the number of effectors for increased metabolism, becomes equal to the fitness cost of production of new effector, or some spatial limit is reached to simply pack all essential molecules within the cell. There are two broad possible hypotheses to reverse this conclusion and expect an increase in cell size to increase metabolic rate: (1) the space limitation and (2) the IDC scenarios.
The space limitation scenario
A consequence of having more metabolic effectors and possibly more DNA is that the mass of the cell should increase as well. Thus, a tight and positive relationship between DNA content, cell volume, cell mass and metabolic rates should be observed. However, DNA content and/or cell weight should increase first in the LTEE and later (or at best concomitantly) cause an adjustment of cell size to maintain a nearly constant mass/volume or DNA content/volume ratio.
The IDC Scenario 1: molecular crowding
Reducing size and/or increasing the number of effectors may also change the physical properties of the cytosol. Increased macromolecular crowding in the cytosol (scaling as E /volume) is known to increase within-cell traffic times ( Ellis, 2001 ; Schulz and Jorgensen, 2001 ; Golding and Cox, 2006 ; Ando and Skolnick, 2010 ). As a first order approximation, let us assume that the glucose diffusion coefficient is linearly varying with E / z 3 , with a slope c 4 . Metabolic rates are now becoming dependent on size:
Hence, as long as glucose diffusion coefficient decreases when cytosol concentration (that is, macromolecular crowding) increases (that is, c 4 <0), metabolic rates are expected to increase with cell size. Diffusion coefficient of small molecules like glucose may not be strongly impacted by macromolecular crowding compared with larger ones ( Verkman, 2002 ). However, the same effect would occur if the metabolic rate is limited by diffusion of macromolecules (RNA, proteins). Overall, in this scenario, the mass-to-volume ratio is expected to decrease through time in the LTEE.
The IDC Scenario 2: cell shape
The idc scenario 3: cell compartmentalization.
In this scenario too, there might be a selection pressure to increase cell size in order to achieve a higher metabolic rate. Here, the weight-to-volume ratio is expected to decrease in the LTEE, and if observable, effectors should tend to occur closer to membranes in larger cells.
We found an increase in cell volume, cell dry mass, total population biomass, DNA content, volume-to-DNA content ratio, relative fitness, growth rate, per-capita CO 2 production rate over the 40 000 generations of the experiment ( Figure 1 ). They increased steadily through time (except for the volume-to-DNA content ratio which started to increase and then stayed relatively constant), with an acceleration between generation 20 000 and 40 000 for cell volume, DNA content and relative fitness. Meanwhile, the cell dry mass-to-volume ratio decreased over time. Growth rate, per-capita CO 2 production rate, total population biomass, DNA content and cell volume were positively correlated to fitness while the cell dry mass-to-volume ratio was negatively correlated ( Table 1 ). Cell dry mass increased less consistently than the other measured traits ( Figure 1b ) and was not correlated to fitness ( Table 1 ).
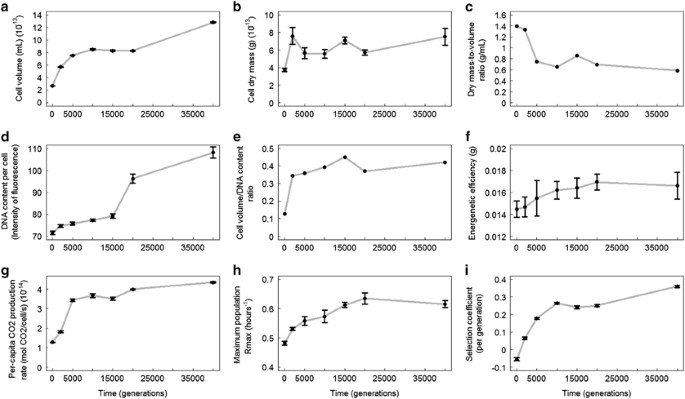
Trajectories of size-related ( a – e ), metabolic rates ( f, g ), maximum population growth rate ( r max ) ( h ) and selection coefficient ( i ) of the Ara-1 population in the LTEE. Each dot represents mean values (±s.e., when applicable).
Next, we analyzed scaling relationships between size-related traits and metabolic rates. We found no scaling relationship between per-capita CO 2 production rate and cell dry mass ( Figure 2a ) or per-capita CO 2 production rate and cell volume-to-DNA content ratio ( Figure 2d ). Conversely, we found a significant scaling relationship between per-capita CO 2 production rate and cell volume with a scaling slope not different from 1 ( Figure 2b ). The per-capita CO 2 production rate also scaled negatively with the mass-to-volume ratio ( Figure 2c ). We obtained similar trends with the scaling of maximum population growth rate ( r max ; Figures 2e–h ) and energetic efficiency (total biomass; Figures 2i–l ).
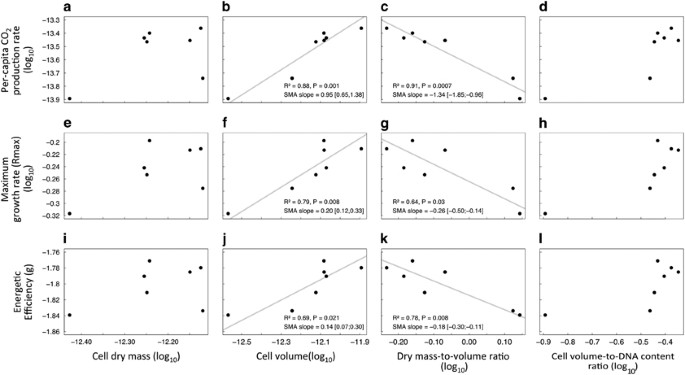
Scaling allometry relationships: size-related traits against CO 2 production rate ( a – d ), maximum population growth rate ( r max ) ( e – h ) and energetic efficiency ( i – l ). Standardized major axis regressions, appropriated to the analysis of scaling relationships ( Warton et al., 2006 , 2012 ), are shown when significant (gray lines). Variables were log-transformed for the study of allometric relationships (see Figure 1 for non-transformed values and unit information).
To understand which constraints are directing the evolution of cell size, we tracked the evolution of several key biological traits in the LTEE. These included dry mass, per-capita dry mass-to-volume ratio, total population biomass, DNA content, volume-to-DNA content ratio and CO 2 production rate traits that have not been explored previously in the LTEE or other experiments ( Hindré et al., 2012 ). This multi-trait approach allowed us to test our qualitative predictions about the biophysical and ecophysiological constraints that act on the evolutionary trajectory of cell size.
An increase in cell volume, growth rate and relative fitness were previously observed in the LTEE ( Lenski and Travisano, 1994 ; Wiser et al., 2013 ). Our results confirmed these observations. The fact that growth rate and cell volume were positively correlated to fitness also corroborates previous results showing that both traits are under selection in this experiment ( Lenski and Travisano, 1994 ; Mongold and Lenski, 1996 ). We attribute the acceleration in the increase of cell size and fitness to the occurrence of a mutator phenotype at generation 26 500 in this population ( Wiser et al., 2013 ). In addition, here we revealed a striking increase in the per-capita CO 2 production rate, energetic efficiency, cell mass, DNA content and volume-to-DNA ratio and decrease in cell mass-to-volume ratio over the course of the LTEE. Interestingly, CO 2 production rate, energetic efficiency, DNA content, volume-to-DNA content ratio and mass-to-volume ratio were highly correlated to fitness whereas cell mass was not ( Table 1 ).
The selection of a higher growth rate during the LTEE was probably due to the fact that the E. coli strain REL606 had to adapt from a lifestyle where it had to survived in stab cultures, to a new lifestyle where it was competing for glucose in a liquid culture medium ( Daegelen et al., 2009 ). In other words, this bacterial strain had to adapt to a new environment in which growth rate and fast resource consumption was under selection. Investigating scaling relationships between size-related traits and metabolic rates can provide insights into the biophysical and ecophysiological constraints that act on phenotypes ( Enquist et al., 2007 ; Glazier, 2010 ) and more broadly on the mechanisms that regulate body size evolution ( Kempes et al., 2012 ; Okie et al., 2013 ). Here, such analysis could help understand why cell size, metabolic rates and fitness correlate positively. Our analyses of metabolic scaling relationships in the LTEE highlighted a volume-based, rather than a mass-based ( DeLong et al., 2010 ), scaling for whole-cell metabolism (cell mass was seldom assessed in previous studies since a dry mass-to-volume ratio of one was systematically used to convert volume to dry mass ( DeLong et al., 2010 )). However, our results indicate that this assumption is not upheld in the E. coli . We used these metabolic scaling relationships to explore two alternatives, the space limitation and the IDC scenarios.
Under the space limitation scenario, space is the only factor limiting metabolic rates. Thus, if an increase in cell mass (more effectors) and/or DNA content is necessary to reach higher metabolic rates, cell volume should increase accordingly and the mass/volume and/or DNA/volume ratio stay nearly constant. Table 1 shows that cell mass does not correlate significantly with cell volume or metabolic rates which discard the hypothesis that cell size increased to only fit more effectors. However, Table 1 also shows significant positive correlations between DNA content, cell size and metabolic rates ( Table 1 ), which could indicate that the DNA content actually drove the increase in metabolic rates and cell size. Nevertheless, the evolutionary trajectory of the volume-to-DNA content ratio contradicts this conclusion. It shows that between generation 0 and 2000, that is, when the fitness increase was the most important, the volume of the cell increased three times faster than the DNA content. In other words, cell size did not only increase to fit more DNA, but for a different reason. Thus, the space limitation scenario (for DNA or effectors) is not sufficient to explain the increase in cell size that we observe. Overall, while the EDC and the space limitation scenarios seem to be good predictors of cell size of unicellular organisms at the interspecific level ( DeLong et al., 2010 ), our data show that these theories cannot fully explain the evolution of cell size in the LTEE.
As expected under the IDC scenario, we found that cell volume (× 4.76 after 40 000 generations) increased faster than cell mass (× 2.25), indicating that cell volume has not evolved simply to compensate the increase in cell mass. This shift of the cell mass-to-volume ratio scaled with all metabolic rates (CO 2 production rate, growth rate and metabolic efficiency) and fitness, suggesting that it was adaptive. This shift—in complete accordance with the predictions given by the IDC—occurred between generation 0 and 10 000, when the fitness and metabolic rates showed the highest increase. Everything else being equal, such a change in the dry mass-to-volume ratio could result in reduced macromolecular crowding in the cytosol (as the cell volume increased faster than the mass, see Equation (5)) or a better packing of metabolic effectors near membranes (as the membrane surface also increased faster than the mass, see Equation (6)). In both cases, such a change would shorten metabolite traffic time and hence increase metabolism and fitness ( Beveridge, 1988 ; Koch, 1996 ; Schulz and Jorgensen, 2001 ; Golding and Cox, 2006 ; Young, 2006 ; Beg et al., 2007 ; Ando and Skolnick, 2010 ; Dill et al., 2011 ). Thus, we interpret the strong increase in cell volume in the LTEE as resulting from internal diffusion constraints. Under the IDC hypothesis, cell volume would increase to (1) fit more effectors in the cell and (2) to reduce the dry mass-to-volume ratio (by a factor 2.11); two requirements to achieve higher metabolic rates. Selection would favor bigger cells, as packing more effectors than small ones would enable cells to better exploit and deplete glucose. As a result, the cell lag phase would then be reduced. In addition, such cells would have less concentrated cytoplasm allowing faster chemical reactions and higher metabolic efficiency. Further exploration of this idea would require fine-scale within-cell metabolic measures. Studying cell shape variation could also be worth investigating in the light of the theory outlined in this paper. In any case, our findings point to the importance of other physical constraints, rather than the usual constraints based on cell geometry associated with variation in the surface-to-volume ratio. Internal diffusion constraints may be particularly important when selection applies on growth rate, that is, when metabolic rates are important.
Our findings illustrate the combined importance of biophysical and ecophysiological constraints to understand the evolution of morphology ( Lynch et al., 2014 , and our Figure 3 ), in addition to the more classical evo-devo constraints ( Brakefield, 2006 ). Biophysical constraints related to allometric relationships are often considered as ‘universal’ constraints in interspecific comparative biology ( Enquist et al., 1999 ; West et al., 1997 , 1999 , 2002 ). They also seem to play a key role in the evolution of traits at the intraspecific level, as theoretically envisioned ( Shoval et al., 2012 ). Finally, our findings opens the intriguing possibility that, while body size of metazoans appears mostly constrained by external diffusion in allometry theories ( Damuth, 2001 ; Brown et al., 2004 ; DeLong et al., 2010 ; Glazier, 2010 ), cell size of microbes (bacteria, fungi, algae, etc.) would be constrained in a different way, by a combination of internal and external diffusion constraints. Such a difference is perhaps not entirely unexpected: in metazoans, each cell type has its optimal shape and size to perform a specific function, and increasing body size primarily requires increasing the number of cells. Therefore, the metabolism of metazoans is mostly limited by EDC, and the intensity of this constraint is proportional to the number of cells and vascularization. In unicellular organisms, where body size is the same as cell size, the metabolism is limited by EDC and IDC the effects of which are opposite on cell size. The balance between these two biophysical constraints may differ according to the ecology of the organism, or whether growth rate is under selection or not. Biophysical constraints may also vary between prokaryote and eukaryote unicellulars, since the latter have compartmentalized cytoplasms where reactions are likely to be less dependent on diffusion time in the cytosol. This theory may thus help to explain the differences observed for metabolic scaling with size in these different unicellular organisms ( DeLong et al., 2010 ), which opens a wide range of possible empirical tests.
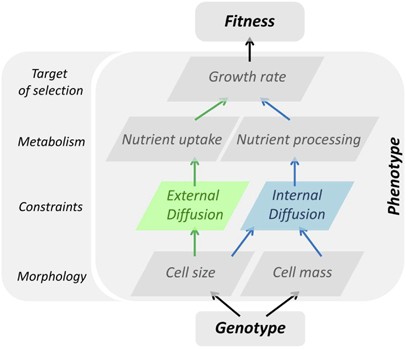
The role of biophysical constraints in the genotype to phenotype to fitness map. Sketch of the cascading effects on metabolism, growth rate and fitness of modification of cell morphology (size, mass). First, cell size modification changes the surface-to-volume ratio and, hence, nutrient uptake (green arrows). This is the consequence of a physical constraint caused by diffusion from/to the external environment. Second, both cell size and cell mass change cell density (blue arrows) and hence internal density that impacts the rate of nutrient processing (internal diffusion constraint due to inner molecular crowding). Both nutrient uptake and processing impact growth rate and fitness.
Ando T, Skolnick J . (2010). Crowding and hydrodynamic interactions likely dominate in vivo macromolecular motion. Proc Natl Acad Sci USA 107 : 18457–18462.
Article CAS Google Scholar
Barrick JE, Yu DS, Yoon SH, Jeong H, Oh TK, Schneider D et al . (2009). Genome evolution and adaptation in a long-term experiment with Escherichia coli . Nature 461 : 1243–1247.
Barton N, Partridge L . (2000). Limits to natural selection. BioEssays 22 : 1075–1084.
Beg QK, Vazquez A, Ernst J, de Menezes MA, Bar-Joseph Z, Barabási A-L et al . (2007). Intracellular crowding defines the mode and sequence of substrate uptake by Escherichia coli and constrains its metabolic activity. Proc Natl Acad Sci USA 104 : 12663–12668.
Beveridge T . (1988). The bacterial surface: general considerations towards design and function. Can J Microbiol 34 : 363–372.
Brakefield PM . (2006). Evo-devo and constraints on selection. Trends Ecol Evol 21 : 362–368.
Article Google Scholar
Brown JH, Gillooly JF, Allen AP, Savage VM, West GB . (2004). Toward a metabolic theory of ecology. Ecology 85 : 1771–1789.
Cooper VS, Bennett AF, Lenski RE . (2001). Evolution of thermal dependence of growth rate of Escherichia coli populations during 20000 generations in a constant environment. Evolution 55 : 889–896.
Daegelen P, Studier FW, Lenski RE, Cure S, Kim JF . (2009). Tracing ancestors and relatives of Escherichia coli B, and the derivation of B strains REL606 and BL21(DE3). J Mol Biol 394 : 634–643.
Damuth J . (2001). Scaling of growth: plants and animals are not so different. Proc Natl Acad Sci USA 98 : 2113–2114.
DeLong JP, Okie JG, Moses ME, Sibly RM, Brown JH . (2010). Shifts in metabolic scaling, production, and efficiency across major evolutionary transitions of life. Proc Natl Acad Sci USA 107 : 12941–12945.
Dill Ka, Ghosh K, Schmit JD . (2011). Inaugural article: Physical limits of cells and proteomes. Proc Natl Acad Sci USA 108 : 17876–17882.
Edelstein-Keshet L . (1989) Mathematical Models in Biology . McGraw-Hill: New York, NY, USA.
Google Scholar
Ellis RJ . (2001). Macromolecular crowding: an important but neglected aspect of the intracellular environment. Curr Opin Struct Biol 11 : 114–119.
Enquist BJ, Tiffney BH, Niklas KJ . (2007). Metabolic scaling and the evolutionary dynamics of plant size, form, and diversity: toward a synthesis of ecology, evolution, and paleontology. Int J Plant Sci 168 : 729–749.
Enquist BJ, West GB, Charnov EL, Brown JH, Santa T, Road HP et al . (1999). Allometric scaling of production and life history variation in vascular plants. Nature 401 : 907–911.
Gallet R, Cooper TF, Elena SF, Lenormand T . (2012). Measuring selection coefficients below 10 -3 : method, questions, and prospects. Genetics 190 : 175–186.
Glazier DS . (2010). A unifying explanation for diverse metabolic scaling in animals and plants. Biol Rev 85 : 111–138.
Golding I, Cox EC . (2006). Physical nature of bacterial cytoplasm. Phys Rev Lett 96 : 98102.
Gounand I, Daufresne T, Gravel D, Bouvier C, Bouvier T, Combe M et al . (2016). Size evolution in microorganisms masks trade-offs predicted by the growth rate hypothesis. Proc R Soc Lond B Biol Sci 283 : 20162272.
Haldane JBS . (1926). On being the right size. Harper’s Mag 1–5. Available at: http://harpers.org/archive/1926/03/ .
Hindré T, Knibbe C, Beslon G, Schneider D . (2012). New insights into bacterial adaptation through in vivo and in silico experimental evolution. Nat Rev Microbiol 10 : 352–365.
Kempes CP, Dutkiewicz S, Follows MJ . (2012). Growth, metabolic partitioning, and the size of microorganisms. Proc Natl Acad Sci USA 109 : 495–500.
Kirchman DL . (2015). Growth rates of microbes in the oceans. Ann Rev Mar Sci 8 : 1–25.
Koch A . (1996). What size should a bacterium be? A question of scale. Annu Rev Microbiol 50 : 317–348.
LaBarbera M . (1990). Principles of design of fluid transport systems in zoology. Science (80-) 249 : 992–1000.
Lenski RE . (2001). Testing Antonovics’ five tenets of ecological genetics: experiments with bacteria at the interface of ecology and genetics. In: Press MC, Huntly NJ, Levin S (eds). Ecology: Achievement and Challenge . Blackwell Science: Oxford, UK, pp 25–45.
Lenski RE, Rose MR, Simpson SC, Tadler SC . (1991). Long-term experimental evolution in Escherichia coli . I. Adaptation and divergence during 2000 generations. Am Nat 138 : 1315–1341.
Lenski RE, Travisano M . (1994). Dynamics of adaptation and diversification—a 10000 generation experiment with bacterial populations. Proc Natl Acad Sci USA 91 : 6808–6814.
Lynch M, Field MC, Goodson HV, Malik HS, Pereira-Leal JB, Roos DS et al . (2014). Evolutionary cell biology: two origins, one objective. Proc Natl Acad Sci USA 111 : 16990–16994.
Maynard-Smith J, Burian R, Kauffman S, Alberch P, Campbell J, Goodwin B et al . (1985). Developmental constraints and evolution. Q Rev Biol 60 : 265–287.
Mongold JA, Lenski RE . (1996). Experimental rejection of a nonadaptive explanation for increased cell size in Escherichia coli . J Bacteriol 178 : 5333–5334.
Moses ME, Hou C, Woodruff WH, West GB, Nekola JC, Zuo W et al . (2008). Revisiting a model of ontogenetic growth: estimating model parameters from theory and data. Am Nat 171 : 632–645.
Okie JG, Boyer AG, Brown JH, Costa DP, Ernest SKM, Evans AR et al . (2013). Effects of allometry, productivity and lifestyle on rates and limits of body size evolution. Proc R Soc B 280 : 20131007.
Peters R . (1983) The Ecological Implications of Body Size . Cambridge University press: Cambridge, UK.
Book Google Scholar
Schulz H, Jorgensen B . (2001). Big bacteria. Annu Rev Microbiol 55 : 105–137.
Shoval O, Sheftel H, Shinar G, Hart Y, Ramote O, Mayo A et al . (2012). Evolutionary trade-offs, pareto optimality, and the geometry of phenotype space. Science 336 : 1157–1160.
Spor A, Kvitek DJ, Nidelet T, Martin J, Legrand J, Dillmann C et al . (2014). Phenotypic and genotypic convergences are influenced by historical contingency and environment in yeast. Evolution 68 : 772–790.
Vasi F, Travisano M, Lenski RE . (1994). Long-term experimental evolution in Escherichia coli. II. Changes in life-history traits during adaptation to a seasonal environment. Am Nat 144 : 432–456.
Verkman AS . (2002). Solute and macromolecule diffusion in cellular aqueous compartments. Trends Biochem Sci 27 : 27–33.
Volkmer B, Heinemann M . (2011). Condition-dependent cell volume and concentration of Escherichia coli to facilitate data conversion for systems biology modeling. PLoS One 6 : e23126.
Warton DI, Duursma RA, Falster DS, Taskinen S . (2012). SMATR 3—an R package for estimation and inference about allometric lines. Methods Ecol Evol 3 : 257–259.
Warton DI, Wright IJ, Falster DS, Westoby M . (2006). Bivariate line-fitting methods for allometry. Biol Rev 81 : 259.
Weart RB, Lee AH, Chien AC, Haeusser DP, Hill NS, Levin PA . (2007). A metabolic sensor governing cell size in bacteria. Cell 130 : 335–347.
Welch GR, Easterby JS . (1994). Metabolic channeling versus free diffusion: Transition-time analysis. Trends Biochem Sci 19 : 193–197.
West GB, Brown JH, Enquist BJ . (1997). A general model for the origin of allometric scaling laws in biology. Science 276 : 122–126.
West GB, Enquist BJ, Brown JH . (1999). The fourth dimension of life: fractal geometry and allometric scaling of organisms. Science (80-) 284 : 1677–1679.
West GB, Woodruff WH, Brown JH . (2002). Allometric scaling of metabolic rate from molecules and mitochondria to cells and mammals. Proc Natl Acad Sci USA 99 : 2473–2478.
Wiser MJ, Ribeck N, Lenski RE . (2013). Long-term dynamics of adaptation in asexual populations. Science (80-) 342 : 1364–1367.
Young KD . (2006). The selective value of bacterial shape. Microbiol Mol Biol Rev 70 : 660–703.
Download references
Acknowledgements
We thank RE Lenski and D Schneider for sharing strains, RE Lenski, J DeLong and T Hindré and the anonymous reviewers for insightful comments on the manuscript and Hervé Isambert for insightful discussions. We thank Bruno Buatois for technical assistance and Marie Pierre Dubois for lab management (UMR 5175 CEFE, Campus CNRS). The CO 2 production analysis was performed at the Plateforme d’Analyses Chimiques en Ecologie technical facilities of the LabEx Centre Méditerranéen de l'Environnement et de la Biodiversité. Microbiological experiments were performed at the Service des Marqueurs Génétiques en Ecologie, CEFE. We thank Christophe Duperray for technical assistance on flow cytometry measurements performed at the RIO imaging platform of Montpellier—Institut de Recheche en Biothérapie. BJE was supported by a NSF Macrosystems award EF- 1065861. CV was supported by a Marie Curie International Outgoing Fellowship within the Seventh European Community Framework Program (DiversiTraits project, no. 221060) and by the European Research Council (ERC) Starting Grant Project ‘Ecophysiological and biophysical constraints on domestication in crop plants’ (Grant ERC-StG-2014-639706-CONSTRAINTS). RG and TL were supported by the QuantEvol ERC grant.
Author information
Romain Gallet and Cyrille Violle: These authors contributed equally to this work.
Authors and Affiliations
CEFE UMR 5175, CNRS, Université de Montpellier, Université Paul-Valéry Montpellier, EPHE, Montpellier Cedex 5, France
Romain Gallet, Cyrille Violle, Nathalie Fromin, Roula Jabbour-Zahab & Thomas Lenormand
UMR-BGPI-INRA-TA A-54/K Campus International de Baillarguet, Montpellier Cedex 5, France
Romain Gallet
Department of Ecology and Evolutionary Biology, University of Arizona, Tucson, AZ, USA
Brian J Enquist
Santa Fe Institute, Santa Fe, NM, USA
You can also search for this author in PubMed Google Scholar
Corresponding author
Correspondence to Romain Gallet .
Ethics declarations
Competing interests.
The authors declare no conflict of interest.
Additional information
Supplementary Information accompanies this paper on The ISME Journal website
Supplementary information
Supplementary information (docx 30 kb), rights and permissions.
Reprints and permissions
About this article
Cite this article.
Gallet, R., Violle, C., Fromin, N. et al. The evolution of bacterial cell size: the internal diffusion-constraint hypothesis. ISME J 11 , 1559–1568 (2017). https://doi.org/10.1038/ismej.2017.35
Download citation
Received : 21 September 2016
Revised : 13 January 2017
Accepted : 06 February 2017
Published : 04 April 2017
Issue Date : July 2017
DOI : https://doi.org/10.1038/ismej.2017.35
Share this article
Anyone you share the following link with will be able to read this content:
Sorry, a shareable link is not currently available for this article.
Provided by the Springer Nature SharedIt content-sharing initiative
This article is cited by
- Michael Lynch
- Bogi Trickovic
- Christopher P. Kempes
Scientific Reports (2022)
Primordial mimicry induces morphological change in Escherichia coli
- Honoka Aida
- Tetsuya Yomo
Communications Biology (2022)

Biological scaling in green algae: the role of cell size and geometry
- Helena Bestová
- Jules Segrestin
- Cyrille Violle
Scientific Reports (2021)
Quick links
- Explore articles by subject
- Guide to authors
- Editorial policies

- News & Opinion
Slow Bacterial Growth Enables Antibiotic Resistance
In salmonella , two seemingly similar antibiotic survival strategies result from very different molecular mechanisms..
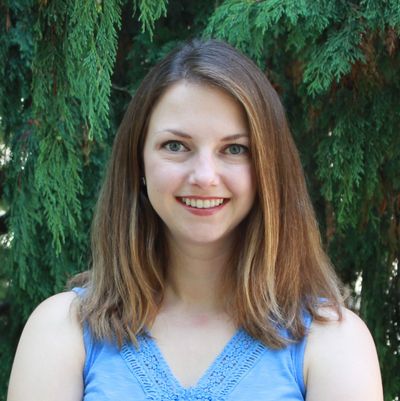
With a PhD in Genetics and Genomics, Niki channels her infectious disease research and science communication experiences into her role as a senior science editor for the TS Creative Services team.
View full profile.
Learn about our editorial policies.
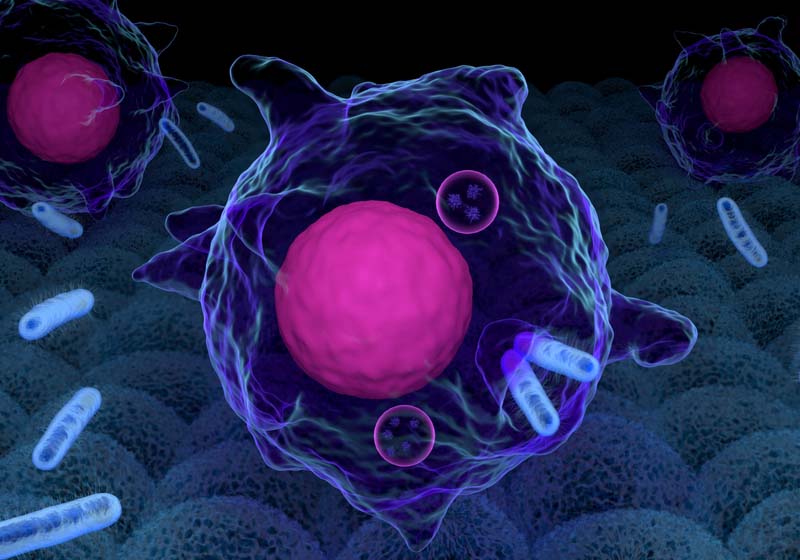
Bacteria that are resistant to antibiotics thrive in the face of drug treatment, which can lead to life threatening, often incurable infections. Other bacteria can exist in a pre-resistant state. To survive, slow bacterial growth allows mutations to arise that help the bugs tolerate the drug, or shifting phenotypes help them persist in its presence. Once the antibiotic treatment ends, the few remaining bacteria can grow again, restoring the infection. 1
Infections that can’t be treated are a significant problem. To search for ways to fight this growing health threat, Peter Hill and his colleagues at Harvard Medical School teased apart how antibiotic tolerance and persistence arise. 2 They recently reported in Cell Host & Microbe that tolerance comes from mutations in genes related to nutrient production while persistent bacteria activate a specific DNA repair pathway to survive.
“Antibiotic resistance is, in some ways, the end stage of a problem,” said Peter Hill, now a research fellow at Imperial College London and co-first author of the recent work. “If you can stop those…initial processes from happening, you can stop the end process.”
Playing Dead to Survive
To find out which mutations were responsible for tolerance, Hill’s team infected macrophages with a strain of Salmonella that causes recurrent diarrheal disease, 3 induced tolerance by exposing them to antibiotics, and sequenced the genomes of the surviving bacteria. They found mutations that stopped the bacteria from making certain molecules essential for life. Bacteria that cannot make these compounds grow slowly, which causes them to survive in the face of antibiotics that target dividing cells. Because tolerant bacteria only grow in nutrient-rich environments, Hill saw that the tolerant Salmonella were sensitive to antibiotics once they moved to favorable conditions.
Next, the team studied antibiotic persistence, which results from a phenotypic switch that temporarily slows or stops growth in a small portion of antibiotic-susceptible bacteria. Like the tolerant bugs, the persistent bacteria grew slowly, if at all, within macrophages treated with antibiotics. But the researchers found that persistent Salmonella came alive in the macrophages once they removed the antibiotic stress.
Because persistence arises from a phenotypic shift rather than a mutation, Hill and his colleagues performed RNA-sequencing on the persisters to see how antibiotic treatment affected gene expression in this dormant population. They observed an increase in a stress response pathway that bacteria induce in response to DNA double-strand breaks (DSBs)—a common side effect of genome replication within hostile macrophages.
“It's usually assumed that when you have DNA replication, bacteria will then go through cell division,” Hill said. “We found that DNA replication, or at least some form of DNA replication, is happening in these non-proliferating bacteria.”
Additionally, Hill’s team discovered that to survive in the face of drugs and macrophages, Salmonella persisters required a DNA repair mechanism to activate the stress response and repair the DSBs. After damage repair, the persisters reinitiated infection in new host cells, which mimics recurrent clinical infections.
From the Lab to the Clinic
Testing macrophages in a lab offers a lot of information, but Hill and his team wanted to know what happens during infections in the human body. They obtained patient samples of a similar Salmonella strain and sequenced the bacterial genomes. To their surprise, they did not identify any of the tolerance mutations that showed up in their cell culture experiments. Instead, the isolates’ growth in antibiotic-treated macrophages mimicked that of persistent bacteria; there was a subpopulation that was not as susceptible to antibiotics and had robust activation of the DNA repair response.
