Copyright © 2024 AudiologyOnline - All Rights Reserved
- Back to Basics: Speech Audiometry

Janet R. Schoepflin, PhD
- Hearing Evaluation - Adults
Editor's Note: This is a transcript of an AudiologyOnline live seminar. Please download supplemental course materials . Speech is the auditory stimulus through which we communicate. The recognition of speech is therefore of great interest to all of us in the fields of speech and hearing. Speech audiometry developed originally out of the work conducted at Bell Labs in the 1920s and 1930s where they were looking into the efficiency of communication systems, and really gained momentum post World War II as returning veterans presented with hearing loss. The methods and materials for testing speech intelligibility were of interest then, and are still of interest today. It is due to this ongoing interest as seen in the questions that students ask during classes, by questions new audiologists raise as they begin their practice, and by the comments and questions we see on various audiology listservs about the most efficient and effective ways to test speech in the clinical setting, that AudiologyOnline proposed this webinar as part of their Back to Basics series. I am delighted to participate. I am presenting a review of the array of speech tests that we use in clinical evaluation with a summary of some of the old and new research that has come about to support the recommended practices. The topics that I will address today are an overview of speech threshold testing, suprathreshold speech recognition testing, the most comfortable listening level testing, uncomfortable listening level, and a brief mention of some new directions that speech testing is taking. In the context of testing speech, I will assume that the environment in which you are testing meets the ANSI permissible noise criteria and that the audiometer transducers that are being used to perform speech testing are all calibrated to the ANSI standards for speech. I will not be talking about those standards, but it's of course important to keep those in mind.
Speech Threshold testing involves several considerations. They include the purposes of the test or the reasons for performing the test, the materials that should be used in testing, and the method or procedure for testing. Purposes of Speech Threshold Testing A number of purposes have been given for speech threshold testing. In the past, speech thresholds were used as a means to cross-check the validity of pure tone thresholds. This purpose lacks some validity because we have other physiologic and electrophysiologic procedures like OAEs and imittance test results to help us in that cross-check. However, the speech threshold measure is a test of hearing. It is not entirely invalid to be performed as a cross-check for pure tone hearing. I think sometimes we are anxious to get rid of things because we feel we have a better handle from other tests, but in this case, it may not be the wisest thing to toss out. Also in past years, speech thresholds were used to determine the level for suprathreshold speech recognition testing. That also lacks validity, because the level at which suprathreshold testing is conducted depends on the reason you are doing the test itself. It is necessary to test speech thresholds if you are going to bill 92557. Aside from that, the current purpose for speech threshold testing is in the evaluation of pediatric and difficult to test patients. Clinical practice surveys tell us that the majority of clinicians do test speech thresholds for all their patients whether it is for billing purposes or not. It is always important that testing is done in the recommended, standardized manner. The accepted measures for speech thresholds are the Speech Recognition Threshold (SRT) and the Speech Detection Threshold (SDT). Those terms are used because they specify the material or stimulus, i.e. speech, as well as the task that the listener is required to do, which is recognition or identification in the case of the SRT, and detection or noticing of presence versus absence of the stimulus in the case of SDT. The terms also specify the criterion for performance which is threshold or generally 50%. The SDT is most commonly performed on those individuals who have been unable to complete an SRT, such as very young children. Because recognition is not required in the speech detection task, it is expected that the SDT will be about 5 to 10 dB better than the SRT, which requires recognition of the material. Materials for Speech Threshold Testing The materials that are used in speech threshold testing are spondees, which are familiar two-syllable words that have a fairly steep psychometric function. Cold running speech or connected discourse is an alternative for speech detection testing since recognition is not required in that task. Whatever material is used, it should be noted on the audiogram. It is important to make notations on the audiogram about the protocols and the materials we are using, although in common practice many of us are lax in doing so. Methods for Speech Threshold Testing The methods consideration in speech threshold testing is how we are going to do the test. This would include whether we use monitored live voice or recorded materials, and whether we familiarize the patient with the materials and the technique that we use to elicit threshold. Monitored live voice and recorded speech can both be used in SRT testing. However, recorded presentation is recommended because recorded materials standardize the test procedure. With live voice presentation, the monitoring of each syllable of each spondee, so that it peaks at 0 on the VU meter can be fairly difficult. The consistency of the presentation is lost then. Using recorded materials is recommended, but it is less important in speech threshold testing than it is in suprathreshold speech testing. As I mentioned with the materials that are used, it is important to note on the audiogram what method of presentation has been used. As far as familiarization goes, we have known for about 50 years, since Tillman and Jerger (1959) identified familiarity as a factor in speech thresholds, that familiarization of the patient with the test words should be included as part of every test. Several clinical practice surveys suggest that familiarization is not often done with the patients. This is not a good practice because familiarization does influence thresholds and should be part of the procedure. The last consideration under methods is regarding the technique that is going to be used. Several different techniques have been proposed for the determination of SRT. Clinical practice surveys suggest the most commonly used method is a bracketing procedure. The typical down 10 dB, up 5 dB is often used with two to four words presented at each level, and the threshold then is defined as the lowest level at which 50% or at least 50% of the words are correctly repeated. This is not the procedure that is recommended by ASHA (1988). The ASHA-recommended procedure is a descending technique where two spondees are presented at each decrement from the starting level. There are other modifications that have been proposed, but they are not widely used.
