Exploring Experimental Research: Methodologies, Designs, and Applications Across Disciplines
- SSRN Electronic Journal

- The National University of Cheasim Kamchaymear
Discover the world's research
- 25+ million members
- 160+ million publication pages
- 2.3+ billion citations

- Khaoula Boussalham

- COMPUT COMMUN REV

- Debbie Rohwer

- Sorakrich Maneewan
- Ravinder Koul
- Int J Contemp Hospit Manag

- J EXP ANAL BEHAV
- Alan E. Kazdin
- Jimmie Leppink
- Keith Morrison
- Louis Cohen
- Lawrence Manion
- ACCOUNT ORG SOC
- Wim A. Van der Stede
- Recruit researchers
- Join for free
- Login Email Tip: Most researchers use their institutional email address as their ResearchGate login Password Forgot password? Keep me logged in Log in or Continue with Google Welcome back! Please log in. Email · Hint Tip: Most researchers use their institutional email address as their ResearchGate login Password Forgot password? Keep me logged in Log in or Continue with Google No account? Sign up

Have a language expert improve your writing
Run a free plagiarism check in 10 minutes, automatically generate references for free.
- Knowledge Base
- Methodology
Research Design | Step-by-Step Guide with Examples
Published on 5 May 2022 by Shona McCombes . Revised on 20 March 2023.
A research design is a strategy for answering your research question using empirical data. Creating a research design means making decisions about:
- Your overall aims and approach
- The type of research design you’ll use
- Your sampling methods or criteria for selecting subjects
- Your data collection methods
- The procedures you’ll follow to collect data
- Your data analysis methods
A well-planned research design helps ensure that your methods match your research aims and that you use the right kind of analysis for your data.
Table of contents
Step 1: consider your aims and approach, step 2: choose a type of research design, step 3: identify your population and sampling method, step 4: choose your data collection methods, step 5: plan your data collection procedures, step 6: decide on your data analysis strategies, frequently asked questions.
- Introduction
Before you can start designing your research, you should already have a clear idea of the research question you want to investigate.
There are many different ways you could go about answering this question. Your research design choices should be driven by your aims and priorities – start by thinking carefully about what you want to achieve.
The first choice you need to make is whether you’ll take a qualitative or quantitative approach.
Qualitative approach | Quantitative approach |
---|---|
Qualitative research designs tend to be more flexible and inductive , allowing you to adjust your approach based on what you find throughout the research process.
Quantitative research designs tend to be more fixed and deductive , with variables and hypotheses clearly defined in advance of data collection.
It’s also possible to use a mixed methods design that integrates aspects of both approaches. By combining qualitative and quantitative insights, you can gain a more complete picture of the problem you’re studying and strengthen the credibility of your conclusions.
Practical and ethical considerations when designing research
As well as scientific considerations, you need to think practically when designing your research. If your research involves people or animals, you also need to consider research ethics .
- How much time do you have to collect data and write up the research?
- Will you be able to gain access to the data you need (e.g., by travelling to a specific location or contacting specific people)?
- Do you have the necessary research skills (e.g., statistical analysis or interview techniques)?
- Will you need ethical approval ?
At each stage of the research design process, make sure that your choices are practically feasible.
Prevent plagiarism, run a free check.
Within both qualitative and quantitative approaches, there are several types of research design to choose from. Each type provides a framework for the overall shape of your research.
Types of quantitative research designs
Quantitative designs can be split into four main types. Experimental and quasi-experimental designs allow you to test cause-and-effect relationships, while descriptive and correlational designs allow you to measure variables and describe relationships between them.
Type of design | Purpose and characteristics |
---|---|
Experimental | |
Quasi-experimental | |
Correlational | |
Descriptive |
With descriptive and correlational designs, you can get a clear picture of characteristics, trends, and relationships as they exist in the real world. However, you can’t draw conclusions about cause and effect (because correlation doesn’t imply causation ).
Experiments are the strongest way to test cause-and-effect relationships without the risk of other variables influencing the results. However, their controlled conditions may not always reflect how things work in the real world. They’re often also more difficult and expensive to implement.
Types of qualitative research designs
Qualitative designs are less strictly defined. This approach is about gaining a rich, detailed understanding of a specific context or phenomenon, and you can often be more creative and flexible in designing your research.
The table below shows some common types of qualitative design. They often have similar approaches in terms of data collection, but focus on different aspects when analysing the data.
Type of design | Purpose and characteristics |
---|---|
Grounded theory | |
Phenomenology |
Your research design should clearly define who or what your research will focus on, and how you’ll go about choosing your participants or subjects.
In research, a population is the entire group that you want to draw conclusions about, while a sample is the smaller group of individuals you’ll actually collect data from.
Defining the population
A population can be made up of anything you want to study – plants, animals, organisations, texts, countries, etc. In the social sciences, it most often refers to a group of people.
For example, will you focus on people from a specific demographic, region, or background? Are you interested in people with a certain job or medical condition, or users of a particular product?
The more precisely you define your population, the easier it will be to gather a representative sample.
Sampling methods
Even with a narrowly defined population, it’s rarely possible to collect data from every individual. Instead, you’ll collect data from a sample.
To select a sample, there are two main approaches: probability sampling and non-probability sampling . The sampling method you use affects how confidently you can generalise your results to the population as a whole.
Probability sampling | Non-probability sampling |
---|---|
Probability sampling is the most statistically valid option, but it’s often difficult to achieve unless you’re dealing with a very small and accessible population.
For practical reasons, many studies use non-probability sampling, but it’s important to be aware of the limitations and carefully consider potential biases. You should always make an effort to gather a sample that’s as representative as possible of the population.
Case selection in qualitative research
In some types of qualitative designs, sampling may not be relevant.
For example, in an ethnography or a case study, your aim is to deeply understand a specific context, not to generalise to a population. Instead of sampling, you may simply aim to collect as much data as possible about the context you are studying.
In these types of design, you still have to carefully consider your choice of case or community. You should have a clear rationale for why this particular case is suitable for answering your research question.
For example, you might choose a case study that reveals an unusual or neglected aspect of your research problem, or you might choose several very similar or very different cases in order to compare them.
Data collection methods are ways of directly measuring variables and gathering information. They allow you to gain first-hand knowledge and original insights into your research problem.
You can choose just one data collection method, or use several methods in the same study.
Survey methods
Surveys allow you to collect data about opinions, behaviours, experiences, and characteristics by asking people directly. There are two main survey methods to choose from: questionnaires and interviews.
Questionnaires | Interviews |
---|---|
Observation methods
Observations allow you to collect data unobtrusively, observing characteristics, behaviours, or social interactions without relying on self-reporting.
Observations may be conducted in real time, taking notes as you observe, or you might make audiovisual recordings for later analysis. They can be qualitative or quantitative.
Quantitative observation | |
---|---|
Other methods of data collection
There are many other ways you might collect data depending on your field and topic.
Field | Examples of data collection methods |
---|---|
Media & communication | Collecting a sample of texts (e.g., speeches, articles, or social media posts) for data on cultural norms and narratives |
Psychology | Using technologies like neuroimaging, eye-tracking, or computer-based tasks to collect data on things like attention, emotional response, or reaction time |
Education | Using tests or assignments to collect data on knowledge and skills |
Physical sciences | Using scientific instruments to collect data on things like weight, blood pressure, or chemical composition |
If you’re not sure which methods will work best for your research design, try reading some papers in your field to see what data collection methods they used.
Secondary data
If you don’t have the time or resources to collect data from the population you’re interested in, you can also choose to use secondary data that other researchers already collected – for example, datasets from government surveys or previous studies on your topic.
With this raw data, you can do your own analysis to answer new research questions that weren’t addressed by the original study.
Using secondary data can expand the scope of your research, as you may be able to access much larger and more varied samples than you could collect yourself.
However, it also means you don’t have any control over which variables to measure or how to measure them, so the conclusions you can draw may be limited.
As well as deciding on your methods, you need to plan exactly how you’ll use these methods to collect data that’s consistent, accurate, and unbiased.
Planning systematic procedures is especially important in quantitative research, where you need to precisely define your variables and ensure your measurements are reliable and valid.
Operationalisation
Some variables, like height or age, are easily measured. But often you’ll be dealing with more abstract concepts, like satisfaction, anxiety, or competence. Operationalisation means turning these fuzzy ideas into measurable indicators.
If you’re using observations , which events or actions will you count?
If you’re using surveys , which questions will you ask and what range of responses will be offered?
You may also choose to use or adapt existing materials designed to measure the concept you’re interested in – for example, questionnaires or inventories whose reliability and validity has already been established.
Reliability and validity
Reliability means your results can be consistently reproduced , while validity means that you’re actually measuring the concept you’re interested in.
Reliability | Validity |
---|---|
For valid and reliable results, your measurement materials should be thoroughly researched and carefully designed. Plan your procedures to make sure you carry out the same steps in the same way for each participant.
If you’re developing a new questionnaire or other instrument to measure a specific concept, running a pilot study allows you to check its validity and reliability in advance.
Sampling procedures
As well as choosing an appropriate sampling method, you need a concrete plan for how you’ll actually contact and recruit your selected sample.
That means making decisions about things like:
- How many participants do you need for an adequate sample size?
- What inclusion and exclusion criteria will you use to identify eligible participants?
- How will you contact your sample – by mail, online, by phone, or in person?
If you’re using a probability sampling method, it’s important that everyone who is randomly selected actually participates in the study. How will you ensure a high response rate?
If you’re using a non-probability method, how will you avoid bias and ensure a representative sample?
Data management
It’s also important to create a data management plan for organising and storing your data.
Will you need to transcribe interviews or perform data entry for observations? You should anonymise and safeguard any sensitive data, and make sure it’s backed up regularly.
Keeping your data well organised will save time when it comes to analysing them. It can also help other researchers validate and add to your findings.
On their own, raw data can’t answer your research question. The last step of designing your research is planning how you’ll analyse the data.
Quantitative data analysis
In quantitative research, you’ll most likely use some form of statistical analysis . With statistics, you can summarise your sample data, make estimates, and test hypotheses.
Using descriptive statistics , you can summarise your sample data in terms of:
- The distribution of the data (e.g., the frequency of each score on a test)
- The central tendency of the data (e.g., the mean to describe the average score)
- The variability of the data (e.g., the standard deviation to describe how spread out the scores are)
The specific calculations you can do depend on the level of measurement of your variables.
Using inferential statistics , you can:
- Make estimates about the population based on your sample data.
- Test hypotheses about a relationship between variables.
Regression and correlation tests look for associations between two or more variables, while comparison tests (such as t tests and ANOVAs ) look for differences in the outcomes of different groups.
Your choice of statistical test depends on various aspects of your research design, including the types of variables you’re dealing with and the distribution of your data.
Qualitative data analysis
In qualitative research, your data will usually be very dense with information and ideas. Instead of summing it up in numbers, you’ll need to comb through the data in detail, interpret its meanings, identify patterns, and extract the parts that are most relevant to your research question.
Two of the most common approaches to doing this are thematic analysis and discourse analysis .
Approach | Characteristics |
---|---|
Thematic analysis | |
Discourse analysis |
There are many other ways of analysing qualitative data depending on the aims of your research. To get a sense of potential approaches, try reading some qualitative research papers in your field.
A sample is a subset of individuals from a larger population. Sampling means selecting the group that you will actually collect data from in your research.
For example, if you are researching the opinions of students in your university, you could survey a sample of 100 students.
Statistical sampling allows you to test a hypothesis about the characteristics of a population. There are various sampling methods you can use to ensure that your sample is representative of the population as a whole.
Operationalisation means turning abstract conceptual ideas into measurable observations.
For example, the concept of social anxiety isn’t directly observable, but it can be operationally defined in terms of self-rating scores, behavioural avoidance of crowded places, or physical anxiety symptoms in social situations.
Before collecting data , it’s important to consider how you will operationalise the variables that you want to measure.
The research methods you use depend on the type of data you need to answer your research question .
- If you want to measure something or test a hypothesis , use quantitative methods . If you want to explore ideas, thoughts, and meanings, use qualitative methods .
- If you want to analyse a large amount of readily available data, use secondary data. If you want data specific to your purposes with control over how they are generated, collect primary data.
- If you want to establish cause-and-effect relationships between variables , use experimental methods. If you want to understand the characteristics of a research subject, use descriptive methods.
Cite this Scribbr article
If you want to cite this source, you can copy and paste the citation or click the ‘Cite this Scribbr article’ button to automatically add the citation to our free Reference Generator.
McCombes, S. (2023, March 20). Research Design | Step-by-Step Guide with Examples. Scribbr. Retrieved 9 September 2024, from https://www.scribbr.co.uk/research-methods/research-design/
Is this article helpful?
Shona McCombes
Instant insights, infinite possibilities
Experimental design: Guide, steps, examples
Last updated
27 April 2023
Reviewed by
Miroslav Damyanov
Short on time? Get an AI generated summary of this article instead
Experimental research design is a scientific framework that allows you to manipulate one or more variables while controlling the test environment.
When testing a theory or new product, it can be helpful to have a certain level of control and manipulate variables to discover different outcomes. You can use these experiments to determine cause and effect or study variable associations.
This guide explores the types of experimental design, the steps in designing an experiment, and the advantages and limitations of experimental design.
Make research less tedious
Dovetail streamlines research to help you uncover and share actionable insights
- What is experimental research design?
You can determine the relationship between each of the variables by:
Manipulating one or more independent variables (i.e., stimuli or treatments)
Applying the changes to one or more dependent variables (i.e., test groups or outcomes)
With the ability to analyze the relationship between variables and using measurable data, you can increase the accuracy of the result.
What is a good experimental design?
A good experimental design requires:
Significant planning to ensure control over the testing environment
Sound experimental treatments
Properly assigning subjects to treatment groups
Without proper planning, unexpected external variables can alter an experiment's outcome.
To meet your research goals, your experimental design should include these characteristics:
Provide unbiased estimates of inputs and associated uncertainties
Enable the researcher to detect differences caused by independent variables
Include a plan for analysis and reporting of the results
Provide easily interpretable results with specific conclusions
What's the difference between experimental and quasi-experimental design?
The major difference between experimental and quasi-experimental design is the random assignment of subjects to groups.
A true experiment relies on certain controls. Typically, the researcher designs the treatment and randomly assigns subjects to control and treatment groups.
However, these conditions are unethical or impossible to achieve in some situations.
When it's unethical or impractical to assign participants randomly, that’s when a quasi-experimental design comes in.
This design allows researchers to conduct a similar experiment by assigning subjects to groups based on non-random criteria.
Another type of quasi-experimental design might occur when the researcher doesn't have control over the treatment but studies pre-existing groups after they receive different treatments.
When can a researcher conduct experimental research?
Various settings and professions can use experimental research to gather information and observe behavior in controlled settings.
Basically, a researcher can conduct experimental research any time they want to test a theory with variable and dependent controls.
Experimental research is an option when the project includes an independent variable and a desire to understand the relationship between cause and effect.
- The importance of experimental research design
Experimental research enables researchers to conduct studies that provide specific, definitive answers to questions and hypotheses.
Researchers can test Independent variables in controlled settings to:
Test the effectiveness of a new medication
Design better products for consumers
Answer questions about human health and behavior
Developing a quality research plan means a researcher can accurately answer vital research questions with minimal error. As a result, definitive conclusions can influence the future of the independent variable.
Types of experimental research designs
There are three main types of experimental research design. The research type you use will depend on the criteria of your experiment, your research budget, and environmental limitations.
Pre-experimental research design
A pre-experimental research study is a basic observational study that monitors independent variables’ effects.
During research, you observe one or more groups after applying a treatment to test whether the treatment causes any change.
The three subtypes of pre-experimental research design are:
One-shot case study research design
This research method introduces a single test group to a single stimulus to study the results at the end of the application.
After researchers presume the stimulus or treatment has caused changes, they gather results to determine how it affects the test subjects.
One-group pretest-posttest design
This method uses a single test group but includes a pretest study as a benchmark. The researcher applies a test before and after the group’s exposure to a specific stimulus.
Static group comparison design
This method includes two or more groups, enabling the researcher to use one group as a control. They apply a stimulus to one group and leave the other group static.
A posttest study compares the results among groups.
True experimental research design
A true experiment is the most common research method. It involves statistical analysis to prove or disprove a specific hypothesis .
Under completely experimental conditions, researchers expose participants in two or more randomized groups to different stimuli.
Random selection removes any potential for bias, providing more reliable results.
These are the three main sub-groups of true experimental research design:
Posttest-only control group design
This structure requires the researcher to divide participants into two random groups. One group receives no stimuli and acts as a control while the other group experiences stimuli.
Researchers perform a test at the end of the experiment to observe the stimuli exposure results.
Pretest-posttest control group design
This test also requires two groups. It includes a pretest as a benchmark before introducing the stimulus.
The pretest introduces multiple ways to test subjects. For instance, if the control group also experiences a change, it reveals that taking the test twice changes the results.
Solomon four-group design
This structure divides subjects into two groups, with two as control groups. Researchers assign the first control group a posttest only and the second control group a pretest and a posttest.
The two variable groups mirror the control groups, but researchers expose them to stimuli. The ability to differentiate between groups in multiple ways provides researchers with more testing approaches for data-based conclusions.
Quasi-experimental research design
Although closely related to a true experiment, quasi-experimental research design differs in approach and scope.
Quasi-experimental research design doesn’t have randomly selected participants. Researchers typically divide the groups in this research by pre-existing differences.
Quasi-experimental research is more common in educational studies, nursing, or other research projects where it's not ethical or practical to use randomized subject groups.
- 5 steps for designing an experiment
Experimental research requires a clearly defined plan to outline the research parameters and expected goals.
Here are five key steps in designing a successful experiment:
Step 1: Define variables and their relationship
Your experiment should begin with a question: What are you hoping to learn through your experiment?
The relationship between variables in your study will determine your answer.
Define the independent variable (the intended stimuli) and the dependent variable (the expected effect of the stimuli). After identifying these groups, consider how you might control them in your experiment.
Could natural variations affect your research? If so, your experiment should include a pretest and posttest.
Step 2: Develop a specific, testable hypothesis
With a firm understanding of the system you intend to study, you can write a specific, testable hypothesis.
What is the expected outcome of your study?
Develop a prediction about how the independent variable will affect the dependent variable.
How will the stimuli in your experiment affect your test subjects?
Your hypothesis should provide a prediction of the answer to your research question .
Step 3: Design experimental treatments to manipulate your independent variable
Depending on your experiment, your variable may be a fixed stimulus (like a medical treatment) or a variable stimulus (like a period during which an activity occurs).
Determine which type of stimulus meets your experiment’s needs and how widely or finely to vary your stimuli.
Step 4: Assign subjects to groups
When you have a clear idea of how to carry out your experiment, you can determine how to assemble test groups for an accurate study.
When choosing your study groups, consider:
The size of your experiment
Whether you can select groups randomly
Your target audience for the outcome of the study
You should be able to create groups with an equal number of subjects and include subjects that match your target audience. Remember, you should assign one group as a control and use one or more groups to study the effects of variables.
Step 5: Plan how to measure your dependent variable
This step determines how you'll collect data to determine the study's outcome. You should seek reliable and valid measurements that minimize research bias or error.
You can measure some data with scientific tools, while you’ll need to operationalize other forms to turn them into measurable observations.
- Advantages of experimental research
Experimental research is an integral part of our world. It allows researchers to conduct experiments that answer specific questions.
While researchers use many methods to conduct different experiments, experimental research offers these distinct benefits:
Researchers can determine cause and effect by manipulating variables.
It gives researchers a high level of control.
Researchers can test multiple variables within a single experiment.
All industries and fields of knowledge can use it.
Researchers can duplicate results to promote the validity of the study .
Replicating natural settings rapidly means immediate research.
Researchers can combine it with other research methods.
It provides specific conclusions about the validity of a product, theory, or idea.
- Disadvantages (or limitations) of experimental research
Unfortunately, no research type yields ideal conditions or perfect results.
While experimental research might be the right choice for some studies, certain conditions could render experiments useless or even dangerous.
Before conducting experimental research, consider these disadvantages and limitations:
Required professional qualification
Only competent professionals with an academic degree and specific training are qualified to conduct rigorous experimental research. This ensures results are unbiased and valid.
Limited scope
Experimental research may not capture the complexity of some phenomena, such as social interactions or cultural norms. These are difficult to control in a laboratory setting.
Resource-intensive
Experimental research can be expensive, time-consuming, and require significant resources, such as specialized equipment or trained personnel.
Limited generalizability
The controlled nature means the research findings may not fully apply to real-world situations or people outside the experimental setting.
Practical or ethical concerns
Some experiments may involve manipulating variables that could harm participants or violate ethical guidelines .
Researchers must ensure their experiments do not cause harm or discomfort to participants.
Sometimes, recruiting a sample of people to randomly assign may be difficult.
- Experimental research design example
Experiments across all industries and research realms provide scientists, developers, and other researchers with definitive answers. These experiments can solve problems, create inventions, and heal illnesses.
Product design testing is an excellent example of experimental research.
A company in the product development phase creates multiple prototypes for testing. With a randomized selection, researchers introduce each test group to a different prototype.
When groups experience different product designs , the company can assess which option most appeals to potential customers.
Experimental research design provides researchers with a controlled environment to conduct experiments that evaluate cause and effect.
Using the five steps to develop a research plan ensures you anticipate and eliminate external variables while answering life’s crucial questions.
Should you be using a customer insights hub?
Do you want to discover previous research faster?
Do you share your research findings with others?
Do you analyze research data?
Start for free today, add your research, and get to key insights faster
Editor’s picks
Last updated: 18 April 2023
Last updated: 27 February 2023
Last updated: 22 August 2024
Last updated: 5 February 2023
Last updated: 16 August 2024
Last updated: 9 March 2023
Last updated: 30 April 2024
Last updated: 12 December 2023
Last updated: 11 March 2024
Last updated: 4 July 2024
Last updated: 6 March 2024
Last updated: 5 March 2024
Last updated: 13 May 2024
Latest articles
Related topics, .css-je19u9{-webkit-align-items:flex-end;-webkit-box-align:flex-end;-ms-flex-align:flex-end;align-items:flex-end;display:-webkit-box;display:-webkit-flex;display:-ms-flexbox;display:flex;-webkit-flex-direction:row;-ms-flex-direction:row;flex-direction:row;-webkit-box-flex-wrap:wrap;-webkit-flex-wrap:wrap;-ms-flex-wrap:wrap;flex-wrap:wrap;-webkit-box-pack:center;-ms-flex-pack:center;-webkit-justify-content:center;justify-content:center;row-gap:0;text-align:center;max-width:671px;}@media (max-width: 1079px){.css-je19u9{max-width:400px;}.css-je19u9>span{white-space:pre;}}@media (max-width: 799px){.css-je19u9{max-width:400px;}.css-je19u9>span{white-space:pre;}} decide what to .css-1kiodld{max-height:56px;display:-webkit-box;display:-webkit-flex;display:-ms-flexbox;display:flex;-webkit-align-items:center;-webkit-box-align:center;-ms-flex-align:center;align-items:center;}@media (max-width: 1079px){.css-1kiodld{display:none;}} build next, decide what to build next.
- Types of experimental
Log in or sign up
Get started for free

Want to create or adapt books like this? Learn more about how Pressbooks supports open publishing practices.
6.2 Experimental Design
Learning objectives.
- Explain the difference between between-subjects and within-subjects experiments, list some of the pros and cons of each approach, and decide which approach to use to answer a particular research question.
- Define random assignment, distinguish it from random sampling, explain its purpose in experimental research, and use some simple strategies to implement it.
- Define what a control condition is, explain its purpose in research on treatment effectiveness, and describe some alternative types of control conditions.
- Define several types of carryover effect, give examples of each, and explain how counterbalancing helps to deal with them.
In this section, we look at some different ways to design an experiment. The primary distinction we will make is between approaches in which each participant experiences one level of the independent variable and approaches in which each participant experiences all levels of the independent variable. The former are called between-subjects experiments and the latter are called within-subjects experiments.
Between-Subjects Experiments
In a between-subjects experiment , each participant is tested in only one condition. For example, a researcher with a sample of 100 college students might assign half of them to write about a traumatic event and the other half write about a neutral event. Or a researcher with a sample of 60 people with severe agoraphobia (fear of open spaces) might assign 20 of them to receive each of three different treatments for that disorder. It is essential in a between-subjects experiment that the researcher assign participants to conditions so that the different groups are, on average, highly similar to each other. Those in a trauma condition and a neutral condition, for example, should include a similar proportion of men and women, and they should have similar average intelligence quotients (IQs), similar average levels of motivation, similar average numbers of health problems, and so on. This is a matter of controlling these extraneous participant variables across conditions so that they do not become confounding variables.
Random Assignment
The primary way that researchers accomplish this kind of control of extraneous variables across conditions is called random assignment , which means using a random process to decide which participants are tested in which conditions. Do not confuse random assignment with random sampling. Random sampling is a method for selecting a sample from a population, and it is rarely used in psychological research. Random assignment is a method for assigning participants in a sample to the different conditions, and it is an important element of all experimental research in psychology and other fields too.
In its strictest sense, random assignment should meet two criteria. One is that each participant has an equal chance of being assigned to each condition (e.g., a 50% chance of being assigned to each of two conditions). The second is that each participant is assigned to a condition independently of other participants. Thus one way to assign participants to two conditions would be to flip a coin for each one. If the coin lands heads, the participant is assigned to Condition A, and if it lands tails, the participant is assigned to Condition B. For three conditions, one could use a computer to generate a random integer from 1 to 3 for each participant. If the integer is 1, the participant is assigned to Condition A; if it is 2, the participant is assigned to Condition B; and if it is 3, the participant is assigned to Condition C. In practice, a full sequence of conditions—one for each participant expected to be in the experiment—is usually created ahead of time, and each new participant is assigned to the next condition in the sequence as he or she is tested. When the procedure is computerized, the computer program often handles the random assignment.
One problem with coin flipping and other strict procedures for random assignment is that they are likely to result in unequal sample sizes in the different conditions. Unequal sample sizes are generally not a serious problem, and you should never throw away data you have already collected to achieve equal sample sizes. However, for a fixed number of participants, it is statistically most efficient to divide them into equal-sized groups. It is standard practice, therefore, to use a kind of modified random assignment that keeps the number of participants in each group as similar as possible. One approach is block randomization . In block randomization, all the conditions occur once in the sequence before any of them is repeated. Then they all occur again before any of them is repeated again. Within each of these “blocks,” the conditions occur in a random order. Again, the sequence of conditions is usually generated before any participants are tested, and each new participant is assigned to the next condition in the sequence. Table 6.2 “Block Randomization Sequence for Assigning Nine Participants to Three Conditions” shows such a sequence for assigning nine participants to three conditions. The Research Randomizer website ( http://www.randomizer.org ) will generate block randomization sequences for any number of participants and conditions. Again, when the procedure is computerized, the computer program often handles the block randomization.
Table 6.2 Block Randomization Sequence for Assigning Nine Participants to Three Conditions
Participant | Condition |
---|---|
4 | B |
5 | C |
6 | A |
Random assignment is not guaranteed to control all extraneous variables across conditions. It is always possible that just by chance, the participants in one condition might turn out to be substantially older, less tired, more motivated, or less depressed on average than the participants in another condition. However, there are some reasons that this is not a major concern. One is that random assignment works better than one might expect, especially for large samples. Another is that the inferential statistics that researchers use to decide whether a difference between groups reflects a difference in the population takes the “fallibility” of random assignment into account. Yet another reason is that even if random assignment does result in a confounding variable and therefore produces misleading results, this is likely to be detected when the experiment is replicated. The upshot is that random assignment to conditions—although not infallible in terms of controlling extraneous variables—is always considered a strength of a research design.
Treatment and Control Conditions
Between-subjects experiments are often used to determine whether a treatment works. In psychological research, a treatment is any intervention meant to change people’s behavior for the better. This includes psychotherapies and medical treatments for psychological disorders but also interventions designed to improve learning, promote conservation, reduce prejudice, and so on. To determine whether a treatment works, participants are randomly assigned to either a treatment condition , in which they receive the treatment, or a control condition , in which they do not receive the treatment. If participants in the treatment condition end up better off than participants in the control condition—for example, they are less depressed, learn faster, conserve more, express less prejudice—then the researcher can conclude that the treatment works. In research on the effectiveness of psychotherapies and medical treatments, this type of experiment is often called a randomized clinical trial .
There are different types of control conditions. In a no-treatment control condition , participants receive no treatment whatsoever. One problem with this approach, however, is the existence of placebo effects. A placebo is a simulated treatment that lacks any active ingredient or element that should make it effective, and a placebo effect is a positive effect of such a treatment. Many folk remedies that seem to work—such as eating chicken soup for a cold or placing soap under the bedsheets to stop nighttime leg cramps—are probably nothing more than placebos. Although placebo effects are not well understood, they are probably driven primarily by people’s expectations that they will improve. Having the expectation to improve can result in reduced stress, anxiety, and depression, which can alter perceptions and even improve immune system functioning (Price, Finniss, & Benedetti, 2008).
Placebo effects are interesting in their own right (see Note 6.28 “The Powerful Placebo” ), but they also pose a serious problem for researchers who want to determine whether a treatment works. Figure 6.2 “Hypothetical Results From a Study Including Treatment, No-Treatment, and Placebo Conditions” shows some hypothetical results in which participants in a treatment condition improved more on average than participants in a no-treatment control condition. If these conditions (the two leftmost bars in Figure 6.2 “Hypothetical Results From a Study Including Treatment, No-Treatment, and Placebo Conditions” ) were the only conditions in this experiment, however, one could not conclude that the treatment worked. It could be instead that participants in the treatment group improved more because they expected to improve, while those in the no-treatment control condition did not.
Figure 6.2 Hypothetical Results From a Study Including Treatment, No-Treatment, and Placebo Conditions

Fortunately, there are several solutions to this problem. One is to include a placebo control condition , in which participants receive a placebo that looks much like the treatment but lacks the active ingredient or element thought to be responsible for the treatment’s effectiveness. When participants in a treatment condition take a pill, for example, then those in a placebo control condition would take an identical-looking pill that lacks the active ingredient in the treatment (a “sugar pill”). In research on psychotherapy effectiveness, the placebo might involve going to a psychotherapist and talking in an unstructured way about one’s problems. The idea is that if participants in both the treatment and the placebo control groups expect to improve, then any improvement in the treatment group over and above that in the placebo control group must have been caused by the treatment and not by participants’ expectations. This is what is shown by a comparison of the two outer bars in Figure 6.2 “Hypothetical Results From a Study Including Treatment, No-Treatment, and Placebo Conditions” .
Of course, the principle of informed consent requires that participants be told that they will be assigned to either a treatment or a placebo control condition—even though they cannot be told which until the experiment ends. In many cases the participants who had been in the control condition are then offered an opportunity to have the real treatment. An alternative approach is to use a waitlist control condition , in which participants are told that they will receive the treatment but must wait until the participants in the treatment condition have already received it. This allows researchers to compare participants who have received the treatment with participants who are not currently receiving it but who still expect to improve (eventually). A final solution to the problem of placebo effects is to leave out the control condition completely and compare any new treatment with the best available alternative treatment. For example, a new treatment for simple phobia could be compared with standard exposure therapy. Because participants in both conditions receive a treatment, their expectations about improvement should be similar. This approach also makes sense because once there is an effective treatment, the interesting question about a new treatment is not simply “Does it work?” but “Does it work better than what is already available?”
The Powerful Placebo
Many people are not surprised that placebos can have a positive effect on disorders that seem fundamentally psychological, including depression, anxiety, and insomnia. However, placebos can also have a positive effect on disorders that most people think of as fundamentally physiological. These include asthma, ulcers, and warts (Shapiro & Shapiro, 1999). There is even evidence that placebo surgery—also called “sham surgery”—can be as effective as actual surgery.
Medical researcher J. Bruce Moseley and his colleagues conducted a study on the effectiveness of two arthroscopic surgery procedures for osteoarthritis of the knee (Moseley et al., 2002). The control participants in this study were prepped for surgery, received a tranquilizer, and even received three small incisions in their knees. But they did not receive the actual arthroscopic surgical procedure. The surprising result was that all participants improved in terms of both knee pain and function, and the sham surgery group improved just as much as the treatment groups. According to the researchers, “This study provides strong evidence that arthroscopic lavage with or without débridement [the surgical procedures used] is not better than and appears to be equivalent to a placebo procedure in improving knee pain and self-reported function” (p. 85).