“The observation that clinical isolates seem to behave closer to the persisters in terms of the [DNA repair] response is very interesting,” Nathalie Balaban, a professor at the Hebrew University of Jerusalem who was not involved in this study, wrote in an email. “It would be also good to see whether [the clinical isolates] reinfect macrophages better.”
Understanding the role of DNA repair in driving persistence and tolerance will help researchers develop other treatment strategies for bacterial infections. Blocking the DNA repair necessary for Salmonella survival in combination with antibiotic treatment may stop infection relapse and slow the development of antibiotic resistance.
What is antibiotic resistance?
- Bacteria that are resistant to antibiotics are not susceptible to treatment and can grow unchecked despite treatment, which may lead to life-threatening, often incurable infections.
Can an infection come back after antibiotics?
- Some antibiotic-susceptible bacteria temporarily slow or stop growth in response to antibiotics, allowing them to avoid drug effects and bounce back after treatment. This process is called antibiotic persistence.
This article was originally published on March 7, 2022. It was updated on August 26, 2024 by Deanna MacNeil , PhD .
- P.W.S. Hill, S. Helaine, “ Antibiotic persisters and relapsing Salmonella enterica infections ,” in Persister Cells and Infectious Disease, K. Lewis, ed., Springer International Publishing, 2019, pp. 19-38.
- P.W.S. Hill et al., “ The vulnerable versatility of Salmonella antibiotic persisters during infection ,” Cell Host Microbe, 29:1757-73.e10, 2021.
- C.K. Okoro et al., “ High-resolution single nucleotide polymorphism analysis distinguishes recrudescence and reinfection in recurrent invasive nontyphoidal Salmonella typhimurium disease ,” Clin Infect Dis, 54:955-63, 2012.
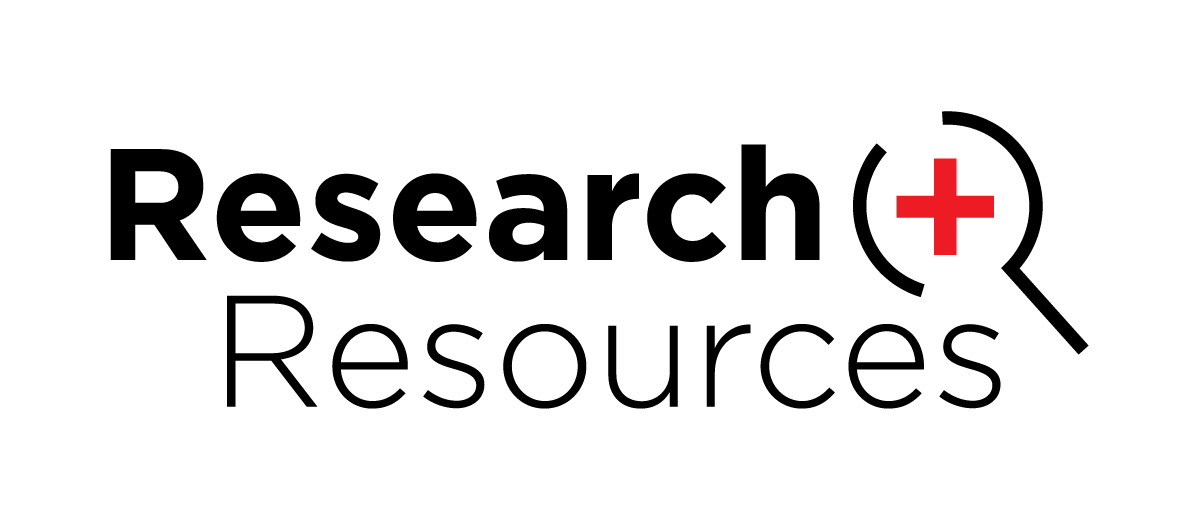
- Open access
- Published: 16 August 2024
Microbial assimilatory sulfate reduction-mediated H 2 S: an overlooked role in Crohn’s disease development
- Wanrong Luo 1 , 2 na1 ,
- Min Zhao 3 na1 ,
- Mohammed Dwidar 4 , 5 ,
- Yang Gao 2 ,
- Liyuan Xiang 1 ,
- Xueting Wu 1 ,
- Marnix H. Medema 6 ,
- Xiaozhi Li 1 ,
- Hendrik Schäfer 7 ,
- Minhu Chen 1 na2 ,
- Rui Feng 1 na2 &
- Yijun Zhu 2 , 8 na2
Microbiome volume 12 , Article number: 152 ( 2024 ) Cite this article
784 Accesses
6 Altmetric
Metrics details
H 2 S imbalances in the intestinal tract trigger Crohn's disease (CD), a chronic inflammatory gastrointestinal disorder characterized by microbiota dysbiosis and barrier dysfunction. However, a comprehensive understanding of H 2 S generation in the gut, and the contributions of both microbiota and host to systemic H 2 S levels in CD, remain to be elucidated. This investigation aimed to enhance comprehension regarding the sulfidogenic potential of both the human host and the gut microbiota.
Our analysis of a treatment-naive CD cohorts' fecal metagenomic and biopsy metatranscriptomic data revealed reduced expression of host endogenous H 2 S generation genes alongside increased abundance of microbial exogenous H 2 S production genes in correlation with CD. While prior studies focused on microbial H 2 S production via dissimilatory sulfite reductases, our metagenomic analysis suggests the assimilatory sulfate reduction (ASR) pathway is a more significant contributor in the human gut, given its high prevalence and abundance. Subsequently, we validated our hypothesis experimentally by generating ASR-deficient E. coli mutants ∆cysJ and ∆cysM through the deletion of sulfite reductase and L-cysteine synthase genes. This alteration significantly affected bacterial sulfidogenic capacity, colon epithelial cell viability, and colonic mucin sulfation, ultimately leading to colitis in murine model. Further study revealed that gut microbiota degrade sulfopolysaccharides and assimilate sulfate to produce H 2 S via the ASR pathway, highlighting the role of sulfopolysaccharides in colitis and cautioning against their use as food additives.
Conclusions
Our study significantly advances understanding of microbial sulfur metabolism in the human gut, elucidating the complex interplay between diet, gut microbiota, and host sulfur metabolism. We highlight the microbial ASR pathway as an overlooked endogenous H 2 S producer and a potential therapeutic target for managing CD.
Video Abstract
Introduction
Crohn's disease (CD) and ulcerative colitis (UC) are two main forms of Inflammatory Bowel Disease (IBD), characterized by symptoms including diarrhea, rectal bleeding, abdominal pain, fatigue, and weight loss, significantly impacting patients' lives. IBD incidence and prevalence are rising globally, particularly in newly industrialized regions [ 1 , 2 ]. The growing global burden of this disease underscores the need for preventive and therapeutic measures [ 2 ]. Although the precise etiology remains elusive, it is believed to result from dysregulated mucosal immune responses triggered by gut bacteria, especially in individuals with genetic predispositions [ 3 , 4 ].
Sulfur metabolism and sulfur-containing metabolites play a pivotal role in IBD [ 5 , 6 , 7 ]. Hydrogen sulfide (H 2 S) is the sulfur derivative that garners the most attention in the context of colonic health. In the gastrointestinal system, the H 2 S pathway supports epithelial, immune, and enteric nervous system health through various mechanisms, including posttranslational modification of protein cysteine residues, activation of K ATP channels, and serving as an inorganic fuel for colonocytes [ 8 , 9 , 10 ]. However, excessive exposure to H 2 S can be detrimental to the host, damaging the intestinal epithelium and leading to chronic inflammation, as well as disrupting the balance between cellular proliferation and apoptosis [ 11 ]. An association between elevated H 2 S levels and IBD has long been suspected [ 12 , 13 ]. Several studies suggest that pharmacological interventions targeting H 2 S may improve outcomes in IBD through mechanisms such as driving regulatory T cell differentiation, stabilizing hypoxia-inducible factor 1-alpha (HIF-1α), promoting biofilm formation, and reducing planktonic bacteria growth [ 14 , 15 , 16 ].
However, a comprehensive mechanistic model elucidating the relationship between H 2 S generation and IBD is still lacking. The production and release of H 2 S are regulated by both endogenous and exogenous factors, but the relative contributions of the host and gut microbiota to overall systemic H 2 S levels in humans remain uncertain. Endogenous H 2 S production primarily results from the enzymatic degradation of organic sulfur compounds, particularly cysteine. Key enzymes in this process include cystathionine beta-synthase (CBS), cystathionine gamma-lyase (CTH), 3-mercaptopyruvate sulfur transferase (MPST), and methanethiol oxidase (SELENBP1) [ 17 , 18 ]. On the other hand, our understanding of microbial-mediated H 2 S generation remains limited. Nevertheless, several studies have shown that bacteria generate H 2 S to mitigate oxidative stress from antibiotics [ 19 ], drive cryptic redox chemistry to shape gut metabolism [ 7 ], regulate intracellular cysteine levels [ 20 ], and influence bacterial virulence via proteome S-sulfhydration [ 21 ], highlighting the necessity to clarify the mechanisms of bacterial H 2 S generation.
Bacteria produce H 2 S through the utilization of both organic sulfur compounds like L-cysteine and taurine, as well as inorganic sulfur compounds such as sulfate and sulfite. The two primary pathways for sulfate metabolism are Assimilatory Sulfate Reduction (ASR) [ 22 , 23 ], involving the reduction of sulfate to H 2 S, which is subsequently incorporated into cysteine and methionine biosynthesis, and Dissimilatory Sulfate Reduction (DSR), a process found in sulfate-reducing bacteria where these microbes produce H 2 S from sulfate without integrating it into L-cysteine [ 24 ] (Fig. 1 ).
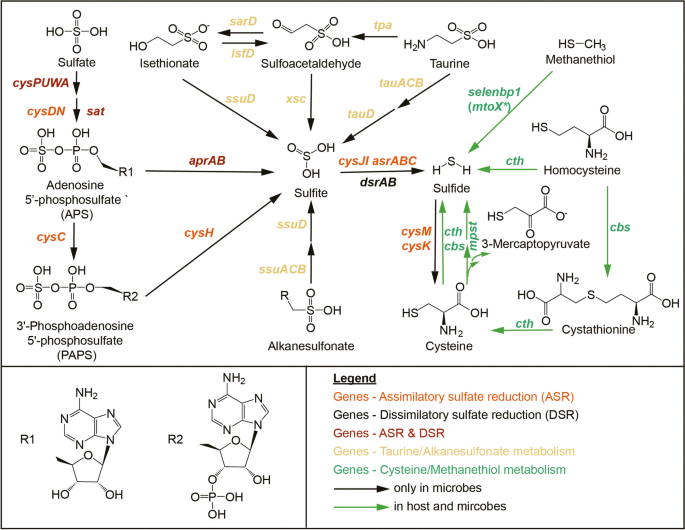
Exogenous microbial sulfur metabolism results in the production of genotoxic H 2 S via metabolism of inorganic sulfate and organic sulfur like cysteine, taurine, isethionate, methanethiol and alkanesulfonate (black and green). Endogenous H 2 S is produced via metabolism of sulfur containing amino acids like cysteine and homocysteine, as well as organic sulfur methanethiol (green). Gene names and KEGG ID are listed in Supplementary dataset 1. * Recent research has demonstrated that sulfane sulfur is the direct product of the bacterial methanethiol oxidase (MtoX) [ 25 ]
Previous investigations into microbial sulfidogenesis found the contributions of gut microbiota to systemic total H 2 S levels varied widely across subjects [ 26 ], and have mainly focused on the fermentation of organic sulfur compounds [ 5 , 27 ] and sulfate-reducing bacteria DSR [ 28 , 29 ]. Meanwhile, ASR, a common strategy employed by many microbes to fix sulfur and manipulate organosulfur compounds, has been routinely overlooked.
Here, we employed genomic and metagenomic tools to gain a deeper understanding of the colonic sulfidogenic capacity of both the host and gut microbiome in a newly-onset treatment-naïve CD cohort, and observed that CD exhibit reduced endogenous H 2 S production alongside increased gut microbial H 2 S generation, primarily via the ASR pathway. Mechanistically, we genetically manipulated E. coli ASR pathway to evaluate the impact on (i) E. coli 's sulfidogenic capacity, (ii) colon epithelial cell viability, and (iii) the development of colitis and maintenance of mucus integrity in a mouse model. Our data elucidate a previously unappreciated role of microbial ASR pathway in dietary sulfate metabolism, intestinal sulfur homeostasis and mucus integrity, emphasizing its pivotal role in CD pathogenesis.
CD is associated with up-regulation of gut microbial assimilatory sulfate reduction
We conducted a comprehensive investigation into the H 2 S production capabilities of the human gut microbiome, focusing on key genes responsible for sulfide generation from various sources, including organic compounds (such as dietary rich L-cysteine and taurine) and inorganic sulfate (Fig. 1 , Supplementary dataset 1). Our analysis was based on stool metagenomic samples from two independent IBD cohorts, FAH-SYSU (treatment naïve IBD cohort enrolled at the First Affiliated Hospital of Sun Yat-sen University) [ 30 ] and PRISM (Prospective Registry of IBD study at MGH) [ 3 ]. ShortBRED was employed to identify unique sequence markers of related family members and quantifying their relative abundance in metagenomic data with high specificity [ 31 ]. It is noteworthy that ShortBRED was not specifically developed for gene cluster identification and quantification. Nevertheless, our search within metagenomic datasets using individual genes revealed a moderate to high degree of consistency among genes from the same cluster (spearman r 0.59–0.94, p < 0.001, Supplementary dataset 2), confirming the accuracy of the predictions.
We found that genes associated with ASR, including sulfate adenylyltransferase ( cysDN ) and adenylylsulfate kinase ( cysC ), were highly prevalent in both cohorts. In the FAH-SYSU cohort, these genes were present in 100% of both CD and HC subjects, while in the PRISM cohort, their prevalence ranged from 82 to 100%. These genes were also abundant, with RPKM values of 98.0–646.7 in the FAH-SYSU cohort and 20.6–57.2 in the PRISM cohort for both CD and HC subjects (Fig. 2 , Supplementary dataset 3). The PRISM cohort exhibited lower abundance, possibly due to differences in sequencing procedures. In the ASR pathway, organisms use different strategies: 1) Adenosine-5’-phosphosulfate (APS) is phosphorylated into 3’-Phosphoadenosine-5’-phosphosulfate (PAPS) by CysC, which is further reduced into sulfite (SO 3 2− ) by PAPS reductase (CysH); 2) APS is directly reduced by an APS reductase (AprAB) to generate adenosine monophosphate (AMP) and SO 3 2− . Both scenarios generate SO 3 2− which could further be reduced by anaerobic sulfite reductase (AsrABC) [ 32 ] or sulfite reductase (CysJI) to form sulfide (S 2− ), which subsequently yield L-cysteine mediated by cysteine synthase A (CysK ) and cysteine synthase B (CysM) (Fig. 1 ). We found ASR downstream genes, including cysH , cysJI , cysM , cysK , and asrABC , were also more abundant in CD subjects ( p < 0.01), suggesting a significant role for ASR in H 2 S production from SO 4 2− in CD individuals (Fig. 2 A, Supplementary dataset 3).
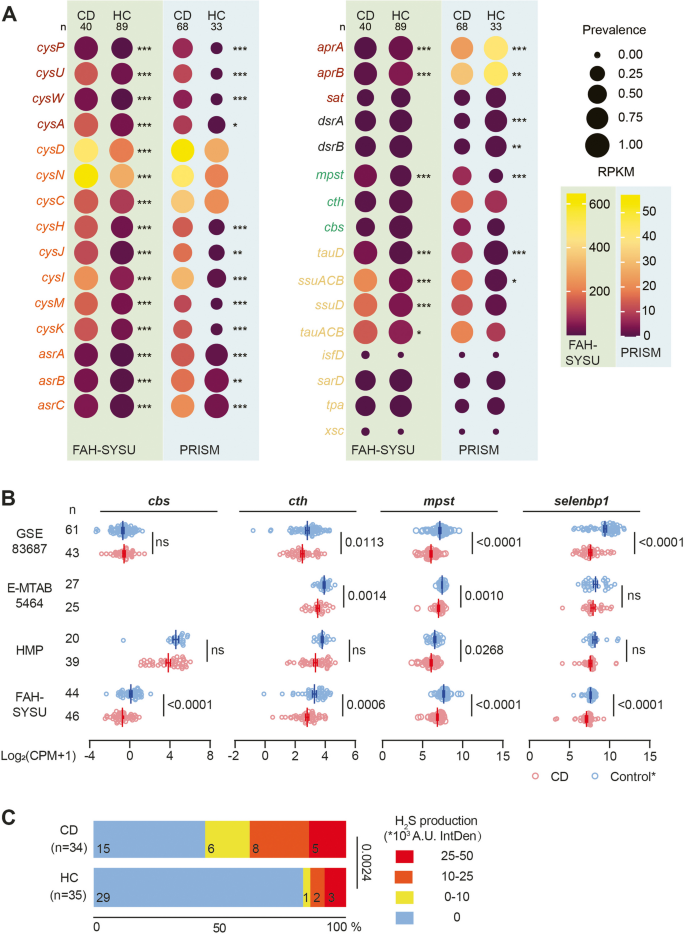
CD is associated with decreased endogenous sulfidogenic gene expression and increased gut microbial exogenous sulfidogenic gene expression. A Dot plots comparing selected genes related to microbial sulfide generation from CD versus healthy control subjects (HC) in FAH-SYSU (light green background) cohort and PRISM (light blue background) cohort. The size of each dot indicates the proportion of participants detected in each group of the indicated gene and the color of each dot indicates RPKM with that gene in each group. RPKM, reads per kilobase per million mapped reads. Genes related to ASR, DSR, and organic sulfur metabolism are color-coded according to the scheme in Fig. 1 . B Analysis of cbs , cth , mpst and selenbp1 gene expression in CD and non-IBD control subjects’ mucosa in different IBD cohorts. CBS, cystathionine beta-synthase; CTH, cystathionine gamma-lyase; MPST, 3-mercaptopyruvate sulfurtransferase; SELENBP1, methanethiol oxidase. * Various control groups were utilized in different cohorts. FAH-SYSU, non-disease control; HMP, symptomatic non-IBD controls; E-MTAB5464, non-disease control; GSE83687, normal non inflamed bowel away from the tumor from sporadic colon cancer patients. C CD patients exhibit increased assimilatory sulfate reduction activity in their fecal microbial community compared to healthy subjects. Significance was determined by nonparametric Mann–Whitney test. * p < 0.05, ** p < 0.01, *** p < 0.001
We observed that the prevalence and abundance of dsrAB genes (key genes for the DSR but not the ASR pathway) were notably lower compared to asr -associated genes in both cohorts. In the PRISM cohort, dsrAB genes were detected in approximately 30.9–32.4% of CD subjects, while this percentage increased to 67.6–76.5% in HC subjects. Moreover, their abundance increased from approximately 0.48 to 0.60–0.72 RPKM ( p < 0.01). However, no significant difference in dsrAB genes was observed in the FAH-SYSU cohort (Fig. 2 A). Additionally, aprAB , responsible for converting APS to SO 3 2− in both DSR and ASR, showed a marked reduction in CD subjects (Fig. 2 A). Furthermore, we investigated cysPUWA , which encodes a sulfate transporter common to both pathways. These transporter genes were more prevalent and abundant in CD subjects, indicating increased microbial sulfate transport in CD patients.
Organic sulfur metabolism has been reported to be enriched in individuals with IBD and colorectal cancer (CRC) [ 33 , 34 ]. Therefore, we investigated microbial genes associated with organic sulfur metabolism in our study. We found that the bacterial gene mpst , important for converting L-cysteine to H 2 S, exhibited more prevalent and significantly elevated levels in CD subjects compared to HC subjects in both cohorts ( p < 0.001, Fig. 2 A). Additionally, genes related to taurine and alkanesulfonate metabolism, including taurine transporter ( tauABC ), taurine dioxygenase ( tauD ), sulfonate transporter ( ssuACB ), and alkanesulfonate monooxygenase ( ssuD ), were more abundant in CD subjects in FAH-SYSU cohort ( p < 0.001, Fig. 2 A). Microbial methanethiol oxidase ( mtoX ), widely distributed in the biosphere [ 35 ], was not detected in human associated bacteria and therefore was excluded in the ShortBRED analysis. In the PRISM cohort, an increasing trend was observed in tauABC and ssuD among CD subjects, although statistical significance was not attained.
CD patients demonstrate impaired endogenous H 2 S production
Endogenous H 2 S production arises from the host's utilization of sulfur-containing amino acids (Fig. 1 ). To shed light on endogenous sulfidogenic activity, we evaluated the expression levels of the host cbs , cth , mpst and selenbp1 by examining intestinal biopsies obtained from newly diagnosed CD patients (n = 46) and non-disease controls (n = 44) from the FAH-SYSU cohort [ 36 ]. Our analysis revealed that all of these 4 genes exhibited significant decreases in inflamed mucosal biopsies from CD subjects (Fig. 2 B). A similar trend was observed in three independent IBD cohorts, including the Mount Sinai Hospital cohort (GSE83687) [ 37 ], a treatment-naive pediatric IBD cohort (E-MTAB-5464) [ 38 ] and the HMP IBD cohort [ 4 ] (Fig. 2 B), although statistical significance was not achieved in some cases. In the E-MTAB-5464 cohort, transcriptomic data were generated from purified intestinal epithelial cells. CBS raw counts in this cohort were generally less than 10, hence not analysed. This finding strongly suggests a substantial reduction in the endogenous sulfidogenic capacity of CD patients.
To uncover whether CD patients have impaired H 2 S catabolism capacity, we examined the expression levels of key enzymes responsible for host H 2 S catabolism in these cohort datasets, including thiosulfate sulfurtransferase (TST), thiosulfate sulfide:quinone oxidoreductase (SQOR), and persulfide dioxygenase (ETHE1) (Fig. S1 A) [ 13 , 39 ]. Our analysis revealed that tst was significantly downregulated, while sqor was upregulated in CD subjects in FAH-SYSU cohort. A similar trend was observed in the HPM IBD cohort, although tst did not reach statistical significance between CD and non-IBD groups in this cohort. The expression of ethe1 remained similar in both FAH-SYSU and HMP cohorts, irrespective of CD or control groups (Fig. S1 B). Therefore, further research is needed to explore the H 2 S catabolism capacity in CD patients.
Oxygen-insensitive ASR is functionally more active in fecal microbiota from CD patients
To further substantiate the contribution of the ASR pathway from gut microbiota to H 2 S generation in CD, we conducted an ex vivo fecal culture experiment using thiosulfate (S 2 O 3 2−2− ) as the sole sulfur source. DSR has been reported in sulfate-reducing bacteria which are strictly anaerobes, whereas ASR has been reported in facultative anaerobes and aerobes [ 40 ]. Therefore, we set up the fecal culture aerobically and measured H 2 S production in fecal samples from both healthy individuals and CD patients to test if oxygen-insensitive ASR activity was enhanced in CD’s gut microbiota. We detected H 2 S production in 19 out of 34 (55.9%) CD stool samples, with 13 showing notably high levels (> 10,000 intensity). In contrast, only 6 out of 35 (17.1%) samples from healthy controls exhibited H 2 S production, with 5 demonstrating high levels (Fig. 2 C). Thus, oxygen-insensitive ASR is more active in CD patients.
The bacterial ASR pathway is prevalent in the human microbiome
The asr gene cluster in E. coli MG1655 [ 22 ] and Salmonella enterica ST8493 [ 23 ], along with the dsr gene cluster in Desulfovibrio gigas DSM 1382 [ 24 ], that have been characterized in previous studies, are shown in Fig. 3 A. To comprehensively assess the distribution of asr - ( cysDN , cysC , cysH , cysJI , cysM, cysK , aprAB and asrABC ) and dsr -associated genes ( dsrAB , aprAB ) among human bacteria, we screened these genes against 1635 Human Microbiome Project (HMP) reference genomes. This extensive analysis revealed that asr -associated genes are more widespread than dsrAB (Fig. 3 B, Supplementary dataset 4). A significant number (88.1%, 1441 out of 1635 reference genomes) of the total reference genomes contain at least one asr -associated gene, distributed predominantly in Firmicutes, Actinobacteria, Proteobacteria, and Bacteroidetes, whilst dsrAB genes are only found in 0.43% (7 genomes) which are from Firmicutes and γ-Proteobacteria (Fig. 3 B, Supplementary dataset 4). The prevalence of cysDN (409 genomes) and cysC (295 genomes) in Bacteroidetes were higher than the other asr -associated genes. On the other hand, cysJI (193 strains) and cysM (275 strains) were more prevalent in Proteobacteria, including facultative aerobic species like E. coli , Proteus mirabilis , Klebsiella oxytoca (Fig. 3 B, Supplementary dataset 4). asrABC (117 genomes) was more commonly found in Firmicutes and Fusobacteria. These observations suggest a potential collaborative interplay among microorganisms in the execution of the ASR pathway.
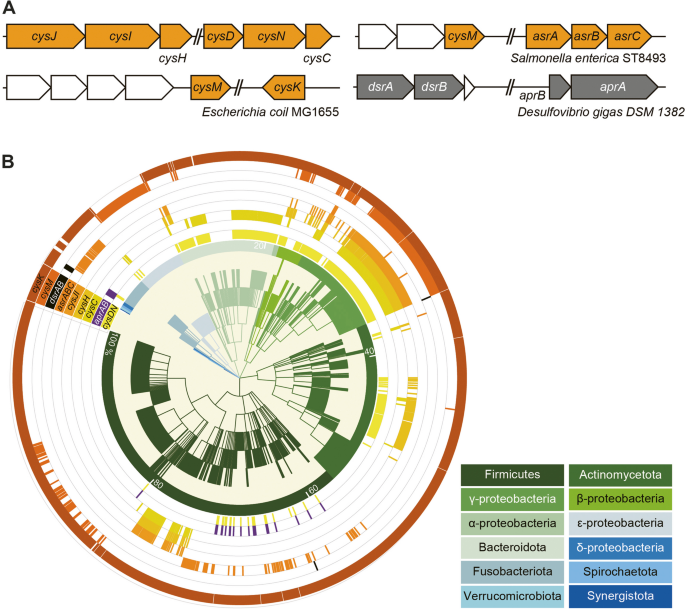
ASR pathway is widely distributed in human microbiota. A Arrangement of asr - and dsr - associated genes and gene clusters in bacteria. Orange ORFs: asr -associated genes; gray ORFs: dsr -associated genes. Other genes not shown are represented by white ORFs. B Phylogenetic distribution of genomes harboing asr - and dsr -associated genes in 1635 reference genomes of the Human Microbiome Project (HMP). Gene and genome names are listed in Supplementary dataset 4
Construction of E . coli mutants with impaired assimilatory sulfate reduction (ASR)
We focused on the cysJI -mediated ASR pathway in this study since metagenomic data indicated that it is more abundant than asrABC (Fig. 2 A). We used E. coli MG1655, a known bacterium with a complete ASR pathway, as the model organism (Fig. 4 A). Using homologous recombination, we deleted two crucial ASR pathway genes, cysJ and cysM . cysJ encodes sulfite reductase alpha subunit ( cysI encodes beta subunit), responsible for the reduction of SO 3 2−2− to S 2−2− while cysM encodes cysteine synthase B, which converts S 2− to L-cysteine. (Fig. 4 A). As expected, deleting cysJ hindered E. coli growth on SO 4 2− as the sole sulfur source (Fig. 4 B and Fig. S2A). E. coli carries a CysM homologue, CysK, which compensates for CysM in incorporating S 2− into L-cysteine (Fig. 4 A). Consequently, E. coli ∆cysM strains exhibited growth similar to the WT strain when SO 4 2− was the sole sulfur source. Alternatively, CysM can use thiosulfate (S 2 O 3 2− ) in place of S 2− to produce L-cysteine via S-sulfocysteine as the intermediate (Fig. 4 A). Therefore, E. coli WT and ∆cysJ mutant grew on S 2 O 3 2− as the sole sulfur source, however ∆cysM displayed diminished growth rate (Fig. 4 B and Fig. S2B).
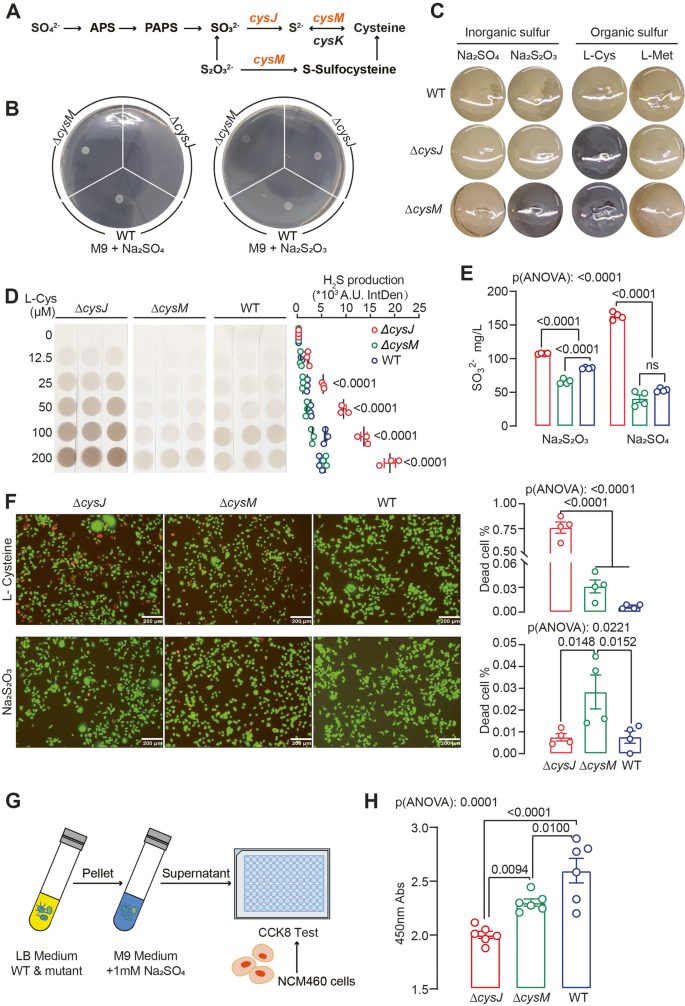
Deletion of cysJ and cysM alters E. coli H 2 S sulfidogenic capacities and modulate cell viability. A Scheme of the E. coli MG1655 sulfate assimilation reduction pathway. B Growth of E. coli WT and mutant strains on M9 medium with 1 mM sulfate (left) or sodium thiosulfate (right) as the sole sulfur source. C WT and mutant strains of E. coli were qualitatively tested for H 2 S in SIM media of different sulfur sources, as evidenced by the formation of black FeS. D Relatively quantitative test of H 2 S produced by E. coli WT and mutant strains in M9 medium with different concentrations of L-cysteine as a sole sulfur source under aerobic conditions. Significance was measured with two-way ANOVA analysis with Tukey's multiple comparisons. E Quantification of sulfite (SO 3 2− ) produced by E. coli WT and mutant strains in M9 medium using 1 mM Na 2 S 2 O 3 or Na 2 SO 4 as the sole sulfur source. F Representative images and quantification of cell death rates cell death rate of NCM460 cells co-cultured with E. coli WT and mutant strains, with either L-cysteine supplementation (upper panel) or sodium thiosulfate supplementation (lower panel), were analyzed using live/dead staining. Living cells are represented in green, while dead cells are shown in red. G - H A CCK-8 assay was performed using NCM460 cells treated with supernatant from M9 medium containing 1 mM Na 2 SO 4 that had been pre-inoculated with E. coli WT or mutant strains. Mean ± SEM is displayed from at least three independent experiments. Significance was measured with one- and two-way ANOVA analysis with Tukey's multiple comparisons
We assessed E. coli WT and mutant strains for their sulfidogenic capabilities using various inorganic and organic sulfur sources. In the modified Sulfur, Indole, Motility (SIM) medium, we observed that deleting cysJ increased H 2 S production from L-cysteine, while deleting cysM enhanced H 2 S generation from both L-cysteine and SO 3 2− (Fig. 4 C). E. coli WT also produced H 2 S from L-cysteine, as indicated by slight medium darkening (Fig. 4 C). We further cultured E. coli WT and mutant strains in M9 medium supplemented with varying concentrations of L-cysteine, and observed that all the strains exhibited a dose-dependent production of H 2 S, with the ∆cysJ mutant demonstrating greater efficiency in converting L-cysteine to sulfide than ∆cysM and WT (Fig. 4 D). Under anaerobic conditions, E. coli ∆cysM actively reduced SO 3 2− to produce H 2 S (Fig. S2C). While the ∆cysJ mutant consistently showed increased H 2 S production in the presence of L-cysteine under anaerobic conditions, both E. coli WT and mutant strains displayed decreased H 2 S production compared to aerobic conditions (Fig. S2D). Additionally, the ∆cysJ mutant accumulated SO 3 2− in M9 medium when SO 4 2− or S 2 O 3 2− was the sole sulfur source due to the loss of sulfite reductase activity (Fig. 4 E). These findings highlight the impact of ASR pathway alterations on both inorganic and organic sulfur metabolism. E. coli WT and ASR-deficient mutants displayed distinct morphological characteristics and proteomic profiles (Fig. S3A, B, Supplementary dataset 5), suggesting that the alteration of the ASR pathway has a profound effect on bacterial physiology.