Suprathreshold speech testing involves considerations as well. They are similar to those that we mentioned for threshold tests, but they are more complicated than the threshold considerations. They include the purposes of the testing, the materials that should be used in testing, whether the test material should be delivered via monitored live voice or recorded materials, the level or levels at which the testing should be conducted, whether a full list, half list, or an abbreviated word list should be used, and whether or not the test should be given in quiet or noise. Purposes of Suprathreshold Testing There are several reasons to conduct suprathreshold tests. They include estimating the communicative ability of the individual at a normal conversational level; determining whether or not a more thorough diagnostic assessment is going to be conducted; hearing aid considerations, and analysis of the error patterns in speech recognition. When the purpose of testing is to estimate communicative ability at a normal conversational level, then the test should be given at a level around 50 to 60 dBHL since that is representative of a normal conversational level at a communicating distance of about 1 meter. While monosyllabic words in quiet do not give a complete picture of communicative ability in daily situations, it is a procedure that people like to use to give some broad sense of overall communicative ability. If the purpose of the testing is for diagnostic assessment, then a psychometric or performance-intensity function should be obtained. If the reason for the testing is for hearing aid considerations, then the test is often given using words or sentences and either in quiet or in a background of noise. Another purpose is the analysis of error patterns in speech recognition and in that situation, a test other than some open set monosyllabic word test would be appropriate. Materials for Suprathreshold Testing The choice of materials for testing depends on the purpose of the test and on the age and abilities of the patients. The issues in materials include the set and the test items themselves.
Closed set vs. Open set. The first consideration is whether a closed set or an open set is appropriate. Closed set tests limit the number of response alternatives to a fairly small set, usually between 4 and 10 depending on the procedure. The number of alternatives influences the guess rate. This is a consideration as well. The Word Intelligibility by Picture Identification or the WIPI test is a commonly used closed set test for children as it requires only the picture pointing response and it has a receptive language vocabulary that is as low as about 5 years. It is very useful in pediatric evaluations as is another closed set test, the Northwestern University Children's Perception of Speech test (NU-CHIPS).
In contrast, the open set protocol provides an unlimited number of stimulus alternatives. Therefore, open set tests are more difficult. The clinical practice surveys available suggest for routine audiometric testing that monosyllabic word lists are the most widely used materials in suprathreshold speech recognition testing for routine evaluations, but sentences in noise are gaining popularity for hearing aid purposes.
CID W-22 vs. NU-6. The most common materials for speech recognition testing are the monosyllabic words, the Central Institute of the Deaf W-22 and the Northwestern University-6 word list. These are the most common open set materials and there has been some discussion among audiologists concerning the differences between those. From a historical perspective, the CID W-22 list came from the original Harvard PAL-PB50 words and the W-22s are a group of the more familiar of those. They were developed into four 50-word lists. They are still commonly used by audiologists today. The NU-6 lists were developed later and instead of looking for phonetic balance, they considered a more phonemic balance. The articulation function for both of those using recorded materials is about the same, 4% per dB. The NU-6 tests are considered somewhat more difficult than the W-22s. Clinical surveys show that both materials are used by practicing audiologists, with usage of the NU-6 lists beginning to surpass usage of W-22s.
Nonsense materials. There are other materials that are available for suprathreshold speech testing. There are other monosyllabic word lists like the Gardner high frequency word list (Gardner, 1971) that could be useful for special applications or special populations. There are also nonsense syllabic tasks which were used in early research in communication. An advantage of the nonsense syllables is that the effects of word familiarity and lexical constraints are reduced as compared to using actual words as test materials. A few that are available are the City University of New York Nonsense Syllable test, the Nonsense Syllable test, and others.
Sentence materials. Sentence materials are gaining popularity, particularly in hearing aid applications. This is because speech that contains contextual cues and is presented in a noise background is expected to have better predictive validity than words in quiet. The two sentence procedures that are popular are the Hearing In Noise Test (HINT) (Nilsson, Soli,& Sullivan, 1994) and the QuickSIN (Killion, Niquette, Gudmundsen, Revit & Banerjee, 2004). Other sentence tests that are available that have particular applications are the Synthetic Sentence Identification test (SSI), the Speech Perception and Noise test (SPIN), and the Connected Speech test.