Research has shown that patients with osteoarthritis of the knee who receive a “sham surgery” experience reductions in pain and improvement in knee function similar to those of patients who receive a real surgery.
Army Medicine – Surgery – CC BY 2.0.
Within-Subjects Experiments
In a within-subjects experiment , each participant is tested under all conditions. Consider an experiment on the effect of a defendant’s physical attractiveness on judgments of his guilt. Again, in a between-subjects experiment, one group of participants would be shown an attractive defendant and asked to judge his guilt, and another group of participants would be shown an unattractive defendant and asked to judge his guilt. In a within-subjects experiment, however, the same group of participants would judge the guilt of both an attractive and an unattractive defendant.
The primary advantage of this approach is that it provides maximum control of extraneous participant variables. Participants in all conditions have the same mean IQ, same socioeconomic status, same number of siblings, and so on—because they are the very same people. Within-subjects experiments also make it possible to use statistical procedures that remove the effect of these extraneous participant variables on the dependent variable and therefore make the data less “noisy” and the effect of the independent variable easier to detect. We will look more closely at this idea later in the book.
Carryover Effects and Counterbalancing
The primary disadvantage of within-subjects designs is that they can result in carryover effects. A carryover effect is an effect of being tested in one condition on participants’ behavior in later conditions. One type of carryover effect is a practice effect , where participants perform a task better in later conditions because they have had a chance to practice it. Another type is a fatigue effect , where participants perform a task worse in later conditions because they become tired or bored. Being tested in one condition can also change how participants perceive stimuli or interpret their task in later conditions. This is called a context effect . For example, an average-looking defendant might be judged more harshly when participants have just judged an attractive defendant than when they have just judged an unattractive defendant. Within-subjects experiments also make it easier for participants to guess the hypothesis. For example, a participant who is asked to judge the guilt of an attractive defendant and then is asked to judge the guilt of an unattractive defendant is likely to guess that the hypothesis is that defendant attractiveness affects judgments of guilt. This could lead the participant to judge the unattractive defendant more harshly because he thinks this is what he is expected to do. Or it could make participants judge the two defendants similarly in an effort to be “fair.”
Carryover effects can be interesting in their own right. (Does the attractiveness of one person depend on the attractiveness of other people that we have seen recently?) But when they are not the focus of the research, carryover effects can be problematic. Imagine, for example, that participants judge the guilt of an attractive defendant and then judge the guilt of an unattractive defendant. If they judge the unattractive defendant more harshly, this might be because of his unattractiveness. But it could be instead that they judge him more harshly because they are becoming bored or tired. In other words, the order of the conditions is a confounding variable. The attractive condition is always the first condition and the unattractive condition the second. Thus any difference between the conditions in terms of the dependent variable could be caused by the order of the conditions and not the independent variable itself.
There is a solution to the problem of order effects, however, that can be used in many situations. It is counterbalancing , which means testing different participants in different orders. For example, some participants would be tested in the attractive defendant condition followed by the unattractive defendant condition, and others would be tested in the unattractive condition followed by the attractive condition. With three conditions, there would be six different orders (ABC, ACB, BAC, BCA, CAB, and CBA), so some participants would be tested in each of the six orders. With counterbalancing, participants are assigned to orders randomly, using the techniques we have already discussed. Thus random assignment plays an important role in within-subjects designs just as in between-subjects designs. Here, instead of randomly assigning to conditions, they are randomly assigned to different orders of conditions. In fact, it can safely be said that if a study does not involve random assignment in one form or another, it is not an experiment.
There are two ways to think about what counterbalancing accomplishes. One is that it controls the order of conditions so that it is no longer a confounding variable. Instead of the attractive condition always being first and the unattractive condition always being second, the attractive condition comes first for some participants and second for others. Likewise, the unattractive condition comes first for some participants and second for others. Thus any overall difference in the dependent variable between the two conditions cannot have been caused by the order of conditions. A second way to think about what counterbalancing accomplishes is that if there are carryover effects, it makes it possible to detect them. One can analyze the data separately for each order to see whether it had an effect.
When 9 Is “Larger” Than 221
Researcher Michael Birnbaum has argued that the lack of context provided by between-subjects designs is often a bigger problem than the context effects created by within-subjects designs. To demonstrate this, he asked one group of participants to rate how large the number 9 was on a 1-to-10 rating scale and another group to rate how large the number 221 was on the same 1-to-10 rating scale (Birnbaum, 1999). Participants in this between-subjects design gave the number 9 a mean rating of 5.13 and the number 221 a mean rating of 3.10. In other words, they rated 9 as larger than 221! According to Birnbaum, this is because participants spontaneously compared 9 with other one-digit numbers (in which case it is relatively large) and compared 221 with other three-digit numbers (in which case it is relatively small).
Simultaneous Within-Subjects Designs
So far, we have discussed an approach to within-subjects designs in which participants are tested in one condition at a time. There is another approach, however, that is often used when participants make multiple responses in each condition. Imagine, for example, that participants judge the guilt of 10 attractive defendants and 10 unattractive defendants. Instead of having people make judgments about all 10 defendants of one type followed by all 10 defendants of the other type, the researcher could present all 20 defendants in a sequence that mixed the two types. The researcher could then compute each participant’s mean rating for each type of defendant. Or imagine an experiment designed to see whether people with social anxiety disorder remember negative adjectives (e.g., “stupid,” “incompetent”) better than positive ones (e.g., “happy,” “productive”). The researcher could have participants study a single list that includes both kinds of words and then have them try to recall as many words as possible. The researcher could then count the number of each type of word that was recalled. There are many ways to determine the order in which the stimuli are presented, but one common way is to generate a different random order for each participant.
Between-Subjects or Within-Subjects?
Almost every experiment can be conducted using either a between-subjects design or a within-subjects design. This means that researchers must choose between the two approaches based on their relative merits for the particular situation.
Between-subjects experiments have the advantage of being conceptually simpler and requiring less testing time per participant. They also avoid carryover effects without the need for counterbalancing. Within-subjects experiments have the advantage of controlling extraneous participant variables, which generally reduces noise in the data and makes it easier to detect a relationship between the independent and dependent variables.
A good rule of thumb, then, is that if it is possible to conduct a within-subjects experiment (with proper counterbalancing) in the time that is available per participant—and you have no serious concerns about carryover effects—this is probably the best option. If a within-subjects design would be difficult or impossible to carry out, then you should consider a between-subjects design instead. For example, if you were testing participants in a doctor’s waiting room or shoppers in line at a grocery store, you might not have enough time to test each participant in all conditions and therefore would opt for a between-subjects design. Or imagine you were trying to reduce people’s level of prejudice by having them interact with someone of another race. A within-subjects design with counterbalancing would require testing some participants in the treatment condition first and then in a control condition. But if the treatment works and reduces people’s level of prejudice, then they would no longer be suitable for testing in the control condition. This is true for many designs that involve a treatment meant to produce long-term change in participants’ behavior (e.g., studies testing the effectiveness of psychotherapy). Clearly, a between-subjects design would be necessary here.
Remember also that using one type of design does not preclude using the other type in a different study. There is no reason that a researcher could not use both a between-subjects design and a within-subjects design to answer the same research question. In fact, professional researchers often do exactly this.
Key Takeaways
- Experiments can be conducted using either between-subjects or within-subjects designs. Deciding which to use in a particular situation requires careful consideration of the pros and cons of each approach.
- Random assignment to conditions in between-subjects experiments or to orders of conditions in within-subjects experiments is a fundamental element of experimental research. Its purpose is to control extraneous variables so that they do not become confounding variables.
- Experimental research on the effectiveness of a treatment requires both a treatment condition and a control condition, which can be a no-treatment control condition, a placebo control condition, or a waitlist control condition. Experimental treatments can also be compared with the best available alternative.
Discussion: For each of the following topics, list the pros and cons of a between-subjects and within-subjects design and decide which would be better.
- You want to test the relative effectiveness of two training programs for running a marathon.
- Using photographs of people as stimuli, you want to see if smiling people are perceived as more intelligent than people who are not smiling.
- In a field experiment, you want to see if the way a panhandler is dressed (neatly vs. sloppily) affects whether or not passersby give him any money.
- You want to see if concrete nouns (e.g., dog ) are recalled better than abstract nouns (e.g., truth ).
- Discussion: Imagine that an experiment shows that participants who receive psychodynamic therapy for a dog phobia improve more than participants in a no-treatment control group. Explain a fundamental problem with this research design and at least two ways that it might be corrected.
Birnbaum, M. H. (1999). How to show that 9 > 221: Collect judgments in a between-subjects design. Psychological Methods, 4 , 243–249.
Moseley, J. B., O’Malley, K., Petersen, N. J., Menke, T. J., Brody, B. A., Kuykendall, D. H., … Wray, N. P. (2002). A controlled trial of arthroscopic surgery for osteoarthritis of the knee. The New England Journal of Medicine, 347 , 81–88.
Price, D. D., Finniss, D. G., & Benedetti, F. (2008). A comprehensive review of the placebo effect: Recent advances and current thought. Annual Review of Psychology, 59 , 565–590.
Shapiro, A. K., & Shapiro, E. (1999). The powerful placebo: From ancient priest to modern physician . Baltimore, MD: Johns Hopkins University Press.
Research Methods in Psychology Copyright © 2016 by University of Minnesota is licensed under a Creative Commons Attribution-NonCommercial-ShareAlike 4.0 International License , except where otherwise noted.

- General & Introductory Statistics
- Survey Research Methods & Sampling

Experimental Methods in Survey Research: Techniques that Combine Random Sampling with Random Assignment
ISBN: 978-1-119-08374-0
October 2019

Paul J. Lavrakas , Michael W. Traugott , Courtney Kennedy , Allyson L. Holbrook , Edith D. de Leeuw , Brady T. West
A thorough and comprehensive guide to the theoretical, practical, and methodological approaches used in survey experiments across disciplines such as political science, health sciences, sociology, economics, psychology, and marketing
This book explores and explains the broad range of experimental designs embedded in surveys that use both probability and non-probability samples. It approaches the usage of survey-based experiments with a Total Survey Error (TSE) perspective, which provides insight on the strengths and weaknesses of the techniques used.
Experimental Methods in Survey Research: Techniques that Combine Random Sampling with Random Assignment addresses experiments on within-unit coverage, reducing nonresponse, question and questionnaire design, minimizing interview measurement bias, using adaptive design, trend data, vignettes, the analysis of data from survey experiments, and other topics, across social, behavioral, and marketing science domains.
Each chapter begins with a description of the experimental method or application and its importance, followed by reference to relevant literature. At least one detailed original experimental case study then follows to illustrate the experimental method’s deployment, implementation, and analysis from a TSE perspective. The chapters conclude with theoretical and practical implications on the usage of the experimental method addressed. In summary, this book:
- Fills a gap in the current literature by successfully combining the subjects of survey methodology and experimental methodology in an effort to maximize both internal validity and external validity
- Offers a wide range of types of experimentation in survey research with in-depth attention to their various methodologies and applications
- Is edited by internationally recognized experts in the field of survey research/methodology and in the usage of survey-based experimentation —featuring contributions from across a variety of disciplines in the social and behavioral sciences
- Presents advances in the field of survey experiments, as well as relevant references in each chapter for further study
- Includes more than 20 types of original experiments carried out within probability sample surveys
- Addresses myriad practical and operational aspects for designing, implementing, and analyzing survey-based experiments by using a Total Survey Error perspective to address the strengths and weaknesses of each experimental technique and method
Experimental Methods in Survey Research: Techniques that Combine Random Sampling with Random Assignment is an ideal reference for survey researchers and practitioners in areas such political science, health sciences, sociology, economics, psychology, public policy, data collection, data science, and marketing. It is also a very useful textbook for graduate-level courses on survey experiments and survey methodology.
Paul J. Lavrakas, PhD, is Senior Fellow at the NORC at the University of Chicago, Adjunct Professor at University of Illinois-Chicago, Senior Methodologist at the Social Research Centre of Australian National University and at the Office for Survey Research at Michigan State University.
Michael W. Traugott, PhD, is Research Professor in the Institute for Social Research at the University of Michigan.
Courtney Kennedy, PhD, is Director of Survey Research at Pew Research Center in Washington, DC.
Allyson L. Holbrook, PhD, is Professor of Public Administration and Psychology at the University of Illinois-Chicago.
Edith D. de Leeuw, PhD, is Professor of Survey Methodology in the Department of Methodology and Statistics at Utrecht University.
Brady T. West, PhD, is Research Associate Professor in the Survey Research Center at the University of Michigan-Ann Arbor.

Understanding Survey Research Designs: Experimental vs Descriptive

Table of Contents
Have you ever wondered how researchers gather data to explore trends, opinions, or behaviors among large groups of people? Survey research designs are a critical tool in the arsenal of social scientists, marketers, and policy makers. But not all surveys are created equal; they come in different formats with varying purposes. Today, let’s demystify two primary types of survey research designs : experimental and descriptive. By understanding their unique characteristics and applications, you’ll gain insights into how conclusions about our world are drawn from carefully collected data.
What is survey research design?
Before diving into the specific types, let’s clarify what we mean by survey research design. It’s a framework that guides the collection, analysis, and interpretation of data gathered through questionnaires or interviews. This design determines how a survey is conducted, the target population, the sampling method, and how results are analyzed to ensure that the information collected is relevant, reliable, and can support or refute a research hypothesis.
Experimental survey research design
In experimental survey research design s, the researcher manipulates one or more variables to observe their effect on another variable. This method is often used to establish cause-and-effect relationships. Here’s what defines an experimental design:
- Controlled manipulation of variables: The researcher introduces changes to the in dependent variable(s) to see the effects on the dependent variable(s).
- Random assignment: Participants are randomly assigned to different groups (e.g., control and experimental) to ensure that the groups are comparable.
- Comparison of groups: By comparing data from different groups, researchers can infer the impact of the manipulated variable.
Types of experimental designs
Within experimental designs, there are several subtypes, including true experiments , quasi-experiments , and pre-experimental designs . True experiments have strict control over variables and random assignment , while quasi-experiments lack random assignment. Pre-experimental designs are the least rigorous, often lacking both control and randomization.
Descriptive survey research design
Unlike experimental designs, descriptive survey research design s do not involve manipulation or control of variables. Instead, they aim to describe characteristics of a population or phenomenon as they naturally occur. Attributes of descriptive design include:
- No manipulation: The researcher observes without intervening in the natural setting.
- Focus on current status: Descriptive surveys often aim to provide a snapshot of the current state of affairs.
- Wide range of data: They can collect a vast array of data, from opinions to demographic information.
Applications of descriptive survey designs
Descriptive surveys are widely used in various fields for different purposes. They can track consumer preferences , measure employee satisfaction , or gauge public opinion on social issues. The key is that they seek to paint a picture of what exists or what people believe at a given moment in time.
Choosing the right survey design
Deciding whether to use an experimental or descriptive survey design hinges on the research question. If the goal is to determine causality , experimental designs are the go-to. However, if the objective is to describe or explore a phenomenon without altering the environment, descriptive designs are more appropriate. Considerations include:
- Research objectives: What are you trying to find out? Do you want to test a hypothesis or simply describe a situation?
- Resources available: Experimental designs often require more resources in terms of time, money, and expertise.
- Ethical considerations: Some questions may not be ethically testable in an experimental design due to the need for manipulation.
Challenges and limitations
Both experimental and descriptive survey research designs come with their own set of challenges and limitations. For experimental designs, ensuring a truly random assignment can be difficult, and external variables may still influence outcomes. Descriptive designs may suffer from biases in self-reporting and are unable to provide causal explanations.
Best practices in survey research design
To maximize the effectiveness of a survey research design, whether experimental or descriptive, researchers should adhere to best practices:
- Clear and concise questionnaire: Questions should be easily understandable and focused on the research objectives.
- Representative sampling: The sample should accurately reflect the population being studied.
- Rigorous analysis: Statistical methods should be appropriate for the data and research questions.
- Transparency: Researchers should be transparent about methodologies, challenges, and potential biases in their work.
Survey research designs are powerful tools that, when used correctly, provide valuable insights into human behavior and preferences. Whether experimental or descriptive, each design has its rightful place depending on the research question. By considering goals, resources, and ethical implications, researchers can select the design that best fits their needs, leading to more accurate and impactful findings.
What do you think? How might understanding these research designs change the way you view poll results or studies shared in the media? Can you think of a situation where one design may be more beneficial than the other?
Submit a Comment Cancel reply
Your email address will not be published. Required fields are marked *
Save my name, email, and website in this browser for the next time I comment.
Submit Comment
Research Methodologies & Methods
1 Logic of Inquiry in Social Research
- A Science of Society
- Comte’s Ideas on the Nature of Sociology
- Observation in Social Sciences
- Logical Understanding of Social Reality
2 Empirical Approach
- Empirical Approach
- Rules of Data Collection
- Cultural Relativism
- Problems Encountered in Data Collection
- Difference between Common Sense and Science
- What is Ethical?
- What is Normal?
- Understanding the Data Collected
- Managing Diversities in Social Research
- Problematising the Object of Study
- Conclusion: Return to Good Old Empirical Approach
3 Diverse Logic of Theory Building
- Concern with Theory in Sociology
- Concepts: Basic Elements of Theories
- Why Do We Need Theory?
- Hypothesis Description and Experimentation
- Controlled Experiment
- Designing an Experiment
- How to Test a Hypothesis
- Sensitivity to Alternative Explanations
- Rival Hypothesis Construction
- The Use and Scope of Social Science Theory
- Theory Building and Researcher’s Values
4 Theoretical Analysis
- Premises of Evolutionary and Functional Theories
- Critique of Evolutionary and Functional Theories
- Turning away from Functionalism
- What after Functionalism
- Post-modernism
- Trends other than Post-modernism
5 Issues of Epistemology
- Some Major Concerns of Epistemology
- Rationalism
- Phenomenology: Bracketing Experience
6 Philosophy of Social Science
- Foundations of Science
- Science, Modernity, and Sociology
- Rethinking Science
- Crisis in Foundation
7 Positivism and its Critique
- Heroic Science and Origin of Positivism
- Early Positivism
- Consolidation of Positivism
- Critiques of Positivism
8 Hermeneutics
- Methodological Disputes in the Social Sciences
- Tracing the History of Hermeneutics
- Hermeneutics and Sociology
- Philosophical Hermeneutics
- The Hermeneutics of Suspicion
- Phenomenology and Hermeneutics
9 Comparative Method
- Relationship with Common Sense; Interrogating Ideological Location
- The Historical Context
- Elements of the Comparative Approach
10 Feminist Approach
- Features of the Feminist Method
- Feminist Methods adopt the Reflexive Stance
- Feminist Discourse in India
11 Participatory Method
- Delineation of Key Features
12 Types of Research
- Basic and Applied Research
- Descriptive and Analytical Research
- Empirical and Exploratory Research
- Quantitative and Qualitative Research
- Explanatory (Causal) and Longitudinal Research
- Experimental and Evaluative Research
- Participatory Action Research
13 Methods of Research
- Evolutionary Method
- Comparative Method
- Historical Method
- Personal Documents
14 Elements of Research Design
- Structuring the Research Process
15 Sampling Methods and Estimation of Sample Size
- Classification of Sampling Methods
- Sample Size
16 Measures of Central Tendency
- Relationship between Mean, Mode, and Median
- Choosing a Measure of Central Tendency
17 Measures of Dispersion and Variability
- The Variance
- The Standard Deviation
- Coefficient of Variation
18 Statistical Inference- Tests of Hypothesis
- Statistical Inference
- Tests of Significance
19 Correlation and Regression
- Correlation
- Method of Calculating Correlation of Ungrouped Data
- Method Of Calculating Correlation Of Grouped Data
20 Survey Method
- Rationale of Survey Research Method
- History of Survey Research
- Defining Survey Research
- Sampling and Survey Techniques
- Operationalising Survey Research Tools
- Advantages and Weaknesses of Survey Research
21 Survey Design
- Preliminary Considerations
- Stages / Phases in Survey Research
- Formulation of Research Question
- Survey Research Designs
- Sampling Design
22 Survey Instrumentation
- Techniques/Instruments for Data Collection
- Questionnaire Construction
- Issues in Designing a Survey Instrument
23 Survey Execution and Data Analysis
- Problems and Issues in Executing Survey Research
- Data Analysis
- Ethical Issues in Survey Research
24 Field Research – I
- History of Field Research
- Ethnography
- Theme Selection
- Gaining Entry in the Field
- Key Informants
- Participant Observation
25 Field Research – II
- Interview its Types and Process
- Feminist and Postmodernist Perspectives on Interviewing
- Narrative Analysis
- Interpretation
- Case Study and its Types
- Life Histories
- Oral History
- PRA and RRA Techniques
26 Reliability, Validity and Triangulation
- Concepts of Reliability and Validity
- Three Types of “Reliability”
- Working Towards Reliability
- Procedural Validity
- Field Research as a Validity Check
- Method Appropriate Criteria
- Triangulation
- Ethical Considerations in Qualitative Research
27 Qualitative Data Formatting and Processing
- Qualitative Data Processing and Analysis
- Description
- Classification
- Making Connections
- Theoretical Coding
- Qualitative Content Analysis
28 Writing up Qualitative Data
- Problems of Writing Up
- Grasp and Then Render
- “Writing Down” and “Writing Up”
- Write Early
- Writing Styles
- First Draft
29 Using Internet and Word Processor
- What is Internet and How Does it Work?
- Internet Services
- Searching on the Web: Search Engines
- Accessing and Using Online Information
- Online Journals and Texts
- Statistical Reference Sites
- Data Sources
- Uses of E-mail Services in Research
30 Using SPSS for Data Analysis Contents
- Introduction
- Starting and Exiting SPSS
- Creating a Data File
- Univariate Analysis
- Bivariate Analysis
31 Using SPSS in Report Writing
- Why to Use SPSS
- Working with SPSS Output
- Copying SPSS Output to MS Word Document
32 Tabulation and Graphic Presentation- Case Studies
- Structure for Presentation of Research Findings
- Data Presentation: Editing, Coding, and Transcribing
- Case Studies
- Qualitative Data Analysis and Presentation through Software
- Types of ICT used for Research
33 Guidelines to Research Project Assignment
- Overview of Research Methodologies and Methods (MSO 002)
- Research Project Objectives
- Preparation for Research Project
- Stages of the Research Project
- Supervision During the Research Project
- Submission of Research Project
- Methodology for Evaluating Research Project
Share on Mastodon
- Skip to secondary menu
- Skip to main content
- Skip to primary sidebar
Statistics By Jim
Making statistics intuitive
Experimental Design: Definition and Types
By Jim Frost 3 Comments
What is Experimental Design?
An experimental design is a detailed plan for collecting and using data to identify causal relationships. Through careful planning, the design of experiments allows your data collection efforts to have a reasonable chance of detecting effects and testing hypotheses that answer your research questions.
An experiment is a data collection procedure that occurs in controlled conditions to identify and understand causal relationships between variables. Researchers can use many potential designs. The ultimate choice depends on their research question, resources, goals, and constraints. In some fields of study, researchers refer to experimental design as the design of experiments (DOE). Both terms are synonymous.

Ultimately, the design of experiments helps ensure that your procedures and data will evaluate your research question effectively. Without an experimental design, you might waste your efforts in a process that, for many potential reasons, can’t answer your research question. In short, it helps you trust your results.
Learn more about Independent and Dependent Variables .
Design of Experiments: Goals & Settings
Experiments occur in many settings, ranging from psychology, social sciences, medicine, physics, engineering, and industrial and service sectors. Typically, experimental goals are to discover a previously unknown effect , confirm a known effect, or test a hypothesis.
Effects represent causal relationships between variables. For example, in a medical experiment, does the new medicine cause an improvement in health outcomes? If so, the medicine has a causal effect on the outcome.
An experimental design’s focus depends on the subject area and can include the following goals:
- Understanding the relationships between variables.
- Identifying the variables that have the largest impact on the outcomes.
- Finding the input variable settings that produce an optimal result.
For example, psychologists have conducted experiments to understand how conformity affects decision-making. Sociologists have performed experiments to determine whether ethnicity affects the public reaction to staged bike thefts. These experiments map out the causal relationships between variables, and their primary goal is to understand the role of various factors.
Conversely, in a manufacturing environment, the researchers might use an experimental design to find the factors that most effectively improve their product’s strength, identify the optimal manufacturing settings, and do all that while accounting for various constraints. In short, a manufacturer’s goal is often to use experiments to improve their products cost-effectively.
In a medical experiment, the goal might be to quantify the medicine’s effect and find the optimum dosage.
Developing an Experimental Design
Developing an experimental design involves planning that maximizes the potential to collect data that is both trustworthy and able to detect causal relationships. Specifically, these studies aim to see effects when they exist in the population the researchers are studying, preferentially favor causal effects, isolate each factor’s true effect from potential confounders, and produce conclusions that you can generalize to the real world.
To accomplish these goals, experimental designs carefully manage data validity and reliability , and internal and external experimental validity. When your experiment is valid and reliable, you can expect your procedures and data to produce trustworthy results.
An excellent experimental design involves the following:
- Lots of preplanning.
- Developing experimental treatments.
- Determining how to assign subjects to treatment groups.
The remainder of this article focuses on how experimental designs incorporate these essential items to accomplish their research goals.
Learn more about Data Reliability vs. Validity and Internal and External Experimental Validity .
Preplanning, Defining, and Operationalizing for Design of Experiments

This phase of the design of experiments helps you identify critical variables, know how to measure them while ensuring reliability and validity, and understand the relationships between them. The review can also help you find ways to reduce sources of variability, which increases your ability to detect treatment effects. Notably, the literature review allows you to learn how similar studies designed their experiments and the challenges they faced.
Operationalizing a study involves taking your research question, using the background information you gathered, and formulating an actionable plan.
This process should produce a specific and testable hypothesis using data that you can reasonably collect given the resources available to the experiment.
- Null hypothesis : The jumping exercise intervention does not affect bone density.
- Alternative hypothesis : The jumping exercise intervention affects bone density.
To learn more about this early phase, read Five Steps for Conducting Scientific Studies with Statistical Analyses .
Formulating Treatments in Experimental Designs
In an experimental design, treatments are variables that the researchers control. They are the primary independent variables of interest. Researchers administer the treatment to the subjects or items in the experiment and want to know whether it causes changes in the outcome.
As the name implies, a treatment can be medical in nature, such as a new medicine or vaccine. But it’s a general term that applies to other things such as training programs, manufacturing settings, teaching methods, and types of fertilizers. I helped run an experiment where the treatment was a jumping exercise intervention that we hoped would increase bone density. All these treatment examples are things that potentially influence a measurable outcome.
Even when you know your treatment generally, you must carefully consider the amount. How large of a dose? If you’re comparing three different temperatures in a manufacturing process, how far apart are they? For my bone mineral density study, we had to determine how frequently the exercise sessions would occur and how long each lasted.
How you define the treatments in the design of experiments can affect your findings and the generalizability of your results.
Assigning Subjects to Experimental Groups
A crucial decision for all experimental designs is determining how researchers assign subjects to the experimental conditions—the treatment and control groups. The control group is often, but not always, the lack of a treatment. It serves as a basis for comparison by showing outcomes for subjects who don’t receive a treatment. Learn more about Control Groups .
How your experimental design assigns subjects to the groups affects how confident you can be that the findings represent true causal effects rather than mere correlation caused by confounders. Indeed, the assignment method influences how you control for confounding variables. This is the difference between correlation and causation .
Imagine a study finds that vitamin consumption correlates with better health outcomes. As a researcher, you want to be able to say that vitamin consumption causes the improvements. However, with the wrong experimental design, you might only be able to say there is an association. A confounder, and not the vitamins, might actually cause the health benefits.
Let’s explore some of the ways to assign subjects in design of experiments.
Completely Randomized Designs
A completely randomized experimental design randomly assigns all subjects to the treatment and control groups. You simply take each participant and use a random process to determine their group assignment. You can flip coins, roll a die, or use a computer. Randomized experiments must be prospective studies because they need to be able to control group assignment.
Random assignment in the design of experiments helps ensure that the groups are roughly equivalent at the beginning of the study. This equivalence at the start increases your confidence that any differences you see at the end were caused by the treatments. The randomization tends to equalize confounders between the experimental groups and, thereby, cancels out their effects, leaving only the treatment effects.
For example, in a vitamin study, the researchers can randomly assign participants to either the control or vitamin group. Because the groups are approximately equal when the experiment starts, if the health outcomes are different at the end of the study, the researchers can be confident that the vitamins caused those improvements.
Statisticians consider randomized experimental designs to be the best for identifying causal relationships.
If you can’t randomly assign subjects but want to draw causal conclusions about an intervention, consider using a quasi-experimental design .
Learn more about Randomized Controlled Trials and Random Assignment in Experiments .
Randomized Block Designs
Nuisance factors are variables that can affect the outcome, but they are not the researcher’s primary interest. Unfortunately, they can hide or distort the treatment results. When experimenters know about specific nuisance factors, they can use a randomized block design to minimize their impact.
This experimental design takes subjects with a shared “nuisance” characteristic and groups them into blocks. The participants in each block are then randomly assigned to the experimental groups. This process allows the experiment to control for known nuisance factors.
Blocking in the design of experiments reduces the impact of nuisance factors on experimental error. The analysis assesses the effects of the treatment within each block, which removes the variability between blocks. The result is that blocked experimental designs can reduce the impact of nuisance variables, increasing the ability to detect treatment effects accurately.
Suppose you’re testing various teaching methods. Because grade level likely affects educational outcomes, you might use grade level as a blocking factor. To use a randomized block design for this scenario, divide the participants by grade level and then randomly assign the members of each grade level to the experimental groups.
A standard guideline for an experimental design is to “Block what you can, randomize what you cannot.” Use blocking for a few primary nuisance factors. Then use random assignment to distribute the unblocked nuisance factors equally between the experimental conditions.
You can also use covariates to control nuisance factors. Learn about Covariates: Definition and Uses .
Observational Studies
In some experimental designs, randomly assigning subjects to the experimental conditions is impossible or unethical. The researchers simply can’t assign participants to the experimental groups. However, they can observe them in their natural groupings, measure the essential variables, and look for correlations. These observational studies are also known as quasi-experimental designs. Retrospective studies must be observational in nature because they look back at past events.
Imagine you’re studying the effects of depression on an activity. Clearly, you can’t randomly assign participants to the depression and control groups. But you can observe participants with and without depression and see how their task performance differs.
Observational studies let you perform research when you can’t control the treatment. However, quasi-experimental designs increase the problem of confounding variables. For this design of experiments, correlation does not necessarily imply causation. While special procedures can help control confounders in an observational study, you’re ultimately less confident that the results represent causal findings.
Learn more about Observational Studies .
For a good comparison, learn about the differences and tradeoffs between Observational Studies and Randomized Experiments .
Between-Subjects vs. Within-Subjects Experimental Designs
When you think of the design of experiments, you probably picture a treatment and control group. Researchers assign participants to only one of these groups, so each group contains entirely different subjects than the other groups. Analysts compare the groups at the end of the experiment. Statisticians refer to this method as a between-subjects, or independent measures, experimental design.
In a between-subjects design , you can have more than one treatment group, but each subject is exposed to only one condition, the control group or one of the treatment groups.
A potential downside to this approach is that differences between groups at the beginning can affect the results at the end. As you’ve read earlier, random assignment can reduce those differences, but it is imperfect. There will always be some variability between the groups.
In a within-subjects experimental design , also known as repeated measures, subjects experience all treatment conditions and are measured for each. Each subject acts as their own control, which reduces variability and increases the statistical power to detect effects.
In this experimental design, you minimize pre-existing differences between the experimental conditions because they all contain the same subjects. However, the order of treatments can affect the results. Beware of practice and fatigue effects. Learn more about Repeated Measures Designs .
Assigned to one experimental condition | Participates in all experimental conditions |
Requires more subjects | Fewer subjects |
Differences between subjects in the groups can affect the results | Uses same subjects in all conditions. |
No order of treatment effects. | Order of treatments can affect results. |
Design of Experiments Examples
For example, a bone density study has three experimental groups—a control group, a stretching exercise group, and a jumping exercise group.
In a between-subjects experimental design, scientists randomly assign each participant to one of the three groups.
In a within-subjects design, all subjects experience the three conditions sequentially while the researchers measure bone density repeatedly. The procedure can switch the order of treatments for the participants to help reduce order effects.
Matched Pairs Experimental Design
A matched pairs experimental design is a between-subjects study that uses pairs of similar subjects. Researchers use this approach to reduce pre-existing differences between experimental groups. It’s yet another design of experiments method for reducing sources of variability.
Researchers identify variables likely to affect the outcome, such as demographics. When they pick a subject with a set of characteristics, they try to locate another participant with similar attributes to create a matched pair. Scientists randomly assign one member of a pair to the treatment group and the other to the control group.
On the plus side, this process creates two similar groups, and it doesn’t create treatment order effects. While matched pairs do not produce the perfectly matched groups of a within-subjects design (which uses the same subjects in all conditions), it aims to reduce variability between groups relative to a between-subjects study.
On the downside, finding matched pairs is very time-consuming. Additionally, if one member of a matched pair drops out, the other subject must leave the study too.
Learn more about Matched Pairs Design: Uses & Examples .
Another consideration is whether you’ll use a cross-sectional design (one point in time) or use a longitudinal study to track changes over time .
A case study is a research method that often serves as a precursor to a more rigorous experimental design by identifying research questions, variables, and hypotheses to test. Learn more about What is a Case Study? Definition & Examples .
In conclusion, the design of experiments is extremely sensitive to subject area concerns and the time and resources available to the researchers. Developing a suitable experimental design requires balancing a multitude of considerations. A successful design is necessary to obtain trustworthy answers to your research question and to have a reasonable chance of detecting treatment effects when they exist.
Share this:

Reader Interactions
March 23, 2024 at 2:35 pm
Dear Jim You wrote a superb document, I will use it in my Buistatistics course, along with your three books. Thank you very much! Miguel
March 23, 2024 at 5:43 pm
Thanks so much, Miguel! Glad this post was helpful and I trust the books will be as well.
April 10, 2023 at 4:36 am
What are the purpose and uses of experimental research design?
Comments and Questions Cancel reply
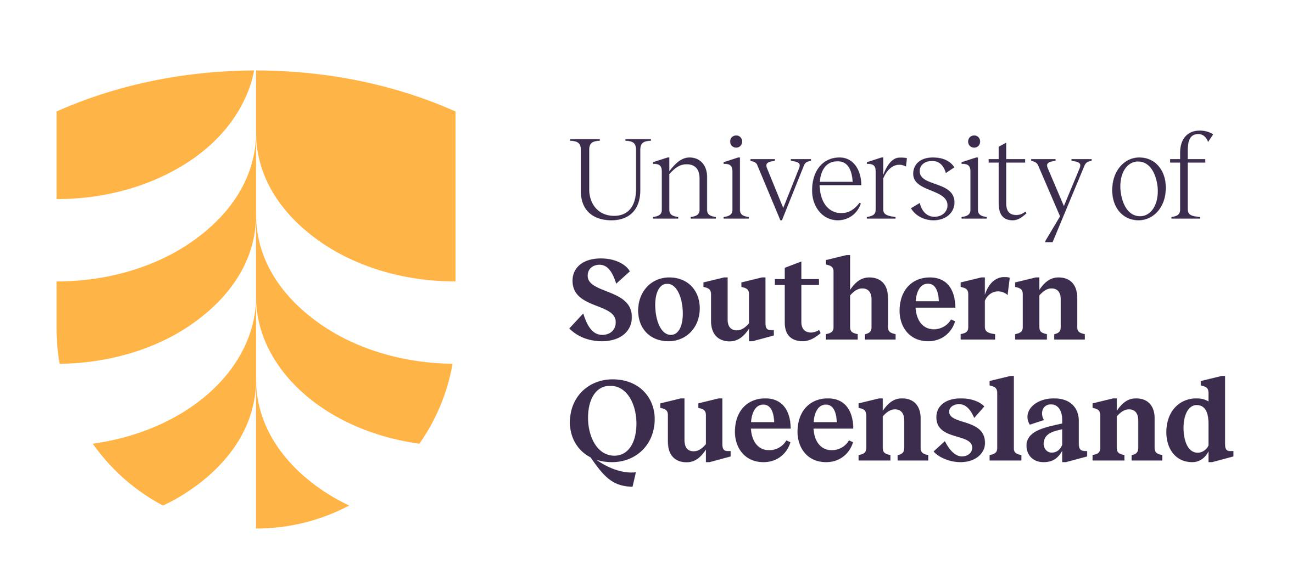
Want to create or adapt books like this? Learn more about how Pressbooks supports open publishing practices.
10 Experimental research
Experimental research—often considered to be the ‘gold standard’ in research designs—is one of the most rigorous of all research designs. In this design, one or more independent variables are manipulated by the researcher (as treatments), subjects are randomly assigned to different treatment levels (random assignment), and the results of the treatments on outcomes (dependent variables) are observed. The unique strength of experimental research is its internal validity (causality) due to its ability to link cause and effect through treatment manipulation, while controlling for the spurious effect of extraneous variable.
Experimental research is best suited for explanatory research—rather than for descriptive or exploratory research—where the goal of the study is to examine cause-effect relationships. It also works well for research that involves a relatively limited and well-defined set of independent variables that can either be manipulated or controlled. Experimental research can be conducted in laboratory or field settings. Laboratory experiments , conducted in laboratory (artificial) settings, tend to be high in internal validity, but this comes at the cost of low external validity (generalisability), because the artificial (laboratory) setting in which the study is conducted may not reflect the real world. Field experiments are conducted in field settings such as in a real organisation, and are high in both internal and external validity. But such experiments are relatively rare, because of the difficulties associated with manipulating treatments and controlling for extraneous effects in a field setting.
Experimental research can be grouped into two broad categories: true experimental designs and quasi-experimental designs. Both designs require treatment manipulation, but while true experiments also require random assignment, quasi-experiments do not. Sometimes, we also refer to non-experimental research, which is not really a research design, but an all-inclusive term that includes all types of research that do not employ treatment manipulation or random assignment, such as survey research, observational research, and correlational studies.
Basic concepts
Treatment and control groups. In experimental research, some subjects are administered one or more experimental stimulus called a treatment (the treatment group ) while other subjects are not given such a stimulus (the control group ). The treatment may be considered successful if subjects in the treatment group rate more favourably on outcome variables than control group subjects. Multiple levels of experimental stimulus may be administered, in which case, there may be more than one treatment group. For example, in order to test the effects of a new drug intended to treat a certain medical condition like dementia, if a sample of dementia patients is randomly divided into three groups, with the first group receiving a high dosage of the drug, the second group receiving a low dosage, and the third group receiving a placebo such as a sugar pill (control group), then the first two groups are experimental groups and the third group is a control group. After administering the drug for a period of time, if the condition of the experimental group subjects improved significantly more than the control group subjects, we can say that the drug is effective. We can also compare the conditions of the high and low dosage experimental groups to determine if the high dose is more effective than the low dose.
Treatment manipulation. Treatments are the unique feature of experimental research that sets this design apart from all other research methods. Treatment manipulation helps control for the ‘cause’ in cause-effect relationships. Naturally, the validity of experimental research depends on how well the treatment was manipulated. Treatment manipulation must be checked using pretests and pilot tests prior to the experimental study. Any measurements conducted before the treatment is administered are called pretest measures , while those conducted after the treatment are posttest measures .
Random selection and assignment. Random selection is the process of randomly drawing a sample from a population or a sampling frame. This approach is typically employed in survey research, and ensures that each unit in the population has a positive chance of being selected into the sample. Random assignment, however, is a process of randomly assigning subjects to experimental or control groups. This is a standard practice in true experimental research to ensure that treatment groups are similar (equivalent) to each other and to the control group prior to treatment administration. Random selection is related to sampling, and is therefore more closely related to the external validity (generalisability) of findings. However, random assignment is related to design, and is therefore most related to internal validity. It is possible to have both random selection and random assignment in well-designed experimental research, but quasi-experimental research involves neither random selection nor random assignment.
Threats to internal validity. Although experimental designs are considered more rigorous than other research methods in terms of the internal validity of their inferences (by virtue of their ability to control causes through treatment manipulation), they are not immune to internal validity threats. Some of these threats to internal validity are described below, within the context of a study of the impact of a special remedial math tutoring program for improving the math abilities of high school students.
History threat is the possibility that the observed effects (dependent variables) are caused by extraneous or historical events rather than by the experimental treatment. For instance, students’ post-remedial math score improvement may have been caused by their preparation for a math exam at their school, rather than the remedial math program.
Maturation threat refers to the possibility that observed effects are caused by natural maturation of subjects (e.g., a general improvement in their intellectual ability to understand complex concepts) rather than the experimental treatment.
Testing threat is a threat in pre-post designs where subjects’ posttest responses are conditioned by their pretest responses. For instance, if students remember their answers from the pretest evaluation, they may tend to repeat them in the posttest exam.
Not conducting a pretest can help avoid this threat.
Instrumentation threat , which also occurs in pre-post designs, refers to the possibility that the difference between pretest and posttest scores is not due to the remedial math program, but due to changes in the administered test, such as the posttest having a higher or lower degree of difficulty than the pretest.
Mortality threat refers to the possibility that subjects may be dropping out of the study at differential rates between the treatment and control groups due to a systematic reason, such that the dropouts were mostly students who scored low on the pretest. If the low-performing students drop out, the results of the posttest will be artificially inflated by the preponderance of high-performing students.
Regression threat —also called a regression to the mean—refers to the statistical tendency of a group’s overall performance to regress toward the mean during a posttest rather than in the anticipated direction. For instance, if subjects scored high on a pretest, they will have a tendency to score lower on the posttest (closer to the mean) because their high scores (away from the mean) during the pretest were possibly a statistical aberration. This problem tends to be more prevalent in non-random samples and when the two measures are imperfectly correlated.
Two-group experimental designs

Pretest-posttest control group design . In this design, subjects are randomly assigned to treatment and control groups, subjected to an initial (pretest) measurement of the dependent variables of interest, the treatment group is administered a treatment (representing the independent variable of interest), and the dependent variables measured again (posttest). The notation of this design is shown in Figure 10.1.

Statistical analysis of this design involves a simple analysis of variance (ANOVA) between the treatment and control groups. The pretest-posttest design handles several threats to internal validity, such as maturation, testing, and regression, since these threats can be expected to influence both treatment and control groups in a similar (random) manner. The selection threat is controlled via random assignment. However, additional threats to internal validity may exist. For instance, mortality can be a problem if there are differential dropout rates between the two groups, and the pretest measurement may bias the posttest measurement—especially if the pretest introduces unusual topics or content.
Posttest -only control group design . This design is a simpler version of the pretest-posttest design where pretest measurements are omitted. The design notation is shown in Figure 10.2.

The treatment effect is measured simply as the difference in the posttest scores between the two groups:
![Rendered by QuickLaTeX.com \[E = (O_{1} - O_{2})\,.\]](https://usq.pressbooks.pub/app/uploads/quicklatex/quicklatex.com-90f2ce47275e7b35def5c5b52b7d7d45_l3.png)
The appropriate statistical analysis of this design is also a two-group analysis of variance (ANOVA). The simplicity of this design makes it more attractive than the pretest-posttest design in terms of internal validity. This design controls for maturation, testing, regression, selection, and pretest-posttest interaction, though the mortality threat may continue to exist.

Because the pretest measure is not a measurement of the dependent variable, but rather a covariate, the treatment effect is measured as the difference in the posttest scores between the treatment and control groups as:
Due to the presence of covariates, the right statistical analysis of this design is a two-group analysis of covariance (ANCOVA). This design has all the advantages of posttest-only design, but with internal validity due to the controlling of covariates. Covariance designs can also be extended to pretest-posttest control group design.
Factorial designs
Two-group designs are inadequate if your research requires manipulation of two or more independent variables (treatments). In such cases, you would need four or higher-group designs. Such designs, quite popular in experimental research, are commonly called factorial designs. Each independent variable in this design is called a factor , and each subdivision of a factor is called a level . Factorial designs enable the researcher to examine not only the individual effect of each treatment on the dependent variables (called main effects), but also their joint effect (called interaction effects).

In a factorial design, a main effect is said to exist if the dependent variable shows a significant difference between multiple levels of one factor, at all levels of other factors. No change in the dependent variable across factor levels is the null case (baseline), from which main effects are evaluated. In the above example, you may see a main effect of instructional type, instructional time, or both on learning outcomes. An interaction effect exists when the effect of differences in one factor depends upon the level of a second factor. In our example, if the effect of instructional type on learning outcomes is greater for three hours/week of instructional time than for one and a half hours/week, then we can say that there is an interaction effect between instructional type and instructional time on learning outcomes. Note that the presence of interaction effects dominate and make main effects irrelevant, and it is not meaningful to interpret main effects if interaction effects are significant.
Hybrid experimental designs
Hybrid designs are those that are formed by combining features of more established designs. Three such hybrid designs are randomised bocks design, Solomon four-group design, and switched replications design.
Randomised block design. This is a variation of the posttest-only or pretest-posttest control group design where the subject population can be grouped into relatively homogeneous subgroups (called blocks ) within which the experiment is replicated. For instance, if you want to replicate the same posttest-only design among university students and full-time working professionals (two homogeneous blocks), subjects in both blocks are randomly split between the treatment group (receiving the same treatment) and the control group (see Figure 10.5). The purpose of this design is to reduce the ‘noise’ or variance in data that may be attributable to differences between the blocks so that the actual effect of interest can be detected more accurately.
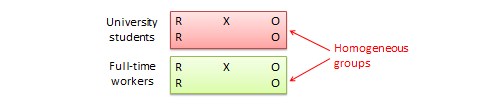
Solomon four-group design . In this design, the sample is divided into two treatment groups and two control groups. One treatment group and one control group receive the pretest, and the other two groups do not. This design represents a combination of posttest-only and pretest-posttest control group design, and is intended to test for the potential biasing effect of pretest measurement on posttest measures that tends to occur in pretest-posttest designs, but not in posttest-only designs. The design notation is shown in Figure 10.6.
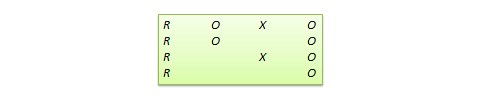
Switched replication design . This is a two-group design implemented in two phases with three waves of measurement. The treatment group in the first phase serves as the control group in the second phase, and the control group in the first phase becomes the treatment group in the second phase, as illustrated in Figure 10.7. In other words, the original design is repeated or replicated temporally with treatment/control roles switched between the two groups. By the end of the study, all participants will have received the treatment either during the first or the second phase. This design is most feasible in organisational contexts where organisational programs (e.g., employee training) are implemented in a phased manner or are repeated at regular intervals.

Quasi-experimental designs
Quasi-experimental designs are almost identical to true experimental designs, but lacking one key ingredient: random assignment. For instance, one entire class section or one organisation is used as the treatment group, while another section of the same class or a different organisation in the same industry is used as the control group. This lack of random assignment potentially results in groups that are non-equivalent, such as one group possessing greater mastery of certain content than the other group, say by virtue of having a better teacher in a previous semester, which introduces the possibility of selection bias . Quasi-experimental designs are therefore inferior to true experimental designs in interval validity due to the presence of a variety of selection related threats such as selection-maturation threat (the treatment and control groups maturing at different rates), selection-history threat (the treatment and control groups being differentially impacted by extraneous or historical events), selection-regression threat (the treatment and control groups regressing toward the mean between pretest and posttest at different rates), selection-instrumentation threat (the treatment and control groups responding differently to the measurement), selection-testing (the treatment and control groups responding differently to the pretest), and selection-mortality (the treatment and control groups demonstrating differential dropout rates). Given these selection threats, it is generally preferable to avoid quasi-experimental designs to the greatest extent possible.

In addition, there are quite a few unique non-equivalent designs without corresponding true experimental design cousins. Some of the more useful of these designs are discussed next.
Regression discontinuity (RD) design . This is a non-equivalent pretest-posttest design where subjects are assigned to the treatment or control group based on a cut-off score on a preprogram measure. For instance, patients who are severely ill may be assigned to a treatment group to test the efficacy of a new drug or treatment protocol and those who are mildly ill are assigned to the control group. In another example, students who are lagging behind on standardised test scores may be selected for a remedial curriculum program intended to improve their performance, while those who score high on such tests are not selected from the remedial program.

Because of the use of a cut-off score, it is possible that the observed results may be a function of the cut-off score rather than the treatment, which introduces a new threat to internal validity. However, using the cut-off score also ensures that limited or costly resources are distributed to people who need them the most, rather than randomly across a population, while simultaneously allowing a quasi-experimental treatment. The control group scores in the RD design do not serve as a benchmark for comparing treatment group scores, given the systematic non-equivalence between the two groups. Rather, if there is no discontinuity between pretest and posttest scores in the control group, but such a discontinuity persists in the treatment group, then this discontinuity is viewed as evidence of the treatment effect.
Proxy pretest design . This design, shown in Figure 10.11, looks very similar to the standard NEGD (pretest-posttest) design, with one critical difference: the pretest score is collected after the treatment is administered. A typical application of this design is when a researcher is brought in to test the efficacy of a program (e.g., an educational program) after the program has already started and pretest data is not available. Under such circumstances, the best option for the researcher is often to use a different prerecorded measure, such as students’ grade point average before the start of the program, as a proxy for pretest data. A variation of the proxy pretest design is to use subjects’ posttest recollection of pretest data, which may be subject to recall bias, but nevertheless may provide a measure of perceived gain or change in the dependent variable.

Separate pretest-posttest samples design . This design is useful if it is not possible to collect pretest and posttest data from the same subjects for some reason. As shown in Figure 10.12, there are four groups in this design, but two groups come from a single non-equivalent group, while the other two groups come from a different non-equivalent group. For instance, say you want to test customer satisfaction with a new online service that is implemented in one city but not in another. In this case, customers in the first city serve as the treatment group and those in the second city constitute the control group. If it is not possible to obtain pretest and posttest measures from the same customers, you can measure customer satisfaction at one point in time, implement the new service program, and measure customer satisfaction (with a different set of customers) after the program is implemented. Customer satisfaction is also measured in the control group at the same times as in the treatment group, but without the new program implementation. The design is not particularly strong, because you cannot examine the changes in any specific customer’s satisfaction score before and after the implementation, but you can only examine average customer satisfaction scores. Despite the lower internal validity, this design may still be a useful way of collecting quasi-experimental data when pretest and posttest data is not available from the same subjects.
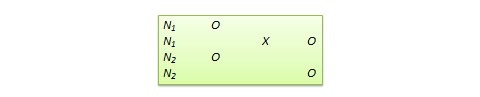
An interesting variation of the NEDV design is a pattern-matching NEDV design , which employs multiple outcome variables and a theory that explains how much each variable will be affected by the treatment. The researcher can then examine if the theoretical prediction is matched in actual observations. This pattern-matching technique—based on the degree of correspondence between theoretical and observed patterns—is a powerful way of alleviating internal validity concerns in the original NEDV design.

Perils of experimental research
Experimental research is one of the most difficult of research designs, and should not be taken lightly. This type of research is often best with a multitude of methodological problems. First, though experimental research requires theories for framing hypotheses for testing, much of current experimental research is atheoretical. Without theories, the hypotheses being tested tend to be ad hoc, possibly illogical, and meaningless. Second, many of the measurement instruments used in experimental research are not tested for reliability and validity, and are incomparable across studies. Consequently, results generated using such instruments are also incomparable. Third, often experimental research uses inappropriate research designs, such as irrelevant dependent variables, no interaction effects, no experimental controls, and non-equivalent stimulus across treatment groups. Findings from such studies tend to lack internal validity and are highly suspect. Fourth, the treatments (tasks) used in experimental research may be diverse, incomparable, and inconsistent across studies, and sometimes inappropriate for the subject population. For instance, undergraduate student subjects are often asked to pretend that they are marketing managers and asked to perform a complex budget allocation task in which they have no experience or expertise. The use of such inappropriate tasks, introduces new threats to internal validity (i.e., subject’s performance may be an artefact of the content or difficulty of the task setting), generates findings that are non-interpretable and meaningless, and makes integration of findings across studies impossible.
The design of proper experimental treatments is a very important task in experimental design, because the treatment is the raison d’etre of the experimental method, and must never be rushed or neglected. To design an adequate and appropriate task, researchers should use prevalidated tasks if available, conduct treatment manipulation checks to check for the adequacy of such tasks (by debriefing subjects after performing the assigned task), conduct pilot tests (repeatedly, if necessary), and if in doubt, use tasks that are simple and familiar for the respondent sample rather than tasks that are complex or unfamiliar.
In summary, this chapter introduced key concepts in the experimental design research method and introduced a variety of true experimental and quasi-experimental designs. Although these designs vary widely in internal validity, designs with less internal validity should not be overlooked and may sometimes be useful under specific circumstances and empirical contingencies.
Social Science Research: Principles, Methods and Practices (Revised edition) Copyright © 2019 by Anol Bhattacherjee is licensed under a Creative Commons Attribution-NonCommercial-ShareAlike 4.0 International License , except where otherwise noted.
Share This Book

Experimental Research Design — 6 mistakes you should never make!
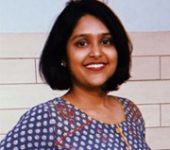
Since school days’ students perform scientific experiments that provide results that define and prove the laws and theorems in science. These experiments are laid on a strong foundation of experimental research designs.
An experimental research design helps researchers execute their research objectives with more clarity and transparency.
In this article, we will not only discuss the key aspects of experimental research designs but also the issues to avoid and problems to resolve while designing your research study.
Table of Contents
What Is Experimental Research Design?
Experimental research design is a framework of protocols and procedures created to conduct experimental research with a scientific approach using two sets of variables. Herein, the first set of variables acts as a constant, used to measure the differences of the second set. The best example of experimental research methods is quantitative research .
Experimental research helps a researcher gather the necessary data for making better research decisions and determining the facts of a research study.
When Can a Researcher Conduct Experimental Research?
A researcher can conduct experimental research in the following situations —
- When time is an important factor in establishing a relationship between the cause and effect.
- When there is an invariable or never-changing behavior between the cause and effect.
- Finally, when the researcher wishes to understand the importance of the cause and effect.
Importance of Experimental Research Design
To publish significant results, choosing a quality research design forms the foundation to build the research study. Moreover, effective research design helps establish quality decision-making procedures, structures the research to lead to easier data analysis, and addresses the main research question. Therefore, it is essential to cater undivided attention and time to create an experimental research design before beginning the practical experiment.
By creating a research design, a researcher is also giving oneself time to organize the research, set up relevant boundaries for the study, and increase the reliability of the results. Through all these efforts, one could also avoid inconclusive results. If any part of the research design is flawed, it will reflect on the quality of the results derived.
Types of Experimental Research Designs
Based on the methods used to collect data in experimental studies, the experimental research designs are of three primary types:
1. Pre-experimental Research Design
A research study could conduct pre-experimental research design when a group or many groups are under observation after implementing factors of cause and effect of the research. The pre-experimental design will help researchers understand whether further investigation is necessary for the groups under observation.
Pre-experimental research is of three types —
- One-shot Case Study Research Design
- One-group Pretest-posttest Research Design
- Static-group Comparison
2. True Experimental Research Design
A true experimental research design relies on statistical analysis to prove or disprove a researcher’s hypothesis. It is one of the most accurate forms of research because it provides specific scientific evidence. Furthermore, out of all the types of experimental designs, only a true experimental design can establish a cause-effect relationship within a group. However, in a true experiment, a researcher must satisfy these three factors —
- There is a control group that is not subjected to changes and an experimental group that will experience the changed variables
- A variable that can be manipulated by the researcher
- Random distribution of the variables
This type of experimental research is commonly observed in the physical sciences.
3. Quasi-experimental Research Design
The word “Quasi” means similarity. A quasi-experimental design is similar to a true experimental design. However, the difference between the two is the assignment of the control group. In this research design, an independent variable is manipulated, but the participants of a group are not randomly assigned. This type of research design is used in field settings where random assignment is either irrelevant or not required.
The classification of the research subjects, conditions, or groups determines the type of research design to be used.
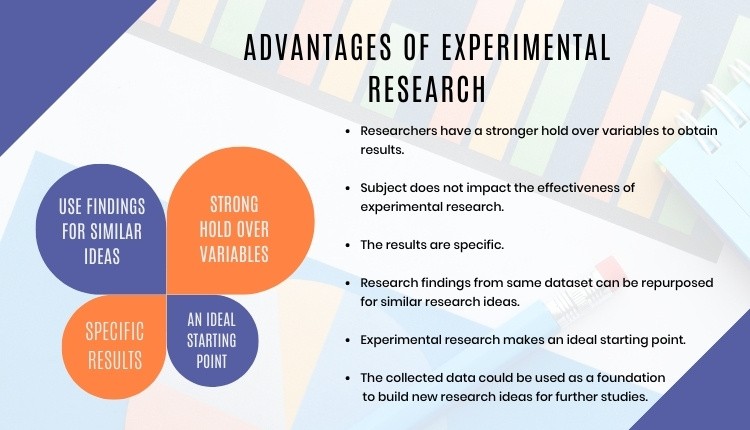
Advantages of Experimental Research
Experimental research allows you to test your idea in a controlled environment before taking the research to clinical trials. Moreover, it provides the best method to test your theory because of the following advantages:
- Researchers have firm control over variables to obtain results.
- The subject does not impact the effectiveness of experimental research. Anyone can implement it for research purposes.
- The results are specific.
- Post results analysis, research findings from the same dataset can be repurposed for similar research ideas.
- Researchers can identify the cause and effect of the hypothesis and further analyze this relationship to determine in-depth ideas.
- Experimental research makes an ideal starting point. The collected data could be used as a foundation to build new research ideas for further studies.
6 Mistakes to Avoid While Designing Your Research
There is no order to this list, and any one of these issues can seriously compromise the quality of your research. You could refer to the list as a checklist of what to avoid while designing your research.
1. Invalid Theoretical Framework
Usually, researchers miss out on checking if their hypothesis is logical to be tested. If your research design does not have basic assumptions or postulates, then it is fundamentally flawed and you need to rework on your research framework.
2. Inadequate Literature Study
Without a comprehensive research literature review , it is difficult to identify and fill the knowledge and information gaps. Furthermore, you need to clearly state how your research will contribute to the research field, either by adding value to the pertinent literature or challenging previous findings and assumptions.
3. Insufficient or Incorrect Statistical Analysis
Statistical results are one of the most trusted scientific evidence. The ultimate goal of a research experiment is to gain valid and sustainable evidence. Therefore, incorrect statistical analysis could affect the quality of any quantitative research.
4. Undefined Research Problem
This is one of the most basic aspects of research design. The research problem statement must be clear and to do that, you must set the framework for the development of research questions that address the core problems.
5. Research Limitations
Every study has some type of limitations . You should anticipate and incorporate those limitations into your conclusion, as well as the basic research design. Include a statement in your manuscript about any perceived limitations, and how you considered them while designing your experiment and drawing the conclusion.
6. Ethical Implications
The most important yet less talked about topic is the ethical issue. Your research design must include ways to minimize any risk for your participants and also address the research problem or question at hand. If you cannot manage the ethical norms along with your research study, your research objectives and validity could be questioned.
Experimental Research Design Example
In an experimental design, a researcher gathers plant samples and then randomly assigns half the samples to photosynthesize in sunlight and the other half to be kept in a dark box without sunlight, while controlling all the other variables (nutrients, water, soil, etc.)
By comparing their outcomes in biochemical tests, the researcher can confirm that the changes in the plants were due to the sunlight and not the other variables.
Experimental research is often the final form of a study conducted in the research process which is considered to provide conclusive and specific results. But it is not meant for every research. It involves a lot of resources, time, and money and is not easy to conduct, unless a foundation of research is built. Yet it is widely used in research institutes and commercial industries, for its most conclusive results in the scientific approach.
Have you worked on research designs? How was your experience creating an experimental design? What difficulties did you face? Do write to us or comment below and share your insights on experimental research designs!
Frequently Asked Questions
Randomization is important in an experimental research because it ensures unbiased results of the experiment. It also measures the cause-effect relationship on a particular group of interest.
Experimental research design lay the foundation of a research and structures the research to establish quality decision making process.
There are 3 types of experimental research designs. These are pre-experimental research design, true experimental research design, and quasi experimental research design.
The difference between an experimental and a quasi-experimental design are: 1. The assignment of the control group in quasi experimental research is non-random, unlike true experimental design, which is randomly assigned. 2. Experimental research group always has a control group; on the other hand, it may not be always present in quasi experimental research.
Experimental research establishes a cause-effect relationship by testing a theory or hypothesis using experimental groups or control variables. In contrast, descriptive research describes a study or a topic by defining the variables under it and answering the questions related to the same.

good and valuable
Very very good
Good presentation.
Rate this article Cancel Reply
Your email address will not be published.
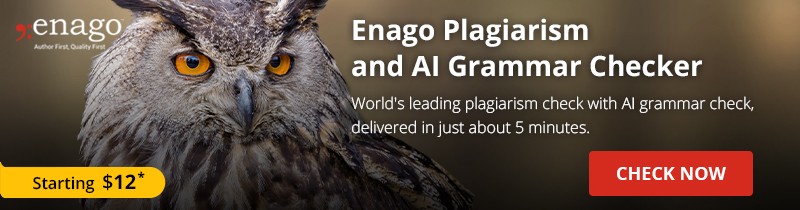
Enago Academy's Most Popular Articles
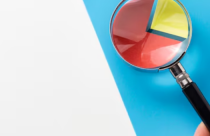
- Promoting Research
Graphical Abstracts Vs. Infographics: Best practices for using visual illustrations for increased research impact
Dr. Sarah Chen stared at her computer screen, her eyes staring at her recently published…
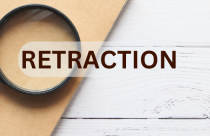
- Publishing Research
10 Tips to Prevent Research Papers From Being Retracted
Research paper retractions represent a critical event in the scientific community. When a published article…
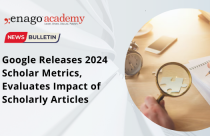
- Industry News
Google Releases 2024 Scholar Metrics, Evaluates Impact of Scholarly Articles
Google has released its 2024 Scholar Metrics, assessing scholarly articles from 2019 to 2023. This…
![experimental survey research design What is Academic Integrity and How to Uphold it [FREE CHECKLIST]](https://www.enago.com/academy/wp-content/uploads/2024/05/FeatureImages-59-210x136.png)
Ensuring Academic Integrity and Transparency in Academic Research: A comprehensive checklist for researchers
Academic integrity is the foundation upon which the credibility and value of scientific findings are…
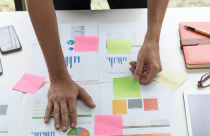
- Reporting Research
How to Optimize Your Research Process: A step-by-step guide
For researchers across disciplines, the path to uncovering novel findings and insights is often filled…
Choosing the Right Analytical Approach: Thematic analysis vs. content analysis for…
Comparing Cross Sectional and Longitudinal Studies: 5 steps for choosing the right…

Sign-up to read more
Subscribe for free to get unrestricted access to all our resources on research writing and academic publishing including:
- 2000+ blog articles
- 50+ Webinars
- 10+ Expert podcasts
- 50+ Infographics
- 10+ Checklists
- Research Guides
We hate spam too. We promise to protect your privacy and never spam you.
- AI in Academia
- Career Corner
- Diversity and Inclusion
- Infographics
- Expert Video Library
- Other Resources
- Enago Learn
- Upcoming & On-Demand Webinars
- Peer Review Week 2024
- Open Access Week 2023
- Conference Videos
- Enago Report
- Journal Finder
- Enago Plagiarism & AI Grammar Check
- Editing Services
- Publication Support Services
- Research Impact
- Translation Services
- Publication solutions
- AI-Based Solutions
- Thought Leadership
- Call for Articles
- Call for Speakers
- Author Training
- Edit Profile
I am looking for Editing/ Proofreading services for my manuscript Tentative date of next journal submission:
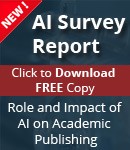
Which among these features would you prefer the most in a peer review assistant?