Bacterial assimilatory sulfate reduction modulates epithelial cell viability
We proceeded to investigate the impact of modifications in the bacterial ASR pathway on the growth of colonic epithelial cells in an in vitro setting. We co-cultured E. coli WT and mutant strains with normal human colonic mucosal epithelial cell line NCM460 with either L-cysteine or S 2 O 3 2− as the sole sulfur source. Cell viability assay revealed that in the presence of L-cysteine, the ∆cysJ mutant led to significantly decreased cell viability, concurrent with increased H 2 S production (Fig. 4 D, F). When S 2 O 3 2− served as the exclusive sulfur source, the ∆cysM mutant induced more pronounced cell death, accompanied by higher H 2 S generation (Fig. 4 C, F).
As ∆cysJ mutant accumulates more SO 3 2− in the medium when SO 4 2− is the sole sulfur source (Fig. 4 E), this accumulation of SO 3 2− may potentially leads to cell toxicity [ 41 ]. To investigate it further, we collected the supernatants from cultures of E. coli WT and mutant strains grown in M9 medium supplemented with Na 2 SO 4 and used them to treat NCM460 cells (Fig. 4 G). As indicated by the cell proliferation assay, the ∆cysJ mutant exhibited the most pronounced inhibition of cell proliferation in agreement with the high levels of SO 3 2− (Fig. 4 H). Thus, the data suggest that bacterial ASR modulates epithelial cell viability through SO 4 2− metabolites.
The gut microbiota is the primary contributor to serum H2S levels in the DSS-induced mouse colitis model
Colitis, a key component of IBD, is frequently studied using murine models. One widely employed method to induce colitis in these models is the administration of dextran sodium sulfate (DSS) via drinking water. Our initial objective was to determine if H 2 S production is linked to the DSS-induced colitis model. We found a significant increase in serum H 2 S levels in mice received DSS compared to vehicle controls (Fig. 5 A), suggesting that serum H 2 S is associated with DSS-induced colitis.
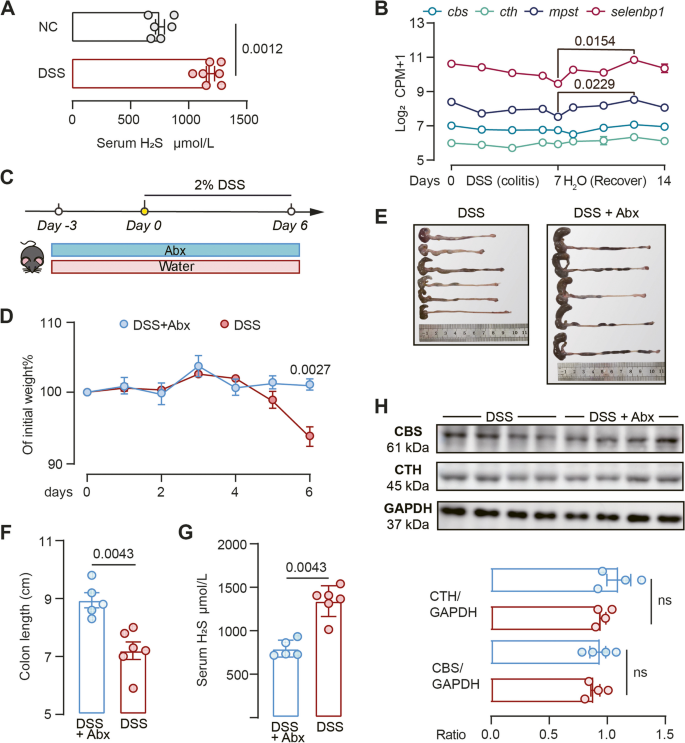
DSS-induced colitis is associated with increased exogenous H 2 S generation. A Serum H 2 S level of mice in normal control (NC) and DSS-treated group. Each dot represents an individual mouse. NC, mice were on water (n = 6–7). B The expression levels of cbs , cth , mpst , and selenbp1 in murine colonic tissues were assessed during the administration of DSS and the subsequent recovery period (GSE131032, n = 2 − 3). P-value was determined by nonparametric one-way ANOVA analysis with Dunn’s multiple comparisons. See Fig. 2 for gene full names. C Schematic diagram showing the experimental design, timeline of mouse models and sampling strategy. D Relative body weight of mice receiving DSS with antibiotics (DSS + Abx) and without (DSS) as shown in Fig B. n = 5–6. Significance was measured with two-way ANOVA with Tukey's multiple comparisons. E–G Colonic morphologies ( E ), colon length ( F ) and serum H 2 S level ( G ) of mice under different treatments. H Cystathionine beta-synthase (CBS) and cystathionine γ-lyase (CTH) protein levels were analysed by western blotting in mouse colon epithelial tissues. n = 4. Nonparametric Mann–Whitney test was used for non-pairwise comparisons. Each dot represents an individual mouse
Although a previous study suggested that germ-free mice exhibit reduced plasma H 2 S levels [ 42 ], the specific contribution of the gut microbiota to systemic H 2 S levels in the context of DSS-induced colitis remained unknown. To illuminate the link between elevated serum H 2 S and gut microbes, we performed two studies. First, we utilized a publicly available colonic tissue transcriptomic dataset from mice undergoing DSS-induced colitis, followed by a tissue regeneration phase (GSE131032) [ 43 ]. During the colitis and recovery stages, the expression of cbs and cth genes remained stable, while mpst and selenbp1 expression displayed a decreasing trend during colitis, followed by a slight elevation during the recovery stage (Fig. 5 B). This suggested that endogenous H 2 S production remained consistent or even decreased during DSS-induced colitis, hence the rise in serum H 2 S observed is probably from gut microbiota. Second, we administered broad spectrum antibiotics (Abx) to mice in the DSS-induced model (Fig. 5 C), and observed a significant reduction in serum H 2 S levels and alleviated DSS-induced colitis, as evidenced by weight and colon length measurements (Fig. 5 D-G). Given mRNA levels of cbs and cth remained stable throughout the DSS-induced colitis and recovery stages (Fig. 5 B), we further examined protein levels of CBS and CTH in Abx-challenge mice experiment, and observed no significant difference between the two groups (Fig. 5 H). Collectively, these findings provide compelling evidence that the gut microbiota plays a central role in the elevation of systemic H 2 S levels in the DSS-induced colitis model. Therefore, we utilized this model to investigate the causal relationship between microbial ASR pathway and colitis in vivo.
The gut bacterial ASR pathway contributes to sulfide generation derived from dietary sulfate
Diet plays a pivotal role in shaping the composition and metabolic activity of the gut microbiota. While prior research mainly concentrated on organic sulfur compounds from dietary proteins, the role of inorganic sulfur (SO 4 2− ) remains understudied [ 5 , 27 ]. Carrageenan, a common sulfated polysaccharide food additive, is linked to UC relapse risk and can induce intestinal inflammation in animal model [ 44 , 45 ]. We hypothesized that gut microbiota-mediated carrageenan degradation and subsequent H 2 S production might contribute to its pro-colitis effects.
To test this hypothesis, we initially cultured E. coli WT and mutant strains in M9 medium supplemented with λ-carrageenan as the sole sulfur source, owing to its high sulfur content (32–39%, Fig. 6 A). Surprisingly, both WT and mutant strains demonstrated H 2 S production (Fig. 6 A), which contrasted with previous findings that ∆cysJ mutant couldn’t grow on inorganic SO 4 2− . Given that carrageenan is a biopolymer derived from red algae, it likely contains trace amounts of organic sulfur compounds that can be utilized by ∆cysJ mutant. As a result, we transitioned to DSS, a synthetic sulfated polysaccharide with approximately 18–20% sulfur content (Fig. 6 B), which as mentioned earlier, is a commonly used as inducer in murine colitis models [ 46 ].
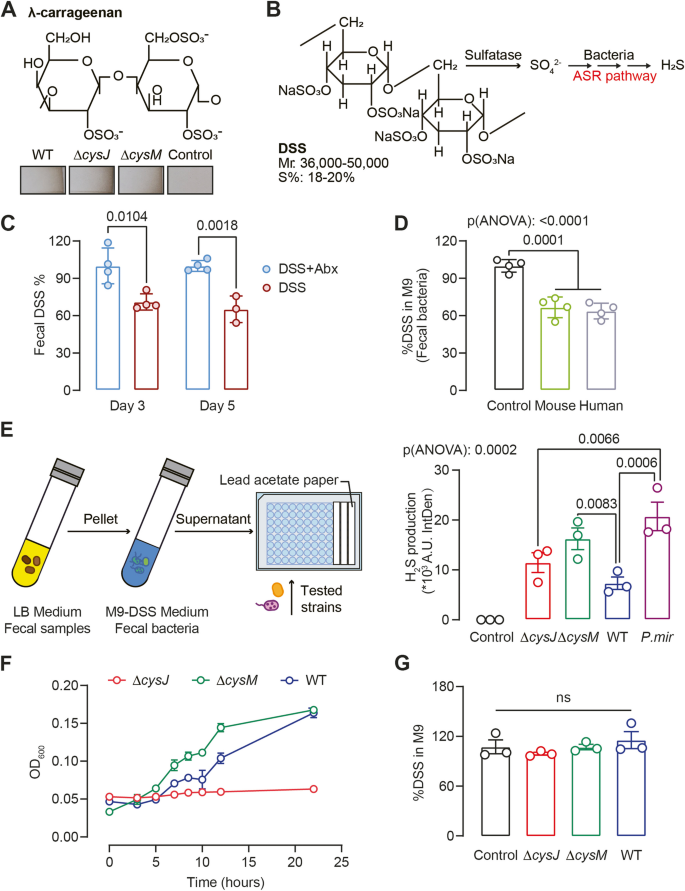
Gut bacterial ASR pathway contributes to H 2 S generation derived from dietary sulfated polysaccharide. A E. coli WT and mutant strains formed H 2 S from λ-carrageenan. Molecular formula of λ-Carrageenan (above). Representatives of H 2 S detection using lead acetate strip after growing E. coli WT and mutant strains in M9 medium supplemented with 1% λ-Carrageenan for 16 h aerobically (below). B Schematic outlining H 2 S production from DSS via bacteria. C DSS residue rate in mouse feces compared with abx-treated group in day3 and day5. Mice treatment was described in Fig. 5 C. Unpaired t-test was used for non-pairwise comparisons. D DSS degradation by fecal flora in mice and humans was tested in M9 supplemented with 1% DSS as the sole sulfur source. Medium without bacteria inoculation was used as controls. E Flow chart of H 2 S production from DSS test (left). H 2 S production analysis by E. coli and P. mirabilis strains was examined using lead acetate strip (right). F Growth of E. coli WT and mutant strains on M9 supplemented with DSS as the sole sulfur source. G DSS degradation by E. coli WT and mutant strains was tested in M9 supplemented with 1% DSS as the sole sulfur source. Medium without bacteria inoculation was used as controls. Values are Mean ± SEM from at least three independent experiments. P-value was determined by ordinary one-way ANOVA analysis with Tukey's multiple comparisons
We first tested whether the gut microbiota was involved in DSS degradation. Abx-treated mice exhibited significantly higher fecal DSS levels compared to vehicle control mice, suggesting active DSS degradation by gut microbiota in vivo (Fig. 5 C, 6 C). Ex vivo experiments with mouse and human stool samples showed about 35% of the DSS was consumed after overnight incubation (Fig. 6 D), confirmed microbiota-mediated DSS degradation.
We postulated that DSS degradation releases SO 4 [2−], which are subsequently assimilated by bacteria employing the ASR pathway. To test this hypothesis, we initiated an experiment involving 1% DSS incubation with human/mouse fecal cultures for 16 h, followed by supernatant collection, and subsequent inoculation with E. coli WT and mutant strains (Fig. 6 E). As anticipated, E. coli WT and mutant strains formed H 2 S in the presence of pre-incubated DSS. E. coli ∆cysM mutant produced higher levels of H 2 S than WT and the ∆cysJ mutant, although statistic significance was not attained (Fig. 6 E). P. mirabilis , which carries the asr -gene cluster, generated H 2 S as well (Fig. 6 E, Supplementary dataset 4). Direct culture of E. coli ∆cysJ mutant in M9 medium with DSS as the sole sulfur source did not yield growth (Fig. 6 F). Although E. coli WT and ∆cysM mutant grew on DSS as the sole sulfur source, they showed low utilization and negligible DSS degradation, emphasizing metabolic cross-feeding among bacterial species for efficient sulfated polysaccharide metabolism (Fig. 6 F-G).
The ASR pathway modulates DSS-induced colitis in vivo
SPF mice received Abx-cocktail were subsequently inoculated with E. coli WT, ∆cysJ and ∆cysM , then subjected to DSS administration (Fig. 7 A). Mice colonized with the E. coli ∆cysJ mutant exhibited elevated serum H 2 S and fecal SO 3 2− levels associated with more severe disease phenotype evidenced by a greater body weight loss, a worsening of disease activity, and more severe intestinal inflammation characterized by increased mucosal erosion, crypt destruction and inflammatory cell infiltration in the colon (Fig. 7 B-H). The heightened serum H 2 S levels may be ascribed to the degradation of organic sulfur compounds within the gastrointestinal tract, such as L-cysteine, catalyzed by E. coli ∆cysJ . We found a significant reduction in plasma levels of ursodeoxycholic acid (UDCA), α- and ω-muricholic acid (MCA), and an increase in cholic acid-7-sulfate (CA-7S) in mice colonized with the ∆cysJ strain (Fig. S4A), indicating that alteration of E. coli ASR pathway strongly influenced the bile acid profile in mice.
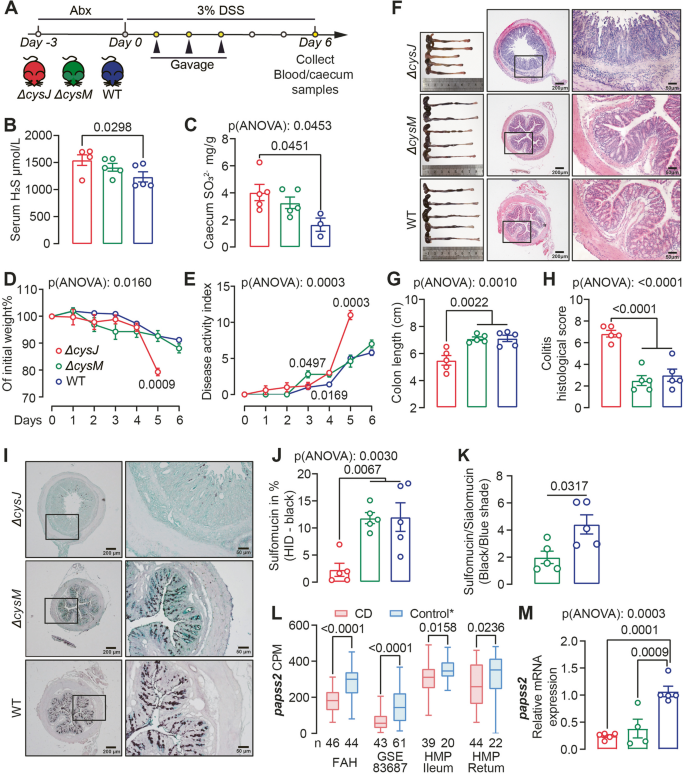
E. coli ASR pathway modulates intestinal sulfation and DSS-induced colitis in vivo . A Schematic diagram showing the experimental design, timeline of mouse models and sampling strategy. B , C Serum H 2 S and caecum content SO 3 2− levels in mice colonized with E. coli WT, ∆cysJ , and ∆cysM mutant strains. n = 3–5. Two cecum samples from the WT group were contaminated and excluded from the analysis. D Body weight was tracked after DSS administration. n = 5. E Disease activity over the duration of the experiment. F Colonic morphologies and representative H&E-Stained mouse colon sections at the termination of the experiment on day 6. G , H Colon length ( G ) and histological assessment of disease severity ( H ). J Representative micrographs of High-Iron Diamine-Alcian Blue (HID-AB) staining in mouse colon sections. Sulfomucin is stained black/brown and sialomucin is stained blue. K-L Sulfomucin ( K ) and Sulfomucin/Sialomucin ( L ) area ratio among ∆cysJ , ∆cysM and WT groups. M The box-whiskers plot showed abundance of papss2 from CD and control subjects from FAH-SYSU, GSE-83687 and HMP cohorts. CPM, copy per million. The highest and lowest values are denoted by the upper and lower extremities of the vertical line, respectively, while the median is represented by the central horizontal line. * Refer to Fig. 2 B for details regarding the control groups in each cohort. N Relative mRNA levels of papss2 in the colonic tissue of the mice shown in Fig. 7 A. Significance was measured with ordinary one- or two- way ANOVA analysis with Tukey's multiple comparisons. Nonparametric Mann–Whitney test was used for non-pairwise comparisons. Data shown as mean ± SEM. Each point represents an individual mouse
While ∆cysM and WT-colonized mice exhibited similar disease severity, serum H 2 S and fecal SO 3 2− levels, a significant difference in colonic mucin composition was observed. The colonic mucus layer, essential for maintaining homeostasis between resident microbiota and underlying immune cells, is primarily composed of acidomucins, broadly categorized as sialomucins or sulfomucins depending on the presence of sialic acid or sulfate groups [ 47 ]. Colonic tissues from ∆cysM -colonized mice showed a reduced sulfomucin:sialomucin ratio in (F i g. 6 I-K), indicating compromised host sulfation than WT mice. Intestinal sulfation, crucial for colitis protection, is dependent on the host PAPS synthase 2 (PAPSS2), which is central in generating PAPS, the universal sulfonate donor for sulfation [ 41 ]. Our analysis of transcriptomic data from the FAH-SYSU, HMP and GSE83687 cohorts indicated a significant decrease in colonic papss2 gene expression in actively inflamed CD patients compared to non-disease and non-IBD controls (Fig. 7 L). Mice deficient in papss2 have been previously demonstrated to manifest reduced intestinal sulfomucin content, rendering them susceptible to DSS-induced colitis [ 41 ]. Therefore, we reasoned that reduced host sulfate in ∆cysM -colonized mice were due to the downregulation of papss2 . Indeed, we observed decreased colonic mRNA expression of papss2 in both ∆cysJ and ∆cysM groups compared to the WT group, as confirmed by real-time PCR analysis (Fig. 7 M).
The burden of IBD, which encompasses conditions like CD and UC, is substantial and often leads to hospitalizations and surgical interventions [ 1 ]. Current treatments primarily target host inflammatory pathways using non-specific immunosuppressive agents, which can pose significant risks and may not always be effective, necessitating the exploration of alternative approaches [ 48 ]. Mounting evidence suggests that an imbalance in H 2 S production, either insufficient or excessive, can act as an environmental trigger for CD [ 29 , 49 ]. Studies have shown that the administration of H 2 S donors can suppress the expression of proinflammatory cytokines and ameliorate colitis in murine models [ 12 , 50 ]. This raises the possibility that modulating H 2 S concentrations in the gut lumen could be an exciting therapeutic strategy for treating CD [ 51 ]. However, research into this potential link between H 2 S and CD has been hindered by a limited understanding of sulfur metabolism within the human gut.
To address this knowledge gap, we conducted a comprehensive investigation into the functional capacity of both the gut microbiota and host in H 2 S production. Our findings suggest that microbial sulfur metabolism within the human colon is more complex and widespread than previously recognized. We analyzed metagenomic data from independent IBD cohorts and found that CD is associated with an increase in microbial generation through ASR pathways, as evidenced by the increased abundance and prevalence of asr -associated genes. Ex vivo fecal culture confirmed ASR-mediated H 2 S generation is more functionally active in stool samples from CD patients. This aligns with the “oxygen hypothesis”, which suggests that chronic inflammation of the intestinal walls leads to an increased release of hemoglobin carrying oxygen and reactive oxygen species into the intestinal lumen [ 52 ]. This process creates a microenvironment that favors facultative anaerobes. Using E. coli as the model organism, we generated ∆cysJ and ∆cysM mutants deficient in the ASR pathway. We conducted in vitro and in vivo studies to validate that the bacterial ASR pathway modulates cell viability, host sulfate homeostasis, and colitis pathogenesis. Our investigation has brought into focus the pivotal role played by ASR pathway in reshaping the utilization of L-cysteine and generation of H 2 S. The deletion of cysJ gene in E. coli amplifies H 2 S production from L-cysteine. The heightened metabolism of L-cysteine by gut microbes and increased abundance of cysM has recently been associated with CRC [ 34 ]. We noticed that asrABC is enriched in CD subjects, warranting further investigation into its potential association with CD.
In contrast to previous research, our findings suggest that the DSR pathway is unlikely to be the primary contributor to the elevated fecal microbial sulfidogenic capacity in CD. Prior research on exogenous H 2 S generation primarily centered on DSR, based on the culturing and sequencing of Desulfovibrio genus, sulfate-reducing bacteria frequently found in the human and animal gut [ 28 , 53 ]. However, Anantharaman et al. [ 54 ] revealed that dsrAB -mediated dissimilatory sulfur metabolism is predicted in a much broader diversity of bacterial and archaeal groups than previously recognized, primarily due to horizontal gene transfer, such as Bilophila wadsworthia , an opportunistic pathogen inhabiting the gut. Consequently, it is more reasonable to predict DSR-mediated H 2 S generation based on dsr -gene cluster quantification, rather than relying solely on Desulfovibrio quantification. The gut microbiota not only generates H 2 S but also has the capability to oxidize it using sulfide:quinone oxidoreductase and persulfide dioxygenase [ 55 , 56 ]. Further studies are warranted to gain a comprehensive understanding of gut microbiota sulfur metabolism.
Analysis of intestinal biopsy transcriptomic data from multiple IBD cohorts has unveiled a compromised endogenous sulfidogenic capacity in CD patients. This is evident from the downregulation of key genes, specifically cbs , cth , mpst and selenbp1 . Severe CD manifestations in a child with cbs deficiency has been reported [ 57 ]. Reduced expression of cbs mpst and selenbp1 has been linked to the exacerbation of inflammation-induced intestinal barrier injury in UC and CD [ 58 , 59 , 60 ],. Animal studies have provided additional evidence highlighting the critical role of endogenous H 2 S generation in colitis. MPST −/− and MPST ± mice exhibit exacerbated DSS-induced colitis [ 59 ]. Inhibition of endogenous H 2 S synthesis through the use of CBS and CTH inhibitors, such as β-cyanoalanine, propargylglycine, and O-carboxymethyl-hydroxylamine hemihydrochloride, has been demonstrated to worsen colitis in mouse model [ 12 ]. A correlation has been identified in immune deficiency and impaired H 2 S synthesis. Numerous studies have demonstrated that IL-10 plays an essential role in maintaining mucosal immunological tolerance in patients with IBD [ 61 ]. Flannigan et al.'s study with IL-10-/- mice, which spontaneously develop colitis, revealed a significant impairment in colonic H 2 S synthesis. This impairment was reversed by the administration of recombinant IL-10, confirmed an interplay between IL-10 and H 2 S synthesis [ 62 ].
Considerable efforts are underway to investigate the potential contributions of dietary factors to the pathophysiology of IBD [ 63 , 64 ]. The interplay of genetic, environmental, microbial, and immunological factors makes diet a crucial aspect of IBD etiology [ 65 ]. Dietary sulfur intake, primarily from inorganic sulfate and sulfur-containing amino acids (SAAs) such as methionine, cysteine, and taurine, plays a significant role [ 5 , 27 ]. However, estimations of dietary sulfur content often fail to account for sulfur-containing food modifiers or additives, such as carrageenan and sulfiting agents ( e.g. , potassium bisulfate, sodium bisulfate) [ 66 ]. Daily intake of inorganic sulfate is estimated to range from 1.5 to 16.0 mmol [ 66 ]. Interestingly, fecal sulfate (and sulfide) excretion is minimal compared to dietary intake, suggesting that sulfate is actively removed from the fecal stream during passage through the gut by both host and gut microbiota [ 67 ]. Carrageenan, a sulfated polysaccharide, contains approximately 15% to 40% sulfur, depending on the specific type of carrageenan ( e.g., kappa, iota, lambda) and the seaweed species used for extraction [ 68 ]. Carrageenan is widely used as a food additive in the Western diet, and its consumption has substantially increased over the past 50 years, paralleling the rising prevalence of IBD [ 69 ]. Successful dietary interventions that induced CD remission have excluded processed foods containing carrageenan, further supporting the notion that carrageenan may trigger or exacerbate inflammation in IBD [ 70 ]. In animal models, carrageenan administration consistently induces intestinal ulcerations resembling human IBD histopathologically [ 45 ]. We reasoned that SO 4 2− released during carrageenan degradation are utilized by gut microbes, contributing to IBD pathogenesis. To investigate, we used synthetic sulfated polysaccharide DSS due to carrageenan's organic sulfur contaminants. The DSS-induced colitis model is known for consistently mimicking epithelial damage seen in IBD, underscoring the complex interplay between dietary elements, gut microbiota, and disease pathogenesis [ 46 ]. DSS mouse colitis model is known for its variability even among genetically identical mice and across different mouse facilities. A recent study found that gut microbiota plays a significant role in driving this variability within the model [ 71 ].
Through in vivo and ex vivo studies, we've demonstrated that the gut microbiota can degrade DSS, releasing SO 4 2− that fuel bacterial ASR pathways. This alteration in microbial sulfur metabolism ultimately modulates disease severity. Our study highlights the crucial role of gut microbial ASR metabolism in dietary sulfate metabolism and susceptibility to colitis. It's important to acknowledge the significant daily intake of inorganic sulfate and the potential exacerbation of microbial H 2 S production by carrageenan used as food additives in processed foods, which can lead to mucosal damage. Furthermore, it is worth noting that CD pathogenesis is unlikely to be solely attributed to the presence and activities of single species. Genes associated with the ASR pathway exhibited varying distribution patterns among human bacteria. Additionally, in DSS degradation, mouse and human fecal microbial communities are more efficient than E. coli monocultures, suggesting cross-feeding among different bacterial species for the efficient metabolism of sulfated polysaccharides.
In summary, our study reveals the extensive diversity of microbial sulfur metabolism pathways. These findings highlight the association between CD and reduced endogenous H 2 S production alongside increased gut microbial H 2 S generation, primarily via the ASR pathway. Microbial ASR-mediated dietary sulfate metabolism emerges as a crucial factor in colitis. Thus, it is essential to maintain the homeostasis of microbial assimilatory sulfate reduction. Further research is needed to elucidate the regulation of the cysteine regulon and its impact on CD. Our research sheds light on the complex interaction between diet, the gut microbiota, and inorganic sulfate metabolism, highlighting their potential as promising therapeutic targets for managing CD.
Materials and Methods
Human subjects.
All study protocols abided by the Declaration of Helsinki principles and were approved by Ethical Committees of the First Affiliated Hospital of Sun Yat-sen University. Intestinal biopsies and stool specimens were collected as part of the FAH-SYSU cohort study (2016[113]). Subject stool samples were collected at the FAH, SYSU gastroenterology clinic and stored at -80 °C immediately. For culturing assays, fecal samples were collected and diluted to make a 10% (w/v) fecal slurry by resuspension of the feces in 10% (w/v) glycerol solution, and aliquots were stored in cryogenic vials at -80 °C until use. The exclusion criteria applied to all groups were as follows: recent (< 3 months prior) use of any antibiotic therapy, current extreme diet ( e.g. , parenteral nutrition or macrobiotic diet), known history of malignancy, current consumption of probiotics, any gastrointestinal tract surgery leaving permanent residua ( e.g. , gastrectomy, bariatric surgery, colectomy), or significant liver, renal, or peptic ulcer disease.
Analyses of asr - and dsr -associated genes in Human Microbiome project (HMP) references genomes
HMP references genomes (1635 genomes as of June 30, 2023) were selected and analyzed through the IMG program on the Joint Genome Institute website ( https://img.jgi.doe.gov/) [ 72 ]. The functions (Supplementary dataset 1) were used to carry out a “Function Profile” against all selected reference genomes to identify those carrying asr - and dsr -associated genes. Hits were manually inspected. Genomes carrying sulfidogenic gene(s) were selected to generate a phylogenetic tree using phyloT ( https://phylot.biobyte.de/ ) based on NCBI taxonomy and visualized using iTOL [ 73 ]. Genome and gene IMG ID are available in Supplementary dataset 4.
Metagenomic data analysis
We used ShortBRED [ 31 ] to accurately profile the abundance of genes involved in the H 2 S generation in metagenomes sourced from the FAH-SYSU (BioProject: PRJNA793776) [ 74 ] and PRISM (BioProject: PRJNA400072) [ 3 ] datasets. We initially compiled a set of identified bacterial sulfidogenic genes as our query sequences (Supplementary dataset 6). Subsequently, ShortBRED-Identify was employed to generate markers for these key bacterial sulfidogenic gene sequences using UniRef90 (May, 2023) as a reference list with an 85% cluster ID threshold. These markers were applied in ShortBRED-Quantify to assess gene abundance in paired metagenomes, which had previously undergone quality control via the KneadData workflow ( http://huttenhower.sph.harvard.edu/kneaddata ). The output from ShortBRED-Quantify was expressed as reads per million reads per kilobase million (RPKM).
Cultivation of wild type bacteria and mutants
Escherichia coli MG1655 wild type, mutants (Δ cysJ and Δ cysM ) and Proteus mirabilis ATCC 29906 were generally cultivated in Luria broth (LB) containing tryptone (10 g· L −1 ), yeast extract (5 g· L −1 ) and NaCl (10 g· L −1 ). To characterize the growth of E. coli wild type and mutant strains, they were cultivated in 5 mL LB overnight at 37 °C in a shaking incubator (250 rpm) and the pellet was collected by centrifugation at 3,000 × g for 10 min. Cell pellets were then washed and re-suspended in fresh M9 media (inoculum size 1:20, v/v ). The defined M9 medium contained NaCl (0.5 g·L −1 ), KH 2 PO 4 (3 g·L −1 ), Na 2 HPO 4 ·12H 2 O (6 g·L −1 ), NH 4 Cl (1 g·L −1 ), MgCl 2 (95 mg·L −1 ), CaCl 2 (11.1 mg·L −1 ) and glucose (0.1%, w/v). 1 mM Na 2 SO 4 , Na 2 S 2 O 3 , L-cysteine or DSS was used as sole sulfur source. 200 µL samples were collected from each tube at the indicated time points, and their optical density at 600 nm was measured in flat-bottom 96-well plates (200 µL per well). Sulfite in the supernatant was quantified as described in Sulfite Quantification section.