Monitored Live Voice vs. Recorded. As with speech threshold testing, the use of recorded materials for suprathreshold speech testing standardizes the test administration. The recorded version of the test is actually the test in my opinion. This goes back to a study in 1969 where the findings said the test is not just the written word list, but rather it is a recorded version of those words.
Inter-speaker and intra-speaker variability makes using recorded materials the method of choice in almost all cases for suprathreshold testing. Monitored live voice (MLV) is not recommended. In years gone by, recorded materials were difficult to manipulate, but the ease and flexibility that is afforded us by CDs and digital recordings makes recorded materials the only way to go for testing suprathreshold speech recognition. Another issue to consider is the use of the carrier phrase. Since the carrier phrase is included on recordings and recorded materials are the recommended procedure, that issue is settled. However, I do know that monitored live voice is necessary in certain situations and if monitored live voice is used in testing, then the carrier phrase should precede the test word. In monitored live voice, the carrier phrase is intended to allow the test word to have its own natural inflection and its own natural power. The VU meter should peak at 0 for the carrier phrase and the test word then is delivered at its own natural or normal level for that word in the phrase.
Levels. The level at which testing is done is another consideration. The psychometric or performance-intensity function plots speech performance in percent correct on the Y-axis, as a function of the level of the speech signal on the X-axis. This is important because testing at only one level, which is fairly common, gives us insufficient information about the patient's optimal performance or what we commonly call the PB-max. It also does not allow us to know anything about any possible deterioration in performance if the level is increased. As a reminder, normal hearers show a function that reaches its maximum around 25 to 40 dB SL (re: SRT) and that is the reason why suprathreshold testing is often conducted at that level. For normals, the performance remains at that level, 100% or so, as the level increases. People with conductive hearing loss also show a similar function. Individuals with sensorineural hearing loss, however, show a performance function that reaches its maximum at generally less than 100%. They can either show performance that stays at that level as intensity increases, or they can show a curve that reaches its maximum and then decreases in performance as intensity increases. This is known as roll-over. A single level is not the best way to go as we cannot anticipate which patients may have rollover during testing, unless we test at a level higher than where the maximum score was obtained. I recognize that there are often time constraints in everyday practice, but two levels are recommended so that the performance-intensity function can be observed for an individual patient at least in an abbreviated way.
Recently, Guthrie and Mackersie (2009) published a paper that compared several different presentation levels to ascertain which level would result in maximum word recognition in individuals who had different hearing loss configurations. They looked at a number of presentation levels ranging from 10 dB above the SRT to a level at the UCL (uncomfortable listening level) -5 dB. Their results indicated that individuals with mild to moderate losses and those with more steeply sloping losses reached their best scores at a UCL -5 dB. That was also true for those patients who had moderately-severe to severe losses. The best phoneme recognition scores for their populations were achieved at a level of UCL -5 dB. As a reminder about speech recognition testing, masking is frequently needed because the test is being presented at a level above threshold, in many cases well above the threshold. Masking will always be needed for suprathreshold testing when the presentation level in the test ear is 40 dB or greater above the best bone conduction threshold in the non-test ear if supra-aural phones are used.
Full lists vs. half-lists. Another consideration is whether a full list or a half-list should be administered. Original lists were composed of 50 words and those 50 words were created for phonetic balance and for simplicity in scoring. It made it easy for the test to be scored if 50 words were administered and each word was worth 2%. Because 50-word lists take a long time, people often use half-lists or even shorter lists for the purpose of suprathreshold speech recognition testing. Let's look into this practice a little further.
An early study was done by Thornton and Raffin (1978) using the Binomial Distribution Model. They investigated the critical differences between one score and a retest score that would be necessary for those scores to be considered statistically significant. Their findings showed that with an increasing set size, variability decreased. It would seem that more items are better. More recently Hurley and Sells (2003) conducted a study that looked at developing a test methodology that would identify those patients requiring a full 50 item suprathreshold test and allow abbreviated testing of patients who do not need a full 50 item list. They used Auditec recordings and developed 10-word and 25-word screening tests. They found that the four lists of NU-6 10-word and the 25-word screening tests were able to differentiate listeners who had impaired word recognition who needed a full 50-word list from those with unimpaired word recognition ability who only needed the 10-word or 25-word list. If abbreviated testing is important, then it would seem that this would be the protocol to follow. These screening lists are available in a recorded version and their findings were based on a recorded version. Once again, it is important to use recorded materials whether you are going to use a full list or use an abbreviated list.