An official website of the United States government
The .gov means it’s official. Federal government websites often end in .gov or .mil. Before sharing sensitive information, make sure you’re on a federal government site.
The site is secure. The https:// ensures that you are connecting to the official website and that any information you provide is encrypted and transmitted securely.
- Publications
- Account settings
Preview improvements coming to the PMC website in October 2024. Learn More or Try it out now .
- Advanced Search
- Journal List
- J Athl Train
- v.45(1); Jan-Feb 2010
Study/Experimental/Research Design: Much More Than Statistics
Kenneth l. knight.
Brigham Young University, Provo, UT
The purpose of study, experimental, or research design in scientific manuscripts has changed significantly over the years. It has evolved from an explanation of the design of the experiment (ie, data gathering or acquisition) to an explanation of the statistical analysis. This practice makes “Methods” sections hard to read and understand.
To clarify the difference between study design and statistical analysis, to show the advantages of a properly written study design on article comprehension, and to encourage authors to correctly describe study designs.
Description:
The role of study design is explored from the introduction of the concept by Fisher through modern-day scientists and the AMA Manual of Style . At one time, when experiments were simpler, the study design and statistical design were identical or very similar. With the complex research that is common today, which often includes manipulating variables to create new variables and the multiple (and different) analyses of a single data set, data collection is very different than statistical design. Thus, both a study design and a statistical design are necessary.
Advantages:
Scientific manuscripts will be much easier to read and comprehend. A proper experimental design serves as a road map to the study methods, helping readers to understand more clearly how the data were obtained and, therefore, assisting them in properly analyzing the results.
Study, experimental, or research design is the backbone of good research. It directs the experiment by orchestrating data collection, defines the statistical analysis of the resultant data, and guides the interpretation of the results. When properly described in the written report of the experiment, it serves as a road map to readers, 1 helping them negotiate the “Methods” section, and, thus, it improves the clarity of communication between authors and readers.
A growing trend is to equate study design with only the statistical analysis of the data. The design statement typically is placed at the end of the “Methods” section as a subsection called “Experimental Design” or as part of a subsection called “Data Analysis.” This placement, however, equates experimental design and statistical analysis, minimizing the effect of experimental design on the planning and reporting of an experiment. This linkage is inappropriate, because some of the elements of the study design that should be described at the beginning of the “Methods” section are instead placed in the “Statistical Analysis” section or, worse, are absent from the manuscript entirely.
Have you ever interrupted your reading of the “Methods” to sketch out the variables in the margins of the paper as you attempt to understand how they all fit together? Or have you jumped back and forth from the early paragraphs of the “Methods” section to the “Statistics” section to try to understand which variables were collected and when? These efforts would be unnecessary if a road map at the beginning of the “Methods” section outlined how the independent variables were related, which dependent variables were measured, and when they were measured. When they were measured is especially important if the variables used in the statistical analysis were a subset of the measured variables or were computed from measured variables (such as change scores).
The purpose of this Communications article is to clarify the purpose and placement of study design elements in an experimental manuscript. Adopting these ideas may improve your science and surely will enhance the communication of that science. These ideas will make experimental manuscripts easier to read and understand and, therefore, will allow them to become part of readers' clinical decision making.
WHAT IS A STUDY (OR EXPERIMENTAL OR RESEARCH) DESIGN?
The terms study design, experimental design, and research design are often thought to be synonymous and are sometimes used interchangeably in a single paper. Avoid doing so. Use the term that is preferred by the style manual of the journal for which you are writing. Study design is the preferred term in the AMA Manual of Style , 2 so I will use it here.
A study design is the architecture of an experimental study 3 and a description of how the study was conducted, 4 including all elements of how the data were obtained. 5 The study design should be the first subsection of the “Methods” section in an experimental manuscript (see the Table ). “Statistical Design” or, preferably, “Statistical Analysis” or “Data Analysis” should be the last subsection of the “Methods” section.
Table. Elements of a “Methods” Section

The “Study Design” subsection describes how the variables and participants interacted. It begins with a general statement of how the study was conducted (eg, crossover trials, parallel, or observational study). 2 The second element, which usually begins with the second sentence, details the number of independent variables or factors, the levels of each variable, and their names. A shorthand way of doing so is with a statement such as “A 2 × 4 × 8 factorial guided data collection.” This tells us that there were 3 independent variables (factors), with 2 levels of the first factor, 4 levels of the second factor, and 8 levels of the third factor. Following is a sentence that names the levels of each factor: for example, “The independent variables were sex (male or female), training program (eg, walking, running, weight lifting, or plyometrics), and time (2, 4, 6, 8, 10, 15, 20, or 30 weeks).” Such an approach clearly outlines for readers how the various procedures fit into the overall structure and, therefore, enhances their understanding of how the data were collected. Thus, the design statement is a road map of the methods.
The dependent (or measurement or outcome) variables are then named. Details of how they were measured are not given at this point in the manuscript but are explained later in the “Instruments” and “Procedures” subsections.
Next is a paragraph detailing who the participants were and how they were selected, placed into groups, and assigned to a particular treatment order, if the experiment was a repeated-measures design. And although not a part of the design per se, a statement about obtaining written informed consent from participants and institutional review board approval is usually included in this subsection.
The nuts and bolts of the “Methods” section follow, including such things as equipment, materials, protocols, etc. These are beyond the scope of this commentary, however, and so will not be discussed.
The last part of the “Methods” section and last part of the “Study Design” section is the “Data Analysis” subsection. It begins with an explanation of any data manipulation, such as how data were combined or how new variables (eg, ratios or differences between collected variables) were calculated. Next, readers are told of the statistical measures used to analyze the data, such as a mixed 2 × 4 × 8 analysis of variance (ANOVA) with 2 between-groups factors (sex and training program) and 1 within-groups factor (time of measurement). Researchers should state and reference the statistical package and procedure(s) within the package used to compute the statistics. (Various statistical packages perform analyses slightly differently, so it is important to know the package and specific procedure used.) This detail allows readers to judge the appropriateness of the statistical measures and the conclusions drawn from the data.
STATISTICAL DESIGN VERSUS STATISTICAL ANALYSIS
Avoid using the term statistical design . Statistical methods are only part of the overall design. The term gives too much emphasis to the statistics, which are important, but only one of many tools used in interpreting data and only part of the study design:
The most important issues in biostatistics are not expressed with statistical procedures. The issues are inherently scientific, rather than purely statistical, and relate to the architectural design of the research, not the numbers with which the data are cited and interpreted. 6
Stated another way, “The justification for the analysis lies not in the data collected but in the manner in which the data were collected.” 3 “Without the solid foundation of a good design, the edifice of statistical analysis is unsafe.” 7 (pp4–5)
The intertwining of study design and statistical analysis may have been caused (unintentionally) by R.A. Fisher, “… a genius who almost single-handedly created the foundations for modern statistical science.” 8 Most research did not involve statistics until Fisher invented the concepts and procedures of ANOVA (in 1921) 9 , 10 and experimental design (in 1935). 11 His books became standard references for scientists in many disciplines. As a result, many ANOVA books were titled Experimental Design (see, for example, Edwards 12 ), and ANOVA courses taught in psychology and education departments included the words experimental design in their course titles.
Before the widespread use of computers to analyze data, designs were much simpler, and often there was little difference between study design and statistical analysis. So combining the 2 elements did not cause serious problems. This is no longer true, however, for 3 reasons: (1) Research studies are becoming more complex, with multiple independent and dependent variables. The procedures sections of these complex studies can be difficult to understand if your only reference point is the statistical analysis and design. (2) Dependent variables are frequently measured at different times. (3) How the data were collected is often not directly correlated with the statistical design.
For example, assume the goal is to determine the strength gain in novice and experienced athletes as a result of 3 strength training programs. Rate of change in strength is not a measurable variable; rather, it is calculated from strength measurements taken at various time intervals during the training. So the study design would be a 2 × 2 × 3 factorial with independent variables of time (pretest or posttest), experience (novice or advanced), and training (isokinetic, isotonic, or isometric) and a dependent variable of strength. The statistical design , however, would be a 2 × 3 factorial with independent variables of experience (novice or advanced) and training (isokinetic, isotonic, or isometric) and a dependent variable of strength gain. Note that data were collected according to a 3-factor design but were analyzed according to a 2-factor design and that the dependent variables were different. So a single design statement, usually a statistical design statement, would not communicate which data were collected or how. Readers would be left to figure out on their own how the data were collected.
MULTIVARIATE RESEARCH AND THE NEED FOR STUDY DESIGNS
With the advent of electronic data gathering and computerized data handling and analysis, research projects have increased in complexity. Many projects involve multiple dependent variables measured at different times, and, therefore, multiple design statements may be needed for both data collection and statistical analysis. Consider, for example, a study of the effects of heat and cold on neural inhibition. The variables of H max and M max are measured 3 times each: before, immediately after, and 30 minutes after a 20-minute treatment with heat or cold. Muscle temperature might be measured each minute before, during, and after the treatment. Although the minute-by-minute data are important for graphing temperature fluctuations during the procedure, only 3 temperatures (time 0, time 20, and time 50) are used for statistical analysis. A single dependent variable H max :M max ratio is computed to illustrate neural inhibition. Again, a single statistical design statement would tell little about how the data were obtained. And in this example, separate design statements would be needed for temperature measurement and H max :M max measurements.
As stated earlier, drawing conclusions from the data depends more on how the data were measured than on how they were analyzed. 3 , 6 , 7 , 13 So a single study design statement (or multiple such statements) at the beginning of the “Methods” section acts as a road map to the study and, thus, increases scientists' and readers' comprehension of how the experiment was conducted (ie, how the data were collected). Appropriate study design statements also increase the accuracy of conclusions drawn from the study.

CONCLUSIONS
The goal of scientific writing, or any writing, for that matter, is to communicate information. Including 2 design statements or subsections in scientific papers—one to explain how the data were collected and another to explain how they were statistically analyzed—will improve the clarity of communication and bring praise from readers. To summarize:
- Purge from your thoughts and vocabulary the idea that experimental design and statistical design are synonymous.
- Study or experimental design plays a much broader role than simply defining and directing the statistical analysis of an experiment.
- A properly written study design serves as a road map to the “Methods” section of an experiment and, therefore, improves communication with the reader.
- Study design should include a description of the type of design used, each factor (and each level) involved in the experiment, and the time at which each measurement was made.
- Clarify when the variables involved in data collection and data analysis are different, such as when data analysis involves only a subset of a collected variable or a resultant variable from the mathematical manipulation of 2 or more collected variables.
Acknowledgments
Thanks to Thomas A. Cappaert, PhD, ATC, CSCS, CSE, for suggesting the link between R.A. Fisher and the melding of the concepts of research design and statistics.
- Experimental Research Designs: Types, Examples & Methods

Experimental research is the most familiar type of research design for individuals in the physical sciences and a host of other fields. This is mainly because experimental research is a classical scientific experiment, similar to those performed in high school science classes.
Imagine taking 2 samples of the same plant and exposing one of them to sunlight, while the other is kept away from sunlight. Let the plant exposed to sunlight be called sample A, while the latter is called sample B.
If after the duration of the research, we find out that sample A grows and sample B dies, even though they are both regularly wetted and given the same treatment. Therefore, we can conclude that sunlight will aid growth in all similar plants.
What is Experimental Research?
Experimental research is a scientific approach to research, where one or more independent variables are manipulated and applied to one or more dependent variables to measure their effect on the latter. The effect of the independent variables on the dependent variables is usually observed and recorded over some time, to aid researchers in drawing a reasonable conclusion regarding the relationship between these 2 variable types.
The experimental research method is widely used in physical and social sciences, psychology, and education. It is based on the comparison between two or more groups with a straightforward logic, which may, however, be difficult to execute.
Mostly related to a laboratory test procedure, experimental research designs involve collecting quantitative data and performing statistical analysis on them during research. Therefore, making it an example of quantitative research method .
What are The Types of Experimental Research Design?
The types of experimental research design are determined by the way the researcher assigns subjects to different conditions and groups. They are of 3 types, namely; pre-experimental, quasi-experimental, and true experimental research.
Pre-experimental Research Design
In pre-experimental research design, either a group or various dependent groups are observed for the effect of the application of an independent variable which is presumed to cause change. It is the simplest form of experimental research design and is treated with no control group.
Although very practical, experimental research is lacking in several areas of the true-experimental criteria. The pre-experimental research design is further divided into three types
- One-shot Case Study Research Design
In this type of experimental study, only one dependent group or variable is considered. The study is carried out after some treatment which was presumed to cause change, making it a posttest study.
- One-group Pretest-posttest Research Design:
This research design combines both posttest and pretest study by carrying out a test on a single group before the treatment is administered and after the treatment is administered. With the former being administered at the beginning of treatment and later at the end.
- Static-group Comparison:
In a static-group comparison study, 2 or more groups are placed under observation, where only one of the groups is subjected to some treatment while the other groups are held static. All the groups are post-tested, and the observed differences between the groups are assumed to be a result of the treatment.
Quasi-experimental Research Design
The word “quasi” means partial, half, or pseudo. Therefore, the quasi-experimental research bearing a resemblance to the true experimental research, but not the same. In quasi-experiments, the participants are not randomly assigned, and as such, they are used in settings where randomization is difficult or impossible.
This is very common in educational research, where administrators are unwilling to allow the random selection of students for experimental samples.
Some examples of quasi-experimental research design include; the time series, no equivalent control group design, and the counterbalanced design.
True Experimental Research Design
The true experimental research design relies on statistical analysis to approve or disprove a hypothesis. It is the most accurate type of experimental design and may be carried out with or without a pretest on at least 2 randomly assigned dependent subjects.
The true experimental research design must contain a control group, a variable that can be manipulated by the researcher, and the distribution must be random. The classification of true experimental design include:
- The posttest-only Control Group Design: In this design, subjects are randomly selected and assigned to the 2 groups (control and experimental), and only the experimental group is treated. After close observation, both groups are post-tested, and a conclusion is drawn from the difference between these groups.
- The pretest-posttest Control Group Design: For this control group design, subjects are randomly assigned to the 2 groups, both are presented, but only the experimental group is treated. After close observation, both groups are post-tested to measure the degree of change in each group.
- Solomon four-group Design: This is the combination of the pretest-only and the pretest-posttest control groups. In this case, the randomly selected subjects are placed into 4 groups.
The first two of these groups are tested using the posttest-only method, while the other two are tested using the pretest-posttest method.
Examples of Experimental Research
Experimental research examples are different, depending on the type of experimental research design that is being considered. The most basic example of experimental research is laboratory experiments, which may differ in nature depending on the subject of research.
Administering Exams After The End of Semester
During the semester, students in a class are lectured on particular courses and an exam is administered at the end of the semester. In this case, the students are the subjects or dependent variables while the lectures are the independent variables treated on the subjects.
Only one group of carefully selected subjects are considered in this research, making it a pre-experimental research design example. We will also notice that tests are only carried out at the end of the semester, and not at the beginning.
Further making it easy for us to conclude that it is a one-shot case study research.
Employee Skill Evaluation
Before employing a job seeker, organizations conduct tests that are used to screen out less qualified candidates from the pool of qualified applicants. This way, organizations can determine an employee’s skill set at the point of employment.
In the course of employment, organizations also carry out employee training to improve employee productivity and generally grow the organization. Further evaluation is carried out at the end of each training to test the impact of the training on employee skills, and test for improvement.
Here, the subject is the employee, while the treatment is the training conducted. This is a pretest-posttest control group experimental research example.
Evaluation of Teaching Method
Let us consider an academic institution that wants to evaluate the teaching method of 2 teachers to determine which is best. Imagine a case whereby the students assigned to each teacher is carefully selected probably due to personal request by parents or due to stubbornness and smartness.
This is a no equivalent group design example because the samples are not equal. By evaluating the effectiveness of each teacher’s teaching method this way, we may conclude after a post-test has been carried out.
However, this may be influenced by factors like the natural sweetness of a student. For example, a very smart student will grab more easily than his or her peers irrespective of the method of teaching.
What are the Characteristics of Experimental Research?
Experimental research contains dependent, independent and extraneous variables. The dependent variables are the variables being treated or manipulated and are sometimes called the subject of the research.
The independent variables are the experimental treatment being exerted on the dependent variables. Extraneous variables, on the other hand, are other factors affecting the experiment that may also contribute to the change.
The setting is where the experiment is carried out. Many experiments are carried out in the laboratory, where control can be exerted on the extraneous variables, thereby eliminating them.
Other experiments are carried out in a less controllable setting. The choice of setting used in research depends on the nature of the experiment being carried out.
- Multivariable
Experimental research may include multiple independent variables, e.g. time, skills, test scores, etc.
Why Use Experimental Research Design?
Experimental research design can be majorly used in physical sciences, social sciences, education, and psychology. It is used to make predictions and draw conclusions on a subject matter.
Some uses of experimental research design are highlighted below.
- Medicine: Experimental research is used to provide the proper treatment for diseases. In most cases, rather than directly using patients as the research subject, researchers take a sample of the bacteria from the patient’s body and are treated with the developed antibacterial
The changes observed during this period are recorded and evaluated to determine its effectiveness. This process can be carried out using different experimental research methods.
- Education: Asides from science subjects like Chemistry and Physics which involves teaching students how to perform experimental research, it can also be used in improving the standard of an academic institution. This includes testing students’ knowledge on different topics, coming up with better teaching methods, and the implementation of other programs that will aid student learning.
- Human Behavior: Social scientists are the ones who mostly use experimental research to test human behaviour. For example, consider 2 people randomly chosen to be the subject of the social interaction research where one person is placed in a room without human interaction for 1 year.
The other person is placed in a room with a few other people, enjoying human interaction. There will be a difference in their behaviour at the end of the experiment.
- UI/UX: During the product development phase, one of the major aims of the product team is to create a great user experience with the product. Therefore, before launching the final product design, potential are brought in to interact with the product.
For example, when finding it difficult to choose how to position a button or feature on the app interface, a random sample of product testers are allowed to test the 2 samples and how the button positioning influences the user interaction is recorded.
What are the Disadvantages of Experimental Research?
- It is highly prone to human error due to its dependency on variable control which may not be properly implemented. These errors could eliminate the validity of the experiment and the research being conducted.
- Exerting control of extraneous variables may create unrealistic situations. Eliminating real-life variables will result in inaccurate conclusions. This may also result in researchers controlling the variables to suit his or her personal preferences.
- It is a time-consuming process. So much time is spent on testing dependent variables and waiting for the effect of the manipulation of dependent variables to manifest.
- It is expensive.
- It is very risky and may have ethical complications that cannot be ignored. This is common in medical research, where failed trials may lead to a patient’s death or a deteriorating health condition.
- Experimental research results are not descriptive.
- Response bias can also be supplied by the subject of the conversation.
- Human responses in experimental research can be difficult to measure.
What are the Data Collection Methods in Experimental Research?
Data collection methods in experimental research are the different ways in which data can be collected for experimental research. They are used in different cases, depending on the type of research being carried out.
1. Observational Study
This type of study is carried out over a long period. It measures and observes the variables of interest without changing existing conditions.
When researching the effect of social interaction on human behavior, the subjects who are placed in 2 different environments are observed throughout the research. No matter the kind of absurd behavior that is exhibited by the subject during this period, its condition will not be changed.
This may be a very risky thing to do in medical cases because it may lead to death or worse medical conditions.
2. Simulations
This procedure uses mathematical, physical, or computer models to replicate a real-life process or situation. It is frequently used when the actual situation is too expensive, dangerous, or impractical to replicate in real life.
This method is commonly used in engineering and operational research for learning purposes and sometimes as a tool to estimate possible outcomes of real research. Some common situation software are Simulink, MATLAB, and Simul8.
Not all kinds of experimental research can be carried out using simulation as a data collection tool . It is very impractical for a lot of laboratory-based research that involves chemical processes.
A survey is a tool used to gather relevant data about the characteristics of a population and is one of the most common data collection tools. A survey consists of a group of questions prepared by the researcher, to be answered by the research subject.
Surveys can be shared with the respondents both physically and electronically. When collecting data through surveys, the kind of data collected depends on the respondent, and researchers have limited control over it.
Formplus is the best tool for collecting experimental data using survey s. It has relevant features that will aid the data collection process and can also be used in other aspects of experimental research.
Differences between Experimental and Non-Experimental Research
1. In experimental research, the researcher can control and manipulate the environment of the research, including the predictor variable which can be changed. On the other hand, non-experimental research cannot be controlled or manipulated by the researcher at will.
This is because it takes place in a real-life setting, where extraneous variables cannot be eliminated. Therefore, it is more difficult to conclude non-experimental studies, even though they are much more flexible and allow for a greater range of study fields.
2. The relationship between cause and effect cannot be established in non-experimental research, while it can be established in experimental research. This may be because many extraneous variables also influence the changes in the research subject, making it difficult to point at a particular variable as the cause of a particular change
3. Independent variables are not introduced, withdrawn, or manipulated in non-experimental designs, but the same may not be said about experimental research.
Experimental Research vs. Alternatives and When to Use Them
1. experimental research vs causal comparative.
Experimental research enables you to control variables and identify how the independent variable affects the dependent variable. Causal-comparative find out the cause-and-effect relationship between the variables by comparing already existing groups that are affected differently by the independent variable.
For example, in an experiment to see how K-12 education affects children and teenager development. An experimental research would split the children into groups, some would get formal K-12 education, while others won’t. This is not ethically right because every child has the right to education. So, what we do instead would be to compare already existing groups of children who are getting formal education with those who due to some circumstances can not.
Pros and Cons of Experimental vs Causal-Comparative Research
- Causal-Comparative: Strengths: More realistic than experiments, can be conducted in real-world settings. Weaknesses: Establishing causality can be weaker due to the lack of manipulation.
2. Experimental Research vs Correlational Research
When experimenting, you are trying to establish a cause-and-effect relationship between different variables. For example, you are trying to establish the effect of heat on water, the temperature keeps changing (independent variable) and you see how it affects the water (dependent variable).
For correlational research, you are not necessarily interested in the why or the cause-and-effect relationship between the variables, you are focusing on the relationship. Using the same water and temperature example, you are only interested in the fact that they change, you are not investigating which of the variables or other variables causes them to change.
Pros and Cons of Experimental vs Correlational Research
3. experimental research vs descriptive research.
With experimental research, you alter the independent variable to see how it affects the dependent variable, but with descriptive research you are simply studying the characteristics of the variable you are studying.
So, in an experiment to see how blown glass reacts to temperature, experimental research would keep altering the temperature to varying levels of high and low to see how it affects the dependent variable (glass). But descriptive research would investigate the glass properties.
Pros and Cons of Experimental vs Descriptive Research
4. experimental research vs action research.
Experimental research tests for causal relationships by focusing on one independent variable vs the dependent variable and keeps other variables constant. So, you are testing hypotheses and using the information from the research to contribute to knowledge.
However, with action research, you are using a real-world setting which means you are not controlling variables. You are also performing the research to solve actual problems and improve already established practices.
For example, if you are testing for how long commutes affect workers’ productivity. With experimental research, you would vary the length of commute to see how the time affects work. But with action research, you would account for other factors such as weather, commute route, nutrition, etc. Also, experimental research helps know the relationship between commute time and productivity, while action research helps you look for ways to improve productivity
Pros and Cons of Experimental vs Action Research
Conclusion .
Experimental research designs are often considered to be the standard in research designs. This is partly due to the common misconception that research is equivalent to scientific experiments—a component of experimental research design.
In this research design, one or more subjects or dependent variables are randomly assigned to different treatments (i.e. independent variables manipulated by the researcher) and the results are observed to conclude. One of the uniqueness of experimental research is in its ability to control the effect of extraneous variables.
Experimental research is suitable for research whose goal is to examine cause-effect relationships, e.g. explanatory research. It can be conducted in the laboratory or field settings, depending on the aim of the research that is being carried out.
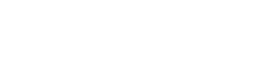
Connect to Formplus, Get Started Now - It's Free!
- examples of experimental research
- experimental research methods
- types of experimental research
- busayo.longe
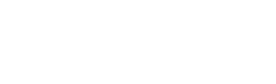
You may also like:
What is Experimenter Bias? Definition, Types & Mitigation
In this article, we will look into the concept of experimental bias and how it can be identified in your research

Response vs Explanatory Variables: Definition & Examples
In this article, we’ll be comparing the two types of variables, what they both mean and see some of their real-life applications in research
Simpson’s Paradox & How to Avoid it in Experimental Research
In this article, we are going to look at Simpson’s Paradox from its historical point and later, we’ll consider its effect in...
Experimental Vs Non-Experimental Research: 15 Key Differences
Differences between experimental and non experimental research on definitions, types, examples, data collection tools, uses, advantages etc.
Formplus - For Seamless Data Collection
Collect data the right way with a versatile data collection tool. try formplus and transform your work productivity today..
- Privacy Policy

Home » Research Design – Types, Methods and Examples
Research Design – Types, Methods and Examples
Table of Contents

Research Design
Definition:
Research design refers to the overall strategy or plan for conducting a research study. It outlines the methods and procedures that will be used to collect and analyze data, as well as the goals and objectives of the study. Research design is important because it guides the entire research process and ensures that the study is conducted in a systematic and rigorous manner.
Types of Research Design
Types of Research Design are as follows:
Descriptive Research Design
This type of research design is used to describe a phenomenon or situation. It involves collecting data through surveys, questionnaires, interviews, and observations. The aim of descriptive research is to provide an accurate and detailed portrayal of a particular group, event, or situation. It can be useful in identifying patterns, trends, and relationships in the data.
Correlational Research Design
Correlational research design is used to determine if there is a relationship between two or more variables. This type of research design involves collecting data from participants and analyzing the relationship between the variables using statistical methods. The aim of correlational research is to identify the strength and direction of the relationship between the variables.
Experimental Research Design
Experimental research design is used to investigate cause-and-effect relationships between variables. This type of research design involves manipulating one variable and measuring the effect on another variable. It usually involves randomly assigning participants to groups and manipulating an independent variable to determine its effect on a dependent variable. The aim of experimental research is to establish causality.
Quasi-experimental Research Design
Quasi-experimental research design is similar to experimental research design, but it lacks one or more of the features of a true experiment. For example, there may not be random assignment to groups or a control group. This type of research design is used when it is not feasible or ethical to conduct a true experiment.
Case Study Research Design
Case study research design is used to investigate a single case or a small number of cases in depth. It involves collecting data through various methods, such as interviews, observations, and document analysis. The aim of case study research is to provide an in-depth understanding of a particular case or situation.
Longitudinal Research Design
Longitudinal research design is used to study changes in a particular phenomenon over time. It involves collecting data at multiple time points and analyzing the changes that occur. The aim of longitudinal research is to provide insights into the development, growth, or decline of a particular phenomenon over time.
Structure of Research Design
The format of a research design typically includes the following sections:
- Introduction : This section provides an overview of the research problem, the research questions, and the importance of the study. It also includes a brief literature review that summarizes previous research on the topic and identifies gaps in the existing knowledge.
- Research Questions or Hypotheses: This section identifies the specific research questions or hypotheses that the study will address. These questions should be clear, specific, and testable.
- Research Methods : This section describes the methods that will be used to collect and analyze data. It includes details about the study design, the sampling strategy, the data collection instruments, and the data analysis techniques.
- Data Collection: This section describes how the data will be collected, including the sample size, data collection procedures, and any ethical considerations.
- Data Analysis: This section describes how the data will be analyzed, including the statistical techniques that will be used to test the research questions or hypotheses.
- Results : This section presents the findings of the study, including descriptive statistics and statistical tests.
- Discussion and Conclusion : This section summarizes the key findings of the study, interprets the results, and discusses the implications of the findings. It also includes recommendations for future research.
- References : This section lists the sources cited in the research design.
Example of Research Design
An Example of Research Design could be:
Research question: Does the use of social media affect the academic performance of high school students?
Research design:
- Research approach : The research approach will be quantitative as it involves collecting numerical data to test the hypothesis.
- Research design : The research design will be a quasi-experimental design, with a pretest-posttest control group design.
- Sample : The sample will be 200 high school students from two schools, with 100 students in the experimental group and 100 students in the control group.
- Data collection : The data will be collected through surveys administered to the students at the beginning and end of the academic year. The surveys will include questions about their social media usage and academic performance.
- Data analysis : The data collected will be analyzed using statistical software. The mean scores of the experimental and control groups will be compared to determine whether there is a significant difference in academic performance between the two groups.
- Limitations : The limitations of the study will be acknowledged, including the fact that social media usage can vary greatly among individuals, and the study only focuses on two schools, which may not be representative of the entire population.
- Ethical considerations: Ethical considerations will be taken into account, such as obtaining informed consent from the participants and ensuring their anonymity and confidentiality.
How to Write Research Design
Writing a research design involves planning and outlining the methodology and approach that will be used to answer a research question or hypothesis. Here are some steps to help you write a research design:
- Define the research question or hypothesis : Before beginning your research design, you should clearly define your research question or hypothesis. This will guide your research design and help you select appropriate methods.
- Select a research design: There are many different research designs to choose from, including experimental, survey, case study, and qualitative designs. Choose a design that best fits your research question and objectives.
- Develop a sampling plan : If your research involves collecting data from a sample, you will need to develop a sampling plan. This should outline how you will select participants and how many participants you will include.
- Define variables: Clearly define the variables you will be measuring or manipulating in your study. This will help ensure that your results are meaningful and relevant to your research question.
- Choose data collection methods : Decide on the data collection methods you will use to gather information. This may include surveys, interviews, observations, experiments, or secondary data sources.
- Create a data analysis plan: Develop a plan for analyzing your data, including the statistical or qualitative techniques you will use.
- Consider ethical concerns : Finally, be sure to consider any ethical concerns related to your research, such as participant confidentiality or potential harm.
When to Write Research Design
Research design should be written before conducting any research study. It is an important planning phase that outlines the research methodology, data collection methods, and data analysis techniques that will be used to investigate a research question or problem. The research design helps to ensure that the research is conducted in a systematic and logical manner, and that the data collected is relevant and reliable.
Ideally, the research design should be developed as early as possible in the research process, before any data is collected. This allows the researcher to carefully consider the research question, identify the most appropriate research methodology, and plan the data collection and analysis procedures in advance. By doing so, the research can be conducted in a more efficient and effective manner, and the results are more likely to be valid and reliable.
Purpose of Research Design
The purpose of research design is to plan and structure a research study in a way that enables the researcher to achieve the desired research goals with accuracy, validity, and reliability. Research design is the blueprint or the framework for conducting a study that outlines the methods, procedures, techniques, and tools for data collection and analysis.
Some of the key purposes of research design include:
- Providing a clear and concise plan of action for the research study.
- Ensuring that the research is conducted ethically and with rigor.
- Maximizing the accuracy and reliability of the research findings.
- Minimizing the possibility of errors, biases, or confounding variables.
- Ensuring that the research is feasible, practical, and cost-effective.
- Determining the appropriate research methodology to answer the research question(s).
- Identifying the sample size, sampling method, and data collection techniques.
- Determining the data analysis method and statistical tests to be used.
- Facilitating the replication of the study by other researchers.
- Enhancing the validity and generalizability of the research findings.
Applications of Research Design
There are numerous applications of research design in various fields, some of which are:
- Social sciences: In fields such as psychology, sociology, and anthropology, research design is used to investigate human behavior and social phenomena. Researchers use various research designs, such as experimental, quasi-experimental, and correlational designs, to study different aspects of social behavior.
- Education : Research design is essential in the field of education to investigate the effectiveness of different teaching methods and learning strategies. Researchers use various designs such as experimental, quasi-experimental, and case study designs to understand how students learn and how to improve teaching practices.
- Health sciences : In the health sciences, research design is used to investigate the causes, prevention, and treatment of diseases. Researchers use various designs, such as randomized controlled trials, cohort studies, and case-control studies, to study different aspects of health and healthcare.
- Business : Research design is used in the field of business to investigate consumer behavior, marketing strategies, and the impact of different business practices. Researchers use various designs, such as survey research, experimental research, and case studies, to study different aspects of the business world.
- Engineering : In the field of engineering, research design is used to investigate the development and implementation of new technologies. Researchers use various designs, such as experimental research and case studies, to study the effectiveness of new technologies and to identify areas for improvement.
Advantages of Research Design
Here are some advantages of research design:
- Systematic and organized approach : A well-designed research plan ensures that the research is conducted in a systematic and organized manner, which makes it easier to manage and analyze the data.
- Clear objectives: The research design helps to clarify the objectives of the study, which makes it easier to identify the variables that need to be measured, and the methods that need to be used to collect and analyze data.
- Minimizes bias: A well-designed research plan minimizes the chances of bias, by ensuring that the data is collected and analyzed objectively, and that the results are not influenced by the researcher’s personal biases or preferences.
- Efficient use of resources: A well-designed research plan helps to ensure that the resources (time, money, and personnel) are used efficiently and effectively, by focusing on the most important variables and methods.
- Replicability: A well-designed research plan makes it easier for other researchers to replicate the study, which enhances the credibility and reliability of the findings.
- Validity: A well-designed research plan helps to ensure that the findings are valid, by ensuring that the methods used to collect and analyze data are appropriate for the research question.
- Generalizability : A well-designed research plan helps to ensure that the findings can be generalized to other populations, settings, or situations, which increases the external validity of the study.
Research Design Vs Research Methodology
Research Design | Research Methodology |
---|---|
The plan and structure for conducting research that outlines the procedures to be followed to collect and analyze data. | The set of principles, techniques, and tools used to carry out the research plan and achieve research objectives. |
Describes the overall approach and strategy used to conduct research, including the type of data to be collected, the sources of data, and the methods for collecting and analyzing data. | Refers to the techniques and methods used to gather, analyze and interpret data, including sampling techniques, data collection methods, and data analysis techniques. |
Helps to ensure that the research is conducted in a systematic, rigorous, and valid way, so that the results are reliable and can be used to make sound conclusions. | Includes a set of procedures and tools that enable researchers to collect and analyze data in a consistent and valid manner, regardless of the research design used. |
Common research designs include experimental, quasi-experimental, correlational, and descriptive studies. | Common research methodologies include qualitative, quantitative, and mixed-methods approaches. |
Determines the overall structure of the research project and sets the stage for the selection of appropriate research methodologies. | Guides the researcher in selecting the most appropriate research methods based on the research question, research design, and other contextual factors. |
Helps to ensure that the research project is feasible, relevant, and ethical. | Helps to ensure that the data collected is accurate, valid, and reliable, and that the research findings can be interpreted and generalized to the population of interest. |
About the author
Muhammad Hassan
Researcher, Academic Writer, Web developer
You may also like

Context of the Study – Writing Guide and Examples

Research Topics – Ideas and Examples

Significance of the Study – Examples and Writing...

APA Research Paper Format – Example, Sample and...

Research Paper Title Page – Example and Making...