Allele-exchange mutagenesis of ΔcysJ and ΔcysM in E. coli MG1655
DNA fragments (~ 1 kb) corresponding to the upstream and downstream regions of the target gene were amplified and a subsequent overlap PCR was used to fuse the two fragments which were then ligated into suicide plasmid harboring kanamycin resistance cassette, oriT (mob), sacB counter selection marker and R6K origin of replication using the In-Fusion HD Cloning kit (Clontech). The ligated suicidal plasmid (pKmobSac) was transformed into the donor strain, E. coli S17 λpir. In parallel, E. coli MG1655 was transformed with a temperature-sensitive ampicillin-resistance plasmid carrying oriR101 origin of replication (p101-Amp). The suicidal plasmid (pKmobSac) was then transformed into E. coli MG1655 through conjugation and the resulted conjugants were screened at room temperature on LB agar plates containing ampicillin at 100 ng/µL (to select against E. coli S17 donor cells) and kanamycin at 50 ng/µL. One single-crossover integrant was then selected and re-streaked on LSW-Sucrose agar plate (tryptone 10 g/L, yeast extract 5 g/L, glycerol 5 mL/L, NaCl 0.4 g/L, sucrose 100 g/L and agar 20 g/L) [ 75 ] supplemented with ampicillin at 100 ng/µL to select for the correct double cross-over mutants. One mutant was then selected, re-streaked, and confirmed for the loss of the conjugated plasmid through Sanger sequencing and its ability to grow in presence of ampicillin but not kanamycin. The knockout E. coli MG1655 mutants were then cured from p101-amp plasmid through growing at 37 °C.
H 2 S quantification
Plasma H 2 S levels were quantified using a modified methylene blue method. In brief, ZnAC was added to 100 μL of plasma samples to precipitate H 2 S, HS − , S 2− , and plasma proteins. Subsequently, the ZnS pellet was re-dissolved by adding 130 μL of 2% N, N-dimethyl-p-phenylenediamine and 130 μL of 20% trichloroacetic acid. Methylene blue formation was initiated by addition of FeCl 3 ·6H 2 O and quantified at 665 nm using a spectrophotometer. Microbial culture H 2 S levels were quantified by Modified S ulfur, I ndole, M otility (SIM)-medium and Lead Acetate Test Strip. See online supplemental material for further details.
Dextran sulfate quantification
To measure DSS concentration in mouse fecal pellets, Sample aliquots (50 µL) were injected onto a size exclusion column (SEC-150, 3 μM, 7.8 × 300 mm, Welch, Cat # 00237–21052) and eluted at a flow rate of 1.5 mL/min. The mobile phase consisted of 25 mM KH 2 PO 4 , 25 mM K 2 HPO 4 ·3H 2 O, 50 mM KCl, and 10% ethanol. The eluent passed through a post-column derivatization instrument (LABRAT, LYM-1060), where it mixed with a 10 μg/mL dimethylene blue zinc chloride double salt (DMB, Sigma, 34108) delivered directly by pump A connected to the online mixer. Detection was performed using a VWD detector at 530 nm wavelength, with data collected via OpenLAB CDS chromatography data software (1260 Infinity II, Agilent, Hong Kong, China).
Sulfite quantification
150 μL of culture supernatant or caecum slurry extract was mixed with 350 μL distilled water and 10 μL 10 M NaOH. Sulfite was quantified using the Total Sulfite Assay Kit (JC-HX-04, HK, China) based on the Pararosaniline Method, following the manufacturer's instructions. The reaction formed a purple-red complex, pararosaniline methylsulfonic acid, which exhibited maximal absorption at 550 nm, and absorbance was measured after a 10-min incubation using a plate reader (UV-2450, SHIMADZU, Japan).
Cell culture and viability assay
The NCM460 human colon epithelial cell line (RRID: CVCL_0460) was maintained in RPMI 1640 basic medium (Gibco, Thermo Fisher Scientific, MA, USA) supplemented with 10% fetal bovine serum (FBS; Thermo Fisher Scientific, Waltham, MA, USA), 100 µg/mL penicillin G, and 100 µg/mL streptomycin sulfate (Invitrogen, Carlsbad, CA, USA). Cells were cultured at 37 °C in a 5% CO 2 humidified incubator.
Cell viability was analyzed by live/dead staining or Cell Counting Kit-8 (CCK8, GLPBIO, GK10001) according to the manufacturer's instructions. For live/dead staining, NCM460 cells (4 × 10 3 /well) in 96-well microplates were cultured for 48 h in 100 μL of 1640 medium. After washing with PBS, cells were prepared for bacterial co-culture. E. coli strains were cultivated overnight at 37 °C in LB, washed in sulfur-free M9 media, and resuspended in M9 medium with L-cysteine or Na 2 S 2 O 3 as the sole sulfur source. Cells were co-cultured with these bacteria for 2 h (150 μL bacterial culture per well). Afterward, cells were stained with 30 μL calcein-AM/PI working solution (2 μM calcein-AM and 4.5 μM propidium iodide) at 37 °C for 20 min, followed by fixation with 0.4% polyformaldehyde. Quadruplicate experiments were conducted, and images of cells were acquired and analyzed using an Olympus IX83 fluorescence microscope (Olympus, Tokyo, Japan). All experiments were performed in quadruplicate. Positive cell percentages and average fluorescence intensity were determined using Image-Pro Plus 6.0. For CCK8 assay, NCM460 cells (5 × 10 3 /well) were cultured overnight in 96-well microplates and treated with 50 μL of bacterial supernatants from M9 medium cultures with 2 mM Na 2 SO 4 as the sole sulfur source. After a 6-h incubation, cells were washed, incubated with 100 μL of RPMI 1640 Medium plus 10 μL CCK-8 reagent, and absorbance at 450 nm was monitored. All experiments were performed in six replicates and blank wells without cells served as controls.
Animal studies
Male SPF C57BL/6 mice (6–8 weeks) were maintained on a standard normal rodent diet (Synergy Bio, AIN-93 M, Jiangsu, China). All the mice used in this study were bred and raised in the animal facility of the First Affiliated Hospital of Sun Yat-sen University. Mice (n = 6) received antibiotic cocktail (Abx) [ 76 ] for 5 days prior to 2% DSS ad libitum in drinking water for 6 days. Mice (n = 6) without Abx pre-treatment was used as vehicle control. Fecal samples from days 3 and 5 were processed by mixing 0.05 g of fresh feces with 500 μL PBS, vortexed for 3 min, and centrifuged at 14,000 × g for 10 min. The supernatant was used for DSS quantification as described in DSS quantification section. For E. coli gavage experiment, mice received Abx-water were subsequently administered E. coli wild type and mutant strains via oral gavage at a dose of 1.0 × 10 9 cfu/200 µL each. DSS was supplied in drinking water at 3% (w/v) for 6 days. Caecum was harvested for sulfite quantification as described in Sulfite Quantification section.
Mice were monitored daily for body weight, stool consistency and stool bleeding. Mice were euthanized by cervical dislocation after 5–6 days of DSS treatment. Colon samples were collected for histological, western blot and qRT-PCR analysis. See online supplemental material for further details.
Bacterial H 2 S Production from λ-Carrageenan and DSS
Bacteria from the LB start culture were harvested, washed, and inoculated in 5 mL sulfur-free M9 medium with 1% λ-carrageenan (inoculum size 1:200, v/v ), incubated overnight at 37 °C while a suspended lead acetate strip monitored H 2 S generation. Medium without inoculum was set up as a negative control.
To assess DSS degradation, mouse and human fecal samples (2 CD subjects and 2 healthy individuals) were cultured in 10 mL LB medium (OD 600 = 1.0). Bacterial pellets were resuspended in 5 mL sulfur-free M9 medium with 1% DSS. DSS levels in supernatants were measured via SEC-HPLC, and H 2 S production was monitored with suspended lead acetate strips.
To investigate H 2 S production from DSS by E. coli WT, mutants, and P. mirabilis , cultures were grown overnight in 10 mL LB medium at 37 °C, 250 rpm. After harvesting, cells were resuspended in 1 mL sulfur-free M9 medium. Pre-incubated DSS medium was prepared using supernatant from M9 medium with 1% DSS and cultures from two CD subjects, as described earlier. Subsequently, 200 µL of this pre-incubated DSS medium and sulfur-free M9 medium with 1% DSS were inoculated with 50 µL of bacterial cultures. Incubation was conducted at 37 °C in a 96-well plate with lead acetate strips for sulfide quantification (see Sulfide Quantification, Lead Acetate Strip section).
Statistical analysis
Statistical analyses were performed with Prism v.8.0 (GraphPad). For two-group comparisons, the statistical significance was determined by unpaired t test or nonparametric Mann–Whitney test as indicated. Multiple group comparisons were made by ANOVA for most of the studies as indicated. Each data point denotes an individual human subject, animal, or biological replicate.
Availability of data and materials
All study data are included in the article and/or SI Appendix. Data are available in a public, open access repository. Gene expression profiling by high-throughput sequencing data have been deposited in Gene Expression Omnibus accession no. (GSE83687 and GSE131032) and Biostudies accession no. (E-MTAB-54674). Metagenomic sequences for the PRISM is available via SRA with BioProject number PRJNA400072. Raw metagenomic data of the FAH-SYSU cohort were deposited in the NCBI public repository (Bioproject #PRJNA793776). HMP IBD metagenomics and transcriptomic data can be accessed at https://ibdmdb.org/tunnel/public/summary.html . The raw RNA-seq data of FAH-SYSU cohort have been deposited in the Genome Sequence Archive [ 77 ] in National Genomics Data Center [ 78 ], China National Center for Bioinformation/Beijing Institute of Genomics, Chinese Academy of Sciences (GSA-Human: HRA007763) that are publicly accessible at https://ngdc.cncb.ac.cn/gsa-human . All plasmids, bacterial mutant strains, and reagents generated in this study are available from the lead contact upon completing Material Transfer Agreement. Any additional information required to reanalyze the data reported in this paper is available from the lead contact upon request.
Burisch J, Vardi H, Schwartz D, Friger M, Kiudelis G, Kupcinskas J, et al. Health-care costs of inflammatory bowel disease in a pan-European, community-based, inception cohort during 5 years of follow-up: a population-based study. Lancet Gastroenterol Hepatol. 2020;5:454–64.
Article PubMed Google Scholar
Targownik LE, Kaplan GG, Witt J, Bernstein CN, Singh H, Tennakoon A, et al. Longitudinal trends in the direct costs and health care utilization ascribable to inflammatory bowel disease in the biologic era: results from a Canadian population-based analysis. Am J Gastroenterol. 2020;115:128–37.
Franzosa EA, Sirota-Madi A, Avila-Pacheco J, Fornelos N, Haiser HJ, Reinker S, et al. Gut microbiome structure and metabolic activity in inflammatory bowel disease. Nat Microbiol. 2019;4:293–305.
Article CAS PubMed Google Scholar
Lloyd-Price J, Arze C, Ananthakrishnan AN, Schirmer M, Avila-Pacheco J, Poon TW, et al. Multi-omics of the gut microbial ecosystem in inflammatory bowel diseases. Nature. 2019;569:655–62.
Article CAS PubMed PubMed Central Google Scholar
Magee EA, Richardson CJ, Hughes R, Cummings JH. Contribution of dietary protein to sulfide production in the large intestine: an in vitro and a controlled feeding study in humans. Am J Clin Nutr. 2000;72:1488–94.
Nguyen LH, Ma W, Wang DD, Cao Y, Mallick H, Gerbaba TK, et al. Association between sulfur-metabolizing bacterial communities in stool and risk of distal colorectal cancer in men. Gastroenterology. 2020;158:1313–25.
Wolfson SJ, Hitchings R, Peregrina K, Cohen Z, Khan S, Yilmaz T, et al. Bacterial hydrogen sulfide drives cryptic redox chemistry in gut microbial communities. Nat Metab. 2022;4:1260.
Mustafa AK, Gadalla MM, Sen N, Kim S, Mu W, Gazi SK, et al. H2S signals through protein S-sulfhydration. Sci Signal. 2009;2:ra72.
Article PubMed PubMed Central Google Scholar
Goubern M, Andriamihaja M, Nubel T, Blachier F, Bouillaud F. Sulfide, the first inorganic substrate for human cells. FASEB J. 2007;21:1699–706.
Zhao WM, Zhang J, Lu YJ, Wang R. The vasorelaxant effect of H2S as a novel endogenous gaseous KATP channel opener. Embo J. 2001;20:6008–16.
Blachier F, Beaumont M, Kim E. Cysteine-derived hydrogen sulfide and gut health: a matter of endogenous or bacterial origin. Curr Opin Clin Nutr Metab Care. 2019;22:68–75.
Wallace JL, Vong L, McKnight W, Dicay M, Martin GR. Endogenous and exogenous hydrogen sulfide promotes resolution of colitis in rats. Gastroenterology. 2009;137:569–78, 578 e561.
Mottawea W, Chiang CK, Mülbauer M, Starr AE, Butcher J, Abujamel T. et al. Altered intestinal microbiota-host mitochondria crosstalk in new onset Crohn's disease. Nat Commun. 2016;7:13419
Motta JP, Flannigan KL, Agbor TA, Beatty JK, Blackler RW, Workentine ML, et al. Hydrogen sulfide protects from colitis and restores intestinal microbiota biofilm and mucus production. Inflamm Bowel Dis. 2015;21:1006–17.
Yang R, Qu C, Zhou Y, Konkel JE, Shi S, Liu Y, et al. Hydrogen sulfide promotes Tet1- and Tet2-mediated Foxp3 demethylation to drive regulatory T cell differentiation and maintain immune homeostasis. Immunity. 2015;43:251–63.
Flannigan KL, Agbor TA, Motta JP, Ferraz JG, Wang R, Buret AG, et al. Proresolution effects of hydrogen sulfide during colitis are mediated through hypoxia-inducible factor-1alpha. FASEB J. 2015;29:1591–602.
Pol A, Renkema GH, Tangerman A, Winkel EG, Engelke UF, de Brouwer APM, et al. Mutations in SELENBP1, encoding a novel human methanethiol oxidase, cause extraoral halitosis. Nat Genet. 2018;50:120–9.
Szabo C, Papapetropoulos A. International union of basic and clinical pharmacology. CII: Pharmacological modulation of H2S Levels: H2S donors and H2S biosynthesis inhibitors. Pharmacol Rev. 2017;69:497–564.
Shatalin K, Shatalina E, Mironov A, Nudler E. H2S: a universal defense against antibiotics in bacteria. Science. 2011;334:986–90.
Mironov A, Seregina T, Nagornykh M, Luhachack LG, Korolkova N, Lopes LE, et al. Mechanism of H(2)S-mediated protection against oxidative stress in Escherichia coli. Proc Natl Acad Sci U S A. 2017;114:6022–7.
Peng H, Zhang Y, Palmer LD, Kehl-Fie TE, Skaar EP, Trinidad JC, et al. Hydrogen sulfide and reactive sulfur species impact proteome S-Sulfhydration and global virulence regulation in Staphylococcus aureus. ACS Infect Dis. 2017;3:744–55.
Uria-Nickelsen MR, Leadbetter ER, Godchaux W 3rd. Sulfonate-sulfur utilization involves a portion of the assimilatory sulfate reduction pathway in Escherichia coli. FEMS Microbiol Lett. 1994;123:43–8.
Huang CJ, Barrett EL. Sequence analysis and expression of the Salmonella typhimurium asr operon encoding production of hydrogen sulfide from sulfite. J Bacteriol. 1991;173:1544–53.
Chiang YL, Hsieh YC, Fang JY, Liu EH, Huang YC, Chuankhayan P, et al. Crystal structure of Adenylylsulfate reductase from Desulfovibrio gigas suggests a potential self-regulation mechanism involving the C terminus of the beta-subunit. J Bacteriol. 2009;191:7597–608.
Cao Q, Liu X, Wang Q, Liu Z, Xia Y, Xun L, et al. Rhodobacteraceae methanethiol oxidases catalyze methanethiol degradation to produce sulfane sulfur other than hydrogen sulfide. mBio. 2024;15:e02907-02923.
Malaeb H, Choucair I, Wang Z, Li XS, Li L, Boyd WC, et al. Stable isotope dilution mass spectrometry quantification of hydrogen sulfide and thiols in biological matrices. Redox Biol. 2022;55:102401.
Devkota S, Wang Y, Musch MW, Leone V, Fehlner-Peach H, Nadimpalli A, et al. Dietary-fat-induced taurocholic acid promotes pathobiont expansion and colitis in Il10-/- mice. Nature. 2012;487:104–8.
Pitcher MC, Beatty ER, Cummings JH. The contribution of sulphate reducing bacteria and 5-aminosalicylic acid to faecal sulphide in patients with ulcerative colitis. Gut. 2000;46:64–72.
Medani M, Collins D, Docherty NG, Baird AW, O’Connell PR, Winter DC. Emerging role of hydrogen sulfide in colonic physiology and pathophysiology. Inflamm Bowel Dis. 2011;17:1620–5.
Feng R, Tian Z, Mao R, Ma R, Luo W, Zhao M, et al. Gut Microbiome-generated phenylacetylglutamine from dietary protein is associated with Crohn’s disease and exacerbates colitis in mouse model possibly via platelet activation. J Crohns Colitis. 2023;17:1833–46.
Kaminski J, Gibson MK, Franzosa EA, Segata N, Dantas G, Huttenhower C. High-specificity targeted functional profiling in microbial communities with ShortBRED. PLoS Comput Biol. 2015;11:e1004557.
Zheng R, Wang C, Sun C. Deep-sea in situ and laboratory multi-omics provide insights into the sulfur assimilation of a deep-sea Chloroflexota bacterium. mBio. 2024;15:e0000424.
Metwaly A, Dunkel A, Waldschmitt N, Raj ACD, Lagkouvardos I, Corraliza AM, et al. Integrated microbiota and metabolite profiles link Crohn’s disease to sulfur metabolism. Nat Commun. 2020;11:15.
Article Google Scholar
Wolf PG, Cowley ES, Breister A, Matatov S, Lucio L, Polak P, et al. Diversity and distribution of sulfur metabolic genes in the human gut microbiome and their association with colorectal cancer. Microbiome. 2022;10:64.
Eyice O, Myronova N, Pol A, Carrion O, Todd JD, Smith TJ, et al. Bacterial SBP56 identified as a Cu-dependent methanethiol oxidase widely distributed in the biosphere. ISME J. 2018;12:145–60.
Xu S, Li X, Zhang S, Qi C, Zhang Z, Ma R, et al. Oxidative stress gene expression, DNA methylation, and gut microbiota interaction trigger Crohn’s disease: a multi-omics Mendelian randomization study. BMC Med. 2023;21:179.
Peters LA, Perrigoue J, Mortha A, Iuga A, Song WM, Neiman EM, et al. A functional genomics predictive network model identifies regulators of inflammatory bowel disease. Nat Genet. 2017;49:1437–49.
Howell KJ, Kraiczy J, Nayak KM, Gasparetto M, Ross A, Lee C, et al. DNA methylation and transcription patterns in intestinal epithelial cells from pediatric patients with inflammatory bowel diseases differentiate disease subtypes and associate with outcome. Gastroenterology. 2018;154:585–98.
Picton R, Eggo MC, Merrill GA, Langman MJS, Singh S. Mucosal protection against sulphide: importance of the enzyme rhodanese. Gut. 2002;50:201–5.
Bick JA, Dennis JJ, Zylstra GJ, Nowack J, Leustek T. Identification of a new class of 5′-adenylylsulfate (APS) reductases from sulfate-assimilating bacteria. J Bacteriol. 2000;182:135–42.
Xu P, Xi Y, Zhu J, Zhang M, Luka Z, Stolz DB, et al. Intestinal sulfation is essential to protect against colitis and colonic carcinogenesis. Gastroenterology. 2021;161(271–286): e211.
Google Scholar
Shen X, Carlstrom M, Borniquel S, Jadert C, Kevil CG, Lundberg JO. Microbial regulation of host hydrogen sulfide bioavailability and metabolism. Free Radical Biol Med. 2013;60:195–200.
Article CAS Google Scholar
Czarnewski P, Parigi SM, Sorini C, Diaz OE, Das S, Gagliani N, et al. Conserved transcriptomic profile between mouse and human colitis allows unsupervised patient stratification. Nat Commun. 2019;10:2892.
Guo J, Shang X, Chen P, Huang X. How does carrageenan cause colitis? A review. Carbohydr Polym. 2023;302:120374.
Tobacman JK. Review of harmful gastrointestinal effects of carrageenan in animal experiments. Environ Health Perspect. 2001;109:983–94.
Wirtz S, Popp V, Kindermann M, Gerlach K, Weigmann B, Fichtner-Feigl S, et al. Chemically induced mouse models of acute and chronic intestinal inflammation. Nat Protoc. 2017;12:1295–309.
Matsuo K, Ota H, Akamatsu T, Sugiyama A, Katsuyama T. Histochemistry of the surface mucous gel layer of the human colon. Gut. 1997;40:782–9.
Moschen AR, Tilg H, Raine T. IL-12, IL-23 and IL-17 in IBD: immunobiology and therapeutic targeting. Nat Rev Gastroenterol Hepatol. 2019;16:185–96.
Levine J, Ellis CJ, Furne JK, Springfield J, Levitt MD. Fecal hydrogen sulfide production in ulcerative colitis. Am J Gastroenterol. 1998;93:83–7.
Suarez FL, Furne JK, Springfield J, Levitt MD. Bismuth subsalicylate markedly decreases hydrogen sulfide release in the human colon. Gastroenterology. 1998;114:923–9.
Wallace JL, Nagy P, Feener TD, Allain T, Ditrói T, Vaughan DJ, et al. A proof-of-concept, Phase 2 clinical trial of the gastrointestinal safety of a hydrogen sulfide-releasing anti-inflammatory drug. Br J Pharmacol. 2020;177:769–77.
Rigottier-Gois L. Dysbiosis in inflammatory bowel diseases: the oxygen hypothesis. Isme J. 2013;7:1256–61.
Fite A, Macfarlane GT, Cummings JH, Hopkins MJ, Kong SC, Furrie E, et al. Identification and quantitation of mucosal and faecal desulfovibrios using real time polymerase chain reaction. Gut. 2004;53:523–9.
Anantharaman K, Hausmann B, Jungbluth SP, Kantor RS, Lavy A, Warren LA, et al. Expanded diversity of microbial groups that shape the dissimilatory sulfur cycle. ISME J. 2018;12:1715–28.
Friedrich CG, Rother D, Bardischewsky F, Quentmeier A, Fischer J. Oxidation of reduced inorganic sulfur compounds by bacteria: emergence of a common mechanism? Appl Environ Microbiol. 2001;67:2873–82.
Xia Y, Lu C, Hou N, Xin Y, Liu J, Liu H, et al. Sulfide production and oxidation by heterotrophic bacteria under aerobic conditions. Isme J. 2017;11:2754–66.
Alsahli S, Al Anazi A, Al Hatlani MM, Kashgari A, Al Sufiani F, Alfadhel M, et al. Severe Crohn’s disease manifestations in a child with cystathionine beta-synthase deficiency. ACG Case Rep J. 2018;5:e93.
Chen S, Zuo S, Zhu J, Yue T, Bu D, Wang X, et al. Decreased expression of cystathionine beta-synthase exacerbates intestinal barrier injury in ulcerative colitis. J Crohns Colitis. 2019;13:1067–80.
Zhang J, Cen L, Zhang X, Tang C, Chen Y, Zhang Y, et al. MPST deficiency promotes intestinal epithelial cell apoptosis and aggravates inflammatory bowel disease via AKT. Redox Biol. 2022;56:102469.
Yamamoto-Furusho J, Salazar-Salas L, Fonseca-Camarillo G, Barreto R. Gene expression of SELENBP1 is upregulated in the colonic mucosa and is associated with a long-term remission in patients with ulcerative colitis. J Crohns Colitis. 2016;10:S480–1.
Koelink PJ, Bloemendaal FM, Li B, Westera L, Vogels EWM, van Roest M, et al. Anti-TNF therapy in IBD exerts its therapeutic effect through macrophage IL-10 signalling. Gut. 2020;69:1053–63.
Flannigan KL, Agbor TA, Blackler RW, Kim JJ, Khan WI, Verdu EF, et al. Impaired hydrogen sulfide synthesis and IL-10 signaling underlie hyperhomocysteinemia-associated exacerbation of colitis. Proc Natl Acad Sci U S A. 2014;111:13559–64.
Tian Z, Zhuang X, Zhao M, Zhuo S, Li X, Ma R, et al. Index-based dietary patterns and inflammatory bowel disease: a systematic review of observational studies. Adv Nutr. 2021;12:2288–300.
Bancil AS, Sandall AM, Rossi M, Chassaing B, Lindsay JO, Whelan K. Food additive emulsifiers and their impact on gut microbiome, permeability, and inflammation: mechanistic insights in inflammatory bowel disease. Journal of Crohns & Colitis. 2021;15:1068–79.
Wastyk HC, Fragiadakis GK, Perelman D, Dahan D, Merrill BD, Yu FQB, et al. Gut-microbiota-targeted diets modulate human immune status. Cell. 2021;184:4137.
Florin TH, Neale G, Goretski S, Cummings JH. The sulfate content of foods and beverages. J Food Compos Anal. 1993;6:140–51.
Florin T, Neale G, Gibson GR, Christl SU, Cummings JH. Metabolism of dietary sulphate: absorption and excretion in humans. Gut. 1991;32:766–73.
Muthukumar J, Chidambaram R, Sukumaran S. Sulfated polysaccharides and its commercial applications in food industries-A review. J Food Sci Technol Mysore. 2021;58:2453–66.
Martino JV, Van Limbergen J, Cahill LE. The role of Carrageenan and Carboxymethylcellulose in the development of intestinal inflammation. Front Pediatr. 2017;5:96.
Sigall-Boneh R, Pfeffer-Gik T, Segal I, Zangen T, Boaz M, Levine A. Partial enteral nutrition with a Crohn’s disease exclusion diet is effective for induction of remission in children and young adults with Crohn’s disease. Inflamm Bowel Dis. 2014;20:1353–60.
Forster SC, Clare S, Beresford-Jones BS, Harcourt K, Notley G, Stares MD, et al. Identification of gut microbial species linked with disease variability in a widely used mouse model of colitis. Nat Microbiol. 2022;7:590–9.
Chen IA, Chu K, Palaniappan K, Ratner A, Huang J, Huntemann M, et al. The IMG/M data management and analysis system v.6.0: new tools and advanced capabilities. Nucleic Acids Res. 2021;49:D751–63.
Letunic I, Bork P. Interactive Tree Of Life (iTOL) v5: an online tool for phylogenetic tree display and annotation. Nucleic Acids Res. 2021;49:W293–6.
Tian Z, Zhuang X, Zhuo S, Zhu Y, Hu S, Zhao M, et al. Dietary inflammatory potential mediated gut microbiota and metabolite alterations in Crohn’s disease: a fire-new perspective. Clin Nutr. 2022;41:1260–71.
Howery KE, Rather PN. Allelic exchange mutagenesis in proteus mirabilis. Methods Mol Biol. 2019;2021:77–84.
Nemet I, Saha PP, Gupta N, Zhu W, Romano KA, Skye SM, et al. A cardiovascular disease-linked gut microbial metabolite acts via adrenergic receptors. Cell. 2020;180(862–877):e822.
Chen T, Chen X, Zhang S, Zhu J, Tang B, Wang A, et al. The genome sequence archive family: toward explosive data growth and diverse data types. Genom Proteom Bioinform. 2021;19:578–83.
Members, C.-N. & Partners. Database resources of the national genomics data center, China national center for bioinformation in 2022. Nucleic Acids Res. 2022;50:D27–38.
Download references
Acknowledgements
We thank the First Affiliated Hospital of Sun Yat-sen University Research Computing for computational resources, maintenance, and support. We thank the First Affiliated Hospital of Sun Yat-sen University Mass Spectrometry Core Laboratory, especially Yuanmei Ye, for their assistance with mass spectrometry analysis.
This work is supported by the National Natural Science Foundation of China (82341217 to M.C. 82100577 to Y.Z., 82270579 to R. F., 82370551 to M. C.), Guangxi Natural Science Foundation (2024GXNSFFA010009 to R.F.).
Author information
Wanrong Luo and Min Zhao contributed equally to this work.
Minhu Chen, Rui Feng and Yijun Zhu contributed equally to this work.
Authors and Affiliations
Department of Gastroenterology, the First Affiliated Hospital, Sun Yat-Sen University, No.58 Zhongshan Er Road, Room 1209, Guangzhou, 510080, China
Wanrong Luo, Liyuan Xiang, Xueting Wu, Shu Xu, Xiaozhi Li, Minhu Chen & Rui Feng
Institute of Precision Medicine, the First Affiliated Hospital, Sun Yat-Sen University, Guangzhou, Guangdong, China
Wanrong Luo, Yang Gao & Yijun Zhu
Department of Gastroenterology, Shenzhen No.3 People’s Hospital, Shenzhen, Guangdong, China
Department of Cardiovascular & Metabolic Sciences, Lerner Research Institute, Cleveland Clinic, Cleveland, OH, USA
Mohammed Dwidar
Center for Microbiome and Human Health, Cleveland Clinic, Cleveland, OH, USA
Bioinformatics Group, Wageningen University, Wageningen, The Netherlands
Marnix H. Medema
School of Life Sciences, University of Warwick, Coventry, UK
Hendrik Schäfer
Key Laboratory of Human Microbiome and Chronic Diseases (Sun Yat-Sen University), Ministry of Education, Guangzhou, Guangdong, China
You can also search for this author in PubMed Google Scholar
Contributions
M.C., M.D., R. F., and Y.Z. designed research; W.L., M.Z., W.X., R.F., S.X., X.L., L.X., X.L, and Y. G. performed research; W.L., M.Z., M.D. and X.L. analyzed data; and M.D. M.H.M, H.S., and Y.Z. wrote the paper.
Corresponding authors
Correspondence to Minhu Chen , Rui Feng or Yijun Zhu .
Ethics declarations
Ethics approval and consent to participate.
Study research protocols were reviewed and approved by the Ethical Committees of the First Affiliated Hospital of Sun Yat-sen University (2016[113]). Written informed consent was obtained from all participants. All animal studies were conducted under protocols approved by the Institutional Animal Care and Use Committee (IACUC) at the First Affiliated Hospital of Sun Yat-sen University (2021 [303], 2023 [183]).
Consent for publication
Not applicable.
Competing interests
The authors declare no competing interests.
Additional information
Publisher’s note.
Springer Nature remains neutral with regard to jurisdictional claims in published maps and institutional affiliations.
Supplementary Information
Supplementary material 1, supplementary material 2, rights and permissions.
Open Access This article is licensed under a Creative Commons Attribution 4.0 International License, which permits use, sharing, adaptation, distribution and reproduction in any medium or format, as long as you give appropriate credit to the original author(s) and the source, provide a link to the Creative Commons licence, and indicate if changes were made. The images or other third party material in this article are included in the article's Creative Commons licence, unless indicated otherwise in a credit line to the material. If material is not included in the article's Creative Commons licence and your intended use is not permitted by statutory regulation or exceeds the permitted use, you will need to obtain permission directly from the copyright holder. To view a copy of this licence, visit http://creativecommons.org/licenses/by/4.0/ . The Creative Commons Public Domain Dedication waiver ( http://creativecommons.org/publicdomain/zero/1.0/ ) applies to the data made available in this article, unless otherwise stated in a credit line to the data.
Reprints and permissions
About this article
Cite this article.
Luo, W., Zhao, M., Dwidar, M. et al. Microbial assimilatory sulfate reduction-mediated H 2 S: an overlooked role in Crohn’s disease development. Microbiome 12 , 152 (2024). https://doi.org/10.1186/s40168-024-01873-2
Download citation
Received : 27 March 2024
Accepted : 13 July 2024
Published : 16 August 2024
DOI : https://doi.org/10.1186/s40168-024-01873-2
Share this article
Anyone you share the following link with will be able to read this content:
Sorry, a shareable link is not currently available for this article.