Quiet vs. Noise. Another consideration in suprathreshold speech recognition testing is whether to test in quiet or in noise. The effects of sensorineural hearing loss beyond the threshold loss, such as impaired frequency resolution or impaired temporal resolution, makes speech recognition performance in quiet a poor predictor for how those individuals will perform in noise. Speech recognition in noise is being promoted by a number of experts because adding noise improves the sensitivity of the test and the validity of the test. Giving the test at several levels will provide for a better separation between people who have hearing loss and those who have normal hearing. We know that individuals with hearing loss have a lot more difficulty with speech recognition in noise than those with normal hearing, and that those with sensorineural hearing loss often require a much greater signal-to-noise ratio (SNR), 10 to 15 better, than normal hearers.
Monosyllabic words in noise have not been widely used in clinical evaluation. However there are several word lists that are available. One of them is the Words in Noise test or WIN test which presents NU-6 words in a multi-talker babble. The words are presented at several different SNRs with the babble remaining at a constant level. One of the advantages of using these kinds of tests is that they are adaptive. They can be administered in a shorter period of time and they do not run into the same problems that we see with ceiling effects and floor effects. As I mentioned earlier, sentence tests in noise have become increasingly popular in hearing aid applications. Testing speech in noise is one way to look at amplification pre and post fitting. The Hearing in Noise Test and QuickSin, have gained popularity in those applications. The HINT was developed by Nilsson and colleagues in 1994 and later modified. It is scored as the dB to noise ratio that is necessary to get a 50% correct performance on the sentences. The sentences are the BKB (Bamford-Kowal-Bench) sentences. They are presented in sets of 10 and the listener listens and repeats the entire sentence correctly in order to get credit. In the HINT, the speech spectrum noise stays constant and the signal level is varied to obtain that 50% point. The QuickSin is a test that was developed by Killion and colleagues (2004) and uses the IEEE sentences. It has six sentences per list with five key words that are the scoring words in each sentence. All of them are presented in a multi-talker babble. The sentences get presented one at a time in 5 dB decrements from a high positive SNR down to 0 dB SNR. Again the test is scored as the 50% point in terms of dB signal-to-noise ratio. The guide proposed by Killion on the SNR is if an individual has somewhere around a 0 to 3 dB SNR it would be considered normal, 3 to 7 would be a mild SNR loss, 7 to15 dB would be a moderate SNR loss, and greater than 15 dB would be a severe SNR loss.
Scoring. Scoring is another issue in suprathreshold speech recognition testing. It is generally done on a whole word basis. However phoneme scoring is another option. If phoneme scoring is used, it is a way of increasing the set size and you have more items to score without adding to the time of the test. If whole word scoring is used, the words have to be exactly correct. In this situation, being close does not count. The word must be absolutely correct in order to be judged as being correct. Over time, different scoring categorizations have been proposed, although the percentages that are attributed to those categories vary among the different proposals.
The traditional categorizations include excellent, good, fair, poor, and very poor. These categories are defined as:
- Excellent or within normal limits = 90 - 100% on whole word scoring
- Good or slight difficulty = 78 - 88%
- Fair to moderate difficulty = 66 - 76%
- Poor or great difficulty = 54 - 64 %
- Very poor is < 52%
A very useful test routinely administered to those who are being considered for hearing aids is the level at which a listener finds listening most comfortable. The materials that are used for this are usually cold running speech or connected discourse. The listener is asked to rate the level at which listening is found to be most comfortable. Several trials are usually completed because most comfortable listening is typically a range, not a specific level or a single value. People sometimes want sounds a little louder or a little softer, so the range is a more appropriate term for this than most comfortable level. However whatever is obtained, whether it is a most comfortable level or a most comfortable range, should be recorded on the audiogram. Again, the material used should also be noted on the audiogram. As I mentioned earlier the most comfortable level (MCL) is often not the level at which a listener achieves maximum intelligibility. Using MCL in order to determine where the suprathreshold speech recognition measure will be done is not a good reason to use this test. MCL is useful, but not for determining where maximum intelligibility will be. The study I mentioned earlier showed that maximum intelligibility was reached for most people with hearing loss at a UCL -5. MCL is useful however in determining ANL or acceptable noise level.
The uncomfortable listening level (UCL) is also conducted with cold running speech. The instructions for this test can certainly influence the outcome since uncomfortable or uncomfortably loud for some individuals may not really be their UCL, but rather a preference for listening at a softer level. It is important to define for the patient what you mean by uncomfortably loud. The utility of the UCL is in providing an estimate for the dynamic range for speech which is the difference between the UCL and the SRT. In normals, this range is usually 100 dB or more, but it is reduced in ears with sensorineural hearing loss often dramatically. By doing the UCL, you can get an estimate of the individual's dynamic range for speech.