Background of The Study – Examples and Writing...
Leave a comment x.
Save my name, email, and website in this browser for the next time I comment.
- Key Differences
Know the Differences & Comparisons
Difference Between Survey and Experiment
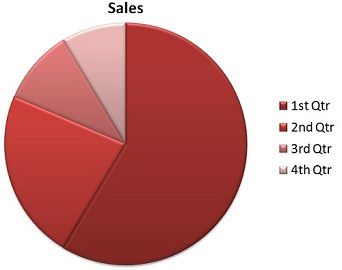
While surveys collected data, provided by the informants, experiments test various premises by trial and error method. This article attempts to shed light on the difference between survey and experiment, have a look.
Content: Survey Vs Experiment
Comparison chart.
Basis for Comparison | Survey | Experiment |
---|---|---|
Meaning | Survey refers to a technique of gathering information regarding a variable under study, from the respondents of the population. | Experiment implies a scientific procedure wherein the factor under study is isolated to test hypothesis. |
Used in | Descriptive Research | Experimental Research |
Samples | Large | Relatively small |
Suitable for | Social and Behavioral sciences | Physical and natural sciences |
Example of | Field research | Laboratory research |
Data collection | Observation, interview, questionnaire, case study etc. | Through several readings of experiment. |
Definition of Survey
By the term survey, we mean a method of securing information relating to the variable under study from all or a specified number of respondents of the universe. It may be a sample survey or a census survey. This method relies on the questioning of the informants on a specific subject. Survey follows structured form of data collection, in which a formal questionnaire is prepared, and the questions are asked in a predefined order.
Informants are asked questions concerning their behaviour, attitude, motivation, demographic, lifestyle characteristics, etc. through observation, direct communication with them over telephone/mail or personal interview. Questions are asked verbally to the respondents, i.e. in writing or by way of computer. The answer of the respondents is obtained in the same form.
Definition of Experiment
The term experiment means a systematic and logical scientific procedure in which one or more independent variables under test are manipulated, and any change on one or more dependent variable is measured while controlling for the effect of the extraneous variable. Here extraneous variable is an independent variable which is not associated with the objective of study but may affect the response of test units.
In an experiment, the investigator attempts to observe the outcome of the experiment conducted by him intentionally, to test the hypothesis or to discover something or to demonstrate a known fact. An experiment aims at drawing conclusions concerning the factor on the study group and making inferences from sample to larger population of interest.
Key Differences Between Survey and Experiment
The differences between survey and experiment can be drawn clearly on the following grounds:
- A technique of gathering information regarding a variable under study, from the respondents of the population, is called survey. A scientific procedure wherein the factor under study is isolated to test hypothesis is called an experiment.
- Surveys are performed when the research is of descriptive nature, whereas in the case of experiments are conducted in experimental research.
- The survey samples are large as the response rate is low, especially when the survey is conducted through mailed questionnaire. On the other hand, samples required in the case of experiments is relatively small.
- Surveys are considered suitable for social and behavioural science. As against this, experiments are an important characteristic of physical and natural sciences.
- Field research refers to the research conducted outside the laboratory or workplace. Surveys are the best example of field research. On the contrary, Experiment is an example of laboratory research. A laboratory research is nothing but research carried on inside the room equipped with scientific tools and equipment.
- In surveys, the data collection methods employed can either be observation, interview, questionnaire, or case study. As opposed to experiment, the data is obtained through several readings of the experiment.
While survey studies the possible relationship between data and unknown variable, experiments determine the relationship. Further, Correlation analysis is vital in surveys, as in social and business surveys, the interest of the researcher rests in understanding and controlling relationships between variables. Unlike experiments, where casual analysis is significant.
You Might Also Like:
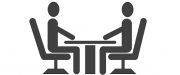
sanjay kumar yadav says
November 17, 2016 at 1:08 am
Ishika says
September 9, 2017 at 9:30 pm
The article was quite helpful… Thank you.
May 21, 2018 at 3:26 pm
Can you develop your Application for Android
Surbhi S says
May 21, 2018 at 4:21 pm
Yeah, we will develop android app soon.
October 31, 2018 at 12:32 am
If I was doing an experiment with Poverty and Education level, which do you think would be more appropriate for me?
Thanks, Chris
Ndaware M.M says
January 7, 2021 at 2:29 am
So interested,
Victoria Addington says
May 18, 2023 at 5:31 pm
Thank you for explaining the topic
Leave a Reply Cancel reply
Your email address will not be published. Required fields are marked *
Save my name, email, and website in this browser for the next time I comment.
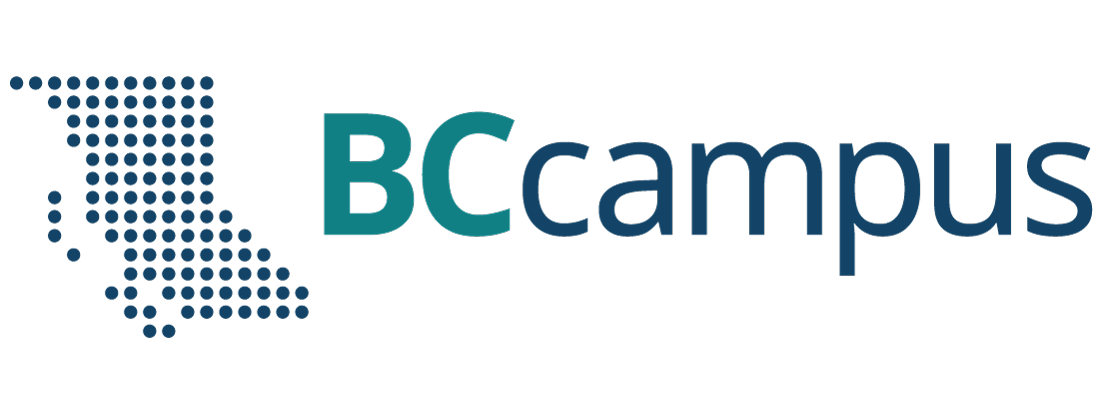
Want to create or adapt books like this? Learn more about how Pressbooks supports open publishing practices.
Chapter 6: Experimental Research
Experimental Design
Learning Objectives
- Explain the difference between between-subjects and within-subjects experiments, list some of the pros and cons of each approach, and decide which approach to use to answer a particular research question.
- Define random assignment, distinguish it from random sampling, explain its purpose in experimental research, and use some simple strategies to implement it.
- Define what a control condition is, explain its purpose in research on treatment effectiveness, and describe some alternative types of control conditions.
- Define several types of carryover effect, give examples of each, and explain how counterbalancing helps to deal with them.
In this section, we look at some different ways to design an experiment. The primary distinction we will make is between approaches in which each participant experiences one level of the independent variable and approaches in which each participant experiences all levels of the independent variable. The former are called between-subjects experiments and the latter are called within-subjects experiments.
Between-Subjects Experiments
In a between-subjects experiment , each participant is tested in only one condition. For example, a researcher with a sample of 100 university students might assign half of them to write about a traumatic event and the other half write about a neutral event. Or a researcher with a sample of 60 people with severe agoraphobia (fear of open spaces) might assign 20 of them to receive each of three different treatments for that disorder. It is essential in a between-subjects experiment that the researcher assign participants to conditions so that the different groups are, on average, highly similar to each other. Those in a trauma condition and a neutral condition, for example, should include a similar proportion of men and women, and they should have similar average intelligence quotients (IQs), similar average levels of motivation, similar average numbers of health problems, and so on. This matching is a matter of controlling these extraneous participant variables across conditions so that they do not become confounding variables.
Random Assignment
The primary way that researchers accomplish this kind of control of extraneous variables across conditions is called random assignment , which means using a random process to decide which participants are tested in which conditions. Do not confuse random assignment with random sampling. Random sampling is a method for selecting a sample from a population, and it is rarely used in psychological research. Random assignment is a method for assigning participants in a sample to the different conditions, and it is an important element of all experimental research in psychology and other fields too.
In its strictest sense, random assignment should meet two criteria. One is that each participant has an equal chance of being assigned to each condition (e.g., a 50% chance of being assigned to each of two conditions). The second is that each participant is assigned to a condition independently of other participants. Thus one way to assign participants to two conditions would be to flip a coin for each one. If the coin lands heads, the participant is assigned to Condition A, and if it lands tails, the participant is assigned to Condition B. For three conditions, one could use a computer to generate a random integer from 1 to 3 for each participant. If the integer is 1, the participant is assigned to Condition A; if it is 2, the participant is assigned to Condition B; and if it is 3, the participant is assigned to Condition C. In practice, a full sequence of conditions—one for each participant expected to be in the experiment—is usually created ahead of time, and each new participant is assigned to the next condition in the sequence as he or she is tested. When the procedure is computerized, the computer program often handles the random assignment.
One problem with coin flipping and other strict procedures for random assignment is that they are likely to result in unequal sample sizes in the different conditions. Unequal sample sizes are generally not a serious problem, and you should never throw away data you have already collected to achieve equal sample sizes. However, for a fixed number of participants, it is statistically most efficient to divide them into equal-sized groups. It is standard practice, therefore, to use a kind of modified random assignment that keeps the number of participants in each group as similar as possible. One approach is block randomization . In block randomization, all the conditions occur once in the sequence before any of them is repeated. Then they all occur again before any of them is repeated again. Within each of these “blocks,” the conditions occur in a random order. Again, the sequence of conditions is usually generated before any participants are tested, and each new participant is assigned to the next condition in the sequence. Table 6.2 shows such a sequence for assigning nine participants to three conditions. The Research Randomizer website will generate block randomization sequences for any number of participants and conditions. Again, when the procedure is computerized, the computer program often handles the block randomization.
Participant | Condition |
---|---|
1 | A |
2 | C |
3 | B |
4 | B |
5 | C |
6 | A |
7 | C |
8 | B |
9 | A |
Random assignment is not guaranteed to control all extraneous variables across conditions. It is always possible that just by chance, the participants in one condition might turn out to be substantially older, less tired, more motivated, or less depressed on average than the participants in another condition. However, there are some reasons that this possibility is not a major concern. One is that random assignment works better than one might expect, especially for large samples. Another is that the inferential statistics that researchers use to decide whether a difference between groups reflects a difference in the population takes the “fallibility” of random assignment into account. Yet another reason is that even if random assignment does result in a confounding variable and therefore produces misleading results, this confound is likely to be detected when the experiment is replicated. The upshot is that random assignment to conditions—although not infallible in terms of controlling extraneous variables—is always considered a strength of a research design.
Treatment and Control Conditions
Between-subjects experiments are often used to determine whether a treatment works. In psychological research, a treatment is any intervention meant to change people’s behaviour for the better. This intervention includes psychotherapies and medical treatments for psychological disorders but also interventions designed to improve learning, promote conservation, reduce prejudice, and so on. To determine whether a treatment works, participants are randomly assigned to either a treatment condition , in which they receive the treatment, or a control condition , in which they do not receive the treatment. If participants in the treatment condition end up better off than participants in the control condition—for example, they are less depressed, learn faster, conserve more, express less prejudice—then the researcher can conclude that the treatment works. In research on the effectiveness of psychotherapies and medical treatments, this type of experiment is often called a randomized clinical trial .
There are different types of control conditions. In a no-treatment control condition , participants receive no treatment whatsoever. One problem with this approach, however, is the existence of placebo effects. A placebo is a simulated treatment that lacks any active ingredient or element that should make it effective, and a placebo effect is a positive effect of such a treatment. Many folk remedies that seem to work—such as eating chicken soup for a cold or placing soap under the bedsheets to stop nighttime leg cramps—are probably nothing more than placebos. Although placebo effects are not well understood, they are probably driven primarily by people’s expectations that they will improve. Having the expectation to improve can result in reduced stress, anxiety, and depression, which can alter perceptions and even improve immune system functioning (Price, Finniss, & Benedetti, 2008) [1] .
Placebo effects are interesting in their own right (see Note “The Powerful Placebo” ), but they also pose a serious problem for researchers who want to determine whether a treatment works. Figure 6.2 shows some hypothetical results in which participants in a treatment condition improved more on average than participants in a no-treatment control condition. If these conditions (the two leftmost bars in Figure 6.2 ) were the only conditions in this experiment, however, one could not conclude that the treatment worked. It could be instead that participants in the treatment group improved more because they expected to improve, while those in the no-treatment control condition did not.
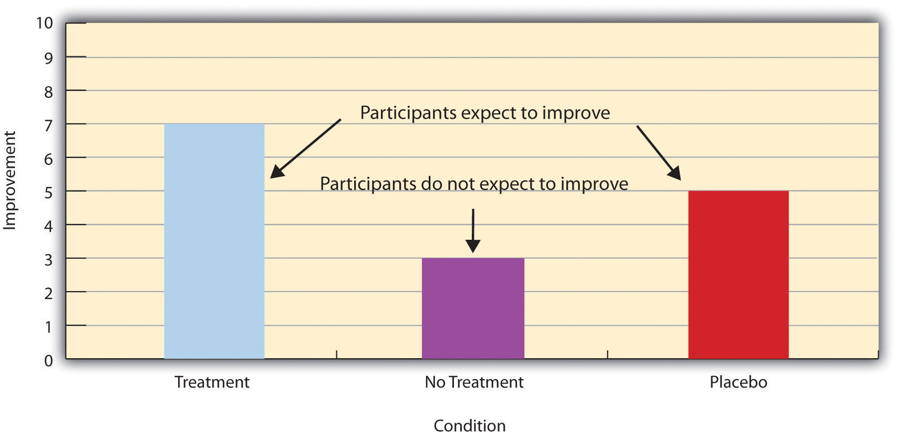
Fortunately, there are several solutions to this problem. One is to include a placebo control condition , in which participants receive a placebo that looks much like the treatment but lacks the active ingredient or element thought to be responsible for the treatment’s effectiveness. When participants in a treatment condition take a pill, for example, then those in a placebo control condition would take an identical-looking pill that lacks the active ingredient in the treatment (a “sugar pill”). In research on psychotherapy effectiveness, the placebo might involve going to a psychotherapist and talking in an unstructured way about one’s problems. The idea is that if participants in both the treatment and the placebo control groups expect to improve, then any improvement in the treatment group over and above that in the placebo control group must have been caused by the treatment and not by participants’ expectations. This difference is what is shown by a comparison of the two outer bars in Figure 6.2 .
Of course, the principle of informed consent requires that participants be told that they will be assigned to either a treatment or a placebo control condition—even though they cannot be told which until the experiment ends. In many cases the participants who had been in the control condition are then offered an opportunity to have the real treatment. An alternative approach is to use a waitlist control condition , in which participants are told that they will receive the treatment but must wait until the participants in the treatment condition have already received it. This disclosure allows researchers to compare participants who have received the treatment with participants who are not currently receiving it but who still expect to improve (eventually). A final solution to the problem of placebo effects is to leave out the control condition completely and compare any new treatment with the best available alternative treatment. For example, a new treatment for simple phobia could be compared with standard exposure therapy. Because participants in both conditions receive a treatment, their expectations about improvement should be similar. This approach also makes sense because once there is an effective treatment, the interesting question about a new treatment is not simply “Does it work?” but “Does it work better than what is already available?
The Powerful Placebo
Many people are not surprised that placebos can have a positive effect on disorders that seem fundamentally psychological, including depression, anxiety, and insomnia. However, placebos can also have a positive effect on disorders that most people think of as fundamentally physiological. These include asthma, ulcers, and warts (Shapiro & Shapiro, 1999) [2] . There is even evidence that placebo surgery—also called “sham surgery”—can be as effective as actual surgery.
Medical researcher J. Bruce Moseley and his colleagues conducted a study on the effectiveness of two arthroscopic surgery procedures for osteoarthritis of the knee (Moseley et al., 2002) [3] . The control participants in this study were prepped for surgery, received a tranquilizer, and even received three small incisions in their knees. But they did not receive the actual arthroscopic surgical procedure. The surprising result was that all participants improved in terms of both knee pain and function, and the sham surgery group improved just as much as the treatment groups. According to the researchers, “This study provides strong evidence that arthroscopic lavage with or without débridement [the surgical procedures used] is not better than and appears to be equivalent to a placebo procedure in improving knee pain and self-reported function” (p. 85).
Within-Subjects Experiments
In a within-subjects experiment , each participant is tested under all conditions. Consider an experiment on the effect of a defendant’s physical attractiveness on judgments of his guilt. Again, in a between-subjects experiment, one group of participants would be shown an attractive defendant and asked to judge his guilt, and another group of participants would be shown an unattractive defendant and asked to judge his guilt. In a within-subjects experiment, however, the same group of participants would judge the guilt of both an attractive and an unattractive defendant.
The primary advantage of this approach is that it provides maximum control of extraneous participant variables. Participants in all conditions have the same mean IQ, same socioeconomic status, same number of siblings, and so on—because they are the very same people. Within-subjects experiments also make it possible to use statistical procedures that remove the effect of these extraneous participant variables on the dependent variable and therefore make the data less “noisy” and the effect of the independent variable easier to detect. We will look more closely at this idea later in the book. However, not all experiments can use a within-subjects design nor would it be desirable to.
Carryover Effects and Counterbalancing
The primary disad vantage of within-subjects designs is that they can result in carryover effects. A carryover effect is an effect of being tested in one condition on participants’ behaviour in later conditions. One type of carryover effect is a practice effect , where participants perform a task better in later conditions because they have had a chance to practice it. Another type is a fatigue effect , where participants perform a task worse in later conditions because they become tired or bored. Being tested in one condition can also change how participants perceive stimuli or interpret their task in later conditions. This type of effect is called a context effect . For example, an average-looking defendant might be judged more harshly when participants have just judged an attractive defendant than when they have just judged an unattractive defendant. Within-subjects experiments also make it easier for participants to guess the hypothesis. For example, a participant who is asked to judge the guilt of an attractive defendant and then is asked to judge the guilt of an unattractive defendant is likely to guess that the hypothesis is that defendant attractiveness affects judgments of guilt. This knowledge could lead the participant to judge the unattractive defendant more harshly because he thinks this is what he is expected to do. Or it could make participants judge the two defendants similarly in an effort to be “fair.”
Carryover effects can be interesting in their own right. (Does the attractiveness of one person depend on the attractiveness of other people that we have seen recently?) But when they are not the focus of the research, carryover effects can be problematic. Imagine, for example, that participants judge the guilt of an attractive defendant and then judge the guilt of an unattractive defendant. If they judge the unattractive defendant more harshly, this might be because of his unattractiveness. But it could be instead that they judge him more harshly because they are becoming bored or tired. In other words, the order of the conditions is a confounding variable. The attractive condition is always the first condition and the unattractive condition the second. Thus any difference between the conditions in terms of the dependent variable could be caused by the order of the conditions and not the independent variable itself.
There is a solution to the problem of order effects, however, that can be used in many situations. It is counterbalancing , which means testing different participants in different orders. For example, some participants would be tested in the attractive defendant condition followed by the unattractive defendant condition, and others would be tested in the unattractive condition followed by the attractive condition. With three conditions, there would be six different orders (ABC, ACB, BAC, BCA, CAB, and CBA), so some participants would be tested in each of the six orders. With counterbalancing, participants are assigned to orders randomly, using the techniques we have already discussed. Thus random assignment plays an important role in within-subjects designs just as in between-subjects designs. Here, instead of randomly assigning to conditions, they are randomly assigned to different orders of conditions. In fact, it can safely be said that if a study does not involve random assignment in one form or another, it is not an experiment.
An efficient way of counterbalancing is through a Latin square design which randomizes through having equal rows and columns. For example, if you have four treatments, you must have four versions. Like a Sudoku puzzle, no treatment can repeat in a row or column. For four versions of four treatments, the Latin square design would look like:
A | B | C | D |
B | C | D | A |
C | D | A | B |
D | A | B | C |
There are two ways to think about what counterbalancing accomplishes. One is that it controls the order of conditions so that it is no longer a confounding variable. Instead of the attractive condition always being first and the unattractive condition always being second, the attractive condition comes first for some participants and second for others. Likewise, the unattractive condition comes first for some participants and second for others. Thus any overall difference in the dependent variable between the two conditions cannot have been caused by the order of conditions. A second way to think about what counterbalancing accomplishes is that if there are carryover effects, it makes it possible to detect them. One can analyze the data separately for each order to see whether it had an effect.
When 9 is “larger” than 221
Researcher Michael Birnbaum has argued that the lack of context provided by between-subjects designs is often a bigger problem than the context effects created by within-subjects designs. To demonstrate this problem, he asked participants to rate two numbers on how large they were on a scale of 1-to-10 where 1 was “very very small” and 10 was “very very large”. One group of participants were asked to rate the number 9 and another group was asked to rate the number 221 (Birnbaum, 1999) [4] . Participants in this between-subjects design gave the number 9 a mean rating of 5.13 and the number 221 a mean rating of 3.10. In other words, they rated 9 as larger than 221! According to Birnbaum, this difference is because participants spontaneously compared 9 with other one-digit numbers (in which case it is relatively large) and compared 221 with other three-digit numbers (in which case it is relatively small) .
Simultaneous Within-Subjects Designs
So far, we have discussed an approach to within-subjects designs in which participants are tested in one condition at a time. There is another approach, however, that is often used when participants make multiple responses in each condition. Imagine, for example, that participants judge the guilt of 10 attractive defendants and 10 unattractive defendants. Instead of having people make judgments about all 10 defendants of one type followed by all 10 defendants of the other type, the researcher could present all 20 defendants in a sequence that mixed the two types. The researcher could then compute each participant’s mean rating for each type of defendant. Or imagine an experiment designed to see whether people with social anxiety disorder remember negative adjectives (e.g., “stupid,” “incompetent”) better than positive ones (e.g., “happy,” “productive”). The researcher could have participants study a single list that includes both kinds of words and then have them try to recall as many words as possible. The researcher could then count the number of each type of word that was recalled. There are many ways to determine the order in which the stimuli are presented, but one common way is to generate a different random order for each participant.
Between-Subjects or Within-Subjects?
Almost every experiment can be conducted using either a between-subjects design or a within-subjects design. This possibility means that researchers must choose between the two approaches based on their relative merits for the particular situation.
Between-subjects experiments have the advantage of being conceptually simpler and requiring less testing time per participant. They also avoid carryover effects without the need for counterbalancing. Within-subjects experiments have the advantage of controlling extraneous participant variables, which generally reduces noise in the data and makes it easier to detect a relationship between the independent and dependent variables.
A good rule of thumb, then, is that if it is possible to conduct a within-subjects experiment (with proper counterbalancing) in the time that is available per participant—and you have no serious concerns about carryover effects—this design is probably the best option. If a within-subjects design would be difficult or impossible to carry out, then you should consider a between-subjects design instead. For example, if you were testing participants in a doctor’s waiting room or shoppers in line at a grocery store, you might not have enough time to test each participant in all conditions and therefore would opt for a between-subjects design. Or imagine you were trying to reduce people’s level of prejudice by having them interact with someone of another race. A within-subjects design with counterbalancing would require testing some participants in the treatment condition first and then in a control condition. But if the treatment works and reduces people’s level of prejudice, then they would no longer be suitable for testing in the control condition. This difficulty is true for many designs that involve a treatment meant to produce long-term change in participants’ behaviour (e.g., studies testing the effectiveness of psychotherapy). Clearly, a between-subjects design would be necessary here.
Remember also that using one type of design does not preclude using the other type in a different study. There is no reason that a researcher could not use both a between-subjects design and a within-subjects design to answer the same research question. In fact, professional researchers often take exactly this type of mixed methods approach.
Key Takeaways
- Experiments can be conducted using either between-subjects or within-subjects designs. Deciding which to use in a particular situation requires careful consideration of the pros and cons of each approach.
- Random assignment to conditions in between-subjects experiments or to orders of conditions in within-subjects experiments is a fundamental element of experimental research. Its purpose is to control extraneous variables so that they do not become confounding variables.
- Experimental research on the effectiveness of a treatment requires both a treatment condition and a control condition, which can be a no-treatment control condition, a placebo control condition, or a waitlist control condition. Experimental treatments can also be compared with the best available alternative.
- You want to test the relative effectiveness of two training programs for running a marathon.
- Using photographs of people as stimuli, you want to see if smiling people are perceived as more intelligent than people who are not smiling.
- In a field experiment, you want to see if the way a panhandler is dressed (neatly vs. sloppily) affects whether or not passersby give him any money.
- You want to see if concrete nouns (e.g., dog ) are recalled better than abstract nouns (e.g., truth ).
- Discussion: Imagine that an experiment shows that participants who receive psychodynamic therapy for a dog phobia improve more than participants in a no-treatment control group. Explain a fundamental problem with this research design and at least two ways that it might be corrected.
- Price, D. D., Finniss, D. G., & Benedetti, F. (2008). A comprehensive review of the placebo effect: Recent advances and current thought. Annual Review of Psychology, 59 , 565–590. ↵
- Shapiro, A. K., & Shapiro, E. (1999). The powerful placebo: From ancient priest to modern physician . Baltimore, MD: Johns Hopkins University Press. ↵
- Moseley, J. B., O’Malley, K., Petersen, N. J., Menke, T. J., Brody, B. A., Kuykendall, D. H., … Wray, N. P. (2002). A controlled trial of arthroscopic surgery for osteoarthritis of the knee. The New England Journal of Medicine, 347 , 81–88. ↵
- Birnbaum, M.H. (1999). How to show that 9>221: Collect judgments in a between-subjects design. Psychological Methods, 4(3), 243-249. ↵
An experiment in which each participant is only tested in one condition.
A method of controlling extraneous variables across conditions by using a random process to decide which participants will be tested in the different conditions.
All the conditions of an experiment occur once in the sequence before any of them is repeated.
Any intervention meant to change people’s behaviour for the better.
A condition in a study where participants receive treatment.
A condition in a study that the other condition is compared to. This group does not receive the treatment or intervention that the other conditions do.
A type of experiment to research the effectiveness of psychotherapies and medical treatments.
A type of control condition in which participants receive no treatment.
A simulated treatment that lacks any active ingredient or element that should make it effective.
A positive effect of a treatment that lacks any active ingredient or element to make it effective.
Participants receive a placebo that looks like the treatment but lacks the active ingredient or element thought to be responsible for the treatment’s effectiveness.
Participants are told that they will receive the treatment but must wait until the participants in the treatment condition have already received it.
Each participant is tested under all conditions.
An effect of being tested in one condition on participants’ behaviour in later conditions.
Participants perform a task better in later conditions because they have had a chance to practice it.
Participants perform a task worse in later conditions because they become tired or bored.
Being tested in one condition can also change how participants perceive stimuli or interpret their task in later conditions.
Testing different participants in different orders.
Research Methods in Psychology - 2nd Canadian Edition Copyright © 2015 by Paul C. Price, Rajiv Jhangiani, & I-Chant A. Chiang is licensed under a Creative Commons Attribution-NonCommercial-ShareAlike 4.0 International License , except where otherwise noted.
Share This Book
Click through the PLOS taxonomy to find articles in your field.
For more information about PLOS Subject Areas, click here .
Loading metrics
Open Access
Peer-reviewed
Research Article
Police violence reduces trust in the police among Black residents
Contributed equally to this work with: Jonathan Ben-Menachem, Gerard Torrats-Espinosa
Roles Conceptualization, Methodology, Writing – original draft, Writing – review & editing
* E-mail: [email protected] (JBM); [email protected] (GTE)
Affiliation Department of Sociology, Columbia University, New York, New York, United States of America