Provided by the Springer Nature SharedIt content-sharing initiative
- Inflammatory bowel disease
- Inorganic sulfate
- Sulfopolysaccharide
- 3’-Phosphoadenosine-5’-Phosphosulfate PAPS
- Adenosine-5’-Phosphosulfate APS
ISSN: 2049-2618
- General enquiries: [email protected]

An official website of the United States government
The .gov means it’s official. Federal government websites often end in .gov or .mil. Before sharing sensitive information, make sure you’re on a federal government site.
The site is secure. The https:// ensures that you are connecting to the official website and that any information you provide is encrypted and transmitted securely.
- Publications
- Account settings
Preview improvements coming to the PMC website in October 2024. Learn More or Try it out now .
- Advanced Search
- Journal List
- HHS Author Manuscripts

Fundamental limits on the rate of bacterial growth and their influence on proteomic composition
Nathan m. belliveau.
1 Department of Biology, Howard Hughes Medical Institute, University of Washington, Seattle, WA 98105, USA
9 These authors contributed equally
Griffin Chure
2 Department of Applied Physics, California Institute of Technology, Pasadena, CA 91125, USA
10 Present address: Department of Biology, Stanford University, CA 94305, USA
Christina L. Hueschen
3 Department of Chemical Engineering, Stanford University, Stanford, CA 94305, USA
Hernan G. Garcia
4 Department of Molecular Cell Biology and Department of Physics, University of California Berkeley, Berkeley, CA 94720, USA
Jane Kondev
5 Department of Physics, Brandeis University, Waltham, MA 02453, USA
Daniel S. Fisher
6 Department of Applied Physics, Stanford University, Stanford, CA 94305, USA
Julie A. Theriot
Rob phillips.
7 Division of Biology and Biological Engineering, California Institute of Technology, Pasadena, CA 91125, USA
8 Department of Physics, California Institute of Technology, Pasadena, CA 91125, USA
11 Lead contact
AUTHOR CONTRIBUTIONS
Associated Data
- All data, code, and figure generation scripts are publicly available as a GitHub repository ( https://github.com/rpgroup-pboc/growth_limits ) and is accessible via [ https://doi.org/10.5281/zenodo.4091457 ]. We invite the community to fork this repository and open constructive issues with comments regarding the analysis, annotation, or findings of the work.
- The published article includes two data sets generated during this study. Data S1 combines all data of annotated complexes and protein-level abundances, while Data S2 contains abundances of each individual complex.
KEY RESOURCES TABLE
REAGENT or RESOURCE | SOURCE | IDENTIFIER |
---|---|---|
Deposited data | ||
proteomic data | pp. 2344–2358. DOI: | |
proteomic data | pp. 624–635. DOI: | |
proteomic data | . pp. 1184–1193. DOI: | |
proteomic data | pp. 108 – 110. DOI: | |
Software and algorithms | ||
Python version 3.8.8 distributed via Anaconda | Python Software Foundation; Anaconda Org | |
Matplotlib Python Plotting Library version 3.3.4 | Matplotlib Organization | |
Pandas Python DataFrame Library version 1.2.4 | Pydata Organization | |
Adobe Illustrator 2020 | Adobe Incorporated | |
Pathway Tools | SRI International |
- Any additional information required to reproduce this work is available from the Lead contact.
Despite abundant measurements of bacterial growth rate, cell size, and protein content, we lack a rigorous understanding of what sets the scale of these quantities and when protein abundances should (or should not) depend on growth rate. Here, we estimate the basic requirements and physical constraints on steady-state growth by considering key processes in cellular physiology across a collection of Escherichia coli proteomic data covering ≈4,000 proteins and 36 growth rates. Our analysis suggests that cells are predominantly tuned for the task of cell doubling across a continuum of growth rates; specific processes do not limit growth rate or dictate cell size. We present a model of proteomic regulation as a function of nutrient supply that reconciles observed interdependences between protein synthesis, cell size, and growth rate and propose that a theoretical inability to parallelize ribosomal synthesis places a firm limit on the achievable growth rate. A record of this paper’s transparent peer review process is included in the supplemental information .
Identifying factors that determine growth rate has long been central to the study of bacterial physiology. Belliveau and Chure et al. present order-of-magnitude estimates of the proteomic requirements for various biological processes in Escherichia coli across growth rates and compare them to experimental measurements. This reveals translation to be key in defining the growth rate, while other processes are precisely tuned with little excess. The influence of physiological parameters on growth rate is explored using a simple mathematical model.
Graphical Abstract

INTRODUCTION
The observed range of bacterial growth rates is enormously diverse. In natural environments, some microbial organisms may double only once per year ( Mikucki et al., 2009 ) while in comfortable laboratory conditions, growth can be rapid with several divisions per hour ( Schaechter et al., 1958 ). This six-order-of-magnitude difference in timescales of growth encompasses different microbial species and lifestyles, yet even for a single species such as Escherichia coli , the growth rate can be modulated over a large range by tuning the type and amount of nutrients in the growth medium ( Liu et al., 2005 ). This remarkable plasticity in growth rate illustrates the intimate relationship between environmental conditions and the rates at which cells convert nutrients into new cellular material—a relationship that has remained a major topic of inquiry in bacterial physiology for over a century ( Jun et al., 2018 ).
A key discovery in bacterial physiology of the past 70 years was the identification of bacterial “growth laws” ( Schaechter et al., 1958 ); empirical relationships that relate the bacterial growth rate to the protein and RNA composition of the intracellular milieu in a number of different species. Over the past decade, a flurry of work ( Molenaar et al., 2009 ; Scott et al., 2010 ; Klumpp and Hwa, 2014 ; Basan et al., 2015 ; Dai et al., 2016 ; Erickson et al., 2017 ) has examined these growth laws at a quantitative level, developing a series of phenomenological models from which the growth laws naturally emerge. In parallel, a “molecular revolution” in biology has yielded an increasingly refined molecular census of the cell, particularly for bacteria such as the microbial workhorse E. coli ( Schmidt et al., 2016 ; Davidi et al., 2016 ). In light of the now expansive trove of quantitative biological data, it is important to revisit several of the evergreen questions about bacterial growth and physiology that were originally raised by microbiologists in the middle of the 20th century. Further, it is timely to consider whether different measurements of the proteomic content are concordant. Specifically, what biological processes are the primary determinants for how quickly bacterial cells can grow and reproduce. Why do cells modulate the absolute numbers and relative ratios of their molecular constituents in response to changes in growth rate or nutrient availability? These questions remain under intense inquiry and have implicated processes ranging from ribosomal biogenesis and transcription to cell wall and lipid synthesis as key determinants of growth rate and cell size ( Bremer and Dennis, 2008 ; Scott et al., 2010 ; Si et al., 2017 ; Vadia et al., 2017 ; Harris and Theriot, 2018 ; Büke et al., 2020 ; Zhang et al., 2020 ).
In this work, we consider these two questions in E. coli by considering both the biosynthetic capacity of key cellular processes—meaning, the minimal number of enzymes needed to synthesize one cell’s worth of a particular biomolecule given the observed doubling time—as well as the physical constraints given the now well-characterized change in cell size as a function of growth rate ( Taheri-Araghi et al., 2015 ; Si et al., 2017 ; Basan et al., 2015 ). As a result of an array of high-quality proteome-wide measurements under diverse growth conditions, we have generated a census that allows us to explore how the number of key molecular players change as a function of growth rate. Here, we have assembled a singular dataset of protein copy numbers using measurements collected over the past decade via mass spectrometry ( Schmidt et al., 2016 ; Peebo et al., 2015 ; Valgepea et al., 2013 ) or ribosomal profiling ( Li et al., 2014 ) of the composition of the E. coli proteome across a gamut of growth rates. Due to notable changes in both cell size and cellular composition as a function of growth rate ( Bremer and Dennis, 2008 ; Taheri-Araghi et al., 2015 ), as well as differences in normalization and standardization schemes used in each experimental work, substantial care was taken to ensure consistency on a per cellular basis ( Figure 1A ; see the supplemental information for a detailed analysis and further discussion). To our knowledge, this compiled and curated dataset represents the most comprehensive view to date of the E. coli proteome, covering ≈4,000 proteins and 36 unique growth rates, with the observed abundance of any given protein being directly comparable between datasets and across growth rates. This allows us to interrogate the E.-coli -specific physiology underlying the observed abundances while minimizing the effects of experimental noise as ≈75% of the proteins are observed in at least two separate datasets.

(A) The growth rate dependent changes in bacterial size and composition provide a basis to both predict the protein abundances necessary to double a cell, and compile and compare proteomic measurements on a per cell basis across the recent datasets from Schmidt et al. (2016) ; Li et al. (2014) ; Peebo et al. (2015) , and Valgepea et al. (2013) . Predictions rely on the wealth of molecular turnover rate measurements and additional data tabulated on the BioNumbers Database ( bionumbers.hms.harvard.edu , Milo et al. [2010 ]).
(B) We consider an array of processes necessary for a cell to double its molecular components, broadly grouped into four classes. These categories are nutrient transport across the cell membrane, cell envelope biogenesis, energy production (namely, ATP synthesis), and processes associated with the central dogma. Numbers shown are the approximate number of complexes of each type observed at a growth rate of 0.5 h −1 , or a cell doubling time of ≈5,000 s.
By compiling molecular turnover rate measurements for many of the fundamental processes associated with bacterial growth, we make quantitative order-of-magnitude estimates across key cellular processes in nutrient transport, cell envelope biogenesis, energy generation, and the central dogma ( Table S1 ; schematized in Figure 1B ) to determine whether our current understanding of the kinetics of these processes are sufficient to explain the magnitude of the observed protein copy numbers across conditions. We consider each set of processes in turn and explore which process(es) may impose a limit as to how quickly cells can replicate. The census, combined with these estimates, provide a window into the question of whether the rates of central processes such as energy generation or DNA synthesis vary systematically as a function of cell growth rate by altering protein copy number, and in particular, whether any of these processes pose a molecular bottleneck or rate-limiting step. Though of course others have systematically examined the growth rate dependence for specific processes, our intent here was rather to provide a synthesis across a broad range of processes required in cellular growth.
For the majority of the processes considered, we find that the protein copy numbers are apparently tuned for the task of cell doubling across a continuum of growth rates. This suggests that most processes must be operating near their maximal biosynthetic capacity, particularly under moderate to fast growth rates, with cellular protein abundances increasing at faster growth rates to support the more rapid cell doublings. This observation contrasts with the perspective that there exists any single process that is the arbiter of growth rate. Rather, it is through the coordinated increase in protein abundance across these disparate processes that E. coli is able to increase its growth rate as nutrient conditions improve. This hypothesis is bolstered by the observation that, when we consider the change in cell size and the diminishing surface area to volume ratio at faster growth rates, there still appears to be sufficient space in the cell membrane for the key proteins required for energy production and nutrient uptake.
Given an observed importance of parallelization, where the synthesis of additional proteins provides increased biosynthetic capacity of each cellular process, our analysis also provides insight into the well-characterized dependence of growth rate on ribosomal mass fraction. Here, a theoretical inability to parallelize ribosomal protein synthesis places a firm upper bound on the achievable growth rate that is observed at moderate to fast growth rates, where ribosomes appear maximally utilized and where the proteomic requirements for other cellular processes still appear to be maintained. Importantly, the strict dependence between the maximal growth rate and ribosomal mass fraction coincides with the regime where the growth laws appear most valid ( Amir, 2017 ; Scott et al., 2010 ). This enables us to suggest that the long-observed correlation between growth rate and cell size ( Schaechter et al., 1958 ; Si et al., 2017 ) can be simply attributed to a required increase in absolute number of ribosomes per cell under conditions supporting extremely rapid growth. To better understand how the observed alterations in absolute protein abundances influence growth rate across different nutrient conditions, we consider a minimal model of cellular growth rate control to quantitatively explore the influence of proteomic composition and cell size on growth rate. Our conclusions from these analyses provide important insight into how E. coli regulates growth across both nutrient-poor and nutrient-rich regimes and identifies fundamental constraints in bacterial growth more broadly.
The “order-of-magnitude estimation protocol”
This work relies heavily on “back-of-the-envelope” estimates to understand the growth-rate-dependent abundances of molecular complexes. This moniker arises from the limitation that any estimate should be able to fit on the back of a postage envelope, meaning that we frequently make reasonable assumptions to arrive at approximate answers rather than performing a detailed calculation with the objective of high precision and many significant digits ( Mahajan, 2010 ). All of the estimates performed in this work follow the same basic “protocol,” as is outlined in Figure 2A . For any given cellular process, we begin our estimate by first determining “how much” of a given molecule must be transported or synthesized to enable a doubling of cell biomass. For example, these molecules may be carbon atoms (in the form of transported sugars), the lipids making up the cell membrane, or the ATP that is consumed in doubling of the proteome.

(A) Nearly all order-of-magnitude estimates undertaken in this work follow the same basic estimate scheme. For a given process, we first consider how much of a given material X (e.g., carbon atoms, lipid molecules, or ATP) the cell must transport or synthesize. This is dependent on the elemental composition of the cellular dry mass, the cellular surface area, or the cellular energy expenditure. With a value for the amount to be synthesized, we consider how quickly the process can occur given the literature values of the in vivo or in vitro kinetics of the key complexes involved in the process. The number of complexes needed to meet the synthetic or transport demand is dependent on the doubling time of the cell, which can be taken to be a specific value or evaluated over a continuum of growth rates. Together, these three quantities can be combined to estimate the number of complexes needed to meet the demand in a given time, highlighted in red, with order-of-magnitude or better precision. Example estimates are given for (B) the number of carbon transporters, (C) the number of lipid synthesis enzymes, and (D) the number of ATP synthases. Similar diagrams of other estimates can be found in Table S1 .
With numbers in hand for the amount of the material to be synthesized/transported we turn our focus to the central players of these works, the molecular complexes. The vast literature of in vivo and in vitro biochemistry has left us replete with quantitative properties of kinetic rates. We use this primary literature— and, when possible, their entries on the BioNumbers database ( Milo et al., 2010 ) denoted with their accession numbers as BNIDs) —as a means to approximate the typical flux of material through a single enzyme, transporter, or biochemical pathway. To assemble these quantities into a singular estimate for the number of molecular complexes needed for a given process, we require a sense of the amount of time in which the synthesis/transport must take place. In this work, we consider two temporal regimes. First, we present a “point estimate” of the number of complexes needed to facilitate a doubling time of around 5,000 s, or a growth rate λ of ≈0.5 h −1 . We choose this archetypal growth rate as it is the clearest narrative way to present our estimates and is the growth regime that the proteomic data heavily sample. This point estimate is always presented as a translucent brown point in the plots that follow.
Additionally, we also explore these estimates across a continuum of growth rates. The continuum estimates, displayed as a gray curve on the various plots, relax some of the assertions made while formulating the point estimate and incorporate empirical findings from the literature of how cell masses, volumes, and surface areas scale with the cellular growth rate ( Si et al., 2017 , 2019 ; Basan et al., 2015 ; Ojkic et al., 2019 ). As we rely on empirical descriptions of how volume, mass, and surface area scale with the growth rate, the point and continuum estimates may not always exactly agree. Finally, as growth rates become very slow ( λ ≈ 0.2 h − 1 , t double ≈ 3 h ), protein degradation and cellular homeostasis may become an important factor ( Feist et al., 2007 ; Stouthamer, 1973 ), one that we have chosen to ignore for the purposes of this work. Thus, we have indicated this region in the plots that follow as a dashed line to indicate that estimates in this regime may be too simplistic.
Figure 2A shows a schematic representation of this “estimation protocol.” In Figure 2B , we present a series of three examples, each considering a different scaling dependence as shown in the top panel of A. In estimating the number of carbon transporters ( Figure 2B ), we consider how many carbon atoms must be transported to double the biomass and use the elemental composition of the cell dry mass as a means to do so. In Figure 2C , we consider the cell surface area to estimate how many lipid molecules must be synthesized and, as described later, the number of ACP dehydratases needed for their synthesis. Finally, Figure 2D shows an estimate for the number of ATP molecules that must be consumed, given that peptide bond formation is the primary energy expenditure of growth. Similar diagrams for all estimate categories outlined in Figure 1B are provided in Table S1 .
Nutrient transport
Here, we begin by considering the critical transport processes diagrammed in Figure 1B . We consider how cells scavenge building blocks (namely carbon, sulfur, and phosphorus) from the environment and the kinetics of nutrient transporters. We then calculate an estimated number of transporters required to support a given growth rate, and we compare these calculations with proteomic data.
In order to build new cellular mass, the molecular and elemental building blocks must be scavenged from the environment in different forms. Carbon, for example, is acquired via the transport of carbohydrates and sugar alcohols with some carbon sources receiving preferential treatment in their consumption ( Monod, 1947 ). Phosphorus, sulfur, and nitrogen, on the other hand, are harvested primarily in the forms of inorganic salts, namely phosphate, sulfate, and ammonium/ammonia ( Jun et al., 2018 ; Assentoft et al., 2016 ; Stasi et al., 2019 ; Antonenko et al., 1997 ; Rosenberg et al., 1977 ; Willsky et al., 1973 ). All of these compounds have different membrane permeabilities ( Phillips, 2018 ), and most require some energetic investment either via ATP hydrolysis or through the proton electrochemical gradient to bring the material across the hydrophobic cell membrane.
The elemental composition of E. coli has received much quantitative attention over the past half century ( Neidhardt et al., 1991 ; Taymaz-Nikerel et al., 2010 ; Heldal et al., 1985 ; Bauer and Ziv, 1976 ), providing us with a starting point for estimating how many atoms of each element must be scavenged from the environment: ≈50% carbon (BioNumbers, ID [BNID]: 100649; obtained from the BioNumbers database, Milo et al. [2010 ], ≈15% nitrogen [BNID: 106666], ≈3% phosphorus [BNID: 100653], and 1% sulfur [BNID: 100655]) with the remainder being attributable to oxygen, hydrogen, and various transition metals. Here, we estimate the abundance and growth rate dependence of a variety of transporters responsible for carbon uptake, and provide more extensive investigation of the other critical elements in the supplemental information “Estimates across fundamental biological processes.” Using ≈0.3 pg as the typical E. coli dry mass at a growth rate of ≈0.5 h −1 (BNID: 103904), coupled with the approximation that z50% of this mass is carbon, we estimate that ≈1×10 10 carbon atoms must be brought into the cell in order to double all of the carbon-containing molecules.
Typical laboratory growth conditions provide carbon as a single class of sugar (such as glucose, galactose, or xylose) often transported cross the cell membrane by a transporter complex specific to that particular sugar. One such mechanism of transport is via the phosphotransferase system (PTS), which is a highly modular system capable of transporting a diverse range of sugars with high specificity ( Escalante et al., 2012 ). The glucose-specific component of this system transports ≈200 glucose molecules (≈1,200 carbon atoms) per second per transporter (BNID: 114686). Making the assumption that this is a typical sugar transport rate for the PTS system, coupled with the need to transport ≈1 × 10 10 carbon atoms, we then expect on the order of ≈2,000 transporters must be expressed per cell in order to bring in enough carbon atoms. We find, however, that the experimental measurements exceed this by several fold ( Figure 3A ), implying that the cell is capable of transporting more carbon atoms than strictly needed for biosynthesis. This holds true even at the fastest growth rates, with cells exhibiting no apparent growth rate dependence.

Dashed black lines indicate order of magnitude estimate needed at a growth rate of ≈0.5 per h (light-brown point), while the gray line accounts for the growth rate dependence changes in cell size and doubling time. Dashed region of gray line represents growth rates with a doubling time ≥ 3 h where protein maintenance costs may be important but are not considered.
(A) Estimate for the minimum number of generic carbohydrate transport systems. Colored points correspond to the mean number of complexes involved in carbohydrate import (complexes annotated with the gene ontology terms GO:0009401 and GO:0098704) for different growth conditions across different published datasets.
(B) Number of PitA phosphate transport systems needed to maintain a 3% phosphorus dry mass.
(C) Number of CysUWA complexes necessary to maintain a 1% sulfur E. coli dry mass and the experimentally observed complex copy numbers using the transporter stoichiometry [CysA] 2 [CysU][CysW][Sbp/CysP].
(D) Number of ACP dehydratases necessary to form functional phospholipids, which is assumed to be a rate-limiting step on lipid synthesis, and the experimentally observed complex copy numbers using the stoichiometries [FabA] 2 and [FabZ] 2 .
(E) Number of peptidoglycan transpeptidases needed to complete maturation of the peptidoglycan and experimental measurements of the transpeptidase complexes, following the stoichiometries [MrcA] 2 , [MrcB] 2 , [MrdA] 1 , and [MrdB] 1 .
(F) Number of F 1 -F 0 ATP synthase complexes needed to accommodate peptide bond formation and other NTP dependent processes and experimental measurements following the stoichiometry [AtpE] 10 [AtpF] 2 [AtpB][AtpC][AtpH] [AtpA] 3 [AtpG][AtpD] 3 .
(G) Number of electron transport chain complexes needed to maintain a membrane potential of −200 mV. Points in plot correspond to the average number of complexes identified as being involved in aerobic respiration by the GO identifier GO:0019646. These complexes include cytochromes bd1 ([CydA][CydB][CydX] [CydH]), bdII ([AppC][AppB]), bo 3 ,([CyoD][CyoA][CyoB][CyoC]) and NADH:quinone oxioreductase I ([NuoA][NuoH][NuoJ][NuoK][NuoL][NuoM][NuoN][NuoB] [NuoC] [NuoE][NuoF][NuoG][NuoI]) and II ([Ndh]).
This constancy in the expression appears to be specific to glucose transporters, which are known to be the preferential carbon source ( Monod, 1947 ; Liu et al., 2005 ; Aidelberg et al., 2014 ), and stands in contrast with other species of transporters for glycerol, xylose, or fructose, which we find match the required transporter abundances according to the achieved doubling time (adjusting for the specific carbon source in terms of number of carbon atoms per molecule and the rate of transport for the particular transporter species) ( Figure S1 ). This also contrasts with our observations for uptake of phosphorus and sulfur, which turn out to align well with our expectations across different growth conditions ( Figures 3B and and3C 3C and discussed further in the supplemental information “estimates across fundamental biological processes”). In summary, we researched and integrated quantitative information about cellular composition, nutrient transport, and transporter kinetics to estimate the minimum nutrient transporter copy numbers required across a spectrum of growth rates, and a comparison of these calculations to proteomic data suggests E. coli devotes excess proteomic resources toward glucose uptake but otherwise tunes transporter copy numbers to better match the nutrient requirements given their doubling time.
Lastly, we consider nutrient transport in the context of a different question: what sets an upper limit on very fast growth? If acquisition of nutrients was acting as a bottleneck on cellular growth, the growth rate could be theoretically increased simply by expressing more transporters, but is this feasible at a physiological level? A way to approach this question is to compute the amount of space in the bacterial membrane that could be occupied by nutrient transporters. Considering a rule-of-thumb for the surface area of E. coli of about 5 μm 2 (BNID: 101792), we expect an areal density for 2,000 transporters to be approximately a few hundred transporters per μm 2 . For a typical transporter occupying about 50 nm 2 , this amounts to about only ≈1% of the total inner membrane surface area ( Szenk et al., 2017 ). In contrast, bacterial cell membranes typically have densities of ≈1 × 10 5 proteins/mm 2 ( Phillips, 2018 ), with roughly 60% of the surface area occupied by protein (BNID: 100078), implying that the cell could easily accommodate more transporters. There are, however, additional constraints on the space that can be devoted to nutrient uptake due to occupancy by proteins involved in processes such as cell wall synthesis and energy production, and we will consider this further in the coming sections.
Cell envelope biogenesis
In this section, we consider the synthesis of lipids as well as the complexes involved in assembling the peptidoglycan scaffold that makes up the cell envelope. We discuss rate-limiting steps in fatty acid and peptidoglycan synthesis, calculate the copy number of fatty-acid synthases and peptidoglycan transpeptidases required to support a given growth rate, and compare these predictions to data. In contrast to nutrient transporters, which support the synthesis of biomolecules throughout the cell and therefore need to scale with the cell size, here, we must consider the synthesis of components that will need to scale with the surface area of the cell.
E. coli is a rod-shaped bacterium with a remarkably robust length-to-width aspect ratio of ≈4:1 ( Harris and Theriot, 2018 ; Ojkic et al., 2019 ). The membranes of E. coli are composed of a variety of different lipids, each of which are unique in their structures and biosynthetic pathways ( Sohlenkamp and Geiger, 2016 ). Recently, a combination of stochastic kinetic modeling ( Ruppe and Fox, 2018 ) and in vitro kinetic measurements ( Ranganathan et al., 2012 ; Yu et al., 2011 ) has revealed remarkably slow steps in the fatty-acid synthesis pathways, which may serve as the rate-limiting reactions for making new membrane fatty acids (that become components of a variety of membrane lipids) in E. coli . One such step is the removal of hydroxyl groups from the fatty-acid chain by ACP dehydratase that leads to the formation of carbon-carbon double bonds. This reaction, catalyzed by proteins FabZ and FabA ( Yu et al., 2011 ), has been estimated to have kinetic turnover rates of ≈1 dehydration per second per enzyme ( Ruppe and Fox, 2018 ). Thus, given this rate and the need to synthesize ≈2 × 10 7 lipids over 5,000 s, one can estimate that a typical cell requires ≈4,000 ACP dehydratases. This is in reasonable agreement with the experimentally observed copy numbers of FabZ and FabA ( Figure 3D ), though here, we also find notable discordance between measurements from different studies that may reflect systematic biases in how these measurements were performed.
The exquisite control of bacteria over their cell shape is due primarily to a stiff, several nanometer thick meshwork of polymerized disaccharides that makes up the cell wall termed the peptidoglycan. The formation of the peptidoglycan is an intricate process involving many macromolecular players ( Shi et al., 2018 ; Morgenstein et al., 2015 ), whose coordinated action synthesizes the individual subunits and integrates them into the peptidoglycan network that maintains cell shape and integrity even in the face of large-scale chemical and osmotic perturbations ( Harris and Theriot, 2018 ; Shi et al., 2018 ). Due to the extensive degree of chemical crosslinks between glycan strands, the entire peptidoglycan is a single molecule comprising ≈3% of the cellular dry mass (BNID: 1019360), making it the most massive molecule in E. coli . The polymerized unit of the peptidoglycan is a N-acetylglucosamine and N-acetylmuramic acid disaccharide, of which the former is functionalized with a short pentapeptide. With a mass of ≈1,000 Da, this unit, which we refer to as a murein subunit, is polymerized to form long strands in the periplasm which are then attached to each other via their peptide linkers. Together, these quantities provide an estimate of ≈5 × 10 6 murein subunits per cell.
There are various steps which one could consider a priori to be a limiting process in the synthesis of peptidoglycan, including the biosynthesis steps that occur in the cytoplasm, the transglycosylation reaction, which adds new subunits to the glycan strands, and the formation of the peptide crosslinks between strands ( Shi et al., 2018 ; Morgenstein et al., 2015 ; Lovering et al., 2012 ; Barreteau et al., 2008 ). Despite the extensive mechanistic characterization of these components, quantitative characterization of the individual reaction rates along their entire kinetic pathway remain scarce and make identification of any particularly slow steps difficult. However, recent measurements for the crosslinking machinery (transpeptidases, Catherwood et al., 2020 ) of the peptidodglycan, which provides lateral structural integrity to the peptidoglycan shell, have found the turnover of transpeptidases to be rather slow (≈2 crosslinking reactions per second). As the primary mechanism of subunit integration occurs by a complex with both transglycosylation and transpeptidation activities ( Shi et al., 2018 ), we therefore consider the transpeptidation reaction as a reasonable candidate for a rate-limiting step in growth as it is vital for cell size and shape homeostasis. We estimate that on the order of ≈100 transpeptidases per cell are needed for complete maturation of the peptidoglycan, given a division time of ≈5,000 s; a value that is comparable with experimental observations ( Figure 3E ). Expanding this estimate to account for the changing mass of the peptidoglycan as a function of growth rate (gray line in Figure 3E ) predicts an order-of-magnitude increase in the abundance of the transpeptidases when the growth rate is increased by a factor of four. Here, however, the measured complex abundances across the different proteomic datasets show systematic disagreements and obfuscates any significant dependence on growth rate.
Lastly, we consider whether cell envelope biogenesis may set a cap on fast growth. While the processes explored above represent a small portion of the proteins devoted to cell envelope biogenesis, we find it unlikely that envelope biogenesis limits cellular growth in general. The relative amount of mass required for lipid and peptidoglycan components will decrease at faster growth rates due to a decrease in the cell’s surface area to volume ratio. Furthermore, despite the slow catalytic rate of fatty-acid synthesis and transpeptidation, there appears to be sufficient protein abundance to support growth. For FabZ and FabA in lipid synthesis, experimental data and recent computational modeling has shown that the rate of fatty-acid synthesis can be drastically increased by increasing the concentration of FabZ ( Yu et al., 2011 ; Ruppe and Fox, 2018 ). With a proteome size of ≈ 3 × 10 6 proteins, a hypothetical 10-fold increase in expression from 4,000 to 40,000 ACP dehydratases would result in a paltry ≈1% increase in the size of the proteome.
Energy production
Cells consume and generate energy predominantly in the form of nucleoside triphosphates (NTPs). The high-energy phosophodiester bonds of (primarily) ATP power a variety of cellular processes that drive biological systems away from hermodynamic equilibrium. We therefore turn to the synthesis of ATP as another process that may limit growth, which will also require us to consider the maintenance of the electrochemical proton gradient that powers it. In this section, we calculate the energy required to build a daughter cell, the number of ATP synthases needed to supply this energy budget, and the number of electron transport complexes necessary to power the ATP synthases. We then compare these predictions with proteomic data.
Hydrolysis of the terminal phosphodiester bond of ATP into ADP (or alternatively GTP into GDP) and an inorganic phosphate provides the thermodynamic driving force in a wide array of biochemical reactions. One such reaction is the formation of peptide bonds during translation, which requires ≈2 ATPs for the charging of an amino acid to the tRNA and ≈2 GTPs for the formation of each peptide bond. Assuming the ATP costs associated with error correction and post-translational modifications of proteins are negligible, we can make the approximation that each peptide bond has a net cost of ≈4 ATP (BNID: 101442). Formation of GTP from ATP is achieved via the action of nucleoside diphosphate kinase, which catalyzes this reaction without an energy investment ( Lascu and Gonin, 2000 ). We therefore consider all NTP requirements of the cell to be functionally equivalent to being exclusively ATP. In total, the energetic costs of peptide bond formation consumes ≈80% of the cells ATP budget (BNID: 107782, 106158, 101637, 111918; Lynch and Marinov [2015 ]; Stouthamer [1973 ]) and is primarily produced by the F 1 -F 0 ATP synthase—a membrane-bound rotary motor which under ideal conditions can yield ≈300 ATP per second (BNID: 114701; Weber and Senior [2003 ]).
To estimate the total number of ATP equivalents consumed during a cell cycle, we make the approximation that there are ≈ 3 × 10 6 proteins per cell with an average protein length of ≈300 peptide bonds (BNID: 115702, 108986, 104877). With ≈4 ATP equivalents per peptide bond, we find that the typical E. coli cell consumes ≈ 5 × 10 9 ATP per cell cycle on protein synthesis alone. Assuming that each ATP synthases operates at its maximal speed, ≈3,000 ATP synthases are needed to keep up with the energy demands of the cell. This estimate is comparable with the experimental observations, shown in Figure 3F . Since this estimate assumes all ATP is synthesized via ATP synthase and neglects synthesis via fermentative metabolism, this may explain why at the fastest growth rates (≈ 2 h −1 ), our continuum estimate predicts more synthase than is experimentally observed (data points below the gray line in Figure 3F at fast growth rates). Here, E. coli enters a type of overflow metabolism where non-respiratory routes for ATP synthesis become more pronounced and provide the remaining ATP demand ( Molenaar et al., 2009 ; Zhuang et al., 2011 ; Szenk et al., 2017 ).