Acceptable Noise Level (ANL) is the amount of background noise that a listener is willing to accept while listening to speech (Nabelek, Tucker, & Letowski, 1991). It is a test of noise tolerance and it has been shown to be related to the successful use of hearing aids and to potential benefit with hearing aids (Nabelek, Freyaldenhoven, Tampas, & Muenchen, 2006). It uses the MCL and a measure known as BNL or background noise level. To conduct the test, a recorded speech passage is presented to the listener in the sound field for the MCL. Again note the use of recorded materials. The noise is then introduced to the listener to a level that will be the highest level that that person is able to accept or "put up with" while they are listening to and following the story in the speech passage. The ANL then becomes the difference between the MCL and the BNL. Individuals that have very low scores on the ANL are considered successful hearing aid users or good candidates for hearing aids. Those that have very high scores are considered unsuccessful users or poor hearing aid candidates. Obviously there are number of other applications for speech in audiologic practice, not the least of which is in the assessment of auditory processing. Many seminars could be conducted on this topic alone. Another application or future direction for speech audiometry is to more realistically assess hearing aid performance in "real world" environments. This is an area where research is currently underway.
Question: Are there any more specific instructions for the UCL measurement? Answer: Instructions are very important. We need to make it clear to a patient exactly what we expect them to do. I personally do not like things loud. If I am asked to indicate what is uncomfortably loud, I am much below what is really my UCL. I think you have to be very direct in instructing your patients in that you are not looking for a little uncomfortable, but where they just do not want to hear it or cannot take it. Question: Can you sum up what the best methods are to test hearing aid performance? I assume this means with speech signals. Answer: I think the use of the HINT or the QuickSin would be the most useful on a behavioral test. We have other ways of looking at performance that are not behavioral. Question: What about dialects? In my area, some of the local dialects have clipped words during speech testing. I am not sure if I should count those as correct or incorrect. Answer: It all depends on your situation. If a patient's production is really reflective of the dialect of that region and they are saying the word as everyone else in that area would say it, then I would say they do have the word correct. If necessary, if you are really unclear, you can always ask the patient to spell the word or write it down. This extra time can be inconvenient, but that is the best way to be sure that they have correctly identified the word. Question: Is there a reference for the bracketing method? Answer: The bracketing method is based on the old modified Hughson-Westlake that many people use for pure tone threshold testing. It is very similar to that traditional down 10 dB, up 5 dB. I am sure there are more references, but the Hughson-Westlake is what bracketing is based on. Question: Once you get an SRT result, if you want to compare it to the thresholds to validate your pure tones, how do you compare it to the audiogram? Answer: If it is a flat hearing loss, then you can compare to the 3-frequency pure tone average (PTA). If there is a high frequency loss, where audibility at perhaps 2000 Hz is greatly reduced, then it is better to use just the average of 500Hz and 1000Hz as your comparison. If it is a steeply sloping loss, then you look for agreement with the best threshold, which would probably be the 500 Hz threshold. The reverse is also true for patients who have rising configurations. Compare the SRT to the best two frequencies of the PTA, if the loss has either a steep slope or a steep rise, or the best frequency in the PTA if it is a really precipitous change in configuration. Question: Where can I find speech lists in Russian or other languages? Answer: Auditec has some material available in languages other than English - it would be best to contact them directly. You can also view their catalog at www.auditec.com Carolyn Smaka: This raises a question I have. If an audiologist is not fluent in a particular language, such as Spanish, is it ok to obtain a word list or recording in that language and conduct speech testing? Janet Schoepflin: I do not think that is a good practice. If you are not fluent in a language, you do not know all the subtleties of that language and the allophonic variations. People want to get an estimation of suprathreshold speech recognition and this would be an attempt to do that. This goes along with dialect. Whether you are using a recording, or doing your best to say these words exactly as there are supposed to be said, and your patient is fluent in a language and they say the word back to you, since you are not familiar with all the variations in the language it is possible that you will score the word incorrectly. You may think it is correct when it is actually incorrect, or you may think it is incorrect when it is correct based on the dialect or variation of that language. Question: In school we were instructed to use the full 50-word list for any word discrimination testing at suprathreshold, but if we are pressed for time, a half word list would be okay. However, my professor warned us that we absolutely must go in order on the word list. Can you clarify this? Answer: I'm not sure why that might have been said. I was trained in the model to use the 50-word list. This was because the phonetic balance that was proposed for those words was based on the 50 words. If you only used 25 words, you were not getting the phonetic balance. I think the more current findings from Hurley and Sells show us that it is possible to use a shorter list developed specifically for this purpose. It should be the recorded version of those words. These lists are available through Auditec. Question: On the NU-6 list, the words 'tough' and 'puff' are next to each other. 