Roles Conceptualization, Data curation, Formal analysis, Methodology, Project administration, Supervision, Visualization, Writing – original draft, Writing – review & editing
- Jonathan Ben-Menachem,
- Gerard Torrats-Espinosa
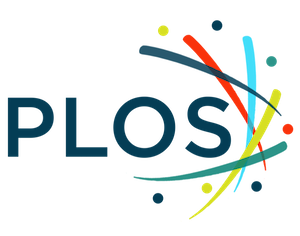
- Published: September 11, 2024
- https://doi.org/10.1371/journal.pone.0308487
- Reader Comments
Recent high-profile incidents involving the shooting or killing of unarmed Black men have intensified the debate about how police violence affects trust in the criminal justice system, particularly among communities of color. In this article, we propose a quasi-experimental design that leverages the timing of the shooting of Jacob Blake by the Kenosha Police Department relative to when a large survey was fielded in the city of Chicago. We demonstrate that individuals interviewed 4 weeks before and 4 weeks after the shooting are comparable across a large set of observed characteristics, thus approximating an experimental setting. We find that Blake’s shooting caused substantial reductions in Black respondents’ trust in the police, concentrated among younger residents and criminalized residents. These results suggest that police violence against racial minorities may lead to lower civic engagement and cooperation with law enforcement in those communities, exacerbating issues of public safety and community well-being. The pronounced distrust among younger Black residents suggests a generational rift that could risk further entrenching systemic biases and inequalities within the criminal justice system. Additionally, the higher levels of distrust among criminalized respondents could have implications for research detailing this population’s decreased willingness to engage with public institutions more broadly.
Citation: Ben-Menachem J, Torrats-Espinosa G (2024) Police violence reduces trust in the police among Black residents. PLoS ONE 19(9): e0308487. https://doi.org/10.1371/journal.pone.0308487
Editor: W. David Allen, University of Alabama in Huntsville, UNITED STATES OF AMERICA
Received: April 4, 2024; Accepted: July 22, 2024; Published: September 11, 2024
Copyright: © 2024 Ben-Menachem, Torrats-Espinosa. This is an open access article distributed under the terms of the Creative Commons Attribution License , which permits unrestricted use, distribution, and reproduction in any medium, provided the original author and source are credited.
Data Availability: The data and code used in our analyses is available here: https://doi.org/10.7910/DVN/CO404V .
Funding: The author(s) received no specific funding for this work.
Competing interests: The authors have declared that no competing interests exist.
Introduction
In recent years, the escalation of high-profile incidents of police violence, particularly against members of the Black community, has brought police violence to the forefront of domestic policy debates. From 2015 to 2020, there were 4,740 civilians fatally shot by police in the U.S., with a disproportionately high 26.7% being Black, while Hispanic fatalities stood at 18.8%, and white fatalities accounted for 51% of these cases [ 1 ]. This over-representation of minorities in fatal police encounters signifies a distressing trend that has made police violence a leading cause of death among young minority men [ 2 ].
The publicized killings of individuals such as Eric Garner, Philando Castile, George Floyd, and Jacob Blake have not only captured national attention but have also sparked a reevaluation of the role of law enforcement in society. Such incidents, often documented on video and spread across social media and news outlets, provide a raw glimpse into the interactions between police officers and Black individuals, potentially influencing public perceptions and trust in the police as an institution. These highly publicized events of police violence can change police-community dynamics. Individuals who have not been victims of police violence themselves may lose trust in law enforcement if they perceive the police as a discriminatory institution that systematically targets their racial group. This cycle of mistrust can result in a downward spiral where a heightened sense of alienation fuels more violence, particularly in the communities where police violence is high. Prior research finds that individuals reporting low trust in police are more likely to acquire a firearm for self-protection, either from nearby residents or from the police themselves [ 3 , 4 ].
The relationship between law enforcement and the communities they serve has been a focal point of sociological research for decades [ 5 – 7 ]. Central to this discourse is the issue of trust in the criminal justice system, particularly among communities of color. Legal cynicism, or the belief in the incompetence and illegitimacy of the criminal justice system, is prevalent in many minority communities [ 8 ]. This cynicism, exacerbated by incidents of police violence, can lead to a reluctance to cooperate with law enforcement agencies.
Prior research has examined the extent to which police violence and misconduct perpetuate legal cynicism using interviews and correlational survey designs, finding that police contact can alienate residents who are subjected to it [ 9 – 11 ]. A growing number of studies have relied on 911 calls to document how city- and neighborhood-level patterns of crime reporting decline following police violence [ 7 , 12 ]. Despite their important contributions, efforts to identify causal effects of police violence events have limitations. 911 calls may not be a reliable proxy to capture underlying trust in law enforcement. Furthermore, such studies often rely on data at the neighborhood or area level. The use of aggregate data poses challenges for causal inference and prevents the assessment of heterogeneous effects across different demographic groups.
The present study investigates how highly publicized events of police violence change trust in law enforcement, examining effect heterogeneity across race and ethnicity, age, and contact with the criminal legal system. These features of our research design could have implications for behaviors beyond cooperation with law enforcement such as system avoidance [ 13 ] or political participation [ 14 ].
To estimate the causal effect of police violence on trust in law enforcement, we leverage individual-level survey data in a quasi-experimental design that exploits the timing of the shooting of Jacob Blake (on August 23, 2020) relative to the fielding of the 2020 Healthy Chicago Survey (HCS), a survey of Chicago residents that the Chicago Department of Public Health ran between June 27, 2020 and December 9, 2020. Due to sample size limitations that prevent us to test effects by age, gender, and prior arrest record for other racial groups, our study primarily focuses on Black and White individuals. That being said, we do show results of an analysis of all Hispanic respondents in the S1 File . We show that individuals interviewed 4 weeks before and 4 weeks after the shooting took place are statistically identical. This enables a research design in which the group surveyed 4 weeks before the events serves as a plausible counterfactual for the group surveyed 4 weeks after.
We find large declines in trust in law enforcement among Black residents in Chicago after the shooting of Jacob Blake. During the two weeks that followed the shooting, trust in law enforcement declined by an average of 15 percentage points, a 31-percent decline from its baseline before the shooting. For Hispanic and white residents, trust in law enforcement remained unchanged. Among Black young adults (ages 18–44), law enforcement trust declined by up to 32 percentage points, with this effect lasting for three weeks. Among Black individuals who reported prior contact with the criminal legal system (e.g., having been arrested, booked, or charged at some point in the past), law enforcement trust declined by up to 36 percentage points in the two weeks following the shooting.
Our focus on individuals with prior exposure to policing and incarceration has theoretical and methodological importance; data limitations have made it difficult for researchers to identify the causal effect of police violence on institutional trust for this group. Generating causal evidence on the impact of police violence on trust is particularly important given the growing evidence showing that aggressive policing practices and police violence disproportionately affect people of color, low-income individuals, and other marginalized groups [ 15 – 17 ]. Accordingly, for sociologists of punishment, our findings constitute new evidence regarding the influence of police violence on perceptions of police among the most-policed communities. For political sociologists and political scientists, we contribute to a fast-growing literature showing how the behavior of “street-level bureaucrats” (rather than e.g. Presidential candidates) can shape citizens’ orientation toward government institutions [ 14 ].
Literature and theory
American trust in police has been historically theorized via police legitimacy, or a person’s linked perception of law enforcement and their own obligation to obey the law [ 11 ]. Legitimacy theory is complemented by the concept of legal cynicism, a cultural frame in criminalized communities holding that law enforcement agencies are illegitimate, inadequate, or otherwise harmful [ 8 , 18 ]. Bell further develops the concept of legal estrangement, wherein the experience of living in heavily policed communities reinforces a broader sense of marginalization at the hands of the law [ 19 ].
Empirical work measuring these interrelated concepts historically involved interviews or surveys digging into the mechanisms that drive e.g. Black Americans’ comparatively lower trust in police [ 5 , 20 ]. Many such studies examine the connection between legitimacy and gun violence [ 3 , 21 ]. A related body of work incorporates administrative data to assess whether events that negatively affect perceptions of police can also reduce residents’ willingness to report crimes or cooperate with law enforcement. This measure is consistent with the logic of police legitimacy, i.e., people who distrust the police are less likely to perceive reporting a crime to police as a viable solution. Desmond and coauthors found that calls for service in Milwaukee were reduced following the police beating of Frank Jude [ 7 ]. While this finding has been revised [ 22 , 23 ], recent research found a similar negative effect following the murder of George Floyd [ 12 ].
This literature overlaps with work on social organization and collective efficacy, or a group’s ability to achieve collectively desired goals [ 24 , 25 ]. Work in this spatial-ecological tradition has examined the connection between differential rates of interpersonal violence and interrelated measures of police legitimacy and willingness to enact informal social controls. Police violence may hinder collective efficacy in part by eroding trust in law enforcement and government institutions, particularly in communities where police violence is more prevalent.
Two recent studies provide strong evidence regarding the causal effects of police violence on trust. White and coauthors leverage a quasi-experimental design to assess the effect of the police killing of Freddie Gray on Baltimore residents’ perceptions of police legitimacy, but did not observe changes in perceptions of police. The authors suggest that this could follow from the lack of video footage depicting police brutality [ 26 ]. Additionally, their sample was not intended to be representative of Baltimore residents, but instead clustered in “hot spots.” Reny and Newman use a regression discontinuity design with nationally representative survey data to gauge the effect of George Floyd protests on perceptions of police, finding that protests diminished police favorability reports among politically liberal and “low-prejudice” respondents [ 27 ]. Although this study focuses on the effects of police brutality protests rather than police violence itself, these phenomena are tightly linked.
Apart from studies examining trust in police, recent work has improved the scholarly understanding of behaviors which follow from criminal legal contact (including police violence). Scholars argue that routine encounters with law enforcement constitute significant learning experiences with respect to government and one’s relationship with it [ 14 , 28 ]. Political science studies detailing these dynamics generally contribute to prior theories of political socialization and focus on outcomes including voting or other political behaviors like volunteering for political candidates. Such work finds that many forms of criminal legal contact reduce voter turnout, often with pronounced effects for Black or Latinx residents [ 29 – 31 ]. This work focuses on personal and proximal contact–being personally arrested or learning about the arrest of a friend or family member. Yet criminal legal contact may also affect perceptions of police through less direct channels. To that end, Morris and Shoub theorize “community contact”: “diffuse contact an individual has with the police via community incidents, word of mouth, and/or the media” [ 32 ]. The observed community-level effect of police violence supports a key assumption of our own analysis–that police violence can politically socialize Americans who are not personally subjected to it.
The preceding literature motivates our first hypothesis (H1): Jacob Blake’s shooting negatively affected perceptions of police for both white and Black respondents. We expect to see an effect among Black respondents in large part because Jacob Blake is Black; Morris and Shoub find stronger effects of police violence against Black victims on other Black residents’ political behavior [ 32 ]. Yet other recent work found more strongly diminished trust in police for white residents compared to Black residents [ 27 , 33 ]. These apparently contradictory findings suggest that this theoretical space is still developing.
Sociologists have argued that criminal legal contact can shape individuals’ orientations towards a wide variety of public institutions, including but not limited to law enforcement. In her analysis of “system avoidance,” Brayne found that criminalized respondents were less likely to interact with institutions that might be perceived as surveilling due to a fear of re-arrest (e.g. hospitals, schools, banks), and the negative effect intensified alongside criminal legal contact (police stops, arrests, conviction, and incarceration) [ 13 ]. These findings were further substantiated by Remster and Kramer [ 34 ], and they track with the increasing severity of withdrawal observed in the political participation literature cited above (e.g. [ 29 ]). The system avoidance literature informs our second hypothesis (H2): We expect the effect of Jacob Blake’s shooting to be more pronounced for residents who have had contact with the criminal justice system in the past (e.g., having been arrested, booked, jailed, or incarcerated) .
Prior research leads us to believe that observed effects are likely to vary by age and gender. Exploring effect heterogeneity by age is important given that young adults and early middle-aged individuals play a key role in political activism and social movements [ 35 ]–and people who recently attended protests were much younger than the general population [ 36 ]. The presence of a strong narrative of racial injustice can compel members of oft-criminalized communities to participate in non-voting political behaviors such as signing petitions or volunteering for candidates [ 37 , 38 ]. This theory is compatible with legal cynicism or legal estrangement to the extent that individual grievances are newly construed as signs of group-level inequality and accords with prior accounts of criminal legal contact catalyzing heightened political consciousness [ 17 ]. Protests are one site where such narratives could be cultivated and propagated. To the extent that protests may politically socialize participants [ 27 , 32 ] we can propose our third hypothesis (H3): The negative effect of Jacob Blake’s shooting on perceptions of police was more pronounced for younger Chicagoans .
Evidence on the gendered experience of police encounters and police violence suggests that men, particularly those from minority groups, may be more likely to experience racial profiling, excessive use of force, or discriminatory treatment [ 15 , 17 , 39 ]. Such experiences can create a higher likelihood of mistrust and negative perceptions of the police. Yet women are also subjected to similarly alienating forms of police misconduct; for instance, young Black women’s views of police are colored by sexual harassment and sexual violence, and Black mothers consistently report fears that their children will experience police violence [ 9 , 18 , 40 ]. Accordingly, we formulate our fourth hypothesis (H4): We expect trust in law enforcement to be similarly affected across Black men and women .
To sum up, police legitimacy theory proposes that unjust interactions with police or high-profile police violence events experienced vicariously lead to reduced trust in police, thus leading to reduced legal compliance. The present study differs from past literature in that we estimate individual-level average causal effects and stratify our analyses along a wide range of individual characteristics.
The events in Kenosha in August of 2020
The summer of 2020 in the United States was marked by racial tensions and social upheaval. The killing of George Floyd, a Black man, by a Minneapolis police officer on May 25, 2020 sparked nationwide protests. Floyd’s death became a symbol of entrenched systemic racism and police brutality. These racial tensions were further complicated by the COVID-19 pandemic, which disproportionately affected communities of color, exposing deep-seated disparities in healthcare, economic opportunities, and education.
It was against this backdrop that the shooting of Jacob Blake took place in Kenosha, Wisconsin. On August 23, 2020, officers responded to a domestic disturbance call involving Blake, a 29-year-old African-American who was unarmed. As Blake walked away from the officers, he was grabbed by his shirt and shot multiple times in the back by Officer Rusten Sheskey. Blake survived, but was left paralyzed from the waist down. The incident was captured on video and went viral, igniting widespread outrage and protests both in Kenosha and across the nation for 8 days following the shooting. The city declared a state of emergency, and the National Guard was deployed.
Amidst these protests, Kyle Rittenhouse, a white 17-year-old from Antioch, Illinois, crossed state lines armed with an AR-15-style rifle and arrived at Kenosha on August 24. Rittenhouse claimed he went to Kenosha to protect local businesses and provide medical aid, but on the night of August 25, 2020, during ongoing protests, Rittenhouse was involved in a series of confrontations that culminated in the shooting of three individuals.
This adds a layer of complexity to our study as it becomes difficult to disentangle the effects driven by the shooting of Jacob Blake from those driven by the Rittenhouse events. It could be that observed changes in trust in the week that followed Blake’s shooting resulted from the frustration of seeing police officers do nothing against an armed White man who shot three protesters. We will address these concerns in our empirical strategy by assessing the extent to which changes in trust in law enforcement were already visible in the two days before Rittenhouse shot three protesters.
Identifying the causal effect of police violence on trust in the police is complicated by the fact that exposure to these events is not random. Black Americans are simultaneously more likely to be victims of lethal and aggressive police violence [ 15 ] and to show lower levels of trust in the police [ 41 ]. Although previous studies relying on survey data have shown that individuals with prior contact with the police report lower levels of trust in government [ 42 ], establishing a causal relationship between the experience of police violence and trust in law enforcement can be challenging. Correlational evidence may not accurately capture the sequence of events; an individual who reports low trust following a police violence event may have lost trust long before that event, suggesting reverse causality. Similarly, self-reported data often lack control over confounding variables such as racial identity and the experience of police violence that may influence both the experience of police violence and trust in the police. Without accounting for these variables, it is challenging to establish a direct causal relationship between police violence and trust.
In this study, we overcome these challenges by using a quasi-experimental design in which we leverage the timing of a police violence event relative to a wave of survey data collection. This approach is commonly called “unexpected event during survey design,” or UESD [ 43 , 44 ]. Under the assumptions that underlie this research design, individuals surveyed before the shooting took place serve as plausible counterfactuals for individuals surveyed after the shooting. We assess the validity of this assumption in the next section.
To set up the UESD to answer our research questions, we link data from a survey that was fielded in Chicago around the time when the shooting of Jacob Blake took place (August 23, 2020). Our identification strategy relies on the exogenous timing of the shooting relative to when the survey was fielded. This allows us to compare the answers to questions about trust in the police across two sets of survey participants: those interviewed four weeks before the shooting took place and those interviewed four weeks after. We show that these two sets of respondents are statistically identical in terms of a large set of observed attributes.
Our analysis draws on Healthy Chicago Survey (HCS) data collected by the Chicago Department of Public Health between June 27 and December 9, 2020. The survey has been used to identify health concerns for each community in Chicago and to understand environmental, neighborhood, and social factors associated with health. It has been conducted annually since 2014, but the 2020 wave was the first iteration including questions about trust in police. In 2020, the survey’s format also shifted from a random-digit dial telephone survey to a self-administered, mixed-mode design wherein informants sampled by address could complete the survey online or by sending in a pencil-and-paper form.
The sampling frame in the HCS survey ensured that the data were representative of the Chicago population. RTI International, the organization in charge of designing and implementing the 2020 HCS survey, geocoded all Chicago postal addresses (N = 1,201,979) and nested them from the 77 community areas that divide the city of Chicago. From that universe of addresses, RTI targeted a minimum of 35 survey completes within each of the 77 community areas and a total of 4,500 survey completes overall. We refer readers to the 2020 Healthy Chicago Survey Methodology Report for additional details on the design and implementation of the survey [ 45 ]. The HCS sample includes 4,474 Chicago residents who are at least 18 years old. Due to its large sample size and city-wide coverage, a number of studies have used the HCS survey to study health outcomes in Chicago ([ 46 , 47 ]).
We focus on the subset of 584 Black and 939 white respondents interviewed during the eight weeks that surrounded the shooting. During that same period, only 389 Hispanic Chicagoans were surveyed, leaving us statistically under-powered to test all hypotheses for this group. The duration of this window is informed by prior studies using similar windows [ 48 ]. We focus on Black and white respondents due to statistical power limitations that prevent us from carrying out subgroup analyses for respondents of other racial and ethnic backgrounds. The constraints of the UESD design may raise concerns about the representativeness of our analytical sample. We evaluate these concerns by assessing differences across the pool of Black and white HCS survey respondents that made it to our analytical sample and Black and white HCS survey respondents that were excluded because they happened to be interviewed outside the four weeks before and after the shooting.
Ethics approval (i.e., IRB) for this study was not necessary because we are analyzing non-identifiable, publicly available survey data. All of the analyses are on secondary data, and there is no linkage of individual-level survey data to any other datasets.
The first column in Table 1 reports descriptive statistics for the subsample of Black and white respondents included in our UESD analyses. The second column shows descriptive statistics for Black and white respondents whom we excluded due to their survey response date being prior to the four weeks before or posterior to the four weeks after the shooting. We report proportions in the different categories that define race (Black and white); gender (male, female, and other gender identities); age (aged 18–29, 30–44, 45–64, and 65 and above); educational attainment (less than high school, high school diploma, and bachelor’s degree or more); home ownership status; employment status; having lived in the neighborhood for more than 5 years; having ever been arrested, booked, or jailed; and having any children. We find that our UESD analytical sample of 1,523 survey participants is almost identical to the 1,425 survey respondents that we excluded from the UESD analyses. One pattern that stands out from Table 1 is that the HCS data are not fully representative of the Chicago population. This is apparent by looking at the gender composition of the included and excluded subsamples, which are both a third male and two-thirds female. While RTI International randomly selected addresses to be contacted in each of the 77 community areas, they did not impose any restrictions on which adult member of the household would respond to the questionnaire. It is important to note, however, that demographic composition discrepancies do not pose any threats to the internal validity of our findings. 62% of respondents in our analytical sample are white and 38% are Black; 37% are male, 62% are female, and 1% identify with another gender; 16% have ages between 18 and 29, 26% have ages between 30 and 44, 31% have ages between 45 and 64, and 27% have ages 65 and above. When compared to the race, gender, and age composition of the rest of the HCS data, we don’t observe substantial differences except for the somewhat larger share of individuals aged 65 and above in our sample. Moving to educational attainment, we see that 3% of respondents in our sample did not complete high school, 13% ended their education with a high school degree only, 24% have completed some years of college education, and 58% have a college degree or more. These proportions are almost identical in the subsample of excluded HCS survey respondents. The last set of covariates show that 45% of our respondents are homeowners, 56% were employed at the time of the survey, 59% of them had lived in the neighborhood for at least 5 years, and 12% had been arrested, booked, or charged at some point in the past.
- PPT PowerPoint slide
- PNG larger image
- TIFF original image
https://doi.org/10.1371/journal.pone.0308487.t001
The outcome measures in our analysis of HCS data reflect respondents’ trust in local police. We construct the dependent variable from respondents’ answers to these this question: “To what extent do you trust your law enforcement agency?” The possible responses to this question include “a great extent, somewhat, a little, not at all.” We code these variables as binary, taking on value 1 for those that responded “a great extent” or “somewhat” and 0 for the rest. Fig 1 shows the distribution of responses to the questions that we use as outcomes regarding trust in police. We find that 49 percent of Black respondents express great or some trust in the police, with this percentage increasing to 69 for whites. In S1 Table in S1 File , we show regression-adjusted differences in trust across Black and White respondents.
Data are from the 2020 Healthy Chicago Survey. The sample includes 584 Black respondents and 939 white respondents interviewed in the four weeks prior and four weeks after the shooting of Jacob Blake. The survey question underlying the graphs is “To what extent do you trust your law enforcement agency?” .
https://doi.org/10.1371/journal.pone.0308487.g001
Fig 2 begins to examine changes in our outcome of interest in a temporal way. It presents the city-wide share of survey participants that reported trust in law enforcement in the days before and after Jacob Blake’s shooting, with separate panels for different racial groups: Black respondents (Panel a), Hispanic respondents (Panel b), and White respondents (Panel c). The horizontal axis of each graph represents the days from Jacob Blake’s shooting, with the day of the shooting denoted by a vertical red line at day 0. The vertical axis measures the share of respondents that trust the police, ranging from 0 to 1. Each panel’s data is represented by individual dots for each day, with a smoothed line to indicate the overall trend over time. In Panel (a), trust among Black respondents shows a noticeable decline beginning shortly before the shooting and reaching its nadir in the days immediately following the incident. The pattern indicates a sharp drop in trust in the day after the shooting, which then fluctuates but stays below the pre-incident levels for the observed period. In Panel (b), Hispanic respondents’ trust appears to fluctuate widely before and after the shooting, with a less pronounced dip in trust immediately after the shooting. Panel (c) shows the trust levels among White respondents, which does not exhibit a significant change at the time of the shooting but does show some fluctuation in the days following. Overall, the level of trust among white respondents remains relatively stable compared to Black respondents.
https://doi.org/10.1371/journal.pone.0308487.g002
S2 Table in S1 File shows the proportion of respondents who showed some or a great deal of trust in the police for the different subgroups for whom we estimate the impact of the shooting (i.e., race, race by age, race by criminal justice contact, and race by gender). The baseline levels of trust among the group of respondents surveyed in the four weeks before the shooting will be useful to interpret the relative size of the causal effects that our models estimate. We also show the number of respondents in each subgroup. For the analyses of Black respondents, our data include 430 respondents in the four weeks prior and 154 respondents in the four weeks after. The analyses of whites include 730 respondents in the four weeks prior and 209 respondents in the four weeks after. The sample shrinks substantially as we further stratify by other attributes. For example, for the analyses of respondents with prior contact with the police, we have 75 Black respondents in the four weeks prior and 26 in the four weeks after. For white respondents with prior contact with the police, we are left with 49 in the four weeks prior and 27 in the four weeks after. One clear pattern in S2 Table in S1 File is that there are fewer observations in the group that responded four weeks after the shooting. Across all subgroups analyses, individuals in post-shooting group are approximately evenly distributed across the four weeks that followed the event. This is a feature of the data rather than a bug. Looking at S1 Fig in S1 File , it is clear that the number of daily survey responses was generally declining over time. This downward trend in survey responses is “smooth” and does not show any discontinuity around the date of Blake’s shooting, thus strengthening the validity of our UESD design. What is relevant is that the composition of the before and after groups is generally balanced across covariates, something that we have shown in Table 2 . The modeling approach that we describe below is designed to deal with these features of the data and their related sample size concerns.
https://doi.org/10.1371/journal.pone.0308487.t002
Quasi-experimental design
We propose a UESD design that allows us to compare responses from HCS survey participants interviewed four weeks before and after the police violence events occurred. We construct a control group by pooling survey responses submitted in the 4-week period preceding the shooting. This larger control group is compared against four treatment groups, each corresponding to a one-week period in the four weeks following the shooting (i.e. August 24 to August 31 is the first treatment group). The analytical sample includes 584 Black respondents and 939 white respondents. S2 Table in S1 File shows the sample size for the treatment and control groups in the different subgroup analyses that we run.
The UESD design exploits survey response timing, which makes it conceptually similar to the regression discontinuity and interrupted time series designs. Two assumptions allowing identification of causal effects using UESD are excludability, or that the timing of the interview only affects the outcome through the treatment; and ignorability, or that the timing of each interview is independent from the potential outcomes [ 43 ].
In order to assess whether the ignorability assumption is satisfied, we conduct a covariate balance test and show that the set of survey respondents interviewed four weeks before the shooting is statistically equivalent to the set of survey respondents interviewed four weeks afterward, resembling the balance that one would expect if exposure to police violence had been randomly assigned. The empirical argument that informs our UESD design is that the timing of Jacob Blake’s shooting is exogenous with respect to the timing of when the HCS surveys were completed. This assumption could be violated if survey participants were contacted in a manner that prioritized respondents living in certain community areas over others. Having a larger share of Black respondents in the group interviewed after the shooting could confound any observed differences in trust after the shooting given that Black residents are generally less likely to trust law enforcement. Similarly, our ability to identify the causal effect of police violence on trust would be compromised if the occurrence of the shooting was what encouraged some survey participants to respond to the survey. Because the survey was self-administered, participants had the freedom to respond to the survey within a specified time frame. The selected survey participants received an invitation letter containing instructions to access the web survey and personalized login credentials. A week later, a reminder postcard was mailed to all addresses encouraging participation online. Two weeks later, non-respondents received a full paper questionnaire packet.
The balance assessment in Table 2 shows proportions for the group of respondents from four weeks before and after Blake’s shooting (first and second columns). To evaluate any imbalances, we perform t-tests for the differences in proportions across the two groups, reporting the size of the difference (third column) and the p-value from the t-test for the difference in proportions (fourth column). For most attributes, the balance assessment test reveals no systematic differences across the pool of respondents—differences in proportions are generally small and statistically non-significant at the 5% level. However, the pool interviewed after the shooting skews Black and has fewer college-educated respondents. It also includes a larger share of respondents with prior contact with the criminal justice system. Looking at patterns of survey response across community areas, we don’t observe any systematic differences in the order in which surveys from different areas were submitted. However, if community areas had been surveyed in sequential order, we could have ended with some demographic groups being more represented in the before or after groups, potentially skewing the results and introducing bias into our analysis. This could be particularly significant if some community areas have residents that are wealthier or have a higher proportion of a given racial group, which we know is the case in Chicago and most American cities.
So the differences observed in Table 2 appear to result from Black, lower-SES respondents feeling more compelled to complete the survey after the shooting. We mitigate concerns about possible compositional changes by running and reporting models with and without controls. Similarly, because we estimate models by race and criminal justice contact status (and the combination of the two), the slight imbalance seen in Table 2 becomes less problematic. Although we cannot be certain that our treatment and control groups do not differ across unobserved attributes, seeing no differences in the comprehensive list of covariates shown in Table 2 give us confidence that the ignorability assumption is not violated.
With respect to the excludability assumption, S1 Fig in S1 File shows that the frequency of HCS survey completion was not significantly altered by Jacob Blake’s shooting. A large discontinuity in responses to the survey following the shooting could suggest selection or sorting on the basis of when the survey was conducted. Fortunately, we observe no such discontinuity. Similarly, it would be cause for concern if the unexpected event appeared to affect responses to theoretically unrelated questions. Our falsification test found no evidence of this (see S6 Fig in S1 File ).
We report the main findings in Figs 3 and 4 and supplementary ones in S3 to S6 Figs in S1 File . As stated above, we lack sufficient power to run subgroup analyses by age, gender and criminal justice contact status among Hispanics, Asians, and respondents of other racial groups. Nonetheless, we report estimated impacts for all Hispanics in S3 Fig in S1 File . Each pair of point estimates in the coefficient plots shows the change in the probability of expressing trust for those surveyed one, two, three, and four weeks after Jacob Blake’s shooting, relative to the pool of survey respondents interviewed in the four weeks prior. Within each pair of estimates, we show results with and without the set of controls listed in Table 2 .
Data are from the 2020 HCS survey. The survey question used to create the measure of trust in law enforcement is “To what extent do you trust your law enforcement agency?” We code answers to this question as binary, taking on value 1 for those that responded “a great extent” or “somewhat” and 0 for the rest. Each pair of point estimates shows the change in the probability of expressing trust for those surveyed one, two, three, and four weeks after Jacob Blake’s shooting, relative to the pool of survey respondents interviewed in the four weeks prior. Within each pair of estimates, we show results with and without the set of controls listed in Table 2 . Confidence intervals are at the 95% level with standard errors robust to heteroskedasticity.
https://doi.org/10.1371/journal.pone.0308487.g003
https://doi.org/10.1371/journal.pone.0308487.g004
Our results provide varying degrees of support for our hypotheses. The clearest evidence is that in support of H1, H2 and H3: the shooting of Jacob Blake led to a decline in trust in law enforcement among the Black community driven by younger cohorts and by individuals who had previously been arrested or incarcerated. Notably, the baseline level of reported trust was lower among these subgroups of Black respondents—49 percent of all Black respondents in the control group said that they trusted police before Jacob Blake’s shooting, whereas only 32 percent of Black respondents aged 18–44 and 36 percent of Black respondents with prior arrests reported that they trusted police.
Focusing on H1, Figs 3a and 4a show results when all Black and white respondents are evaluated as a group. We find a 13 to 16 percentage point decline in trust in police among all Black respondents who completed surveys in the first and second weeks after Jacob Blake was shot. These declines are relative to the control group’s average of 49 percentage points, as shown in S2 Table in S1 File . For whites, we do not observe any substantial changes in police trust after Blake’s shooting. H1 is the only hypothesis that we have sufficient power to test among Hispanics. Results in S3 Fig in S1 File show no effect for this set of respondents.
To test H2, Figs 3b and 4b limit the analysis to respondents who reported previously being arrested, jailed, or imprisoned. We find a significant decline in reported trust in law enforcement among Black respondents: 27 and 42 percentage points in the first and second weeks, relative to a baseline of 36 percentage points. In S4 Fig in S1 File , we report smaller declines in trust in law enforcement among Black respondents who have never been arrested, jailed, or imprisoned. These patterns are consistent with H2. We don’t find any changes in trust among white respondents in this subgroup.
With respect to H3, Figs 3c and 4c show a substantial decline in trust in police among Black respondents aged 18–44. We observe declines ranging from 12 to 18 percent across the first three weeks (from a baseline of 32 percentage points). When we compare these estimates to those among Black respondents that are older than 44 (S4 Fig in S1 File ), we observe somewhat smaller declines in trust that lasted only for two weeks. While the coefficients in the two weeks following the shooting are not statistically different across the two age groups, the effect for the younger cohort increases substantially in the third week whereas the effect for the older cohort disappears. We interpret this as evidence in support of H3. As before, we find no effect among white respondents in these two age groups.
With respect to gender, we find suggestive evidence in support of H4 in Figs 3d and 4d . Black men are the group that experienced more substantial declines in trust in law enforcement in the week after Blake’s shooting: a 24-percentage-point drop from a baseline of 43 percentage points. This effect, however, is only significant at the 10 percent level. As shown in S4 Fig in S1 File , Black women exhibit smaller, statistically non-significant effects in the second week following the shooting.
Separating the Kyle Rittenhouse events
The results presented thus far indicate that Black respondents report lower trust in law enforcement in response to police violence events. One valid concern about our empirical approach is that the effect of Blake’s shooting could be influenced by the effect of the events in the days that followed. Blake was shot in Kenosha on August 23, 2020, and on the night of August 25, Kyle Rittenhouse shot three protesters in Kenosha, with both events receiving extensive news coverage at the national level. It is plausible to think that Black survey respondents lost trust in law enforcement not only because a cop shot another unarmed Black man, but because Kyle Rittenhouse (a white man) was able to attend a protest armed with an AR-15-style rifle, shoot three individuals, and return back to his hometown without being stopped or arrested by the police (he turned himself in on August 26). With a large enough sample, we could run an analysis where we model changes in trust in one-day intervals after the shooting, allowing us to observe daily changes in trust from August 23 to August 25. If the effect is driven by Blake’s shooting, we should see a decline in trust in the days when Kyle Rittenhouse had not yet arrived in Kenosha and shot anyone.
Seeing trust declines in the two days after Blake’s shooting would support our theory that police violence increases feelings of mistrust among the Black population. This is what we find in S5 Fig in S1 File . The coefficient plot shows changes in trust in two-day intervals during the six days that followed Blake’s shooting. The control group remains the set of survey participants who responded to the survey in the four weeks prior to Blake’s shooting. These models include the same set of controls that we use in models that correspond to Figs 3 and 4 . We estimate these models for the two groups for which we found the clearest effects in the models discussed above—all Black respondents and Black respondents with prior contact with the police or the criminal justice system. The coefficients that help us separate the Blake effect from the Rittenhouse effect are those estimating changes in the Aug 24–25 window. Since Rittenhouse began his shooting spree at 11:48pm on August 25, survey responses submitted on August 25 had not yet been influenced by the Rittenhouse events. We find that the trend seen in Fig 3 was set in the immediate aftermath of Blake’s shooting and before Rittenhouse shot anyone. On August 24–25, we see a 26-percentage-point decline in trust in law enforcement among all Black respondents and a 52-percentage-point decline in trust in law enforcement among Black respondents who reported prior contact with the criminal justice system. Both of these estimates are statistically significant at the 5% level. Seeing that levels of trust dropped within 48 hours of Blake’s shooting increases our confidence that the Rittenhouse events are not driving our core findings.
Alternative explanations and falsification test results
Blake’s shooting and the resultant protests unfolded during the most intense days of the COVID-19 pandemic. A change in the frequency and nature of police-civilian interactions in the local context could have influenced perceptions of trust. Evidence from Houston shows that reactive police activities (e.g., deployments of special units) significantly decreased during the pandemic, but proactive patrols significantly increased [ 50 ]. To assess the possibility that the decline in trust that our models identify is a result of Chicagoans’ perceptions of city-wide changes in the frequency of police activity, we look at discontinuities in arrest patterns in the weeks before and after Blake’s shooting. S2 Fig in S1 File shows the number of arrests in Chicago in the weeks before and after Jacob Blake’s killing, broken down by racial categories: Black arrests (Panel a), Hispanic arrests (Panel b), and white arrests (Panel c). The horizontal axis on each graph spans the weeks surrounding Jacob Blake’s shooting, with the event marked by a vertical red line at week 0. The vertical axis represents the number of arrests per week for each group. We don’t see any noticeable discontinuity in the number of each type of arrest in the weeks surrounding Jacob Blake’s shooting.
Additionally, it could be the case that local COVID-19 policies had some effect on perceptions of police (i.e., if police were enforcing stay-at-home orders). A search of Chicago COVID-19 policies and local news articles during the survey period provides little evidence suggesting that COVID-19 policies confound our analysis. The state of Illinois phased out stay-at-home orders in late May, before the 2020 HCS survey was fielded. Although an additional stay-at-home advisory was issued towards the end of the HCS survey period (effective November 16, 2020), this was less stringent than a stay-at-home order and also falls outside the temporal window for the analytical sample used in this study.
In S6 Fig in S1 File , we assess whether Jacob Blake’s shooting changed an outcome that should not have been affected by the shooting. This test is recommended to generate suggestive evidence to support the excludability assumption [ 43 ]. Unfortunately, the HCS data don’t include many questions related to behavioral outcomes that could be used for this test. Most of the questions ask respondents to report on health-related issues retroactively over long periods of time (e.g., having ever been diagnosed with hypertension), rendering them unsuitable for the falsification test. The only question that speaks to a behavioral outcome that could have changed over a short period is one on soda consumption habits. HCS participants were asked “During the past 30 days, how many regular soda or pop or other sweetened drinks like sweetened iced tea, sports drinks, fruit punch or other fruit-flavored drinks have you had per day?” We use this question to test whether the shooting changed the probability of consuming more than one soda drink per day. We find no evidence of any changes in soda consumption in the aftermath of the shooting.
While prior research has thoroughly probed the descriptive relationship between criminalization and perceptions of police, few prior studies credibly identify individual-level causal effects. We examine whether these effects differ by age, gender, racial identity, or prior exposure to criminalization. While some recent work has examined effect heterogeneity according to e.g. partisan identification and media consumption habits [ 27 ], our study builds on gaps in existing literature by testing effects among various demographic subgroups and gauging their durability over time.
We find substantial, yet short-lived declines in perceptions of law enforcement among Black Chicago residents following the shooting of Jacob Blake. These effects were particularly pronounced among younger cohorts and respondents who reported prior contact with the criminal legal system. Unlike prior work, we do not find particularly strong evidence of a stronger effect for Black men compared to Black women. We also find that the decline in trust that we report in our core findings began in the immediate aftermath of Blake’s shooting—before Rittenhouse arrived in Kenosha and shot three protesters.
We believe that three of our four hypotheses pass a ‘hard test’: we saw declines in trust in police among Black Chicagoans whose quasi-experimental counterparts already reported very low levels of trust before Jacob Blake’s shooting. These respondents had likely been exposed to the police murder of George Floyd a few months earlier, which was a similarly salient continuing national news story. If we think about the Black Chicagoans in our study as subjects of many prior, analogous “treatments,” the fact that we observed any effect at all is notable.
We acknowledge that some features of this police violence incident (the Kyle Rittenhouse events and potential confounding related to the the COVID-19 pandemic) may initially give readers reason to doubt the viability of our identification strategy. We endeavoured to address these concerns through a series of robustness tests which ultimately increased our confidence in the analysis and findings.
Another potential threat to our identification strategy is noncompliance, i.e., some survey respondents may not have been aware of the police violence event [ 43 ]. If this were the case, our estimates could be interpreted as “intent-to-treat” (ITT) rather than the average treatment effect (ATE). That being said, Blake’s shooting was highly salient both in Chicago and nationwide and was covered by all major newspapers, TV, and radio channels for several days.
One limitation of the UESD design (in general) is that unique events may limit the generalizability of findings. Unfortunately, police violence is a relatively frequent event in America: according to the nonprofit research organization Mapping Police Violence, at least 1,176 people were killed by police in 2022. Granted, most of these killings do not reach the same level of public awareness as e.g. George Floyd, but police violence is still a regular phenomenon, and media coverage of police violence has increased over time [ 27 ].
We are also unable to probe certain causal mechanisms driving observed effects. For example, Reny and Newman [ 27 ] tests self-reported media consumption and geographical proximity to George Floyd protests. It could be the case that our observed effects were driven in part by news outlets’ amplification of Jacob Blake’s shooting or viral social media posts [ 51 , 52 ]. Although it is true that news coverage of police violence has increased following the rise of the Black Lives Matter movement [ 53 ], we find implausible that our observed effects primarily result from selective media amplification of police violence incidents. Research on social media usage shows that individuals who do not intentionally use social media for news but encounter it incidentally on platforms like Facebook, YouTube, and Twitter tend to engage with a wider array of online news sources compared to those who do not use social media at all [ 54 ]. This incidental exposure effect is notably stronger among younger users and those with a low interest in the news. This suggests that, independently of how traditional news outlets covered Jacob Blake’s shooting, the occurrence of such an event likely reached a broad segment of the population.
Furthermore, past research suggests that individuals who have previously been arrested (one of the groups for which we observe the strongest effects) are less likely to have their views influenced by crime news coverage [ 55 ]. Additionally, even if fewer news stories about the shooting were published, it seems possible that viral social media posts would have ensured that the survey respondents were informed about the shooting. Further research is required to better formulate a complete account of legal cynicism via police violence. Our design also cannot differentiate between the effect of police violence itself and the effect of ensuing protests. Yet there would be no police brutality protests without police brutality.
Finally, survey responses may be an imperfect proxy for actual beliefs and behaviors, and particularly with respect to law enforcement (as highlighted by [ 7 ]). This is particularly true when measuring interactions with the police, as surveys can be less reliable in capturing genuine experiences and sentiments [ 56 ]. While some might express a reluctance to cooperate with the police in surveys, their actions might indicate otherwise. This divergence between stated attitudes and actual behaviors underscores the potential pitfalls of relying on survey data alone. Yet for the specific question examined in this paper—whether police violence affects trust in police (rather than e.g. willingness to report a crime)—these potential shortcomings are likely less salient.
Moving away from the limitations of our study, the fact that we observed substantial effects only among Black respondents is somewhat puzzling because recent, similar studies have found different results. In the wake of George Floyd’s murder, Reny and Newman found that changes in white Americans’ attitudes towards police were larger than changes among Black Americans [ 27 ]. Anoll and coauthors found that white survey respondents’ attitudes towards law enforcement were much more strongly associated with recent criminal legal contact compared to Black men [ 33 ]. Yet we observed a much stronger effect for Black respondents–even when we limited the sample to only those who reported prior police contact. Speculating beyond the survey data, it could be the case that white supremacist organizing in defense of Kyle Rittenhouse following Jacob Blake’s shooting by police influenced the average effect we observed for white respondents. The chronology of police violence events in 2020 may also be explanatory–perhaps changes in white attitudes towards police had already reached a ‘ceiling’ following George Floyd protests in the spring and summer.
The idea that our target population was “pre-treated” via exposure to previous police violence incidents (e.g. George Floyd) may also help to explain why most of the effects we observe here appear to be short-lived. To the extent that acquiring new information about a police violence incident constitutes ‘learning’ about one’s own relationship to police or government more broadly, Black residents have ‘less to learn’ due to their disproportionate historical exposure to policing and incarceration (at the personal, proximal, and community levels). Some recent work has found similar temporal bounds for related treatment effects, i.e., Black residents who were ticketed closer to the date of an election were less likely to vote compared to voters who were ticketed further away from the election date [ 31 ]. It could have been the case that Black respondents experienced a powerful short-term reaction (i.e., “anticipatory stress of police brutality,” per Alang and coauthors [ 57 ]) when hearing about a new police violence incident, and the salience faded as the incident was incorporated into pre-existing knowledge and narratives of group-level exposure to criminalization.
We also note with interest that we observed more pronounced negative effects among Black men than Black women, which may be seen as contrary to the predictions of prior scholarship. It may be the case that Black men responded more strongly to Jacob Blake’s shooting since the specific context of the incident corresponded more directly to the types of police violence or misconduct most commonly experienced by men rather than women; the vast majority of American police shooting victims are men.
To the extent that legal cynicism might mediate police violence and e.g. political participation or system avoidance, the direction of any subsequent causal effect is not a given. For example, while personal exposure to criminalization tends to decrease the likelihood that an individual will vote [ 30 , 31 ], community-level indirect exposure can increase voter turnout [ 32 ]. Although Brantingham and coauthors found stable crime reporting trends in the wake of George Floyd protests [ 58 ], this may reflect an awareness that police were the only available option that residents could turn to in response to e.g. interpersonal violence. Further research is required to determine how legal cynicism affects participation in civic life across a variety of contexts and for different groups of Americans. These effects could also plausibly shift over time, as Americans are exposed to new “injustice narratives” and nascent protest movements.