In order to produce ATP, the F 1 -F 0 ATP synthase itself must consume energy. Rather than burning through its own product (and violating thermodynamics), this intricate macromolecular machine has evolved to exploit the electrochemical potential established across the inner membrane through cellular respiration. This electrochemical gradient is manifest by the pumping of protons into the intermembrane space via the electron transport chains as they reduce NADH. In E. coli , this potential difference is ≈−200 mV (BNID: 102120). However, each rotation of an ATP synthase shuttles ≈4 protons into the cytosol (BNID: 103390). With a few thousand ATP synthases producing ATP at their maximal rate, the potential difference would be rapidly abolished in a few milliseconds if it were not being actively maintained. A recent work ( Szenk et al., 2017 ) examined the respiratory capacity of the E. coli electron transport complexes using structural and biochemical data, revealing that each electron transport chain rapidly pumps protons into the intermembrane space at a rate of ≈1,500 protons per second (BIND: 114704, 114687). Using our estimate of the number of ATP synthases required per cell, coupled with these recent measurements, we estimate that ≈3000 electron transport complexes would be necessary to facilitate the ≈5 × 10 6 protons per second diet of the cellular ATP synthases. This estimate is in agreement with the number of complexes identified in the proteomic datasets ( Figure 3F ). Altogether, the agreement between our two estimates and the proteomic data supports the hypotheses embedded in our calculations: each ATP synthase is accompanied by ≈1 functional electron transport chain, with both complexes operating near their maximum rate across a range of growth conditions.
Limits on biosynthesis within a crowded cell
Our estimates thus far have focused on the biochemistry at the periphery of the cell, with the processes of nutrient transport, cell envelope biogenesis, and energy generation all requiring space to perform their biological functions. The cell’s surface area, however, does not scale as rapidly as cell size ( Harris and Theriot, 2018 ), and there will be diminishing space available at the periphery to support the proteomic requirements at faster growth rates. It is therefore necessary to consider the consequences of a changing cell size and surface area to volume ratio in our effort to identify limitations on growth. Here, we use our analysis of ATP production to better understand this constraint.
In our estimate of ATP production above we found that a cell demands about 5 × 10 9 . ATP per cell cycle or ≈1 × 10 6 ATP/s. With a cell volume of roughly 1 fL (BNID: 100004), this corresponds to about 2 × 10 10 ATP per fL of cell volume, in line with previous estimates ( Stouthamer, 1973 ) and within 3–4 fold of more extensive calculations ( Feist et al., 2007 ; Szenk et al., 2017 ). In Figure 4A , we plot this ATP demand as a function of the surface area to volume ratio in green, where we have considered a range of cell shapes from spherical to rod-shaped with an aspect ratio (length/width) equal to 4. In order to consider the maximum ATP that could be produced, we consider the amount of ATP that can be generated by a membrane filled with ATP synthase and electron transport complexes and a maximal production rate of about 3 ATP / (nm 2 •s) ( Szenk et al., 2017 ). This is shown in blue in Figure 4A , which shows that at least for the growth rates observed (right column in plot), the energy demand is roughly an order of magnitude less. Interestingly, Szenk et al. (2017) found that ATP production by respiration is less efficient than by fermentation on a per membrane area basis, due to the additional proteins of the electron transport chain. This suggests that, even under anaerobic growth, cells will have sufficient membrane space for ATP production.

(A) Scaling of ATP demand and maximum ATP production through respiration as a function of surface area to volume ratio. Cell volumes of 0.5 fL to 50 fL were considered, with the dashed ( - -) line corresponding to a sphere and the dash-dot line (−.) reflecting a rod-shaped bacterium like E. coli with a typical aspect ratio (length/width) of 4 ( Shi et al., 2018 ). The ATP demand is calculated as 10 6 ATP/(μm 3 s), while the maximum ATP production rate is taken to be 3 ATP / (nm 2 •s) ( Szenk et al., 2017 ), with calculations of E. coli volume and surface area detailed in supplemental information section “estimation of cell size and surface area.” In this calculation, 50% of the bacterial inner membrane is assumed to be protein, with the remainder lipid.
(B) Total protein mass per μm 2 calculated for proteins with inner membrane annotation (GO term: 0005886).
(C) Relative protein abundances are grouped by their COG annotations (“metabolic,” “cellular processes and signaling,” “information storage and processing,” and “poorly characterized or not annotated”) for the data from Schmidt et al. (2016) . Metabolic proteins are further separated into respiration (F 1 -F 0 ATP synthase, NADH dehydrogenase I, succinate:quinone oxidoreductase, cytochrome bo 3 ubiquinol oxidase, cytochrome bd-I ubiquinol oxidase) and carbohydrate transport (GO term: GO:0008643). Note that the elongation factor EF-Tu can also associate with the inner membrane but was excluded in this analysis due to its high relative abundance (roughly identical to the summed protein shown in B).
(D) Relative cytosolic protein abundances (GO term: 0005886), grouped by their COG annotations, are plotted as a function of growth rate.
(E) The relative cytosolic protein abundances (GO term: 0005886) associated with the “information storage and processing” and “metabolic” COG categories are plotted against each other and highlight the larger mass fraction devoted to “information storage and processing” at faster growth rates.
Importantly, this analysis highlights that there will indeed be a maximum attainable cell size due to the limited capacity to provide resources as the cell increases in size. The maximum energy production shown in Figure 4A , however, does represent a somewhat unachievable limit since the inner membrane also includes other proteins like those we have considered for nutrient transport and cell wall biogenesis. To better understand the overall proteomic makeup of the inner membrane, we therefore used gene ontology (GO) annotations ( Ashburner et al., 2000 ; The Gene Ontology Consortium, 2019 ) to identify all proteins embedded or peripheral to the inner membrane (GO term: 0005886). Those associated but not membrane-bound include proteins such as MreB and FtsZ that must nonetheless be considered as a vital component occupying space on the membrane. Surprisingly, we find that the total protein mass per μm 2 is nearly constant across growth rates ( Figure 4B ), even though the volumetric demand for resources grows with cell size and growth rate. Interestingly, when we consider the distribution of proteins grouped by their clusters of orthologous groups (COG) ( Tatusov et al., 2000 ), the relative abundance of each category is nearly constant across growth rates ( Figure 4C ). This suggests that no one process (energy production, nutrient uptake, etc.) is dominating even at fast growth rates and is in line with our supposition that each of the processes we have considered so far are not fundamentally limiting the maximum growth rate.
In contrast, when we apply such an analysis to cytosolic proteins (GO term: 0005829), we observe a clear change in the proteomic composition ( Figures 4D and and4E). 4E ). Particularly, with increasing growth rates there is a substantial increase in the relative protein mass associated with “information storage and processing.” This category includes proteins such as DNA polymerase, RNA polymerase, and ribosomes that are associated with the processes of the central dogma, whose increase is predominantly at the expense of “metabolic” proteins as shown in Figure 4E . The notable anticorrelation provides a more extensive characterization of a trend that is consistent with previous reports ( Scott et al., 2010 ; Hui et al., 2015 ; Zhu and Dai, 2019 ). In the next section, we therefore turn our attention to the processes of the central dogma.
Processes of the central dogma
Up to this point, we have considered a variety of transport and biosynthetic processes that are critical to acquiring and generating new cell mass and primarily seated at the cell membrane. We now turn our focus to some of the most important processes, which must be undertaken irrespective of the growth conditions—those of the central dogma. Specifically, we explore the abundance requirements of DNA polymerase, RNA polymerase, and ribosomes, with the latter two expected to have important consequences on the rate of accumulation in mRNA and protein abundances over the course of a cell cycle ( Lin and Amir, 2018 ).
To successfully divide and produce viable progeny, the DNA must be faithfully replicated and segregated into each nascent cell. In rapidly growing cultures, bacteria like E. coli can initiate as many as 10–12 replication forks at a given time ( Bremer and Dennis, 2008 ; Si et al., 2017 ), suggesting only ≈10 DNA polymerases are needed. However, as shown in Figure 5A , DNA polymerase III is nearly an order of magnitude more abundant while still maintaining a predicted growth rate dependence. This discrepancy can be understood by considering its binding constant to DNA. In vitro characterization has quantified the K D of DNA polymerase III holoenzyme to single-stranded and double-stranded DNA to be 50 and 200 nM, respectively ( Ason et al., 2000 ) Figure 5B (discussed further, along with the synthesis of dNTP building blocks in supplemental information “additional process of the central dogma”).

(A) The minimum number of DNA polymerase holoenzyme complexes needed to facilitate replication of the genome. Points correspond to the total number of DNA polymerase III holoenzyme complexes ([DnaE] 3 [DnaQ] 3 [HolE] 3 [DnaX] 5 [HolB] [HolA][DnaN] 4 [HolC] 4 [HolD] 4 ) per cell.
(B) The effective concentration of DNA polymerase III holoenzyme (see supplemental information section “estimation of cell size and surface area” for calculation of cell size). Shaded region corresponds to the range of K D values measured by Ason et al. (2000) , from 50 to 200 nM.
(C) The number of RNA polymerase core enzymes, with measurements corresponding to the average number given a subunit stoichiometry of [RpoA] 2 [R-poC][RpoB].
(D) The abundance of σ 70 as a function of growth rate along with the same prediction from (C).
(E) Number of ribosomes required to synthesize 10 9 peptide bonds with an elongation rate of 15 peptide bonds per second.
(F) Number of tRNA synthetases that will supply the required amino acid demand. The sum of all tRNA synthetases copy numbers are plotted ([ArgS], [CysS], [GlnS], [GltX], [IleS], [LeuS], [ValS], [AlaS] 2 , [AsnS] 2 , [AspS] 2 , [TyrS] 2 , [TrpS] 2 , [ThrS] 2 , [SerS] 2 , [ProS] 2 , [PheS] 2 [PheT] 2 , [MetG] 2 , [lysS] 2 , [HisS] 2 , [GlyS] 2 [GlyQ] 2 ). Dashed black lines indicate order of magnitude estimate needed at a growth rate of ≈0.5 per h (light-brown point), while the gray line accounts for the growth rate dependence changes in cell size and doubling time. Dashed region of gray line represents growth rates with a doubling time ≥ 3 h where protein maintenance costs may be important but are not considered.
We now turn our attention to the transcription of DNA to form RNA. Here, we focus on the synthesis of rRNA, which make up the majority of RNA in the cell, and discuss the synthesis of mRNA and tRNA further in the supplemental information “additional process of the central dogma.” rRNA serves as the catalytic and structural component of the ribosome, comprising approximately 2/3 of the total ribosomal mass, and is decorated with ≈50 ribosomal proteins. Each ribosome contains three rRNA molecules of lengths 120, 1,542, and 2,904 nucleotides (BNID: 108093), meaning each ribosome contains ≈4,500 nucleotides overall. In vivo measurements of the kinetics of rRNA transcription have revealed that RNA polymerases are loaded onto the promoter of an rRNA gene at a rate of ≈1 per s (BNID: 111997, 102362). If RNA polymerases are constantly loaded at this rate, then we can assume that ≈1 functional rRNA unit is synthesized per second per rRNA operon. At a growth rate of ≈0.5 h −1 , the average cell has ≈1 copy of its chromosome and therefore approximately ≈7 copies of the rRNA operons, producing ≈7 rRNA units per second. With a 5,000-second division time, this means the cell is able to generate around 3 × 10 4 functional rRNA units, comparable within an order of magnitude to the number of ribosomes per cell.
How many RNA polymerases are then needed to constantly transcribe the required rRNA? If one polymerase is loaded once every second on average (BNID: 111997), and the transcription rate is ≈40 nucleotides per second (BNID: 101094), then the typical spacing between polymerases will be ≈40 nucleotides. With a total length of ≈4,500 nucleotides per operon and 7 operons per cell, the number of RNA polymerases transcribing rRNA at any given time is then ≈1,000 per cell. We also find that cells require on the order of another ≈400 RNAP for the synthesis of mRNA and tRNA, predicting a total of ≈1,500 RNAP to satisfy its transcriptional demands. As is revealed in Figure 5C , this estimate is about an order of magnitude below the observed number of RNA polymerase complexes per cell (≈5,000–7,000). Consistent with this discrepancy, roughly 80% of RNAP is reported to be transcriptionally inactive and a large majority of this fraction is non-specifically bound to DNA and in search for promoters from which to start transcription ( Klumpp and Hwa, 2008 ; Patrick et al., 2015 ). In Figure 5D , we find that the predicted RNA polymerase copy number indeed is more comparable with the abundance of σ−70 (RpoD), the primary workhorse sigma factor for transcription in E. coli . We can conclude that the observed RNA polymerase abundances are generally sufficient for what appears needed for growth.
We conclude our dialog between back-of-the-envelope estimates and comparison with the proteomic data by examining the final process in the central dogma—translation. We begin with an estimate of the number of ribosomes needed to double the cellular proteome. While the rate at which ribosomes translate is well known to depend on the growth rate ( Dai et al. [2018 ], a phenomenon we consider later in this work) we begin by making the approximation that translation occurs at a modest rate of ≈15 amino acids per s per ribosome (BNID: 100233). Using this approximation and our previous estimate of 10 9 peptide bonds per cell at a growth rate of 0.5 h −1 , we can easily arrive at an estimate of ≈10 4 ribosomes needed per cell to replicate the entire protein mass, which proves comparable with the experimental observations ( Figure 5E ). While the ribosome is responsible for the formation of peptide bonds, we do not diminish the importance of charging tRNAs with their appropriate amino acid, a process with occurs with remarkable fidelity. In Figure 5F , we show our estimate for the required number of tRNA synthetases, which shows similar accord with the experimental data and is discussed further in the supplemental information “estimates across fundamental biological processes.”
Having completed our circuit through key processes of cellular growth outlined in Figure 1B , we can now take stock of our understanding of the observed growth rate dependence and abundances of various protein complexes. We note that, broadly speaking, these simple estimates have been reasonably successful in quantitatively describing the observations in the proteomic data. Importantly, this agreement suggests that the proteome of E. coli is predominantly tuned in composition and absolute abundance to match the growth rate requirements without any one process representing a singular bottleneck or rate-limiting step in division.
In our effort to identify key limitations on growth, there are two notable observations worthy of additional emphasis here. The first is a recurring theme throughout the estimates investigated here, which is that any inherent biochemical rate limitation can be overcome by expressing more proteins. We can view this as a parallelization of each biosynthesis task, which helps explain why bacteria tend to increase their protein content and cell size as growth rate increases ( Ojkic et al., 2019 ). The second, and ultimately the most significant in defining the cellular growth rate, is that the synthesis of ribosomal proteins presents a special case where parallelization is not possible and thereby imposes a limit on the fastest possible growth rate. Each ribosome has ≈7,500 amino acids across all of its protein components which must be strung together as peptide bonds through the action of another ribosome. Once again using a modest elongation rate of ≈15 amino acids per s, we arrive at an estimate of ≈500 s or ≈7 min to replicate a single ribosome. This limit, as remarked upon by others ( Dill et al., 2011 ; Reuveni et al., 2017 ; Kostinski and Reuveni, 2020 ), serves as a hard theoretical boundary for how quickly a bacterium like E. coli can replicate.
Maximum growth rate is determined by the rate of ribosomal synthesis
In the closing sections of this work, we return to the motivating questions posed in the introduction—what biological processes are the primary determinants for how quickly bacterial cells can grow and reproduce, and why do cells modulate the absolute numbers and relative ratios of their molecular constituents in response to changes in growth rate or nutrient availability? In the next two sections we begin by considering the consequences of the 7-minute limit set by ribosomal synthesis in the context of the available proteomic data and measured growth rates. In the final section, we consider how total protein abundance, ribosomal content, and chromosomal replication are intertwined in their control over the cellular growth rate. To do so, we take a more careful view, increasing the sophistication of our analysis by exchanging our order-of-magnitude estimates for a minimal mathematical model of growth rate control. This is defined by parameters with tangible connections to the biological processes underlying cellular growth and protein synthesis. We will draw on the analysis thus far but also draw on other theoretical and experimental work in order to develop a more complete synthesis around these questions.
The 7-min speed limit assumes all proteins in the cell are ribosomal. In order to connect this to the experimental data (and physiological reality more broadly), first, we need to relax this assumption and determine a translation-limited growth rate. Here, we will assume that the cell is composed of N pep peptide bonds and R ribosomes, whose precise values will depend on the growth rate λ. The protein subunits of each ribosomal protein sum to a total of ≈7,500 amino acids as noted earlier, which we denote by L R . With an average mass of an amino acid of m AA ≈ 110 Da (BNID: 104877), the total ribosomal mass fraction Φ R is given by
For exponentially growing cells ( Godin et al., 2010 ), the rate of cellular growth λ will be related to the rate of protein synthesis via
where r t is the translation rate. Here, we have introduced a multiplicative factor f a , which represents the fraction of the ribosomes that are actively translating. This term allows us to account for immature or non-functional ribosomes or active sequestration of ribosomes through the action of the secondary messenger alarmone (p)ppGpp in poorer nutrient conditions ( Hauryliuk et al., 2015 ).
Combining Equations 1 and 2 results in an expression for a translation-limited growth rate, which is given by
This result, derived in a similar manner by others ( Dennis et al., 2004 ; Klumpp et al., 2013 ), reflects mass-balance under steady-state growth and has long provided a rationalization of the apparent linear increase in E. coli ’s ribosomal content as a function of growth rate ( Maalœ, 1979 ; Dennis et al., 2004 ; Scott et al., 2010 ; Dai et al., 2016 ). Figure 6A shows this growth rate plotted as a function of the ribosomal mass fraction (black line). In the regime where all ribosomes are active ( f a = 1) and the entire proteome is composed of ribosomal proteins Φ R = 1), indeed, this line intercepts the maximum theoretical growth rate of r t / L R , and a ≈7-min doubling time for E. coli .

(A) Translation-limited growth rate as a function of the actively translating ribosomal fraction. The actively translating ribosomal fraction is calculated using the estimated values of f a from Dai et al. (2016) (shown in inset; see supplemental information “calculation of active ribosomal fraction” for additional detail). Shaded region defines boundary due to constraint set on growth rate by Equation 3 . The solid line is calculated for an elongation rate of 17 peptide bonds per s. Gray data points show additional measurements based on measurements of cellular RNA to protein ratio, with Φ R ≈ the cellular RNA to protein ratio divided by 2.1 ( Dai et al., 2016 ) and come from Forchhammer and Lindahl (1971) ; Bremer and Dennis (2008) ; Scott et al. (2010) ; Dai et al. (2016) ; Si et al. (2017) .
(B) Maximum number of rRNA units that can be synthesized as a function of growth rate. Solid curve corresponding to the rRNA copy number is calculated by multiplying the number of rRNA operons by the estimated number of 〈 # ori 〉 at each growth rate. The quantity 〈 # ori 〉 was calculated using Equation 4 and the measurements from Si et al. (2017) . The dashed line shows the maximal number of functional rRNA units produced from a single chromosomal initiation per cell cycle. Shaded region defines boundary due to maximal rRNA synthesis.
Connecting Equation 3 to the proteomic data, however, requires knowledge of f a at each growth rate as proteomic measurements only provide a measure of Φ R . While commonly considered constant with growth rate ( Young and Bremer, 1976 ; Klumpp et al., 2013 ; Bosdriesz et al., 2015 ; Kostinski and Reuveni, 2020 ), Dai et al. (2016) recently inferred f a as a function of the growth rate ( Figure 6A , inset), revealing that while f a is close to 1 at growth rates above 0.75 h −1 , it drops dramatically at slower growth rates. Using these data, we inferred the approximate active fraction (see supplemental information section “calculation of active ribosomal fraction”) at each growth rate and used this to compute Φ R × f a ( Figure 6A , colored points). Importantly, these data skirt the translation-limited growth rate determined using Equation 3 , where we have taken r t to be the maximal elongation rate of 17 amino acids per s measured by Dai et al. (2016) . There is a notable discrepancy between the data collected in Schmidt et al. (2016) , Li et al. (2014) and that collected from Valgepea et al. (2013) , and Peebo et al. (2015) . When compared with other measurements (non-proteomic based) of the active ribosome mass fraction based on measurements of total RNA to total protein mass ratios ( Figure 6A , gray points), the data from Valgepea et al. (2013) and Peebo et al. (2015) are notably different, suggesting there may be a systematic bias in these two sets of measurements.
The absolute ribosome copy number is limited by rRNA synthesis under rapid growth
Even under idealized experimental conditions, however, E. coli rarely exhibits growth rates above 2 h −1 ( Bremer and Dennis, 2008 ), which is still well below the synthesis rate of a single ribosome, and below the maximum growth rates reported for several other bacteria ( Roller et al., 2016 ). While we have considered potential limits imposed by translation of ribosomal “proteins,” we must also consider potential limiting regimes specific to the synthesis of rRNA. Due to multiple initiations of chromosomal replication per cell doubling, the effective number of rRNA operons increases with growth rate and will do so in proportion to the average number of chromosomal origins per cell, 〈 # ori 〉 . This later parameter is set by how often replication must be initiated in order to keep up with the cell doubling time τ, whose time may be shorter than the cell cycle time τ c y c (referring to the time from replication initiation to cell division) ( Dennis et al., 2004 ; Ho and Amir, 2015 ). This is quantified by
where the doubling time τ is related to the growth rate by τ = log(2)/λ. As the rRNA operons are predominantly located close to the origin of replication (BNID: 100352), we make the simplifying assumption that that the number of rRNA operons will be directly proportional to 〈 # ori 〉 . We used the experimental measurements of τ cyc and τ ( Figure S10 ) to calculate 〈 # ori 〉 with Equation 4 as a function of growth rate. For growth rates above about 0.5 h −1 , t cyc is approximately constant at about 70 min, implying an exponential increase in 〈 # ori 〉 and the rRNA operon copy number for growth rates above 0.5 h −1 .
Returning to our rule-of-thumb that one functional rRNA unit is produced per second per transcribing operon, we can estimate the maximum number of ribosomes that could be made as a function of growth rate ( Figure 6B , blue curve). This provides a useful reference alongside the proteomic measurements, particularly in the regime of fast growth. For growth rates above about 1 h −1 in particular, we find that cells will need to transcribe rRNA near their maximal rate. The convergence between the maximum rRNA production and measured ribosome copy number shows that rRNA synthesis begins to present a physical bottleneck at the fastest growth rates in E. coli due to the still-limited copies of rRNA genes. While the rapid pace of rRNA synthesis is well documented ( Neidhardt et al., 1991 ; Bremer and Dennis, 2008 ), this analysis helps highlight the difficulty in increasing absolute ribosome abundance further, given the apparent scaling in measured ribosome copy numbers and still-limited number of rRNA gene copies on the chromosome.
Simultaneous tuning of total protein mass and ribosome copy number increase growth rate under nutrient-limited growth
While the preceding two sections highlight a dominant role for ribosomes in setting the achievable growth rate, our analysis thus far has also shown how the proteomic content and cell size will need to change in response to variable growth conditions and growth rate. Here, we now return to the second question posed in the introduction—why do cells modulate the absolute numbers and relative ratios of their molecular constituents in response to changes in growth rate or nutrient availability? In this final section, we consider how the nutrient-dependent changes in total protein content per cell and proteomic composition influence the achievable growth rate.
The variable demand on resources as a function of growth condition places an optimization challenge for the cell—how are the translational demands of the entire proteome met without investing resources in the production of excess ribosomes? This question, more frequently presented as a question of optimal resource allocation, has been the target of an extensive dialog between experiment and theory over the past decade. In now seminal works, Scott et al. (2010 , 2014 ) present an elegant treatment of resource allocation through partitioning of the proteome into sectors—one of which being ribosome-associated proteins whose relative size ultimately defines the total cellular growth rate. In more recent years, this view has been more thoroughly dissected experimentally ( Klumpp et al., 2013 ; Basan et al., 2015 ; Dai et al., 2016 , 2018 ; Erickson et al., 2017 ). However, the quantitative description of these observations is often couched in terms of phenomenological constants and effective parameters with the key observable features of expression often computed in relative, rather than absolute, abundances. Furthermore, these approaches often exclude or integrate away effects of cell size and chromosome content, which we have found through our estimates to have important connections to the observed cellular growth rate and proteomic content.
The specific mechanisms of growth rate control under nutrient limitation that lead to the observed scaling in cell size in E. coli and other bacteria, however, has remained unclear and continues to be intensely investigated ( Si et al., 2017 ; Harris and Theriot, 2018 ; Ojkic et al., 2019 ). From our estimates, we see that the smaller, more economical cell sizes (i.e., smaller proteomic mass) observed in poorer nutrient conditions is consistent with a view that cells are also minimizing total protein abundance to better match their specific growth rate requirements. Under translation-limited growth conditions (λ ≈ 0.7 h −1 ), cells can then only increase their growth rate by increasing ribosome content. The simple addition of more ribosomes is likely constrained by macromolecular crowding ( Delarue et al., 2018 ; Soler-Bistué et al., 2020 ), and we find that the cellular ribosome concentration increases 3–4-fold across growth conditions, compared with a roughly 20-fold change in absolute ribosome abundance ( Figure 7A ). Importantly, the major deviations in protein abundance with growth rate can be solely attributed to the required bias in ribosomal protein synthesis. To see this, we have calculated the position-dependent protein expression across the chromosome by a running Gaussian average of protein copy number (20 kbp SD averaging window) based on each gene’s transcriptional start site ( Figure 7B ). Since E. coli cells add a constant volume per origin of replication ( Si et al., 2017 ), we have median-subtracted the measured protein copy numbers and colored each growth condition from the proteomic data according to 〈 # ori 〉 .

(A) Plot of the ribosome copy number estimated from the proteomic data against the estimated cell size (see supplemental information “estimation of cell size and surface area” for details on the calculation of cell size).
(B) A running Gaussian average (20 kbp SD) of protein copy number is calculated for each growth condition considered by ( Schmidt et al., 2016 ) based on each gene’s transcriptional start site. Since total protein abundance increases with growth rate, protein copy numbers are median subtracted to allow comparison between growth conditions. 〈 # ori 〉 are estimated using data from Si et al. (2017) (see supplemental information “estimation of 〈 # ori 〉 for additional details).
(C) We consider a unit volume of cellular material composed of amino acids (colored spheres) provided at a supply rate r AA . These amino acids are polymerized by a pool of ribosomes (brown blobs) at a rate r t × R × f a , where r t is the elongation rate, R is the ribosome copy number in the unit volume, and f a is the fraction of those ribosomes actively translating. In addition to determining total protein synthesis rate, the nutrient status is gauged by any accumulation of de-acylated tRNAs and synthesis of the secondary messenger (p)ppGpp, which ultimately determine 〈 # ori 〉 , cell size, and proteomic composition.
(D) The observed elongation rate is plotted as a function of the number of ribosomes. The three points correspond to three regimes of ribosome copy numbers and are shown schematically on the left-hand side. The region of the curve shown as dashed lines represents a non-physical copy number but is shown for illustrative purposes. This curve was generated using an amino acid supply rate of 5 × 10 6 AA / s, a maximal elongation rate of 17.1 AA / s, f a = 1, and a unit cell volume of 1 fL. See supplemental information “derivation of minimal model for nutrient-mediated growth rate control” for additional model details.
(E) The cellular growth rate is plotted as a function of total cellular ribosome copy number for different cellular amino acid supply rates, with blue and green curves corresponding to low and high supply rates, respectively. As the ribosome copy number is increased, so too is the cell size and total protein abundance N pep .
To more quantitatively consider the dependencies between cell size, ribosome abundance, and growth rate, we lastly consider a minimal model of growth rate control. For bacteria like E. coli , cell size will vary approximately in proportion to the total protein mass Kubitschek et al., 1984 ; Basan et al. (2015) , and we will again consider a cell containing a total number of peptide bonds N pep and R ribosomes. Following from Equation 2 , the rate of total protein synthesis and cellular growth rate λ will depend on the ribosomal elongation rate r t that each ribosome proceeds at. The elongation rate r t will ultimately depend on how quickly ribosomes can match codons with an amino-acyl tRNA, along with the subsequent steps of peptide bond formation and translocation ( Figure 7C ). This ultimately depends on the cellular concentration of amino acids, which we treat as a single effective species, [ AA ] eff . Here, we follow a similar strategy to that employed by others ( Klumpp and Hwa, 2014 ; Dai et al., 2016 ) and apply a coarse-grained description of translation that allows for a reversible binding of the amino-acyl tRNAs followed by an irreversible addition of the amino acid into the peptide chain (further described in the supplemental information section “derivation of minimal model for nutrient-mediated growth rate control”). More extensive analyses of amino acid supply and consumption have been considered elsewhere ( Elf and Ehrenberg (2005) ; Bosdriesz et al. (2015) ; Hu et al., 2020 ).
Having found that cells do not appear limited in their ability to synthesize and charge tRNA, we determine the rate of peptide elongation r t and achievable growth rate as simply depending on the supply of amino acids (and, therefore, also amino-acyl tRNAs), through a parameter r AA in units of AA per second, and the rate of amino acid consumption by protein synthesis ( r t × R × f a ). The parameter r AA will depend on the specific nutrient conditions, as well as the fraction of the proteome devoted to the supply of amino-acyl tRNAs, and we will consider its value a reflection of the nutrient quality. In Figure 7D , we illustrate how the elongation rate will depend on the ribosomal copy number for constant r AA , and further described in the supplemental information section “derivation of minimal model for nutrient-mediated growth rate control.”
To relate elongation rate to growth rate, we constrain the set of parameters based on our available proteomic measurements; namely, we restrict the values of R , N pep , and cell size V to those associated with the amalgamated proteomic data (described in the supplemental information section “estimation of total protein content per cell”). We then consider how changes in the nutrient conditions, through the parameter r AA , influence the maximum growth rate as determined by Equation 3 . Under this scenario, R and V become interdependent parameters, while in supplemental information section “Relaxing the interdependence of R and V” and Figure S12 , we discuss the resulting growth rate when R and V are treated as independent parameters and may be more relevant to physiological perturbations such as protein overexpression ( Basan et al., 2015 ). Figure 7E shows how the growth rate depends on the rate of amino acid supply r AA as a function of the cellular ribosome copy number and the cell volume. A feature immediately apparent from the plot is the presence of a maximal growth rate that increases with increasing r AA . Importantly, there is a particular combination of values for R , N pep , and cell size V where growth rate λ is maximized. This shows that increasing the ribosomal concentration beyond the cell’s metabolic capacity will have the adverse consequence of depleting the supply of amino acids and lead to a concomitant decrease in the elongation rate r t ( Figure 7D ) and growth rate. This helps us understand that while it is important for cells to increase their ribosomal content and total protein content (and hence, also cell size) in order to increase growth rate, cells will better maximize their achievable growth rate by tuning these parameters according to nutrient conditions, since this is ultimately what allows cells to reach the peak for each curve shown in Figure 7E .
Also of note is the growth rate trends observed at low values of r AA (purple and blue lines in Figure 7E ), representative of growth in nutrient-poor media. This regime is of particular interest due to deviations from expectations of ribosomal and cell size scaling that follow from the bacterial growth law ( Dai et al., 2016 ; Amir, 2017 ; Zheng et al., 2020 ). Here, there no longer exists a peak in the maximum growth rate, at least within the range of physiologically relevant ribosome copy numbers considered. This is the regime, associated with slower growth rates, where cells limit their pool of actively translating ribosomes by decreasing f a ( Figure 6A , inset). By reducing the fraction of actively translating ribosomes, cells instead appear to be prioritizing their pool of available amino acids [ AA ] eff in order to increase their translation elongation rate. Consistent with this hypothesis and our model, while inhibition of translation with chloramphenicol further reduces the fraction of actively translating ribosomes f a , it results in an increase in the elongation rate that has been observed experimentally ( Dai et al., 2016 ) ( Figure S13 and further discussion in relation to other models of translation elongation in supplemental information section “derivation of minimal model for nutrient-mediated growth rate control”). We can then view this slower growth regime (λ 0.7 h −1 ) as one that no longer prioritizes translation, with cells more limited by their amino acid supply ( Forchhammer and Lindahl, 1971 ; Pedersen, 1984 ; Elf and Ehrenberg, 2005 ). Indeed, since ribosomes are not fully engaged in translation, cells are no longer maximizing growth rate according to their potential translation-limited rate. There are likely physiological benefits to this for a bacterium in an uncertain nutrient environment, with an excess pool of ribosomes potentially enabling more rapid recovery upon improvements in nutrient conditions ( Bosdriesz et al., 2015 ; Bergkessel et al., 2016 ).