'Tough' is often mistaken for 'puff' so then when we reads 'puff', the person looks confused. Is it okay to mix up the order on the word list? Answer: I think in that case it is perfectly fine to move that one word down. Question: When do you recommend conducting speech testing, before or after pure tone testing? Answer: I have always been a person who likes to interact with my patients. My own procedure is to do an SRT first. Frequently for an SRT I do use live voice. I do not use monitored live voice for suprathreshold testing. It gives me a time to interact with the patient. People feel comfortable with speech. It is a communicative act. Then I do pure tone testing. Personally I would not do suprathreshold until I finished pure tone testing. My sequence is often SRT, pure tone, and suprathreshold. If this is not a good protocol for you based on time, then I would conduct pure tone testing, SRT, and then suprathreshold. Question: Some of the spondee words are outdated such as inkwell and whitewash. Is it okay to substitute other words that we know are spondee words, but may not be on the list? Or if we familiarize people, does it matter? Answer: The words that are on the list were put there for their so-called familiarity, but also because they were somewhat homogeneous and equal in intelligibility. I think inkwell, drawbridge and whitewash are outdated. If you follow a protocol where you are using a representative sample of the words and you are familiarizing, I think it is perfectly fine to eliminate those words you do not want to use. You just do not want to end up only using five or six words as it will limit the test set. Question: At what age is it appropriate to expect a child to perform suprathreshold speech recognition testing? Answer: If the child has a receptive language age of around 4 or 5 years, even 3 years maybe, it is possible to use the NU-CHIPS as a measure. It really does depend on language more than anything else, and the fact that the child can sit still for a period of time to do the test. Question: Regarding masking, when you are going 40 dB above the bone conduction threshold in the non-test ear, what frequency are you looking at? Are you comparing speech presented at 40 above a pure tone average of the bone conduction threshold? Answer: The best bone conduction threshold in the non-test ear is what really should be used. Question: When seeing a patient in follow-up after an ENT prescribes a steroid therapy for hydrops, do you recommend using the same word list to compare their suprathreshold speech recognition? Answer: I think it is better to use a different list, personally. Word familiarity as we said can influence even threshold and it certainly can affect suprathreshold performance. I think it is best to use a different word list. Carolyn Smaka: Thanks to everyone for their questions. Dr. Schoepflin has provided her email address with the handout. If your question was not answered or if you have further thoughts after the presentation, please feel free to follow up directly with her via email. Janet Schoepflin: Thank you so much. It was my pleasure and I hope everyone found the presentation worthwhile.
American Speech, Language and Hearing Association. (1988). Determining Threshold Level for Speech [Guidelines]. Available from www.asha.org/policy Gardner, H.(1971). Application of a high-frequency consonant discrimination word list in hearing-aid evaluation. Journal of Speech and Hearing Disorders, 36 , 354-355. Guthrie, L. & Mackersie, C. (2009). A comparison of presentation levels to maximize word recognition scores. Journal of the American Academy of Audiology, 20 (6), 381-90. Hurley, R. & Sells, J. (2003). An abbreviated word recognition protocol based on item difficulty. Ear & Hearing, 24 (2), 111-118. Killion, M., Niquette, P., Gudmundsen, G., Revit, L., & Banerjee, S. (2004). Development of a quick speech-in-noise test for measuring signal-to-noise ratio loss in normal-hearing and hearing-impaired listeners. Journal of the Acoustical Society of America, 116 (4 Pt 1), 2395-405. Nabelek, A., Freyaldenhoven, M., Tampas, J., Burchfield, S., & Muenchen, R. (2006). Acceptable noise level as a predictor of hearing aid use. Journal of the American Academy of Audiology, 17 , 626-639. Nabelek, A., Tucker, F., & Letowski, T. (1991). Toleration of background noises: Relationship with patterns of hearing aid use by elderly persons. Journal of Speech and Hearing Research, 34 , 679-685. Nilsson, M., Soli. S,, & Sullivan, J. (1994). Development of the hearing in noise test for the measurement of speech reception thresholds in quiet and in noise. Journal of the Acoustical Society of America, 95 (2), 1085-99. Thornton, A.. & Raffin, M, (1978). Speech-discrimination scores modeled as a binomial variable. Journal of Speech and Hearing Research, 21 , 507-518. Tillman, T., & Jerger, J. (1959). Some factors affecting the spondee threshold in normal-hearing subjects. Journal of Speech and Hearing Research, 2 , 141-146.

Chair, Communication Sciences and Disorders, Adelphi University
Janet Schoepflin is an Associate Professor and Chair of the Department of Communication Sciences and Disorders at Adelphi University and a member of the faculty of the Long Island AuD Consortium. Her areas of research interest include speech perception in children and adults, particularly those with hearing loss, and the effects of noise on audition and speech recognition performance.
Related Courses
Using gsi for cochlear implant evaluations, course: #39682 level: introductory 1 hour, empowerment and behavioral insights in client decision making, presented in partnership with nal, course: #37124 level: intermediate 1 hour, cognition and audition: supporting evidence, screening options, and clinical research, course: #37381 level: introductory 1 hour, innovative audiologic care delivery, course: #38661 level: intermediate 4 hours, aurical hit applications part 1 - applications for hearing instrument fittings and beyond, course: #28678 level: intermediate 1 hour.