Taken together, our findings enhance our understanding of the ways in which racial identity and the lived experience of criminalization affect perceptions of police. In addition to prior literature suggesting that declining trust in police could lead to e.g. lower rates of crime reporting, our study suggests that police violence drives legal cynicism among Black youth, plausibly shaping a much wider range of activities of interest.
Supporting information
S1 file. appendix containing additional figures and tables..
https://doi.org/10.1371/journal.pone.0308487.s001
- View Article
- Google Scholar
- PubMed/NCBI
- 6. Sampson RJ. Neighborhood Inequality, Violence, and the Social Infrastructure of the American City. In: Tate WF IV, editor. Research on Schools, Neighborhoods, and Communities: Toward Civic Responsibility. Published for the American Educational Research Association by Rowman and Littlefield, Inc.; 2012. p. 11–28.
- 11. Fagan J, Tyler T, Meares TL. Street Stops and Police Legitimacy in New York. In Jacqueline E. Ross and Thierry Delpeuch (eds.), Comparing the Democratic Governance of Police Intelligence: New Models of Participation and Expertise in the United States and Europe 203 (2016). URL: https://papers.ssrn.com/abstract=2795175 .
- 12. Ang D, Bencsik P, Bruhn J, Derenoncourt E. Police Violence Reduces Civilian Cooperation and Engagement with Law Enforcement. Working Paper. 2021.
- 17. Rios VM. Punished: Policing the Lives of Black and Latino Boys. NYU Press; 2011. Available from: https://nyupress.org/9780814776384/punished .
- 20. Tyler TR, Huo YJ. Trust in the Law: Encouraging Public Cooperation with the Police and Courts Through. Russell Sage Foundation; 2002. Available from: https://www.jstor.org/stable/10.7758/9781610445429 .
- 21. Papachristos AV, Meares TL, Fagan J. Why Do Criminals Obey the Law? The Influence of Legitimacy and Social Networks on Active Gun Offenders. 2009. https://papers.ssrn.com/abstract=1326631 .
- 29. Lerman AE, Weaver VM. Arresting citizenship: the democratic consequences of American crime control. Chicago studies in American politics. Chicago; London: The University of Chicago Press; 2014.
- 35. Leighley JE, Nagler J. Who votes now?: Demographics, issues, inequality, and turnout in the United States. Princeton University Press; 2013.
- 37. Walker HL. Mobilized by injustice: criminal justice contact, political participation, and race. NewYork: Oxford University Press 2020; 2020.
- 39. Fagan JA, Geller A, Davies G, West V. Street Stops and Broken Windows Revisited: The Demography and Logic of Proactive Policing in a Safe and Changing City. In: Rice SK, White MD, editors. Race, Ethnicity, and Policing. New York University Press; 2010. p. 309–348.
- 45. RTI International. 2020 Healthy Chicago Survey (HCS): Methodology Report; 2020. https://www.chicago.gov/content/dam/city/depts/cdph/statistics_and_reports/2020_HCS_Methodology_Report.pdf .
Have a language expert improve your writing
Run a free plagiarism check in 10 minutes, generate accurate citations for free.
- Knowledge Base
Methodology
- Quasi-Experimental Design | Definition, Types & Examples
Quasi-Experimental Design | Definition, Types & Examples
Published on July 31, 2020 by Lauren Thomas . Revised on January 22, 2024.
Like a true experiment , a quasi-experimental design aims to establish a cause-and-effect relationship between an independent and dependent variable .
However, unlike a true experiment, a quasi-experiment does not rely on random assignment . Instead, subjects are assigned to groups based on non-random criteria.
Quasi-experimental design is a useful tool in situations where true experiments cannot be used for ethical or practical reasons.
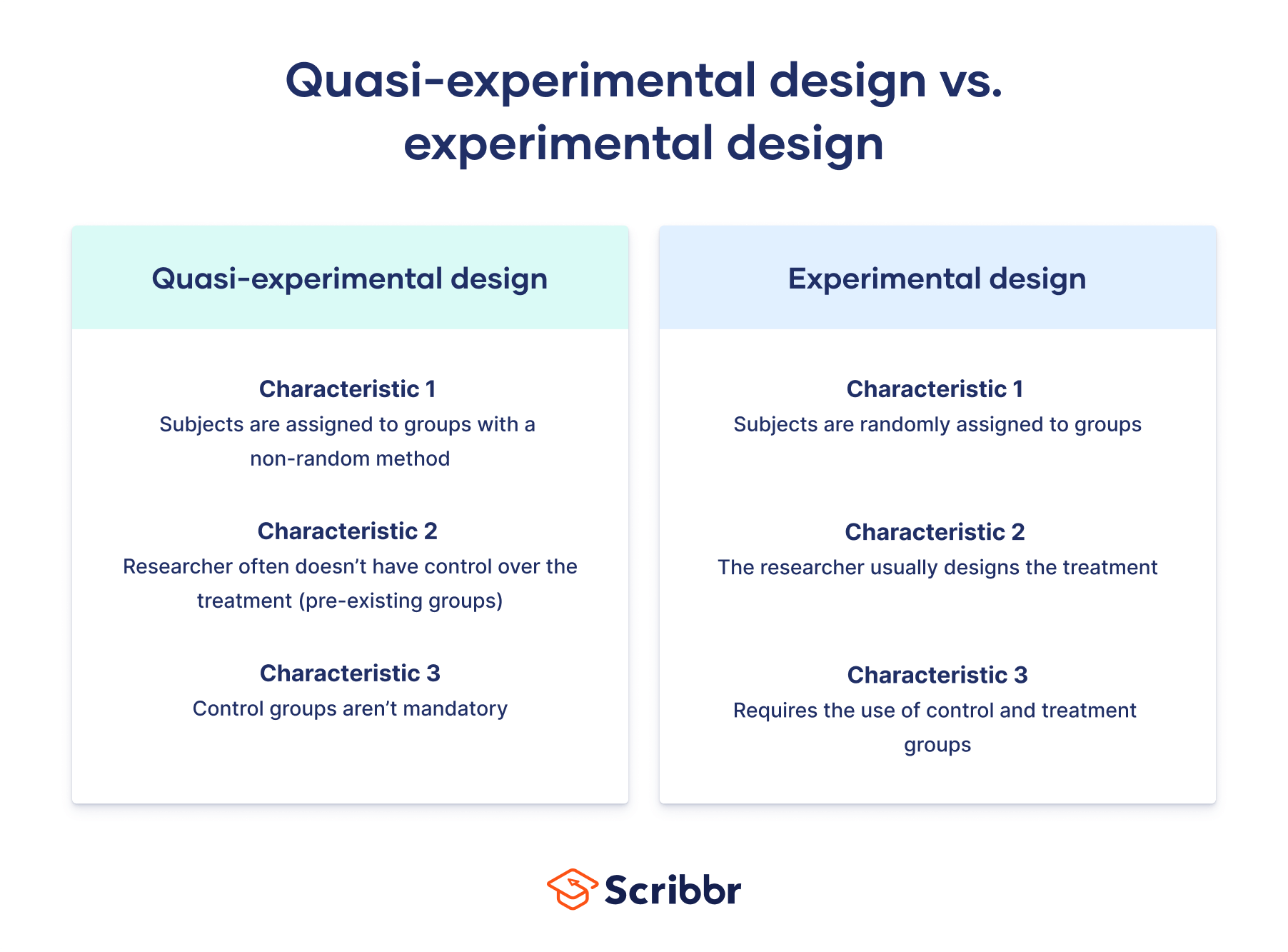
Table of contents
Differences between quasi-experiments and true experiments, types of quasi-experimental designs, when to use quasi-experimental design, advantages and disadvantages, other interesting articles, frequently asked questions about quasi-experimental designs.
There are several common differences between true and quasi-experimental designs.
True experimental design | Quasi-experimental design | |
---|---|---|
Assignment to treatment | The researcher subjects to control and treatment groups. | Some other, method is used to assign subjects to groups. |
Control over treatment | The researcher usually . | The researcher often , but instead studies pre-existing groups that received different treatments after the fact. |
Use of | Requires the use of . | Control groups are not required (although they are commonly used). |
Example of a true experiment vs a quasi-experiment
However, for ethical reasons, the directors of the mental health clinic may not give you permission to randomly assign their patients to treatments. In this case, you cannot run a true experiment.
Instead, you can use a quasi-experimental design.
You can use these pre-existing groups to study the symptom progression of the patients treated with the new therapy versus those receiving the standard course of treatment.
Receive feedback on language, structure, and formatting
Professional editors proofread and edit your paper by focusing on:
- Academic style
- Vague sentences
- Style consistency
See an example
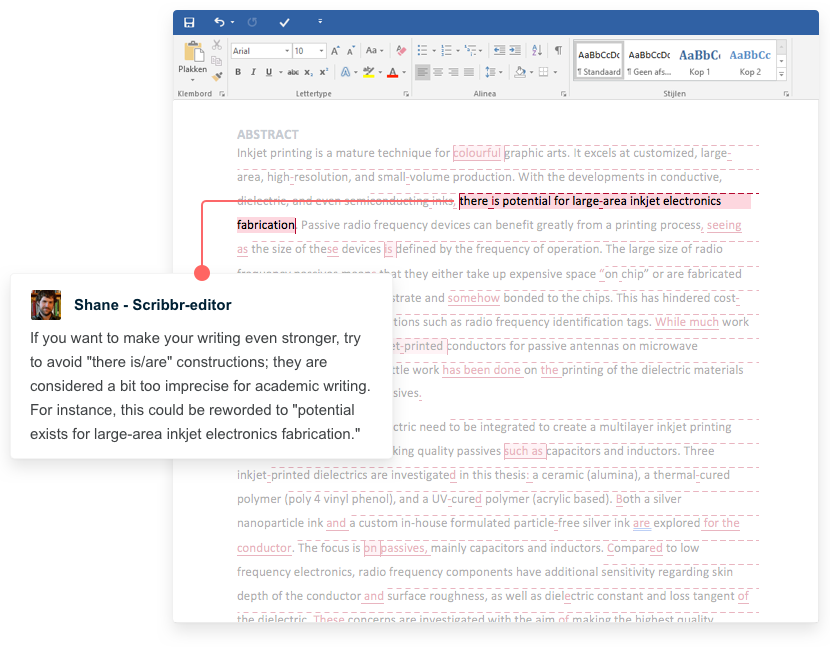
Many types of quasi-experimental designs exist. Here we explain three of the most common types: nonequivalent groups design, regression discontinuity, and natural experiments.
Nonequivalent groups design
In nonequivalent group design, the researcher chooses existing groups that appear similar, but where only one of the groups experiences the treatment.
In a true experiment with random assignment , the control and treatment groups are considered equivalent in every way other than the treatment. But in a quasi-experiment where the groups are not random, they may differ in other ways—they are nonequivalent groups .
When using this kind of design, researchers try to account for any confounding variables by controlling for them in their analysis or by choosing groups that are as similar as possible.
This is the most common type of quasi-experimental design.
Regression discontinuity
Many potential treatments that researchers wish to study are designed around an essentially arbitrary cutoff, where those above the threshold receive the treatment and those below it do not.
Near this threshold, the differences between the two groups are often so minimal as to be nearly nonexistent. Therefore, researchers can use individuals just below the threshold as a control group and those just above as a treatment group.
However, since the exact cutoff score is arbitrary, the students near the threshold—those who just barely pass the exam and those who fail by a very small margin—tend to be very similar, with the small differences in their scores mostly due to random chance. You can therefore conclude that any outcome differences must come from the school they attended.
Natural experiments
In both laboratory and field experiments, researchers normally control which group the subjects are assigned to. In a natural experiment, an external event or situation (“nature”) results in the random or random-like assignment of subjects to the treatment group.
Even though some use random assignments, natural experiments are not considered to be true experiments because they are observational in nature.
Although the researchers have no control over the independent variable , they can exploit this event after the fact to study the effect of the treatment.
However, as they could not afford to cover everyone who they deemed eligible for the program, they instead allocated spots in the program based on a random lottery.
Although true experiments have higher internal validity , you might choose to use a quasi-experimental design for ethical or practical reasons.
Sometimes it would be unethical to provide or withhold a treatment on a random basis, so a true experiment is not feasible. In this case, a quasi-experiment can allow you to study the same causal relationship without the ethical issues.
The Oregon Health Study is a good example. It would be unethical to randomly provide some people with health insurance but purposely prevent others from receiving it solely for the purposes of research.
However, since the Oregon government faced financial constraints and decided to provide health insurance via lottery, studying this event after the fact is a much more ethical approach to studying the same problem.
True experimental design may be infeasible to implement or simply too expensive, particularly for researchers without access to large funding streams.
At other times, too much work is involved in recruiting and properly designing an experimental intervention for an adequate number of subjects to justify a true experiment.
In either case, quasi-experimental designs allow you to study the question by taking advantage of data that has previously been paid for or collected by others (often the government).
Quasi-experimental designs have various pros and cons compared to other types of studies.
- Higher external validity than most true experiments, because they often involve real-world interventions instead of artificial laboratory settings.
- Higher internal validity than other non-experimental types of research, because they allow you to better control for confounding variables than other types of studies do.
- Lower internal validity than true experiments—without randomization, it can be difficult to verify that all confounding variables have been accounted for.
- The use of retrospective data that has already been collected for other purposes can be inaccurate, incomplete or difficult to access.
Here's why students love Scribbr's proofreading services
Discover proofreading & editing
If you want to know more about statistics , methodology , or research bias , make sure to check out some of our other articles with explanations and examples.
- Normal distribution
- Degrees of freedom
- Null hypothesis
- Discourse analysis
- Control groups
- Mixed methods research
- Non-probability sampling
- Quantitative research
- Ecological validity
Research bias
- Rosenthal effect
- Implicit bias
- Cognitive bias
- Selection bias
- Negativity bias
- Status quo bias
A quasi-experiment is a type of research design that attempts to establish a cause-and-effect relationship. The main difference with a true experiment is that the groups are not randomly assigned.
In experimental research, random assignment is a way of placing participants from your sample into different groups using randomization. With this method, every member of the sample has a known or equal chance of being placed in a control group or an experimental group.
Quasi-experimental design is most useful in situations where it would be unethical or impractical to run a true experiment .
Quasi-experiments have lower internal validity than true experiments, but they often have higher external validity as they can use real-world interventions instead of artificial laboratory settings.
Cite this Scribbr article
If you want to cite this source, you can copy and paste the citation or click the “Cite this Scribbr article” button to automatically add the citation to our free Citation Generator.
Thomas, L. (2024, January 22). Quasi-Experimental Design | Definition, Types & Examples. Scribbr. Retrieved September 9, 2024, from https://www.scribbr.com/methodology/quasi-experimental-design/
Is this article helpful?
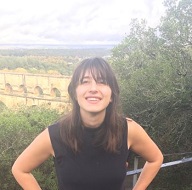
Lauren Thomas
Other students also liked, guide to experimental design | overview, steps, & examples, random assignment in experiments | introduction & examples, control variables | what are they & why do they matter, "i thought ai proofreading was useless but..".
I've been using Scribbr for years now and I know it's a service that won't disappoint. It does a good job spotting mistakes”
- Open access
- Published: 13 September 2024
Application of AI-empowered scenario-based simulation teaching mode in cardiovascular disease education
- Koulong Zheng 1 , 2 na1 ,
- Zhiyu Shen 1 na1 ,
- Zanhao Chen 1 na1 ,
- Chang Che 1 na1 &
- Huixia Zhu 1
BMC Medical Education volume 24 , Article number: 1003 ( 2024 ) Cite this article
Metrics details
Cardiovascular diseases present a significant challenge in clinical practice due to their sudden onset and rapid progression. The management of these conditions necessitates cardiologists to possess strong clinical reasoning and individual competencies. The internship phase is crucial for medical students to transition from theory to practical application, with an emphasis on developing clinical thinking and skills. Despite the critical need for education on cardiovascular diseases, there is a noticeable gap in research regarding the utilization of artificial intelligence in clinical simulation teaching.
This study aims to evaluate the effect and influence of AI-empowered scenario-based simulation teaching mode in the teaching of cardiovascular diseases.
The study utilized a quasi-experimental research design and mixed-methods. The control group comprised 32 students using traditional teaching mode, while the experimental group included 34 students who were instructed on cardiovascular diseases using the AI-empowered scenario-based simulation teaching mode. Data collection included post-class tests, “Mini-CEX” assessments, Clinical critical thinking scale from both groups, and satisfaction surveys from experimental group. Qualitative data were gathered through semi-structured interviews.
Research shows that compared with traditional teaching models, AI-empowered scenario-based simulation teaching mode significantly improve students’ performance in many aspects. The theoretical knowledge scores( P < 0.001), clinical operation skills( P = 0.0416) and clinical critical thinking abilities of students( P < 0.001) in the experimental group were significantly improved. The satisfaction survey showed that students in the experimental group were more satisfied with the teaching scene( P = 0.008), Individual participation( P = 0.006) and teaching content( P = 0.009). There is no significant difference in course discussion, group cooperation and teaching style of teachers( P > 0.05). Additionally, the qualitative data from the interviews highlighted three themes: (1) Positive new learning experience, (2) Improved clinical critical thinking skills, and (3) Valuable suggestions and concerns for further improvement.
The AI-empowered scenario simulation teaching Mode plays an important role in the improvement of clinical thinking and skills of medical undergraduates. This study believes that the AI-empowered scenario simulation teaching mode is an effective and feasible teaching model, which is worthy of promotion in other courses.
Peer Review reports
Introduction
Cardiovascular diseases, including myocardial infarction and arrhythmia, frequently manifest abruptly and progress rapidly, placing individuals in critical situations. In addition to the physical distress, the substantial rates of disability and mortality linked to these conditions impose a significant burden on both families and society. Furthermore, the presence of commodities such as diabetes and chronic obstructive pulmonary disease in many cardiovascular disease patients adds further complexity to treatment strategies [ 1 , 2 ]. In light of this context, the importance of internship training in cardiology is underscored [ 3 ]. In China, when medical students enter their fourth and fifth years of undergraduate study, they will be placed in hospitals for a clinical internship lasting one to two years. Internship plays a critical role in the development of medical students, facilitating the transition from theoretical knowledge to practical application and fostering the growth of clinical reasoning and skills [ 4 ]. Nevertheless, the prevailing mode of internship education primarily relies on conventional instructional approaches, which prioritize teacher-led dissemination of knowledge through lectures and demonstrations [ 5 , 6 ]. Although these methods are successful in facilitating knowledge acquisition, they are inadequate in motivating students, promoting clinical reasoning, and cultivating the skills necessary to manage emergency situations, particularly when dealing with critically ill patients. As a result, it is essential to implement a shift in teaching methodologies, specifically within the realm of cardiology internship training.
In recent years, the rapid development of Artificial Intelligence (AI) technology has led to the emergence of various products profoundly impacting various aspects of people’s lives [ 7 ]. Generative AI, a type of AI based on deep learning, involves training large-scale language models to generate new text, images, or other types of data. Notably, models like OpenAI’s ChatGPT use deep learning algorithms trained on extensive datasets to generate human-like responses in conversation. In the realm of education, generative AI exhibits tremendous potential. Firstly, it can offer personalized learning experiences by tailoring learning paths based on individual student needs and proficiency levels, enhancing learning effectiveness and making education more targeted and efficient [ 8 , 9 ]. Secondly, generative AI plays a crucial role in automatic assessment and feedback, providing students with immediate and constructive feedback, promoting better understanding and mastery of knowledge. Additionally, through simulated dialogues, role-playing, and other mode, generative AI can help students improve communication and problem-solving skills, offering new possibilities for flexible, intelligent teaching mode and driving innovation and progress in education [ 10 ].
Scenario-based simulation teaching is an instructional method that involves simulating real-world situations for teaching purposes, commonly used in clinical education. In this approach, students are placed in virtual or real scenarios where they face specific problems, challenges, or tasks, engaging in practical activities and decision-making to proficiently apply knowledge [ 11 ]. This teaching method emphasizes practicality and interactivity, allowing students not only to apply theoretical knowledge in simulated situations but also to actively participate in discussions, collaborate on problem-solving, and enhance their practical application and teamwork skills [ 12 ]. Research indicates that scenario-based simulation teaching stimulates student interest, increases motivation, and fosters critical thinking and innovation by integrating theoretical knowledge into practice [ 13 ].
Nowadays, with the rapid development of science, new technologies such as Virtual Reality and Augmented Reality have brought significant changes to clinical medicine. For example, clinical scenario simulation surgery allows doctors to create a virtual surgical training platform. This allows them to practice complex surgical skills in a safe, repeatable practice environment [ 14 , 15 , 16 , 17 ]. While studies have demonstrated the effectiveness of scenario-based simulation teaching in clinical courses [ 11 , 12 , 13 , 18 ], there is currently no research on the application of generative AI in simulating clinical scenarios related to cardiovascular diseases. In this study, we aim to investigate the effectiveness of the AI-empowered scenario-based simulation teaching mode in cardiovascular disease education. Our goal is to explore the impact of this innovative teaching model on clinical interns, focusing on their basic knowledge, clinical operation ability and clinical critical thinking ability.
Experimental design
A combination of quasi-experimental research design and descriptive qualitative research methods was employed to form both a control group and an experimental group. Our study integrated Kolb’s experiential learning model into the experimental group’s teaching methods to enhance the learning process [ 19 , 20 ]. Kolb’s experiential learning model involves providing learners with real or simulated situations and activities. Under the guidance of teachers, learners participate in these activities to gain personal experience. They then reflect on and summarize their observations, developing theories or conclusions, which are ultimately applied in practice (Fig. 1 ).
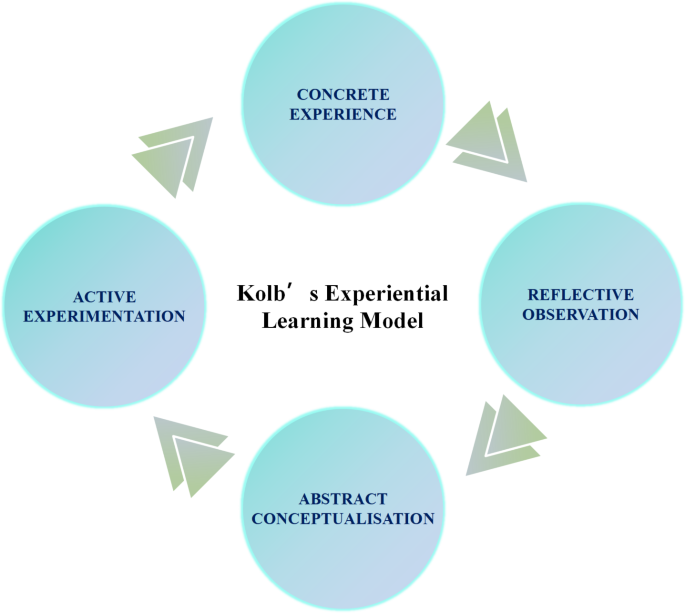
Kolb’s experiential learning model
Study participants
A total of 66 first-year students from two classes in the clinical major at Nantong University were selected as the study participants. Inclusion criteria comprised: (1) absence of current physical or mental abnormalities; (2) full-time undergraduate students in medical majors; (3) no prior experience using the AI platform for medical course learning before the experiment; (4) voluntary participation in the study with the signing of an informed consent form. The control group consisted of 32 students, following a traditional teaching model, while the experimental group comprised 34 students undergoing scenario-based simulation teaching mode empowered by AI.
All students entered university directly through the national college entrance examination (gaokao) after completing 12 years of education. After inclusion, an assessment of the characteristics of the two student groups, including age, gender in pre-professional courses, revealed comparable learning abilities between the two groups ( P > 0.05). Both groups received instruction in internal medicine. The students in both groups used the ninth edition of the textbook “Internal Medicine,” edited by Ge Junbo and others and published by People’s Medical Publishing House, and were taught by the same instructor.
Teaching interventions
Teaching mode of control group.
The control group adopted the traditional teaching model, and the course arrangement was divided into two parts: theoretical classes and practical classes. In weekly theoretical classes, teachers use PPT to impart knowledge according to the teaching objectives and syllabus. The contents of these theoretical courses include basic knowledge of cardiovascular diseases, pathophysiology, diagnostic methods and treatment principles. Teachers help students understand complex medical concepts through detailed explanations and illustrations, and answer students’ questions in class to ensure they master the necessary theoretical knowledge.
In the practical class, the teacher led the students to conduct practical training based on the teaching content of the previous theoretical class. Practical classes were usually conducted in simulated wards or clinical skills laboratories. Teachers first demonstrated the operations on a standardized patient(SP), including specific operating steps such as cardiac examination, auscultation, and electrocardiogram interpretation. Teachers explained in detail the key points and precautions of each operation link, and demonstrated on-site how to communicate with patients to improve students’ clinical operation skills and doctor-patient communication abilities.
After the demonstration, students were divided into groups for operational exercises, with teachers guiding them, correcting mistakes in a timely manner and providing feedback. In this way, students not only consolidated theoretical knowledge, but also enhanced practical operational abilities and developed clinical thinking and decision-making abilities. In addition, practical courses also emphasized teamwork and communication skills. Students simulated real clinical environments through group discussions and role-playing to improve their overall quality and professional abilities.
Formation of teaching research team
The team of this study was composed of 2 chief physicians, 3 attending physicians, 2 resident physicians, 5 teaching assistants, and 4 graduate students. This team consisted of teachers with more than five years of teaching experience. Before the lectures, they all underwent training in scenario simulation teaching mode and were proficient in using ChatGPT.
Implementation plan for educational reform
The teaching model of the experimental group innovatively incorporated generative artificial intelligence technology, providing students with a brand new scene simulation teaching experience. In this teaching model, teachers first provided an in-depth explanation of theoretical knowledge to ensure that students could master the core points of the course, such as the characteristics of different types of arrhythmias in electrocardiograms. These points are the basis for understanding the complexity of cardiovascular disease and are the knowledge that students must skillfully apply in subsequent simulation practices.
Students then watched a video simulating scenarios related to cardiovascular disease. These videos not only vividly reproduced clinical scenes, but also contained rich medical information and situational challenges, which greatly stimulated students’ interest in learning and enthusiasm for participation. While watching the video, students were encouraged to play the role of doctors and use the theoretical knowledge they had learned to conduct detailed analysis and inferences on the signs, symptoms, and pathogenesis shown in the video.
Students needed to use critical thinking to identify the occurrence and development of the disease from the patient’s clinical manifestations and, at the same time, master the key points of diagnosis and the basic principles of treatment. This process not only exercises the students’ clinical thinking skills but also deepens their understanding of the disease diagnosis and treatment process.
After the scenario simulation, students participated in group discussions to share their observations and analyses, complementing each other and improving their understanding of the disease. This interactive learning method promoted the exchange of knowledge and the collision of ideas, helped students examine problems from different angles, and improved their problem-solving abilities.
Finally, students would complete thinking questions related to the course content, consolidate the knowledge they have learned, and test the learning effect. Students could ask ChatGPT questions at any time, and when they had more questions, they could get help from their teachers. Except for learning theoretical knowledge, all clinical practice processes were consistent with those of the control group.
Establishment of experimental group
Reasonable grouping is an important prerequisite for team learning. To enhance group learning and achieve optimal learning outcomes, each group had a maximum of 6 students. Therefore, before class, teachers determined the groups based on students’ average GPAs to ensure that each group had similar overall learning abilities. Eventually, the students in the experimental group were divided into 6 groups. Based on feedback from teachers on student performance, adjustments to group members were made in the first week. In each group, one student was selected as the group leader, responsible for organizing group activities. Clear division of team roles ensured the participation of each member and promoted cooperation within the group.
Preparation of scenario simulation videos
Writing scenario simulation scripts.
The cardiovascular teaching research group wrote script stories based on teaching objectives and typical cardiovascular cases, enriching the background and character features of the plot to make it as close to real clinical situations as possible.
Breaking down script scenes
In the production of clinical case scenario simulation videos, the breakdown script played a crucial role, providing guidance and basis for AI drawing for each scene. By inputting the case directly into ChatGPT and instructing, “How many scenes can this script be broken down into for animation video creation?” ChatGPT would then offer a breakdown of scenes as an example, subject to review by the teachers for alignment with educational goals and accuracy.
Animation drawing
By inputting the prompt “I need you to act as the Midjourney command optimization master, generating scene descriptions for the above scenes separately, I want Midjourney to draw them, please provide concise descriptions in both Chinese and English,” specific instructions would be obtained. This prompt asks ChatGPT to generate a concise description for each scenario. These descriptions should include necessary details to help Midjourney draw the scene accurately. Each scene description was reviewed, and then each English description was input into Midjourney to generate animation materials. These materials were imported into editing software to complete the production of video content, with subtitles automatically generated and added to the video.
Question bank compilation
In the process of compiling a question bank for cardiovascular teaching, ChatGPT generates questions based on the plot content of the script when prompted with the instruction, “This is a case in cardiovascular teaching, what questions can be given to students?” ChatGPT would write questions based on the relevant plot content of the script. The teacher could continue to instruct to change the format and description of the questions and could also request answers and scoring criteria for the corresponding questions.
Synthesis of scenario simulation teaching videos and classroom teaching
The assessment of question and answer accuracy and scientific validity, the adjustment of question difficulty in alignment with teaching objectives, and the precise placement of questions within the video were carried out to finalize the production of cardiovascular scenario simulation teaching videos. Subsequently, these videos were integrated into the class app for classroom instruction. Feedback from both students and teachers was solicited to enhance the content and quality of the scenario simulation teaching videos(Fig. 2 ).
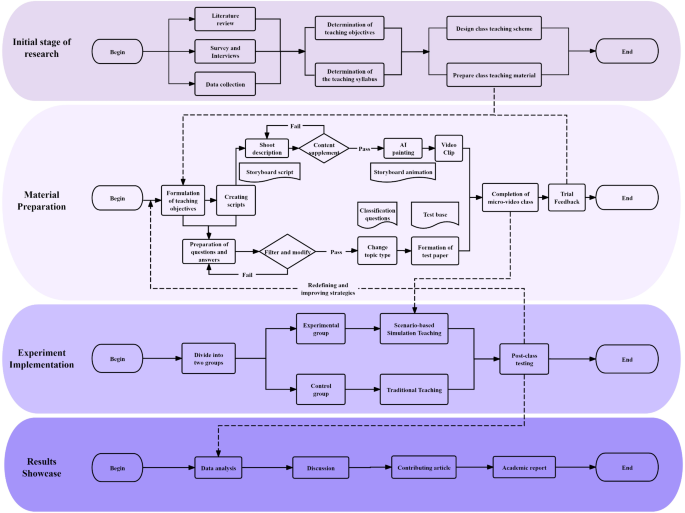
Flow chart of research on teaching reform programmes
Data collection
Post-class test.
Students in both the experimental group and the control group took the post-class test, and the test content and grading criteria were exactly the same. The theoretical knowledge level and practical operational ability were each scored out of 100 points, with higher scores indicating more vital student abilities. The theoretical knowledge assessment used exam questions prepared by the teaching team, while practical operational ability used a “Mini-CEX” scoring sheet customized for cardiovascular medicine. The Mini-CEX evaluation form was adapted by the teaching and research team from a scale for assessing clinical skills written by John J Norcini et al. [ 21 ]. It is designed according to the characteristics of cardiovascular medicine. It mainly evaluates clinical history recording, electrocardiogram interpretation, humanistic care, Clinical diagnosis, communication skills and overall competency. There were five parts in total; each part had four questions, and each question adopted Likert’s five-point scoring system. The Cronbach’s alpha of the scale was 0.90, and the Cronbach’s alpha of each dimension was 0.753–0.772.
Clinical critical thinking scale
Based on Robert Ennis’s critical thinking framework and related theories, relevant questions were adapted according to the experimental purpose and subjects [ 22 ]. The final clinical critical thinking scale consisted of four dimensions, including logical reasoning, central argument, argumentation evidence and organizational structure, with a total of 5 questions in each dimension and 5 points in each question, for a total of 100 points.
Overall teaching satisfaction survey
The teaching and research team developed a teaching satisfaction questionnaire. Students completed the Teaching Satisfaction questionnaire on the WJX.cn at the end of the final exam. The questionnaire included six aspects: teaching scene satisfaction (Q1 ∼ Q4), course discussion satisfaction (Q5 ∼ Q8), group cooperation satisfaction (Q9 ∼ Q11), individual participation (Q12 ∼ Q14), teaching content satisfaction (Q15 ∼ Q18), and teaching teacher satisfaction (Q19 ∼ Q20). Each question was set on a scale of 1 to 5 (strongly disagree to strongly agree on five scales). Final satisfaction (%) is score/total score (100 points) *100%. After analyzing the preliminary collected data, Cronbach’s alpha coefficient was 0.85, indicating high internal consistency and reliability.
Qualitative assessment - semi-structured interviews
At the end of the course, we conducted a semi-structured interview to survey students in the experimental group and teachers on their evaluation of the use of AI in teaching cardiovascular disease. In selecting interviewees, we considered the gender and age and then conducted purposive sampling among the experimental group to ensure a diversity of opinions.
In order to fully understand the teaching effect and the real experience of teachers and students with the application of AI teaching mode, the research team first conducted preliminary interviews with two students and determined the final outline of the interview: (1) How do you feel about the learning of this teaching mode? (2) Do you think your learning/teaching style has changed since before? (3) What are your suggestions for the future development of this teaching mode?
A researcher who was well-versed in interviewing techniques was assigned to conduct the interviews independently. The interviews were conducted during the week following the course in a quiet and relaxing session to avoid errors as much as possible. Based on their final test results, they were divided into three grades, with three boys and three girls randomly selected from six groups from three different levels. Each interview lasted approximately 20 min. The students’ conversations were recorded using a voice recorder, and the research team pledged to keep them confidential. Recordings of the interviews were transcribed verbatim within 24 h of the end of the conversation.
Data analysis
Data entry and analysis were performed using Rstudio software (version 4.3.1). The following R packages were utilized: “stats”, “car”, “doBy”, and “ggplot2”.
For quantitative data, independent sample t-tests were employed to analyze differences between groups. For qualitative data, the chi-square test was utilized. A significance level of P < 0.05 was considered statistically significant, indicating differences between groups.
Baseline comparison between two groups
The experimental group consisted of 34 students aged 22–24 years (mean age 23.03 ± 0.626). The Control group comprised 32 students from clinical professional classes, with ages ranging from 21 to 25 years (mean age 23.14 ± 0.976). Before the class, we assessed the basic clinical knowledge of the two groups of students, and the results showed that there was no significant difference in the demographic characteristics of the two groups ( P > 0.05), and we found that there was no significant difference between them, which was comparable (Table 1 ).
Final scores between two groups
Statistical analysis of examination scores for two groups revealed that students in the experimental group had an average score of 83.26 on the theoretical final exam, whereas students in the control group had an average score of 79.56. The scores of the control group were significantly lower than those of the experimental group ( p < 0.05). Regarding Mini-CEX examination scores, students within the experimental group attained an average score of 76.24, which was notably greater than the average score of 70.19 achieved by students in the control group ( p < 0.001). Furthermore, the clinical critical thinking proficiency of the experimental group surpassed that of the control group, as indicated by statistical significance ( p < 0.001) (Table 2 ).
Satisfaction survey
After investigation and recovery, a total of 66 students completed the satisfaction questionnaire, and 66 valid questionnaires were recovered, with a total completion rate of 100%. As shown in the questionnaire results (Table 3 ), it can be seen that the overall satisfaction of experimental group in teaching scene, individual participation and teaching content is higher than that of control group, and the difference between the two groups is statistically significant ( P < 0.05). There were no significant differences in other aspects ( P > 0.05).
Qualitative data analysis
In summarizing the interview findings, three primary themes emerge for analysis: (1) A new learning (teaching) experience; (2) Enhancement of clinical critical thinking ability; (3) Suggestions for improvement.
Theme 1: a new learning (teaching) experience
“In the past, we have always learned knowledge from books. Some things are very complicated and not easy to understand. With the help of AI, I think a lot of complicated knowledge has suddenly become simple and clear.”(S1).
“It is a very unimaginable experience. Through the scenario simulation course, I can intuitively see the physiological changes of the heart and blood vessels, and many theoretical knowledge are easier to understand.”(S2).
“The scenario simulation course enables us to visually see the electrophysiology and pathophysiological changes of the heart and blood vessels. Seeing the complete process makes it easier to remember and understand.”(S3).
“I’ve seen a lot of animations during the learning process, and through this method, I have a better understanding of clinical analysis and judgment.”(S4).
“I think the course preparation process is very easy, with the help of ChatGPT, many educational resources can be found quickly, and I am even more incredible that it can produce a complete clinical simulation video! I believe I will be able to perform better in the field of clinical teaching in the future!”(T1).
Theme 2: enhancement of clinical critical thinking ability
Leveraging AI in medical and educational fields, students can utilize AI interactive platforms to simulate disease processes, enhancing their understanding of cardiovascular diseases and developing critical thinking and problem-solving skills.(S1).
“With AI assistance, my knowledge becomes more systematic and detailed. For example, when learning about acute myocardial infarction, I saw numerous relevant images such as anatomical slices of coronary arteries, their distribution, and corresponding myocardial perfusion areas, which enhances our analytical and judgment abilities.”(S2).
“During leisure time, I can use AI interactive platforms for learning and engage in question-and-answer conversations with AI, which makes self-directed learning more effective and motivating.”(S5).
“I could see the students’ progress in their learning from the exercise tests at the end of the lesson and the final Mini-CEX exam. Through the communication and discussion with them after the lesson, I found that they became more logical in their thinking about the problems, and their ability to analyse the conditions during the Mini-CEX exam was greatly improved.”(T2).
Theme 3: concerns for improvement
Regarding the application of AI in cardiovascular medicine education, students and teachers actively provided some suggestions.
“This teaching format and content are vivid and illustrative. However, I feel that some content, when interacting with AI, cannot answer my questions well.”(S3).
“With this mode of teaching, I feel that I have a higher level of mastery of this course than any other subject and am more interested and motivated to learn. I have been very willing to use ChatGPT in other courses to assist me in my studies, but I felt slightly uncomfortable communicating with the AI as opposed to the teacher.”(S4).
“This way of preparing teaching materials and the mode of lectures is indeed very innovative, with the help of ChatGPT, my pre-course preparation process will be relatively easier, and the use of it in the classroom has also greatly improved the motivation of students. However, I am concerned that the drawbacks of AI, such as academic honesty and accuracy of answers, will also have an impact on the final teaching results, so we teachers should be cautious about AI.”(T2).
With the rapid development of technology and AI, the form of medical education is undergoing continuous changes [ 23 , 24 ]. Traditional teaching mode, characterized by inefficiency and dull content, no longer meet the needs of modern medical education. This is particularly evident in the teaching of cardiovascular system diseases [ 25 ], where the content is complex and difficult to remember, often leading to a lack of student engagement and understanding during clinical practice, thereby impacting the cultivation of clinical thinking skills [ 26 ]. Currently, AI is widely applied across various fields, and research shows that it plays a crucial role in education [ 27 , 28 ], including personalized learning, intelligent tutoring, instructional design, and student assessment, greatly enhancing learning outcomes and promoting educational innovation. Moreover, studies have also shown the widespread promotion and application of scenario-based teaching models in clinical practice teaching [ 29 , 30 , 31 ].
In this study, the scenario-based teaching model is implemented based on ChatGPT 3.5. We believe that the scenario-based teaching model based on generative AI is an important mode and development direction for educational practice reform. ChatGPT, with its outstanding adaptability, versatility, efficiency, intelligence, and comprehensive coverage, has become a favored choice for many developers and is widely used in the education sector [ 32 , 33 ]. Through clever integration with the scenario-based teaching model, a new teaching experience is created.
For teachers, ChatGPT provides powerful support, significantly improving lesson preparation efficiency. Teachers can use ChatGPT’s intelligently generated dialogue scenarios to present abstract and difficult-to-understand concepts in vivid and interesting scenarios, making it easier for students to understand and remember. Additionally, teachers can adjust the generated dialogues according to students’ learning situations, personalize teaching, and improve teaching effectiveness. For students, in the scenario-based teaching model, they feel as if they are in a vivid teaching theater. They take on detective roles, cultivating clinical thinking and case analysis skills as they solve problems. ChatGPT’s intelligent dialogue can also customize learning plans based on students’ learning styles and progress, improve memory efficiency through mnemonic devices, and stimulate their interest in learning and self-directed learning motivation.
The findings of the study indicate that students enrolled in AI-assisted teaching programs exhibit higher scores in theoretical knowledge, Mini-CEX examination performance, and clinical critical thinking skills compared to their counterparts in traditional teaching settings. These results suggest that a hybrid teaching approach may enhance students’ comprehension of knowledge and proficiency in clinical procedures, this is consistent with the findings of Yujiwang et al [ 34 , 35 , 36 , 37 ]. The possible reason is that in the interaction of scenario simulation, students can independently explore the process of illness and take the initiative to find and solve problems. According to Kolb’s experiential learning model [ 19 , 20 ], experience to reflection to abstract concepts to practice, and finally to experience, interlocking and progressive, prompting students to understand knowledge from scenario simulation, then apply it to practice, and then find problems, which not only improves their independent learning ability, but also improves their critical thinking ability. Additionally, student interviews revealed that the new teaching method facilitates their exploration and identification of clinical issues, thereby preparing them effectively for future clinical practice.
Through an analysis of students’ teaching satisfaction questionnaires, it was found that the experimental group exhibited significantly higher levels of satisfaction in teaching scene, individual participation, and teaching content compared to the control group. These results suggest that the mixed teaching mode utilizing the AI platform may be more feasible and suitable for practical teaching in cardiovascular internal medicine. Although we found no statistically significant differences in course discussion, teamwork, and instructor teaching style, this may be due to the following reasons. First, the small sample size and short duration of this study limited the power to detect significant differences. Future research could improve this by increasing the sample size and extending the duration of the study. In addition, traditional teaching methods are already relatively mature in these aspects, and student satisfaction in these three aspects is already at a high level, and may not show significant advantages in the short term. At the same time, teaching satisfaction is affected by many factors, and a single change is not enough to significantly improve overall satisfaction. Therefore, we will continue to optimize the new AI-powered teaching model and strengthen its integration with course discussions and teamwork. We look forward to seeing more significant effects in future research.
Moreover, students say that the teaching method of scenario simulation not only helps them systematically understand and master the content of the course, but also stimulates their interest in independent learning and improves their ability to discover and solve problems. The vast majority of students hold a positive attitude towards the AI empowered scenario-based simulation teaching mode, and some students also put forward their own views on this teaching mode, mainly focusing on the accuracy and understanding of AI. This also provides us with valuable suggestions for the improvement of further study.
This study also has the following limitations: (1) The number of participants in the survey is relatively small, resulting in insufficient data and interview views collected; (2) In this study, we used version 3.5 of generative AI ChatGPT. However, it is worth noting that a more advanced version 4.0 of ChatGPT is already available on the market. Therefore, the version we use does not fully represent the highest computing power of AI technology.
In comparison to the conventional teaching methodology, the novel teaching mode demonstrates clear benefits. Findings from examinations, assessments, satisfaction surveys, and interviews suggest that this innovative teaching method offers a more efficient means for interns to gain contemporary professional knowledge and enhance their clinical practice proficiency. Additionally, the cultivation of clinical critical thinking and problem-solving skills through this approach is expected to greatly support their long-term career viability. The utilization of an AI-empowered scenario-based simulation teaching mode has the potential to enhance students’ engagement and motivation, as well as improve their problem-solving skills in clinical settings. Consequently, the implementation and dissemination of our AI-empowered scenario-based simulation teaching mode in cardiovascular medicine practice teaching is recommended.
Data availability
Our research encompasses sensitive personal identity information of students. Due to the potential risk of breaching individual privacy, the datasets analyzed in this study cannot be made publicly accessible. We emphasize that the data remains confidential and is not open to the public. However, if you have a compelling need for access, please reach out to the corresponding author at [email protected] to request the data.
Kose E, An T, Kikkawa A, Matsumoto Y, Hayashi H. Analysis of factors affecting rehospitalization of patients with chronic kidney disease after educational hospitalization. Clin Pharmacol. 2014;6:71–8.
Google Scholar
Pena X, Guijarro C. COPD and cardiovascular disease: more than just a co-incidence. Rev Clin Esp (Barc). 2020;220(5):290–1.
Torabi A, Khemka A, Bateman PV. A cardiology handbook app to improve medical education for internal medicine residents: development and usability study. JMIR Med Educ. 2020;6(1):e14983.
Article Google Scholar
Locke R, Mason A, Coles C, Lusznat RM, Masding MG. The development of clinical thinking in trainee physicians: the educator perspective. BMC Med Educ. 2020;20(1):226.
Pimdee P, Ridhikerd A, Moto S, Siripongdee S, Bengthong S. How social media and peer learning influence student-teacher self-directed learning in an online world under the ‘New normal’. Heliyon. 2023;9(3):e13769.
Liu CX, Ouyang WW, Wang XW, Chen D, Jiang ZL. Comparing hybrid problem-based and lecture learning (PBL + LBL) with LBL pedagogy on clinical curriculum learning for medical students in China: a meta-analysis of randomized controlled trials. Med (Baltim). 2020;99(16):e19687.
Chen M, Zhang B, Cai Z, Seery S, Gonzalez MJ, Ali NM, Ren R, Qiao Y, Xue P, Jiang Y. Acceptance of clinical artificial intelligence among physicians and medical students: a systematic review with cross-sectional survey. Front Med (Lausanne). 2022;9:990604.
Yu H. The application and challenges of ChatGPT in educational transformation: new demands for teachers’ roles. Heliyon. 2024;10(2):e24289.
Stamer T, Steinhäuser J, Flägel K. Artificial intelligence supporting the training of communication skills in the education of health care professions: scoping review. J Med Internet Res. 2023;25:e43311.
Knopp MI, Warm EJ, Weber D, Kelleher M, Kinnear B, Schumacher DJ, Santen SA, Mendonça E, Turner L. AI-enabled medical education: threads of change, promising futures, and risky realities across four potential future worlds. JMIR Med Educ. 2023;9:e50373.
Huang CY, Wang YH. Toward an integrative nursing curriculum: combining team-based and problem-based learning with emergency-care scenario simulation. Int J Environ Res Public Health. 2020;17(12):4612.
Sun L, Yang L, Wang X, Zhu J, Zhang X. Hot topics and frontier evolution in college flipped classrooms based on mapping knowledge domains. Front Public Health. 2022;10:950106.
Tseng LP, Hou TH, Huang LP, Ou YK. The effect of nursing internships on the effectiveness of implementing information technology teaching. Front Public Health. 2022;10:893199.
Tyler R, Danilova G, Kruse S, Pierce A. Innovations through virtual reality simulation. Mo Med. 2021;118(5):422–5.
Plotzky C, Lindwedel U, Sorber M, Loessl B, König P, Kunze C, et al. Virtual reality simulations in nurse education: a systematic mapping review. Nurse Educ Today. 2021;101:104868.
Harper HE, Hirt PA, Lev-Tov H. The use of virtual reality in non-burn dermatological care - a review of the literature. J Dermatolog Treat. 2022;33(1):48–53.
Sutherland J, Belec J, Sheikh A, Chepelev L, Althobaity W, Chow BJW, et al. Applying modern virtual and augmented reality technologies to medical images and models. J Digit Imaging. 2019;32(1):38–53.
Du YL, Ma CH, Liao YF, Wang L, Zhang Y, Niu G. Is clinical scenario simulation teaching effective in cultivating the competency of nursing students to recognize and assess the risk of pressure ulcers? Risk Manag Healthc Policy. 2021;14:2887–96.
Figueiredo LDF, Silva NCD, Prado MLD. Primary care nurses’ learning styles in the light of David Kolb. Rev Bras Enferm. 2022;75(6):e20210986. Published 2022 Sep 9.
Wijnen-Meijer M, Brandhuber T, Schneider A, Berberat PO. Implementing Kolb´s experiential learning cycle by linking real experience, case-based discussion and simulation. J Med Educ Curric Dev. 2022;9:23821205221091511. Published 2022 May 12.
Norcini JJ, Blank LL, Duffy FD, Fortna GS. The mini-CEX: a method for assessing clinical skills. Ann Intern Med. 2003;138(6):476–81.
Ennis RH. Critical thinking. Upper Saddle River, NJ: Prentice Hall; 1996.
Masters K. Artificial intelligence in medical education. Med Teach. 2019;41(9):976–80.
Liu J, Wang C, Liu S. Utility of ChatGPT in clinical practice. J Med Internet Res. 2023;25:e48568.
Brown DW, MacRae CA, Gardner TJ. The future of cardiovascular education and training. Circulation. 2016;133(25):2734–42.
Richards JB, Hayes MM, Schwartzstein RM. Teaching clinical reasoning and critical thinking: from cognitive theory to practical application. Chest. 2020;158(4):1617–28.
Keskinbora K, Güven F. Artificial intelligence and ophthalmology. Turk J Ophthalmol. 2020;50(1):37–43.
Nensa F, Demircioglu A, Rischpler C. Artificial intelligence in nuclear medicine. J Nucl Med. 2019;60(Suppl 2):29S-37S.
Lin YP, Liu CH, Chen YT, Li US. Scenario- and discussion-based approach for teaching preclinical medical students the socio-philosophical aspects of psychiatry. Philos Ethics Humanit Med. 2023;18(1):15.
Cannon-Bowers JA. Recent advances in scenario-based training for medical education. Curr Opin Anaesthesiol. 2008;21(6):784–9.
Sultana N, Betran AP, Khan KS, Sobhy S. Simulation-based teaching and models for caesarean sections: a systematic review to evaluate the tools for the ‘See one, practice many, do one’ slogan. Curr Opin Obstet Gynecol. 2020;32(5):305–15.
Dave T, Athaluri SA, Singh S. ChatGPT in medicine: an overview of its applications, advantages, limitations, future prospects, and ethical considerations. Front Artif Intell. 2023;6:1169595.
Lee H. The rise of ChatGPT: exploring its potential in medical education. Anat Sci Educ. 2023 Mar 14.
Wang Y, Peng Y, Huang Y. The effect of typical case discussion and scenario simulation on the critical thinking of midwifery students: evidence from China. BMC Med Educ. 2024;24:340.
Holdsworth C, Skinner EH, Delany CM. Using simulation pedagogy to teach clinical education skills: a randomized trial. Physiother Theory Pract. 2016;32(4):284–95.
Lapkin S, Fernandez R, Levett-Jones T, Bellchambers H. The effectiveness of using human patient simulation manikins in the teaching of clinical reasoning skills to undergraduate nursing students: a systematic review. JBI Libr Syst Rev. 2010;8(16):661–94.
Demirören M, Turan S, Öztuna D. Medical students’ self-efficacy in problem-based learning and its relationship with self-regulated learning. Med Educ Online. 2016;21:30049.
Download references
Acknowledgements
Not applicable.
Innovation and Entrepreneurship Training Program for College Students in Jiangsu Province (202313993027Y). Teaching Reform Research Project of Nantong University (2023B10).
Author information
Koulong Zheng, Zhiyu Shen and Zanhao Chen contributed equally to this work.
Authors and Affiliations
Nantong University, Qi Xiu Road, Nantong, Jiangsu, 226007, China
Koulong Zheng, Zhiyu Shen, Zanhao Chen, Chang Che & Huixia Zhu
The Second Affiliated Hospital of Nantong University, Nantong, Jiangsu, 226001, China
Koulong Zheng
You can also search for this author in PubMed Google Scholar
Contributions
KLZ and HXZ designed the trial. KLZ prepared the clinical cases. HXZ collected the data. HXZ and ZYS analyzed the data. HXZ, ZHC and CC wrote the manuscript. All authors have read and approved the final manuscript.
Corresponding author
Correspondence to Huixia Zhu .
Ethics declarations
Ethics approval and consent to participate.
This study has obtained ethical approval from the Ethics Committee of the Second Affiliated Hospital of Nantong University. All methods were conducted in accordance with relevant guidelines and regulations(approval number 2024KT045). All participation was voluntary and signed informed consent forms were obtained from each participant.
Consent for publication
Competing interests.
The authors declare no competing interests.
Additional information
Publisher’s note.
Springer Nature remains neutral with regard to jurisdictional claims in published maps and institutional affiliations.
Rights and permissions
Open Access This article is licensed under a Creative Commons Attribution-NonCommercial-NoDerivatives 4.0 International License, which permits any non-commercial use, sharing, distribution and reproduction in any medium or format, as long as you give appropriate credit to the original author(s) and the source, provide a link to the Creative Commons licence, and indicate if you modified the licensed material. You do not have permission under this licence to share adapted material derived from this article or parts of it. The images or other third party material in this article are included in the article’s Creative Commons licence, unless indicated otherwise in a credit line to the material. If material is not included in the article’s Creative Commons licence and your intended use is not permitted by statutory regulation or exceeds the permitted use, you will need to obtain permission directly from the copyright holder. To view a copy of this licence, visit http://creativecommons.org/licenses/by-nc-nd/4.0/ .
Reprints and permissions
About this article
Cite this article.
Zheng, K., Shen, Z., Chen, Z. et al. Application of AI-empowered scenario-based simulation teaching mode in cardiovascular disease education. BMC Med Educ 24 , 1003 (2024). https://doi.org/10.1186/s12909-024-05977-z
Download citation
Received : 15 February 2024
Accepted : 02 September 2024
Published : 13 September 2024
DOI : https://doi.org/10.1186/s12909-024-05977-z
Share this article
Anyone you share the following link with will be able to read this content:
Sorry, a shareable link is not currently available for this article.
Provided by the Springer Nature SharedIt content-sharing initiative
- Artificial intelligence
- Cardiovascular diseases
- Educational measurement
- Medical education
BMC Medical Education
ISSN: 1472-6920
- General enquiries: [email protected]