Continued experimental and technological improvements have led to a treasure trove of quantitative biological data ( Hui et al., 2015 ; Schmidt et al., 2016 ; Si et al., 2017 ; Gallagher et al., 2020 ; Peebo et al., 2015 ; Valgepea et al., 2013 ), and an ever advancing molecular view and mechanistic understanding of the constituents that support bacterial growth ( Taheri-Araghi et al., 2015 ; Morgenstein et al., 2015 ; Si et al., 2019 ; Karr et al., 2012 ; Kostinski and Reuveni, 2020 ; Macklin et al., 2020 ). In this work, we have compiled and curated what we believe to be the state-of-the-art knowledge on proteomic copy number across a broad range of growth conditions in E. coli . Beyond compilation, we have taken a detailed approach in ensuring that the absolute protein abundances reported are directly comparable across growth rates and datasets, allowing us to make assertions about the physiology of E. coli rather than chalking up discrepancies from our simple estimates to experimental noise and systematic errors. For example, while there was notable disagreement in the measurements from different studies in some cases (e.g., cell envelope biosynthesis in Figure 3E , or DNA synthesis in Figure 5A ), our predictions were consistent with the trends observed in the data on the whole. We have made this data accessible through a GitHub repository, and an interactive figure that allows exploration of specific protein and protein complex copy numbers.
Through a series of order-of-magnitude estimates that traverse key steps in the bacterial cell cycle, this proteomic data have been a resource to guide our understanding of two key questions: what biological processes limit the absolute speed limit of bacterial growth, and how do cells alter their molecular constituents as a function of changes in growth rate or nutrient availability? While not exhaustive, our series of estimates provide insight on the scales of macromolecular complex abundance across four classes of cellular processes—the transport of nutrients, the production of energy, the synthesis of the membrane and cell wall, and the numerous steps of the central dogma.
In general, the copy numbers of the complexes involved in these processes were in reasonable agreement with our order-of-magnitude estimates. Since many of these estimates represent soft lower-bound quantities, this suggests that cells do not express proteins grossly in excess of what is needed for a particular growth rate. Rather, cells maintain protein abundances that while nearly rate limiting, they are nevertheless sufficient for the require biosynthetic capacity given available nutrient conditions and the observed doubling time. Several exceptions, however, also highlight the dichotomy between a proteome that appears to “optimize” expression according to growth rate and one that must be able to quickly adapt to environments of different nutritional quality. Take, for example, the expression of carbon transporters. Shown in Figure 3A , we find that cells always express a similar number of carbohydrate transporters irrespective of growth condition. Normalizing transporter abundance to total cellular mass, this would result in a decrease in mass fraction associated with carbon uptake for increasing growth rates and improved nutrient conditions, consistent with previous work ( You et al., 2013 ; Hui et al., 2015 ). At the same time, it is interesting to note that many of the alternative carbon transporters are still expressed in low but non-zero numbers (≈10–100 copies per cell) across growth conditions. This may relate to the regulatory configuration for many of these operons, which require the presence of a metabolite signal in order for alternative carbon utilization operons to be induced ( Monod, 1949 ; Laxhuber et al., 2020 ). Furthermore, upon induction, these transporters are expressed and present in abundances in close agreement with a simple estimate ( Figure S1 ).
Of the processes illustrated in Figure 1B , we arrive at a perspective where the different processes of bacterial growth all must be carefully coordinated to support rapid growth, but where ribosomal abundance sets a firm upper limit on the achievable growth rate. This is in some sense unsurprising given the long-held observation that E. coli and many other organisms vary their ribosomal abundance as a function of growth conditions and growth rate ( Scott et al., 2010 ; Metzl-Raz et al., 2017 ). However, through our dialog with the proteomic data, two additional key points emerge. The first relates to our question of what process sets the absolute speed limit of bacterial growth. While a cell can parallelize many of its processes simply by increasing the abundance of specific proteins or firing multiple rounds of DNA replication, this is not so for synthesis of ribosomes as has been noted by others ( Dill et al., 2011 ; Reuveni et al., 2017 ; Kostinski and Reuveni, 2020 ). The translation time for each ribosome (≈7 min) places an inherent limit on the growth rate that can only be surpassed if the cell were to increase their polypeptide elongation rate, or if they could reduce the total protein and rRNA mass of the ribosome. The second point relates to the long-observed correlations between growth rate and cell size ( Schaechter et al., 1958 ; Si et al., 2017 ), and between growth rate and ribosomal mass fraction. While both trends have sparked tremendous curiosity and driven substantial amounts of research in their own regards, these relationships are themselves intertwined. In particular, E. coli ’s protein content is reasonably well-tuned according to their growth rate, there is a predominant need for cells to increase their absolute number of ribosomes under conditions of rapid growth that require cells to also grow in size.
On the question of how bacteria actually achieve the simultaneously tuning of their ribosomal abundance, total proteomic content, and the extent of replication, much work points to the role of secondary messengers like (p)ppGpp ( Cashel and Gallant, 1969 ; Nomura et al., 1984 ). While most commonly associated with a dynamic global response to changes in nutrient conditions through the stringent response, (p)ppGpp increasingly appears to play a role in both the control of the active ribosomal fraction and cell size homeostasis under steady-state nutrient-limited growth ( Dai et al., 2016 ; Zhu and Dai, 2019 ; Büke et al., 2020 ; Vadia et al., 2017 ; Parker et al., 2020 ). In E. coli , an accumulation of de-acylated tRNAs at the ribosome’s A-site leads to a strong increase in (p)ppGpp synthesis activity by the enzyme RelA ( Hauryliuk et al., 2015 ), providing a direct way to sense and adjust ribosomal content according to the level of charged tRNAs, as was shown in the work of Bosdriesz et al. (2015) . (p)ppGpp and co-regulator DksA also strongly repress rRNA synthesis and ribosomal protein gene expression ( Paul et al., 2004 ; Jin et al., 2012 ), and there is more recent evidence that (p)ppGpp acts to inhibit the initiation of DNA replication and DNA supercoiling near the origin of replication ( Kraemer et al., 2019 ; Fernández-Coll et al., 2020 ). E. coli cells are well documented to add a constant volume per origin of replication that is robust to a remarkable array of cellular perturbations ( Si et al., 2017 ) and many bacteria have been found to positively vary nucleoid size with cell size ( Campos et al., 2018 ; Gray et al., 2019 ). It will be interesting to further consider how control by (p)ppGpp aids in tuning 〈 # ori 〉 and cell size to better match available nutrients conditions.
While the generation of new ribosomes plays a dominant role in growth rate control, there exist other physical limits to the function of cellular processes. One of the key motivations for considering energy production was the physical constraints on total volume and surface area as cells vary their size ( Harris and Theriot, 2018 ; Ojkic et al., 2019 ). As E. coli get larger at faster growth rates, an additional constraint begins to arise in energy production and nutrient uptake due to the relative decrease in total surface area, where ATP is predominantly produced ( Szenk et al., 2017 ). Specifically, the cell interior requires an amount of energy that scales cubically with cell size, but the available surface area only grows quadratically ( Figure 4A ). While this threshold does not appear to be met for E. coli cells growing at 2 h −1 or less, it highlights an additional constraint on growth given the apparent need to increase cell size in order to grow faster. This limit is relevant even to eukaryotic organisms, whose mitochondria exhibit convoluted membrane structures that nevertheless remain bacteria-sized organelles ( Guo et al., 2018 ). In the context of bacterial growth and energy production more generally, we have mainly limited our analysis to the aerobic growth conditions associated with the proteomic data, and further consideration will be needed for anaerobic growth.
This work is by no means meant to be a complete dissection of bacterial growth rate control, and there are many aspects of the bacterial proteome and growth that we neglected to consider. For example, other recent work ( Liebermeister et al., 2014 ; Hui et al., 2015 ; Schmidt et al., 2016 ) has explored how the proteome is structured. In the work of Hui et al. (2015) , the authors coarse-grained the proteome into six discrete categories being related to either translation, catabolism, anabolism, and others related to signaling and core metabolism. The relative mass fraction of the proteome occupied by each sector could be modulated by external application of drugs or simply by changing the nutritional content of the medium. While we have explored how the quantities of individual complexes are related to cell growth, we acknowledge that higher-order interactions between groups of complexes or metabolic networks at a systems level may reveal additional insights into how these growth rate dependences are achieved. This is exemplified by recent work highlighting a role for “P-sector” divisor proteins in setting cell size ( Si et al., 2019 ; Panlilio et al., 2020 ; Serbanescu et al., 2020 ), where quantitative treatment of the allocation of cellular resources toward ribosomal and division protein synthesis can help account for morphological changes under nutrient shifts or translational perturbations. Furthermore, while we anticipate the conclusions summarized here are applicable to a wide collection of bacteria with similar lifestyles as E. coli , other bacteria and archaea may have evolved other strategies that were not considered. Further experiments with the level of rigor now possible in E. coli will need to be performed in a variety of microbial organisms to learn more about how regulation of proteomic composition and growth rate control has evolved over the past 3.5 billion years.
STAR★METHODS
Detailed methods are provided in the online version of this paper and include the following:
RESOURCE AVAILABILITY
Lead contact.
Further information and requests for resources should be directed to and will be fulfilled by the Lead Contact, Rob Phillips ( ude.hcetlac.cobp@spillihp ).
Materials availability
This study did not generate new unique reagents.
Data and code availability
Method details.
All data used in this work was collected from primary published literature (see key resources table ), though great care was taken to standardize the measurements such that they are directly comparable, despite being taken in slightly different conditions using different methodologies and by different research groups.
The protein abundance data were cleaned and standardized as described in the supplemental information and collated into a singular long-form tidy.csv file. Annotation of molecular complexes was performed using Python scripts and the PathwayTools utilities available via BioCyc ( Karp et al., 2019 ). The combined data of annotated complexes and protein-level abundances is provided as Data S1 . The abundances of each individual complex (rather than abundances of individual proteins) is available as Data S2 .
Analysis code and figure generation
All code used in the data cleaning, standardization, and figure generation is made publicly available as a GitHub repository available via https://github.com/rpgroup-pboc/growth_limits and the [ https://doi.org/10.5281/zenodo.4091457 ]. Code used in the processing, data cleaning, and annotation is located in the code/processing subdirectory. All code used for figure generation is located in the code/figures subdirectory.
Interactive figures
Associated with this work are two interactive figures that allow for deeper exploration of the data and the the minimal mathematical model. These figures are hosted at the paper website https://rpgroup.caltech.edu/growth_limits and their code is available on the associated GitHub repository.
- Key biological processes are considered as potential growth-rate-limiting steps
- A near-comprehensive dataset of the E. coli proteome across growth rates is presented
- Order-of-magnitude estimates suggest little inefficiency across key processes
- Translation emerges as a rate-governing process and we explore constraints on growth rate
Supplementary Material
Supplemental material, peer review record, acknowledgments.
We thank Matthias Heinemann, Alexander Schmidt, and Gene-Wei Li for additional input regarding their data. We also thank all members of the Phillips, Theriot, Kondev, Garcia labs, as well as Ron Milo and Terry Hwa for useful discussions. We thank Suzannah M. Beeler, Jonas Cremer, Avi Flamholz, Soichi Hirokawa, and Manuel Razo-Mejia for reading and providing comments on drafts of this manuscript. R.P. is supported by La Fondation Pierre-Gilles de Gennes, the Rosen Center at Caltech, and the NIH 1R35 GM118043 (MIRA). J.A.T. is supported by the Howard Hughes Medical Institute, and NIH grant R37-AI036929. N.M.B is a HHMI fellow of the Jane Coffin Childs Memorial Fund. H.G.G. is supported by the Burroughs Wellcome Fund Career Award at the Scientific Interface, the Sloan Research Foundation, the Human Frontiers Science Program, the Searle Scholars Program, the Shurl & Kay Curci Foundation, the Hellman Foundation, the NIH Director’s New Innovator Award (DP2 OD024541-01), and an NSF CAREER award (1652236). D.S.F. is supported by an NSF award (PHY-1607606) and the NIH (NIH R01-AI13699201).
J.A.T. is chief scientific advisor at the Allen Institute for Cell Science (Seattle, WA, 98109).
DECLARATION OF INTERESTS
The authors otherwise declare no competing interests.
SUPPLEMENTAL INFORMATION
Supplemental information can be found online at https://doi.org/10.1016/j.cels.2021.06.002 .
SUPPORTING CITATIONS
The following references appear in the supplemental information: Abelson et al., 1974 , Baba et al., 2006 , Belliveau et al., 2018 , Booth et al., 1979 , Datsenko and Wanner, 2000 , Dong et al., 1996 , Fijalkowska et al., 2012 , Gama-Castro et al., 2016 , Ge et al., 2003 , Harris et al., 2001 , Helmstetter and Cooper, 1968 , Ireland et al., 2020 , Jensen et al., 2001 , Jun et al., 2018 , Keseler et al., 2017 , Khademi et al., 2004 , Lex et al., 2014 , Lu et al., 2003 , Pedersen et al., 1978 , Ramos and Kaback, 1977 , Rudd et al., 2016 , Sánchez-Romero et al., 2011 , Sekowska et al., 2000 , Sirko et al., 1995 , Soufi et al., 2015 , Svenningsen et al., 2017 , Taniguchi et al., 2010 , van Heeswijk et al., 2013 , Virtanen et al., 2020 , Volkmer and Heinemann, 2011 , Zhang et al., 2014a , Zhang et al., 2014b .
- Abelson HT, Johnson LF, Penman S, and Green H. (1974). Changes in RNA in relation to growth of the fibroblast: II. The lifetime of mRNA, rRNA, and tRNA in resting and growing cells . Cell 1 , 161–165. 10.1016/0092-8674(74)90107-X. [ PubMed ] [ CrossRef ] [ Google Scholar ]
- Aidelberg G, Towbin BD, Rothschild D, Dekel E, Bren A, and Alon U. (2014). Hierarchy of non-glucose sugars in Escherichia coli . BMC Syst. Biol 8 , 133. 10.1186/s12918-014-0133-z. [ PMC free article ] [ PubMed ] [ CrossRef ] [ Google Scholar ]
- Amir A. (2017). Is cell size a spandrel? eLife 6 , 18261. [ PMC free article ] [ PubMed ] [ Google Scholar ]
- Antonenko YN, Pohl P, and Denisov GA (1997). Permeation of ammonia across bilayer lipid membranes studied by ammonium ion selective microelectrodes . Biophys. J 72 , 2187–2195. [ PMC free article ] [ PubMed ] [ Google Scholar ]
- Ashburner M, Ball CA, Blake JA, Botstein D, Butler H, Cherry JM, Davis AP, Dolinski K, Dwight SS, Eppig JT, et al. (2000). Gene ontology: tool for the unification of biology . Nat. Genet 25 , 25–29. [ PMC free article ] [ PubMed ] [ Google Scholar ]
- Ason B, Bertram JG, Hingorani MM, Beechem JM, O’Donnell M, Goodman MF, and Bloom LB (2000). A model for Escherichia coli DNA polymerase III holoenzyme assembly at primer/template ends. DNA triggers A change in binding specificity of the γ complex clamp loader . J. Biol. Chem 275 , 3006–3015. 10.1074/jbc.275.4.3006. [ PubMed ] [ CrossRef ] [ Google Scholar ]
- Assentoft M, Kaptan S, Schneider HP, Deitmer JW, de Groot BL, and MacAulay N. (2016). Aquaporin 4 as a NH 3 channel . J. Biol. Chem 291 , 19184–19195. 10.1074/jbc.M116.740217. [ PMC free article ] [ PubMed ] [ CrossRef ] [ Google Scholar ]
- Baba T, Ara T, Hasegawa M, Takai Y, Okumura Y, Baba M, Datsenko KA, Tomita M, Wanner BL, and Mori H. (2006). Construction of Escherichia coli K-12 in-frame, single-gene knockout mutants: the Keio collection . Mol. Syst. Biol 2 , 2006.0008. [ PMC free article ] [ PubMed ] [ Google Scholar ]
- Barreteau H, Kovač A, Boniface A, Sova M, Gobec S, and Blanot D. (2008). Cytoplasmic steps of peptidoglycan biosynthesis . FEMS Microbiol. Rev 32 , 168–207. 10.1111/j.1574-6976.2008.00104.x. [ PubMed ] [ CrossRef ] [ Google Scholar ]
- Basan M, Zhu M, Dai X, Warren M, Sévin D, Wang YP, and Hwa T. (2015). Inflating bacterial cells by increased protein synthesis . Mol. Syst. Biol 11 , 836. [ PMC free article ] [ PubMed ] [ Google Scholar ]
- Bauer S, and Ziv E. (1976). Dense growth of aerobic bacteria in a bench-scale fermentor . Biotechnol. Bioeng 18 , 81–94. 10.1002/bit.260180107. [ PubMed ] [ CrossRef ] [ Google Scholar ]
- Belliveau NM, Barnes SL, Ireland WT, Jones DL, Sweredoski MJ, Moradian A, Hess S, Kinney JB, and Phillips R. (2018). Systematic approach for dissecting the molecular mechanisms of transcriptional regulation in bacteria . Proc. Natl. Acad. Sci. USA 115 , E4796–E4805. 10.1073/pnas.1722055115. [ PMC free article ] [ PubMed ] [ CrossRef ] [ Google Scholar ]
- Bergkessel M, Basta DW, and Newman DK (2016). The physiology of growth arrest: uniting molecular and environmental microbiology . Nat. Rev. Microbiol 14 , 549–562. [ PMC free article ] [ PubMed ] [ Google Scholar ]
- Booth IR, Mitchell WJ, and Hamilton WA (1979). Quantitative analysis of proton-linked transport systems. The lactose permease of Escherichia coli . Biochem. J 182 , 687–696. [ PMC free article ] [ PubMed ] [ Google Scholar ]
- Bosdriesz E, Molenaar D, Teusink B, and Bruggeman FJ (2015). How fast-growing bacteria robustly tune their ribosome concentration to approximate growth-rate maximization . FEBS Journal 282 , 2029–2044. [ PMC free article ] [ PubMed ] [ Google Scholar ]
- Bremer H, and Dennis PP (2008). Modulation of chemical composition and other parameters of the cell at different exponential growth rates . EcoSal Plus 3 , 1–48. [ PubMed ] [ Google Scholar ]
- Büke F, Grilli J, Lagomarsino MC, Bokinsky G, and Tans S. (2020). ppGpp is a bacterial cell size regulator . bioRxiv . 10.1101/2020.06.16.154187. [ PubMed ] [ CrossRef ] [ Google Scholar ]
- Campos M, Govers SK, Irnov I, Dobihal GS, Cornet F, and Jacobs-Wagner CJ (2018). Genomewide phenotypic analysis of growth, cell morphogenesis, and cell cycle events in Escherichia coli . Mol. Syst. Biol 14 , e7573. [ PMC free article ] [ PubMed ] [ Google Scholar ]
- Cashel M, and Gallant J. (1969). Two compounds implicated in the function of the RC gene of Escherichia coli . Nature 221 , 838–841. [ PubMed ] [ Google Scholar ]
- Catherwood AC, Lloyd AJ, Tod JA, Chauhan S, Slade SE, Walkowiak GP, Galley NF, Punekar AS, Smart K, Rea D, et al. (2020). Substrate and stereochemical control of peptidoglycan cross-linking by transpeptidation by Escherichia coli PBP1B . J. Am. Chem. Soc 142 , 5034–5048. 10.1021/jacs.9b08822. [ PubMed ] [ CrossRef ] [ Google Scholar ]
- Dai X, Zhu M, Warren M, Balakrishnan R, Okano H, Williamson JR, Fredrick K, and Hwa T. (2018). Slowdown of translational elongation in Escherichia coli under hyperosmotic stress . mBio 9 , 281. [ PMC free article ] [ PubMed ] [ Google Scholar ]
- Dai X, Zhu M, Warren M, Balakrishnan R, Patsalo V, Okano H, Williamson JR, Fredrick K, Wang YP, and Hwa T. (2016). Reduction of translating ribosomes enables Escherichia coli to maintain elongation rates during slow growth . Nat. Microbiol 2 , 16231. [ PMC free article ] [ PubMed ] [ Google Scholar ]
- Datsenko KA, and Wanner BL (2000). One-step inactivation of chromosomal genes in Escherichia coli K-12 using PCR products . Proc. Natl. Acad. Sci. USA 97 , 6640–6645. [ PMC free article ] [ PubMed ] [ Google Scholar ]
- Davidi D, Noor E, Liebermeister W, Bar-Even A, Flamholz A, Tummler K, Barenholz U, Goldenfeld M, Shlomi T, and Milo R. (2016). Global characterization of in vivo enzyme catalytic rates and their correspondence to in vitro k cat measurements . Proc. Natl. Acad. Sci. USA 113 , 3401–3406. 10.1073/pnas.1514240113. [ PMC free article ] [ PubMed ] [ CrossRef ] [ Google Scholar ]
- Delarue M, Brittingham GP, Pfeffer S, Surovtsev IV, Pinglay S, Kennedy KJ, Schaffer M, Gutierrez JI, Sang D, Poterewicz G, et al. (2018). mTORC1 controls phase separation and the biophysical properties of the cytoplasm by tuning crowding . Cell 174 , 338–349.e20. [ PMC free article ] [ PubMed ] [ Google Scholar ]
- Dennis PP, Ehrenberg M, and Bremer H. (2004). Control of rRNA synthesis in Escherichia coli: a systems biology approach . Microbiol. Mol. Biol. Rev 68 , 639–668. [ PMC free article ] [ PubMed ] [ Google Scholar ]
- Dill KA, Ghosh K, and Schmit JD (2011). Physical limits of cells and proteomes . Proc. Natl. Acad. Sci. USA 108 , 17876–17882. 10.1073/pnas.1114477108. [ PMC free article ] [ PubMed ] [ CrossRef ] [ Google Scholar ]
- Dong H, Nilsson L, and Kurland CG (1996). Co-variation of tRNA abundance and codon usage in Escherichia coli at different growth rates . J. Mol. Biol 260 , 649–663. [ PubMed ] [ Google Scholar ]
- Elf J, and Ehrenberg M. (2005). Near-critical behavior of aminoacyl-tRNA pools in E. coli at rate-limiting supply of amino acids . Biophys. J 88 , 132–146. [ PMC free article ] [ PubMed ] [ Google Scholar ]
- Erickson DW, Schink SJ, Patsalo V, Williamson JR, Gerland U, and Hwa T. (2017). A global resource allocation strategy governs growth transition kinetics of Escherichia coli . Nature 551 , 119–123. 10.1038/nature24299. [ PMC free article ] [ PubMed ] [ CrossRef ] [ Google Scholar ]
- Escalante A, Salinas Cervantes A, Gosset G, and Bolívar F. (2012). Current knowledge of the Escherichia coli phosphoenolpyruvate–carbohydrate phosphotransferase system: peculiarities of regulation and impact on growth and product formation . Appl. Microbiol. Biotechnol 94 , 1483–1494. 10.1007/s00253-012-4101-5. [ PubMed ] [ CrossRef ] [ Google Scholar ]
- Feist AM, Henry CS, Reed JL, Krummenacker M, Joyce AR, Karp PD, Broadbelt LJ, Hatzimanikatis V, and Palsson BØ (2007). A genome-scale metabolic reconstruction for Escherichia coli K-12 MG1655 that accounts for 1260 ORFs and thermodynamic information . Mol. Syst. Biol 3 , 121. 10.1038/msb4100155. [ PMC free article ] [ PubMed ] [ CrossRef ] [ Google Scholar ]
- Fernández-Coll L, Maciag-Dorszynska M, Tailor K, Vadia S, Levin PA, Szalewska-Palasz A, and Cashel M. (2020). The absence of (p)ppGpp renders initiation of Escherichia coli chromosomal DNA synthesis independent of growth rates . mBio 11 , 45. [ PMC free article ] [ PubMed ] [ Google Scholar ]
- Fijalkowska IJ, Schaaper RM, and Jonczyk P. (2012). DNA replication fidelity in Escherichia coli: A multi-DNA polymerase affair . FEMS Microbiol. Rev 36 , 1105–1121. 10.1111/j.1574-6976.2012.00338.x. [ PMC free article ] [ PubMed ] [ CrossRef ] [ Google Scholar ]
- Forchhammer J, and Lindahl L. (1971). Growth rate of polypeptide chains as a function of the cell growth rate in a mutant of Escherichia coli 15 . J. Mol. Biol 55 , 563–568. [ PubMed ] [ Google Scholar ]
- Gallagher LA, Bailey J, and Manoil C. (2020). Ranking essential bacterial processes by speed of mutant death . Proc. Natl. Acad. Sci. USA 117 , 18010–18017. 10.1073/pnas.2001507117. [ PMC free article ] [ PubMed ] [ CrossRef ] [ Google Scholar ]
- Gama-Castro S, Salgado H, Santos-Zavaleta A, Ledezma-Tejeida D, Muñiz-Rascado L, García-Sotelo JS, Alquicira-Hernández K, Martínez-Flores I, Pannier L, Castro-Mondragón JA, et al. (2016). RegulonDB version 9.0: high-level integration of gene regulation, coexpression, motif clustering and beyond . Nucleic Acids Res 44 , D133–D143. 10.1093/nar/gkv1156. [ PMC free article ] [ PubMed ] [ CrossRef ] [ Google Scholar ]
- Ge J, Yu G, Ator MA, and Stubbe J. (2003). Pre-steady-state and steady-state kinetic analysis of E. coli Class I ribonucleotide reductase . Biochemistry 42 , 10071–10083. 10.1021/bi034374r. [ PubMed ] [ CrossRef ] [ Google Scholar ]
- Godin M, Delgado FF, Son S, Grover WH, Bryan AK, Tzur A, Jorgensen P, Payer K, Grossman AD, Kirschner MW, and Manalis SR (2010). Using buoyant mass to measure the growth of single cells . Nat. Methods 7 , 387–390. [ PMC free article ] [ PubMed ] [ Google Scholar ]
- Gray WT, Govers SK, Xiang Y, Parry BR, Campos M, Kim S, and Jacobs-Wagner C. (2019). Nucleoid size scaling and intracellular organization of translation across bacteria . Cell 177 , 1632–1648.e20. [ PMC free article ] [ PubMed ] [ Google Scholar ]
- Guo Y, Li D, Zhang S, Yang Y, Liu JJ, Wang X, Liu C, Milkie DE, Moore RP, Tulu US, et al. (2018). Visualizing intracellular organelle and cytoskeletal interactions at nanoscale resolution on millisecond timescales . Cell 175 , 1430–1442.e17. [ PubMed ] [ Google Scholar ]
- Harris LK, and Theriot JA (2018). Surface area to volume ratio: a natural variable for bacterial morphogenesis . Trends Microbiol 26 , 815–832. [ PMC free article ] [ PubMed ] [ Google Scholar ]
- Harris RM, Webb DC, Howitt SM, and Cox GB (2001). Characterization of PitA and PitB from Escherichia coli . J. Bacteriol 183 , 5008–5014. 10.1128/JB.183.17.5008-5014.2001. [ PMC free article ] [ PubMed ] [ CrossRef ] [ Google Scholar ]
- Hauryliuk V, Atkinson GC, Murakami KS, Tenson T, and Gerdes K. (2015). Recent functional insights into the role of (p)ppGpp in bacterial physiology . Nat. Rev. Microbiol 13 , 298–309. [ PMC free article ] [ PubMed ] [ Google Scholar ]
- Heldal M, Norland S, and Tumyr O. (1985). X-ray microanalytic method for measurement of dry matter and elemental content of individual bacteria . Appl. Environ. Microbiol 50 , 1251–1257. [ PMC free article ] [ PubMed ] [ Google Scholar ]
- Helmstetter CE, and Cooper S. (1968). DNA synthesis during the division cycle of rapidly growing Escherichia coli B/r . J. Mol. Biol 31 , 507–518. [ PubMed ] [ Google Scholar ]
- Ho PY, and Amir A. (2015). Simultaneous regulation of cell size and chromosome replication in bacteria . Front. Microbiol 6 , 662. [ PMC free article ] [ PubMed ] [ Google Scholar ]
- Hu XP, Dourado H, Schubert P, and Lercher MJ (2020). The protein translation machinery is expressed for maximal efficiency in Escherichia coli . Nat. Commun 11 , 5260. [ PMC free article ] [ PubMed ] [ Google Scholar ]
- Hui S, Silverman JM, Chen SS, Erickson DW, Basan M, Wang J, Hwa T, and Williamson JR (2015). Quantitative proteomic analysis reveals a simple strategy of global resource allocation in bacteria . Mol. Syst. Biol 11 , 784. [ PMC free article ] [ PubMed ] [ Google Scholar ]
- Ireland WT, Beeler SM, Flores-Bautista E, McCarty NS, Röschinger T, Belliveau NM, Sweredoski MJ, Moradian A, Kinney JB, and Phillips R. (2020). Deciphering the regulatory genome of Escherichia coli, one hundred promoters at a time . eLife 9 , e55308. [ PMC free article ] [ PubMed ] [ Google Scholar ]
- Jensen RB, Wang SC, and Shapiro L. (2001). A moving DNA replication factory in Caulobacter crescentus . EMBO J 20 , 4952–4963. [ PMC free article ] [ PubMed ] [ Google Scholar ]
- Jin DJ, Cagliero C, and Zhou YN (2012). Growth rate regulation in Escherichia coli . FEMS Microbiol. Rev 36 , 269–287. [ PMC free article ] [ PubMed ] [ Google Scholar ]
- Jun S, Si F, Pugatch R, and Scott M. (2018). Fundamental principles in bacterial physiology-history, recent progress, and the future with focus on cell size control: a review . Rep. Prog. Phys 81 , 056601. 10.1088/1361-6633/aaa628. [ PMC free article ] [ PubMed ] [ CrossRef ] [ Google Scholar ]
- Karp PD, Billington R, Caspi R, Fulcher CA, Latendresse M, Kothari A, Keseler IM, Krummenacker M, Midford PE, Ong Q, et al. (2019). The BioCyc collection of microbial genomes and metabolic pathways . Brief. Bioinform 20 , 1085–1093. [ PMC free article ] [ PubMed ] [ Google Scholar ]
- Karr JR, Sanghvi JC, Macklin DN, Gutschow MV, Jacobs JM, Bolival B, Assad-Garcia N, Glass JI, and Covert MW (2012). A whole-cell computational model predicts phenotype from genotype . Cell 150 , 389–401. 10.1016/j.cell.2012.05.044. [ PMC free article ] [ PubMed ] [ CrossRef ] [ Google Scholar ]
- Keseler IM, Mackie A, Santos-Zavaleta A, Billington R, Bonavides-Martínez C, Caspi R, Fulcher C, Gama-Castro S, Kothari A, Krummenacker M, et al. (2017). The EcoCyc database: reflecting new knowledge about Escherichia coli K-12 . Nucleic Acids Res 45 , D543–D550. [ PMC free article ] [ PubMed ] [ Google Scholar ]