Our site uses cookies to improve your experience. By using our site, you agree to our Privacy Policy .
American Speech-Language-Hearing Association
- Certification
- Publications
- Continuing Education
- Practice Management
- Audiologists
- Speech-Language Pathologists
- Academic & Faculty
- Audiology & SLP Assistants
- Speech Testing
Types of Tests
- Auditory Brainstem Response (ABR)
- Otoacoustic Emissions (OAEs)
- Pure-Tone Testing
- Tests of the Middle Ear
About Speech Testing
An audiologist may do a number of tests to check your hearing. Speech testing will look at how well you listen to and repeat words. One test is the speech reception threshold, or SRT.
The SRT is for older children and adults who can talk. The results are compared to pure-tone test results to help identify hearing loss.
How Speech Testing Is Done
The audiologist will say words to you through headphones, and you will repeat the words. The audiologist will record the softest speech you can repeat. You may also need to repeat words that you hear at a louder level. This is done to test word recognition.
Speech testing may happen in a quiet or noisy place. People who have hearing loss often say that they have the most trouble hearing in noisy places. So, it is helpful to test how well you hear in noise.
Learn more about hearing testing .
To find an audiologist near you, visit ProFind .
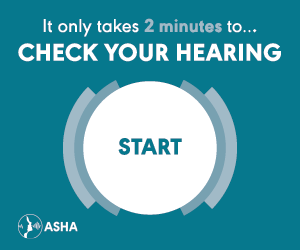
In the Public Section
- Hearing & Balance
- Speech, Language & Swallowing
- About Health Insurance
- Adding Speech & Hearing Benefits
- Advocacy & Outreach
- Find a Professional
- Advertising Disclaimer
- Advertise with us
ASHA Corporate Partners
- Become A Corporate Partner
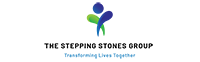
The American Speech-Language-Hearing Association (ASHA) is the national professional, scientific, and credentialing association for 234,000 members, certificate holders, and affiliates who are audiologists; speech-language pathologists; speech, language, and hearing scientists; audiology and speech-language pathology assistants; and students.
- All ASHA Websites
- Work at ASHA
- Marketing Solutions
Information For
Get involved.
- ASHA Community
- Become a Mentor
- Become a Volunteer
- Special Interest Groups (SIGs)
Connect With ASHA
American Speech-Language-Hearing Association 2200 Research Blvd., Rockville, MD 20850 Members: 800-498-2071 Non-Member: 800-638-8255
MORE WAYS TO CONNECT
Media Resources
- Press Queries
Site Help | A–Z Topic Index | Privacy Statement | Terms of Use © 1997- American Speech-Language-Hearing Association
- AudioStar Pro
- TympStar Pro
- Video Library
- 60 Minute Courses
- 30 Minute Courses
- Testing Guides
- All Content
- Get a Quote
- Select Language
Speech Audiometry
Audiometry guides, introduction.
Speech audiometry is an important component of a comprehensive hearing evaluation. There are several kinds of speech audiometry, but the most common uses are to 1) verify the pure tone thresholds 2) determine speech understanding and 3) determine most comfortable and uncomfortable listening levels. The results are used with the other tests to develop a diagnosis and treatment plan.
SDT = Speech Detection Threshold, SAT = Speech Awareness Threshold. These terms are interchangeable and they describe the lowest level at which a patient can hear the presence of speech 50% of the time. They specifically refer to the speech being AUDIBLE, not INTELLIGIBLE.
This test is performed by presenting spondee (two-syllable) words such as baseball, ice cream, hotdog and the patient is to respond when they hear the speech. This is often used with non-verbal patients such as infants or other difficult to test populations. The thresholds should correspond to the PTA and is used to verify the pure tone threshold testing.
How to Test:
Instruct the patient that he or she will be hearing words that have two parts, such as “mushroom” or “baseball.” The patient should repeat the words and if not sure, he or she should not be afraid to guess.
Using either live voice or recorded speech, present the spondee word lists testing the better ear first. Start 20 dB above the 1000 Hz pure tone threshold level. Present one word on the list and, if the response is correct, lower the level by 5 dB. Continue until the patient has difficulty with the words. When this occurs, present more words for each 5 dB step.
Speech Reception Threshold (SRT)
SRT, or speech reception threshold, is a fast way to help verify that the pure tone thresholds are valid. Common compound words - or spondee words - are presented at varying degrees of loudness until it is too soft for the patient to hear. SRT scores are compared to the pure tone average as part of the cross check principle. When these two values agree, the reliability of testing is improved.
Word Recognition
Instruct the patient that he or she is to repeat the words presented. Using either live voice or recorded speech, present the standardized PB word list of your choice. Present the words at a level comfortable to the patient; at least 30 dB and generally 35 to 50 dB above the 1000 Hz pure tone threshold. Using the scorer buttons on the front panel, press the “Correct” button each time the right response is given and the “Incorrect” button each time a wrong response is given.