IMAGES
VIDEO
COMMENTS
Step 1: Define your variables. You should begin with a specific research question. We will work with two research question examples, one from health sciences and one from ecology: Example question 1: Phone use and sleep. You want to know how phone use before bedtime affects sleep patterns.
A thorough and comprehensive guide to the theoretical, practical, and methodological approaches used in survey experiments across disciplines such as political science, health sciences, sociology, economics, psychology, and marketing This book explores and explains the broad range of experimental designs embedded in surveys that use both probability and non-probability samples. It approaches ...
Core theme of this book is a heuristic called the question-design-analysis bridge: there is a bridge connecting research questions and hypotheses, experimental design and sampling procedures, and ...
The research design is a strategy for answering your research questions. It determines how you will collect and analyze your data. ... Experimental design example In an experimental design, ... Survey methods. Surveys allow you to collect data about opinions, behaviors, experiences, and characteristics by asking people directly.
Step 1: establish your question and set variables. In the first stage, establish your research question, and use it to distinguish between dependent and independent variables. Independent vs. dependent variables. Follow these steps to apply experimental research design to your surveys to gain more insight and make them more actionable.
A research design is a strategy for answering your research question using empirical data. Creating a research design means making decisions about: ... Example: Experimental design In an experimental design, ... Survey methods. Surveys allow you to collect data about opinions, behaviours, experiences, ...
Experimental design is a research method that enables researchers to assess the effect of multiple factors on an outcome.. You can determine the relationship between each of the variables by: Manipulating one or more independent variables (i.e., stimuli or treatments). Applying the changes to one or more dependent variables (i.e., test groups or outcomes)
Abstract. Experimental research design is centrally concerned with constructing research that is high in causal (internal) validity. Randomized experimental designs provide the highest levels of causal validity. Quasi-experimental designs have a number of potential threats to their causal validity. Yet, new quasi-experimental designs adopted ...
Random assignment is a method for assigning participants in a sample to the different conditions, and it is an important element of all experimental research in psychology and other fields too. In its strictest sense, random assignment should meet two criteria. One is that each participant has an equal chance of being assigned to each condition ...
<p>A thorough and comprehensive guide to the theoretical, practical, and methodological approaches used in survey experiments across disciplines such as political science, health sciences, sociology, economics, psychology, and marketing</p> <p>This book explores and explains the broad range of experimental designs embedded in surveys that use both probability and non-probability samples ...
Unlike experimental designs, descriptive survey research design s do not involve manipulation or control of variables. Instead, they aim to describe characteristics of a population or phenomenon as they naturally occur. Attributes of descriptive design include: No manipulation: The researcher observes without intervening in the natural setting.
An experimental design is a detailed plan for collecting and using data to identify causal relationships. Through careful planning, the design of experiments allows your data collection efforts to have a reasonable chance of detecting effects and testing hypotheses that answer your research questions. An experiment is a data collection ...
10 Experimental research. 10. Experimental research. Experimental research—often considered to be the 'gold standard' in research designs—is one of the most rigorous of all research designs. In this design, one or more independent variables are manipulated by the researcher (as treatments), subjects are randomly assigned to different ...
There are 3 types of experimental research designs. These are pre-experimental research design, true experimental research design, and quasi experimental research design. 1. The assignment of the control group in quasi experimental research is non-random, unlike true experimental design, which is randomly assigned. 2.
The survey research design is the use of a survey, administered either in written form or orally, to quan-tify, describe, or characterize an individual or a group. A survey is a series of questions or statements, called items, used in a questionnaire or an interview to mea-sure the self-reports or responses of respondents.
What is experimental research design?. Here is a brief overview from the SAGE Encyclopedia of Survey Research Methods:. Experimental design is one of several forms of scientific inquiry employed to identify the cause-and-effect relation between two or more variables and to assess the magnitude of the effect(s) produced. The independent variable is the experiment or treatment applied (e.g. a ...
Types of Research Designs Compared | Guide & Examples. Published on June 20, 2019 by Shona McCombes.Revised on June 22, 2023. When you start planning a research project, developing research questions and creating a research design, you will have to make various decisions about the type of research you want to do.. There are many ways to categorize different types of research.
Study, experimental, or research design is the backbone of good research. It directs the experiment by orchestrating data collection, defines the statistical analysis of the resultant data, and guides the interpretation of the results. When properly described in the written report of the experiment, it serves as a road map to readers, 1 helping ...
True Experimental Research Design. The true experimental research design relies on statistical analysis to approve or disprove a hypothesis. It is the most accurate type of experimental design and may be carried out with or without a pretest on at least 2 randomly assigned dependent subjects. The true experimental research design must contain a ...
Business: Research design is used in the field of business to investigate consumer behavior, marketing strategies, and the impact of different business practices. Researchers use various designs, such as survey research, experimental research, and case studies, to study different aspects of the business world.
A scientific procedure wherein the factor under study is isolated to test hypothesis is called an experiment. Surveys are performed when the research is of descriptive nature, whereas in the case of experiments are conducted in experimental research. The survey samples are large as the response rate is low, especially when the survey is ...
Context: Abortions are substantially underreported in surveys due to social stigma, compromising the study of abortion, pregnancy, fertility, and related demographic and health outcomes. Methods: In this study, we evaluated six methodological approaches identified through formative mixed‐methods research to improve the measurement of abortion in surveys. These approaches included altering ...
Random assignment is a method for assigning participants in a sample to the different conditions, and it is an important element of all experimental research in psychology and other fields too. In its strictest sense, random assignment should meet two criteria. One is that each participant has an equal chance of being assigned to each condition ...
In this study, we overcome these challenges by using a quasi-experimental design in which we leverage the timing of a police violence event relative to a wave of survey data collection. This approach is commonly called "unexpected event during survey design," or UESD [43, 44]. Under the assumptions that underlie this research design ...
True experimental design Quasi-experimental design; Assignment to treatment: The researcher randomly assigns subjects to control and treatment groups.: Some other, non-random method is used to assign subjects to groups. Control over treatment: The researcher usually designs the treatment.: The researcher often does not have control over the treatment, but instead studies pre-existing groups ...
Experimental design. A combination of quasi-experimental research design and descriptive qualitative research methods was employed to form both a control group and an experimental group. Our study integrated Kolb's experiential learning model into the experimental group's teaching methods to enhance the learning process [19, 20]. Kolb's ...