- Khademi S, O’Connell J, Remis J, Robles-Colmenares Y, Miercke LJW, and Stroud RM (2004). Mechanism of ammonia transport by Amt/MEP/Rh: structure of AmtB at 1.35 Å . Science 305 , 1587–1594. 10.1126/science.1101952. [ PubMed ] [ CrossRef ] [ Google Scholar ]
- Klumpp S, and Hwa T. (2008). Growth-rate-dependent partitioning of RNA polymerases in bacteria . Proc. Natl. Acad. Sci. USA 105 , 20245–20250. [ PMC free article ] [ PubMed ] [ Google Scholar ]
- Klumpp S, and Hwa T. (2014). Bacterial growth: global effects on gene expression, growth feedback and proteome partition . Curr. Opin. Biotechnol 28 , 96–102. 10.1016/j.copbio.2014.01.001. [ PMC free article ] [ PubMed ] [ CrossRef ] [ Google Scholar ]
- Klumpp S, Scott M, Pedersen S, and Hwa T. (2013). Molecular crowding limits translation and cell growth . Proc. Natl. Acad. Sci. USA 110 , 16754–16759. [ PMC free article ] [ PubMed ] [ Google Scholar ]
- Kostinski S, and Reuveni S. (2020). Ribosome composition maximizes cellular growth rates in E. coli . Phys. Rev. Lett 125 , 028103. 10.1103/PhysRevLett.125.028103. [ PubMed ] [ CrossRef ] [ Google Scholar ]
- Kraemer JA, Sanderlin AG, and Laub MT (2019). The stringent response inhibits DNA replication initiation in E. coli by modulating supercoiling of oriC . mBio 10 , 822. [ PMC free article ] [ PubMed ] [ Google Scholar ]
- Kubitschek HE, Baldwin WW, Schroeter SJ, and Graetzer R. (1984). Independence of buoyant cell density and growth rate in Escherichia coli . J. Bacteriol 158 , 296–299. [ PMC free article ] [ PubMed ] [ Google Scholar ]
- Lascu I, and Gonin P. (2000). The catalytic mechanism of nucleoside diphosphate kinases . J. Bioenerg. Biomembr 32 , 237–246. 10.1023/A:1005532912212. [ PubMed ] [ CrossRef ] [ Google Scholar ]
- Laxhuber KS, Morrison MJ, Chure G, Belliveau NM, Strandkvist C, Naughton KL, and Phillips R. (2020). Theoretical investigation of a genetic switch for metabolic adaptation . PLoS One 15 , e0226453. [ PMC free article ] [ PubMed ] [ Google Scholar ]
- Lex A, Gehlenborg N, Strobelt H, Vuillemot R, and Pfister H. (2014). UpSet: visualization of intersecting sets . IEEE Trans. Vis. Comput. Graph 20 , 1983–1992. [ PMC free article ] [ PubMed ] [ Google Scholar ]
- Li GW, Burkhardt D, Gross C, and Weissman JS (2014). Quantifying absolute protein synthesis rates reveals principles underlying allocation of cellular resources . Cell 157 , 624–635. 10.1016/j.cell.2014.02.033. [ PMC free article ] [ PubMed ] [ CrossRef ] [ Google Scholar ]
- Liebermeister W, Noor E, Flamholz A, Davidi D, Bernhardt J, and Milo R. (2014). Visual account of protein investment in cellular functions . Proc. Natl. Acad. Sci. USA 111 , 8488–8493. [ PMC free article ] [ PubMed ] [ Google Scholar ]
- Lin J, and Amir A. (2018). Homeostasis of protein and mRNA concentrations in growing cells . Nat. Commun 9 , 4496. 10.1038/s41467-018-06714-z. [ PMC free article ] [ PubMed ] [ CrossRef ] [ Google Scholar ]
- Liu M, Durfee T, Cabrera JE, Zhao K, Jin DJ, and Blattner FR (2005). Global transcriptional programs reveal a carbon source foraging strategy by Escherichia coli . J. Biol. Chem 280 , 15921–15927. 10.1074/jbc.M414050200. [ PubMed ] [ CrossRef ] [ Google Scholar ]
- Lovering AL, Safadi SS, and Strynadka NC (2012). Structural perspective of peptidoglycan biosynthesis and assembly . Annu. Rev. Biochem 81 , 451–478. 10.1146/annurev-biochem-061809-112742. [ PubMed ] [ CrossRef ] [ Google Scholar ]
- Lu D, Grayson P, and Schulten K. (2003). Glycerol conductance and physical asymmetry of the Escherichia coli glycerol facilitator GlpF . Biophys. J 85 , 2977–2987. 10.1016/S0006-3495(03)74718-3. [ PMC free article ] [ PubMed ] [ CrossRef ] [ Google Scholar ]
- Lynch M, and Marinov GK (2015). The bioenergetic costs of a gene . Proc. Natl. Acad. Sci. USA 112 , 15690–15695. 10.1073/pnas.1514974112. [ PMC free article ] [ PubMed ] [ CrossRef ] [ Google Scholar ]
- Maalœ O. (1979). Regulation of the protein-synthesizing machinery. ribosomes, trna, factors, and so on. In Biological Regulation and Development , vol 1 , Goldberger R, ed. (Springer; ), pp. 487–542. [ Google Scholar ]
- Macklin DN, Ahn-Horst TA, Choi H, Ruggero NA, Carrera J, Mason JC, Sun G, Agmon E, DeFelice MM, Maayan I, et al. (2020). Simultaneous cross-evaluation of heterogeneous E. coli datasets via mechanistic simulation . Science 369 , eaav3751. [ PMC free article ] [ PubMed ] [ Google Scholar ]
- Mahajan S. (2010). Street-Fighting Mathematics. The Art of Educated Guessing and Opportunistic Problem Solving (MIT Press; ). [ Google Scholar ]
- Metzl-Raz E, Kafri M, Yaakov G, Soifer I, Gurvich Y, and Barkai N. (2017). Principles of cellular resource allocation revealed by condition-dependent proteome profiling . eLife 6 , e03528. [ PMC free article ] [ PubMed ] [ Google Scholar ]
- Mikucki JA, Pearson A, Johnston DT, Turchyn AV, Farquhar J, Schrag DP, Anbar AD, Priscu JC, and Lee PA (2009). A contemporary microbially maintained subglacial ferrous “ocean” . Science 324 , 397–400. [ PubMed ] [ Google Scholar ]
- Milo R, Jorgensen P, Moran U, Weber G, and Springer M. (2010). BioNumbers—the database of key numbers in molecular and cell biology . Nucleic Acids Res 38 , D750–D753. 10.1093/nar/gkp889. [ PMC free article ] [ PubMed ] [ CrossRef ] [ Google Scholar ]
- Molenaar D, van Berlo R, de Ridder D, and Teusink B. (2009). Shifts in growth strategies reflect tradeoffs in cellular economics . Mol. Syst. Biol 5 , 323. [ PMC free article ] [ PubMed ] [ Google Scholar ]
- Monod J. (1947). The phenomenon of enzymatic adaptation and its bearings on problems of genetics and cellular differentiation . Selected Papers in Molecular Biology by Jacques Monod 1947 , 223–289. [ Google Scholar ]
- Monod J. (1949). The growth of bacterial cultures . Annu. Rev. Microbiol 3 , 371–394. [ Google Scholar ]
- Morgenstein RM, Bratton BP, Nguyen JP, Ouzounov N, Shaevitz JW, and Gitai Z. (2015). RodZ links MreB to cell wall synthesis to mediate MreB rotation and robust morphogenesis . Proc. Natl. Acad. Sci. USA 112 , 12510–12515. 10.1073/pnas.1509610112. [ PMC free article ] [ PubMed ] [ CrossRef ] [ Google Scholar ]
- Neidhardt FC, Ingraham J, and Schaechter M. (1991). Physiology of the Bacterial Cell - A Molecular Approach, vol 1 (Elsevier) . [ Google Scholar ]
- Nomura M, Gourse R, and Baughman G. (1984). Regulation of the synthesis of ribosomes and ribosomal components . Annu. Rev. Biochem 53 , 75–117. [ PubMed ] [ Google Scholar ]
- Ojkic N, Serbanescu D, and Banerjee S. (2019). Surface-to-volume scaling and aspect ratio preservation in rod-shaped bacteria . eLife 8 , 642. [ PMC free article ] [ PubMed ] [ Google Scholar ]
- Panlilio M, Grilli J, Tallarico G, Iuliani I, Sclavi B, Cicuta P, and Lagomarsino MC (2020). Threshold accumulation of a constitutive protein explains E. coli cell division behavior in nutrient upshifts . bioRxiv 10.1101/2020.08.03.233908. [ PMC free article ] [ PubMed ] [ CrossRef ] [ Google Scholar ]
- Parker DJ, Lalanne JB, Kimura S, Johnson GE, Waldor MK, and Li GW (2020). Growth-optimized aminoacyl-tRNA synthetase levels prevent maximal tRNA charging . Cell Syst . 11 , 121–130.e6. [ PMC free article ] [ PubMed ] [ Google Scholar ]
- Patrick M, Dennis PP, Ehrenberg M, and Bremer H. (2015). Free RNA polymerase in Escherichia coli . Biochimie 119 , 80–91. 10.1016/j.biochi.2015.10.015. [ PubMed ] [ CrossRef ] [ Google Scholar ]
- Paul BJ, Barker MM, Ross W, Schneider DA, Webb C, Foster JW, and Gourse RL (2004). DksA: a critical component of the transcription initiation machinery that potentiates the regulation of rRNA promoters by ppGpp and the initiating NTP . Cell 118 , 311–322. [ PubMed ] [ Google Scholar ]
- Pedersen S. (1984). Escherichia coli ribosomes translate in vivo with variable rate . EMBO J . 3 , 2895–2898. [ PMC free article ] [ PubMed ] [ Google Scholar ]
- Pedersen S, Bloch PL, Reeh S, and Neidhardt FC (1978). Patterns of protein synthesis in E. coli: a catalog of the amount of 140 individual proteins at different growth rates . Cell 14 , 179–190. [ PubMed ] [ Google Scholar ]
- Peebo K, Valgepea K, Maser A, Nahku R, Adamberg K, and Vilu R. (2015). Proteome reallocation in Escherichia coli with increasing specific growth rate . Mol. Biosyst 11 , 1184–1193. 10.1039/C4MB00721B. [ PubMed ] [ CrossRef ] [ Google Scholar ]
- Phillips R. (2018). Membranes by the numbers. In Physics Biological Membranes (Springer; ), pp. 73–105. [ Google Scholar ]
- Ramos S, and Kaback HR (1977). The relationship between the electrochemical proton gradient and active transport in Escherichia coli membrane vesicles . Biochemistry 16 , 854–859. 10.1021/bi00624a007. [ PubMed ] [ CrossRef ] [ Google Scholar ]
- Ranganathan S, Tee TW, Chowdhury A, Zomorrodi AR, Yoon JM, Fu Y, Shanks JV, and Maranas CD (2012). An integrated computational and experimental study for overproducing fatty acids in Escherichia coli . Metab. Eng 14 , 687–704. 10.1016/j.ymben.2012.08.008. [ PubMed ] [ CrossRef ] [ Google Scholar ]
- Reuveni S, Ehrenberg M, and Paulsson J. (2017). Ribosomes are optimized for autocatalytic production . Nature 547 , 293–297. [ PMC free article ] [ PubMed ] [ Google Scholar ]
- Roller BRK, Stoddard SF, and Schmidt TM (2016). Exploiting rRNA operon copy number to investigate bacterial reproductive strategies . Nat. Microbiol 1 , 16160. [ PMC free article ] [ PubMed ] [ Google Scholar ]
- Rosenberg H, Gerdes RG, and Chegwidden K. (1977). Two systems for the uptake of phosphate in Escherichia coli . J. Bacteriol 131 , 505–511. [ PMC free article ] [ PubMed ] [ Google Scholar ]
- Rudd SG, Valerie NCK, and Helleday T. (2016). Pathways controlling dNTP pools to maintain genome stability . DNA Repair 44 , 193–204. 10.1016/j.dnarep.2016.05.032. [ PubMed ] [ CrossRef ] [ Google Scholar ]
- Ruppe A, and Fox JM (2018). Analysis of interdependent kinetic controls of fatty acid synthases . ACS Catal . 8 , 11722–11734. 10.1021/acscatal.8b03171. [ PMC free article ] [ PubMed ] [ CrossRef ] [ Google Scholar ]
- Sánchez-Romero MA, Molina F, and Jiménez-Sánchez A. (2011). Organization of ribonucleoside diphosphate reductase during multifork chromosome replication in Escherichia coli . Microbiology (Reading) 157 , 2220–2225. 10.1099/mic.0.049478-0. [ PubMed ] [ CrossRef ] [ Google Scholar ]
- Schaechter M, MaalØe O, and Kjeldgaard NO (1958). Dependency on medium and temperature of cell size and chemical composition during balanced growth of Salmonella typhimurium . Microbiology 19 , 592–606. [ PubMed ] [ Google Scholar ]
- Schmidt A, Kochanowski K, Vedelaar S, Ahrné E, Volkmer B, Callipo L, Knoops K, Bauer M, Aebersold R, and Heinemann M. (2016). The quantitative and condition-dependent Escherichia coli proteome . Nat. Biotechnol 34 , 104–110. 10.1038/nbt.3418. [ PMC free article ] [ PubMed ] [ CrossRef ] [ Google Scholar ]
- Scott M, Gunderson CW, Mateescu EM, Zhang Z, and Hwa T. (2010). Interdependence of cell growth and gene expression: origins and consequences . Science 330 , 1099–1102. [ PubMed ] [ Google Scholar ]
- Scott M, Klumpp S, Mateescu EM, and Hwa T. (2014). Emergence of robust growth laws from optimal regulation of ribosome synthesis . Mol. Syst. Biol 10 , 747. 10.15252/msb.20145379. [ PMC free article ] [ PubMed ] [ CrossRef ] [ Google Scholar ]
- Sekowska A, Kung HF, and Danchin A. (2000). Sulfur metabolism in Escherichia coli and related bacteria: facts and fiction . J. Mol. Microbiol. Biotechnol 2 , 145–177. [ PubMed ] [ Google Scholar ]
- Serbanescu D, Ojkic N, and Banerjee S. (2020). Nutrient-dependent tradeoffs between ribosomes and division protein synthesis control bacterial cell size and growth . Cell Rep . 32 , 108183. [ PubMed ] [ Google Scholar ]
- Shi H, Bratton BP, Gitai Z, and Huang KC (2018). How to build a bacterial cell: MreB as the foreman of E. coli construction . Cell 172 , 1294–1305. 10.1016/j.cell.2018.02.050. [ PMC free article ] [ PubMed ] [ CrossRef ] [ Google Scholar ]
- Si F, Le Treut G, Sauls JT, Vadia S, Levin PA, and Jun S. (2019). Mechanistic origin of cell-size control and homeostasis in bacteria . Curr. Biol 29 , 1760–1770.e7. 10.1016/j.cub.2019.04.062. [ PMC free article ] [ PubMed ] [ CrossRef ] [ Google Scholar ]
- Si F, Li D, Cox SE, Sauls JT, Azizi O, Sou C, Schwartz AB, Erickstad MJ, Jun Y, Li X, and Jun S. (2017). Invariance of initiation mass and predictability of cell size in Escherichia coli . Curr. Biol 27 , 1278–1287. [ PMC free article ] [ PubMed ] [ Google Scholar ]
- Sirko A, Zatyka M, Sadowy E, and Hulanicka D. (1995). Sulfate and thiosulfate transport in Escherichia coli K-12: evidence for a functional overlapping of sulfate-and thiosulfate-binding proteins . J. Bacteriol 177 , 4134–4136. 10.1128/jb.177.14.4134-4136.1995. [ PMC free article ] [ PubMed ] [ CrossRef ] [ Google Scholar ]
- Sohlenkamp C, and Geiger O. (2016). Bacterial membrane lipids: diversity in structures and pathways . FEMS Microbiol. Rev 40 , 133–159. 10.1093/femsre/fuv008. [ PubMed ] [ CrossRef ] [ Google Scholar ]
- Soler-Bistué A, Aguilar-Pierlé S, Garcia-Garcerá M, Val ME, Sismeiro O, Varet H, Sieira R, Krin E, Skovgaard O, Comerci DJ, et al. (2020). Macromolecular crowding links ribosomal protein gene dosage to growth rate in Vibrio cholerae . BMC Biol . 18 , 43. [ PMC free article ] [ PubMed ] [ Google Scholar ]
- Soufi B, Krug K, Harst A, and Macek B. (2015). Characterization of the E. coli proteome and its modifications during growth and ethanol stress . Front. Microbiol 6 , 198. [ PMC free article ] [ PubMed ] [ Google Scholar ]
- Stasi R, Neves HI, and Spira B. (2019). Phosphate uptake by the phosphonate transport system PhnCDE . BMC Microbiol . 19 , 79. 10.1186/s12866-019-1445-3. [ PMC free article ] [ PubMed ] [ CrossRef ] [ Google Scholar ]
- Stouthamer AH (1973). A theoretical study on the amount of ATP required for synthesis of microbial cell material . Antonie Leeuwenhoek 39 , 545–565. 10.1007/BF02578899. [ PubMed ] [ CrossRef ] [ Google Scholar ]
- Svenningsen SL, Kongstad M, Stenum T.S.n., Muñoz-Gómez AJ, and Sørensen MA (2017). Transfer RNA is highly unstable during early amino acid starvation in Escherichia coli . Nucleic Acids Res 45 , 793–804. 10.1093/nar/gkw1169. [ PMC free article ] [ PubMed ] [ CrossRef ] [ Google Scholar ]
- Szenk M, Dill KA, and de Graff AMR (2017). Why do fast-growing bacteria enter overflow metabolism? Testing the membrane real estate hypothesis . Cell Syst . 5 , 95–104. 10.1016/j.cels.2017.06.005. [ PubMed ] [ CrossRef ] [ Google Scholar ]
- Taheri-Araghi S, Bradde S, Sauls JT, Hill NS, Levin PA, Paulsson J, Vergassola M, and Jun S. (2015). Cell-size control and homeostasis in bacteria . Curr. Biol 25 , 385–391. [ PMC free article ] [ PubMed ] [ Google Scholar ]
- Taniguchi Y, Choi PJ, Li GW, Chen H, Babu M, Hearn J, Emili A, and Xie XS (2010). Quantifying E. coli proteome and transcriptome with single-molecule sensitivity in single cells . Science 329 , 533–538. [ PMC free article ] [ PubMed ] [ Google Scholar ]
- Tatusov RL, Galperin MY, Natale DA, and Koonin EV (2000). The COG database: a tool for genome-scale analysis of protein functions and evolution . Nucleic Acids Res . 28 , 33–36. [ PMC free article ] [ PubMed ] [ Google Scholar ]
- Taymaz-Nikerel H, Borujeni AE, Verheijen PJT, Heijnen JJ, and van Gulik WM (2010). Genome-derived minimal metabolic models for Escherichia coli MG1655 with estimated in vivo respiratory ATP stoichiometry . Biotechnol. Bioeng 107 , 369–381. 10.1002/bit.22802. [ PubMed ] [ CrossRef ] [ Google Scholar ]
- The Gene Ontology Consortium (2019). The Gene Ontology resource: 20 years and still GOing strong . Nucleic Acids Res . 47 , D330–D338. [ PMC free article ] [ PubMed ] [ Google Scholar ]
- Vadia S, Tse JL, Lucena R, Yang Z, Kellogg DR, Wang JD, and Levin PA (2017). Fatty acid availability sets cell envelope capacity and dictates microbial cell size . Curr. Biol 27 , 1757–1767.e5. [ PMC free article ] [ PubMed ] [ Google Scholar ]
- Valgepea K, Adamberg K, Seiman A, and Vilu R. (2013). Escherichia coliachieves faster growth by increasing catalytic and translation rates of proteins . Mol. Biosyst 9 , 2344–2358. 10.1039/c3mb70119k. [ PubMed ] [ CrossRef ] [ Google Scholar ]
- van Heeswijk WC, Westerhoff HV, and Boogerd FC (2013). Nitrogen assimilation in Escherichia coli: putting molecular data into a systems perspective . Microbiol. Mol. Biol. Rev 77 , 628–695. 10.1128/MMBR.00025-13. [ PMC free article ] [ PubMed ] [ CrossRef ] [ Google Scholar ]
- Virtanen P, Gommers R, Oliphant TE, Haberland M, Reddy T, Cournapeau D, Burovski E, Peterson P, Weckesser W, Bright JEW, et al. (2020). SciPy 1.0: fundamental algorithms for scientific computing in python . Nat. Methods 17 , 261–272. 10.1038/s41592-019-0686-2. [ PMC free article ] [ PubMed ] [ CrossRef ] [ Google Scholar ]
- Volkmer B, and Heinemann M. (2011). Condition-dependent cell volume and concentration of Escherichia coli to facilitate data conversion for systems biology modeling . PLoS One 6 , e23126. [ PMC free article ] [ PubMed ] [ Google Scholar ]
- Weber J, and Senior AE (2003). ATP synthesis driven by proton transport in F 1 F 0 -ATP synthase . FEBS Lett . 545 , 61–70. 10.1016/S0014-5793(03)00394-6. [ PubMed ] [ CrossRef ] [ Google Scholar ]
- Willsky GR, Bennett RL, and Malamy MH (1973). Inorganic phosphate transport in Escherichia coli: involvement of two genes which play a role in alkaline phosphatase regulation . J. Bacteriol 113 , 529–539. [ PMC free article ] [ PubMed ] [ Google Scholar ]
- You C, Okano H, Hui S, Zhang Z, Kim M, Gunderson CW, Wang YP, Lenz P, Yan D, and Hwa T. (2013). Coordination of bacterial proteome with metabolism by cyclic AMP signalling . Nature 500 , 301–306. [ PMC free article ] [ PubMed ] [ Google Scholar ]
- Young R, and Bremer H. (1976). Polypeptide-chain-elongation rate in Escherichia coli B/r as a function of growth rate . Biochem. J 160 , 185–194. [ PMC free article ] [ PubMed ] [ Google Scholar ]
- Yu X, Liu T, Zhu F, and Khosla C. (2011). In vitro reconstitution and steady-state analysis of the fatty acid synthase from Escherichia coli . Proc. Natl. Acad. Sci. USA 108 , 18643–18648. 10.1073/pnas.1110852108. [ PMC free article ] [ PubMed ] [ CrossRef ] [ Google Scholar ]
- Zhang L, Jiang W, Nan J, Almqvist J, and Huang Y. (2014a). The Escherichia coli CysZ is a pH dependent sulfate transporter that can be inhibited by sulfite . Biochim. Biophys. Acta 1838 , 1809–1816. 10.1016/j.bbamem.2014.03.003. [ PubMed ] [ CrossRef ] [ Google Scholar ]
- Zhang Z, Aboulwafa M, and Saier MH (2014b). Regulation of crp gene expression by the catabolite repressor/activator, cra, in Escherichia coli . J. Mol. Microbiol. Biotechnol 24 , 135–141. 10.1159/000362722. [ PMC free article ] [ PubMed ] [ CrossRef ] [ Google Scholar ]
- Zhang Q, Brambilla E, Li R, Shi H, Lagomarsino MC, Sclavi B, and Bulman Z. (2020). A decrease in transcription capacity limits growth rate upon translation inhibition . mSystems 5 , 46. [ PMC free article ] [ PubMed ] [ Google Scholar ]
- Zheng H, Bai Y, Jiang M, Tokuyasu TA, Huang X, Zhong F, Wu Y, Fu X, Kleckner N, Hwa T, and Liu C. (2020). General quantitative relations linking cell growth and the cell cycle in Escherichia coli . Nat. Microbiol 5 , 995–1001. [ PMC free article ] [ PubMed ] [ Google Scholar ]
- Zhu M, and Dai X. (2019). Growth suppression by altered (p)ppGpp levels results from non-optimal resource allocation in Escherichia coli . Nucleic Acids Res 47 , 4684–4693. [ PMC free article ] [ PubMed ] [ Google Scholar ]
- Zhuang K, Vemuri GN, and Mahadevan R. (2011). Economics of membrane occupancy and respiro-fermentation . Mol. Syst. Biol 7 , 500. [ PMC free article ] [ PubMed ] [ Google Scholar ]

An official website of the United States government
The .gov means it’s official. Federal government websites often end in .gov or .mil. Before sharing sensitive information, make sure you’re on a federal government site.
The site is secure. The https:// ensures that you are connecting to the official website and that any information you provide is encrypted and transmitted securely.
- Publications
- Account settings
- My Bibliography
- Collections
- Citation manager
Save citation to file
Email citation, add to collections.
- Create a new collection
- Add to an existing collection
Add to My Bibliography
Your saved search, create a file for external citation management software, your rss feed.
- Search in PubMed
- Search in NLM Catalog
- Add to Search
Growth Phase Contribution in Dictating Drug Transport and Subcellular Accumulation inside Escherichia coli
Affiliations.
- 1 Department of Chemistry, Indian Institute of Technology Kanpur, Kanpur, Uttar Pradesh 208016, India.
- 2 Center of Excellence: Tropical and Infectious Diseases, Gangwal School of Medical Sciences and Technology, Indian Institute of Technology Kanpur, Kanpur, Uttar Pradesh 208016, India.
- PMID: 39178142
- DOI: 10.1021/acsinfecdis.4c00252
Depending upon nutrient availability, bacteria transit to multiple growth phases. The transition from the active to nongrowing phase results in reduced drug efficacy and, in some cases, even multidrug resistance. However, due to multiple alterations in the cell envelope, probing the drug permeation kinetics during growth phases becomes perplexing, especially across the Gram-negative bacteria's complex dual membrane envelope. To advance the understanding of drug permeation during the life cycle of Gram-negative bacteria, we sought to address two underlying objectives: (a) how changes are occurring inside the bacterial envelope during growth and (b) how the drug permeation and accumulation vary across both the membranes and in subcellular compartments during growth. Both objectives are met with the help of nonlinear optical technique second-harmonic generation spectroscopy (SHG). Specifically, using SHG, we probed the transport kinetics and accumulation of a quaternary ammonium compound (QAC), malachite green, inside Escherichia coli in various growth phases. Further insight about another QAC molecule, propidium iodide, is accomplished using fluorescence microscopy. Results indicate that actively growing cells have faster drug transport and higher cytoplasmic accumulation than slow- or nongrowing cells. In this regard, the rpoS gene plays a crucial role in limiting drug transport across the saturation phase cultures. Moreover, within a particular growth phase, membrane permeability undergoes gradual changes much before the subsequent growth phase commences. These outcomes signify the importance of reporting the growth phase and rate in drug efficacy studies.
Keywords: bacterial growth; drug transport kinetics; lag phase; rpoS gene; second-harmonic generation; starvation.
PubMed Disclaimer
LinkOut - more resources
Full text sources.
- American Chemical Society
Miscellaneous
- NCI CPTAC Assay Portal
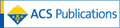
- Citation Manager
NCBI Literature Resources
MeSH PMC Bookshelf Disclaimer
The PubMed wordmark and PubMed logo are registered trademarks of the U.S. Department of Health and Human Services (HHS). Unauthorized use of these marks is strictly prohibited.

IMAGES
COMMENTS
Bacterial growth is of interest to statistical physicists for several reasons. First, the process of division into daughter cells is a branching process with somewhat stochastic timing; the time between successive bacterial divisions is a random variable with a rather broad distribution [ 45, 47, 65, 66 ].
The growth curve. The bacterial growth curve is a marvellous resource - the information contained within is invaluable, but often overlooked. The growth curve has discrete and recognizable phases that reflect distinct physiological states of the cells in culture: the lag phase as the organism adjusts to environmental conditions and adjusts its physiology to enable rapid growth; the ...
"Classic" Microbial Growth Physiology. The rapid developments between 1930 and 1960 in genetic and biochemical techniques, in methods for controlled cultivation, and advances in the quantitative description of microbial growth, led to a true "harvest period" between 1940 and 1970 with respect to our understanding of microbial physiology (in terms of both accumulation of experimental ...
Bacterial growth. Growth is shown as L = log (numbers) where numbers is the number of colony forming units per ml, versus T (time.) Bacterial growth is proliferation of bacterium into two daughter cells, in a process called binary fission. Providing no mutation event occurs, the resulting daughter cells are genetically identical to the original ...
Bacterial growth is of interest to statistical physicists for several reasons. First, the process of division into daughter cells is a branching process with somewhat stochastic timing; the time between successive bacterial divisions is a random variable with a rather broad distribution [ 45, 47, 65, 66 ].
Thermodynamics of growth—non-equilibrium thermodynamics of bacterial-growth—the phenomenological and the mosaic approach. Biochim Biophys Acta 683 : 181-220. Article CAS Google Scholar
In the macroscopic theory of bacterial growth of cell populations, the specific growth rate equals k = ln2/τ g, with τ g as the generation time (also called the doubling time).
Bacterial growth exhibits biphasic growth dynamics. The cells initially grow linearly until a specific size is reached. The growth rate of the cell in this phase is independent of the initial cell size, and therefore smaller cells grow for a longer time. During the second phase the cell grows exponentially for a fixed time duration.
Bacterial suspension is removed at the same rate as nutrients flow in to maintain an optimal growth environment. Figure 9.7 A chemostat is a culture vessel fitted with an opening to add nutrients (feed) and an outlet to remove contents (effluent), effectively diluting toxic wastes and dead cells.
As a test of this theory, we chose a collection of 13 bacterial strains with variable growth rates (Fig. 3a). This group comprised six strains from the American Type Culture Collection (ATCC) and ...
Laboratory studies on bacterial growth have also provided limited information regarding growth in colonies on or in solidified laboratory media [12], [13] and in biofilms ... Viability and isolation of marine bacteria by dilution culture theory, procedures, and initial results. Appl Environ Microbiol, 59 (3) (1993), pp. 881-891. Google Scholar ...
Bacterial growth laws: A. When growth is modulated by changes in nutrient quality, the RNA mass fraction r (proportional to the ribosomal content) of E. coli increases linearly with growth rate γ (solid line): r = r0 +γ/κ t, where the parameter κ t is related to the translation rate [ 8•• ], and r0 is the offset.
where μ is the specific growth rate; μ max is the maximum specific growth rate;S is the concentration of the limiting substrate for growth; and K s is the half-velocity constant, the value of S when μ/μ max = 0.5.. Sometimes, researchers start with statistical models to first identify the effective factors on microbial growth and then use these effects and outcomes of the statistical ...
In our hypothesis,we believed that the higher temperature is, the less bacteria will survive on a certain object (lock) Dependent Variable: Temperature that are added to the lock Independent Variable: The bacteria that is produced Controlled Variables: 1.Amount of agar 2. Amount of time for the growth of bacteria
Bacterial physiology then became a handmaiden of molecular biology and was greatly influenced by the discovery of cellular regulatory mechanisms. Microbial growth, which had come of age with the early work of Hershey, Monod, and others, was later pursued by studies on a whole cell level by what became known as the "Copenhagen School.".
Phytohormones play a crucial role in regulating growth, productivity, and development while also aiding in the response to diverse environmental changes, encompassing both biotic and abiotic factors. Phytohormone levels in soil and plant tissues are influenced by specific soil bacteria, leading to direct effects on plant growth, development, and stress tolerance.
This novel hypothesis offers a promising approach for understanding the evolutionary constraints on cell size. The ISME Journal - The evolution of bacterial cell size: the internal diffusion ...
Bacteria that are resistant to antibiotics thrive in the face of drug treatment, which can lead to life threatening, often incurable infections. Other bacteria can exist in a pre-resistant state. To survive, slow bacterial growth allows mutations to arise that help the bugs tolerate the drug, or shifting phenotypes help them persist in its ...
Medium without bacteria inoculation was used as controls. E Flow chart of H 2 S production from DSS test (left). H 2 S production analysis by E. coli and P. mirabilis strains was examined using lead acetate strip (right). F Growth of E. coli WT and mutant strains on M9 supplemented with DSS as the sole sulfur source.
This hypothesis is bolstered by the observation that, when we consider the change in cell size and the diminishing surface area to volume ratio at faster growth rates, there still appears to be sufficient space in the cell membrane for the key proteins required for energy production and nutrient uptake. ... Bacterial growth: global effects on ...
Depending upon nutrient availability, bacteria transit to multiple growth phases. The transition from the active to nongrowing phase results in reduced drug efficacy and, in some cases, even multidrug resistance. However, due to multiple alterations in the cell envelope, probing the drug permeation …