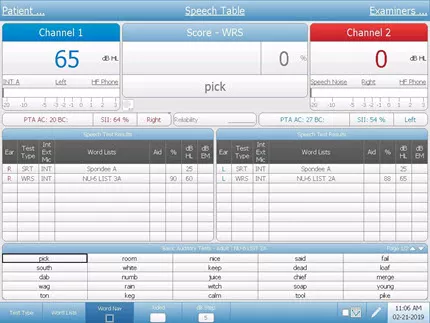
The Discrimination Score is the percentage of words repeated correctly: Discrimination % at HL = 100 x Number of Correct Responses/Number of Trials.
WRS = Word Recognition Score, SRS = Speech Reception Score, Speech Discrimination Score. These terms are interchangeable and describe the patient’s capability to correctly repeat a list of phonetically balanced (PB) words at a comfortable level. The score is a percentage of correct responses and indicates the patient’s ability to understand speech.
Word Recognition Score (WRS)
WRS, or word recognition score, is a type of speech audiometry that is designed to measure speech understanding. Sometimes it is called word discrimination. The words used are common and phonetically balanced and typically presented at a level that is comfortable for the patient. The results of WRS can be used to help set realistic expectations and formulate a treatment plan.
Speech In Noise Test
Speech in noise testing is a critical component to a comprehensive hearing evaluation. When you test a patient's ability to understand speech in a "real world setting" like background noise, the results influence the diagnosis, the recommendations, and the patient's understanding of their own hearing loss.
Auditory Processing
Sometimes, a patient's brain has trouble making sense of auditory information. This is called an auditory processing disorder. It's not always clear that this lack of understanding is a hearing issue, so it requires a very specialized battery of speech tests to identify what kind of processing disorder exists and develop recommendations to improve the listening and understanding for the patient.
QuickSIN is a quick sentence in noise test that quantifies how a patient hears in noise. The patient repeats sentences that are embedded in different levels of restaurant noise and the result is an SNR loss - or Signal To Noise ratio loss. Taking a few additional minutes to measure the SNR loss of every patient seen in your clinic provides valuable insights on the overall status of the patient' s auditory system and allows you to counsel more effectively about communication in real-world situations. Using the Quick SIN to make important decisions about hearing loss treatment and rehabilitation is a key differentiator for clinicians who strive to provide patient-centered care.
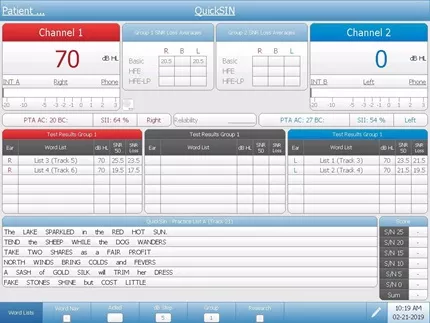
BKB-SIN is a sentence in noise test that quantifies how patients hear in noise. The patient repeats sentences that are embedded in different levels of restaurant noise an the result is an SNR loss - or signal to noise ratio loss. This test is designed to evaluate patients of many ages and has normative corrections for children and adults. Taking a few additional minutes to measure the SNR loss of every patient seen in your clinic is a key differentiator for clinicians who strive to provide patient-centered care.
- Education >
- Testing Guides >
- Speech Audiometry >
GRASON-STADLER
- Our Approach
- Cookie Policy
- AMTAS Pro
Corporate Headquarters 10395 West 70th St. Eden Prairie, MN 55344
General Inquires +1 800-700-2282 +1 952-278-4402 [email protected] (US) [email protected] (International)
Technical Support Hardware: +1 877-722-4490 Software: +1 952-278-4456
DISTRIBUTOR LOGIN
- GSI Extranet
- Request an Account
- Forgot Password?
Get Connected
- Distributor Locator
© Copyright 2024
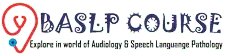
Speech Reception Thresholds – Procedure and Application
Speech Reception Thresholds – Procedure and Application: The speech reception threshold is the minimum hearing level for speech (ANSI, 2010) at which an individual can recognize 50% of the speech material. Speech reception thresholds are achieved in each ear. The term speech reception threshold is synonymous with speech recognition threshold.
Purpose of Speech Reception Thresholds:
- To validate the thresholds obtained through PTA
- To serve as a reference point for supra-threshold tests
- To ascertain the need for aural (re)-habilitation and monitor its progress
- To determine hearing sensitivity in difficult to test population
Materials for Speech Reception Thresholds:
- Spondaic words are the usual and recommended test material for the SRT test. They are 2-syllable words that have equal stress on both syllables.
- Word familiarization can be done prior to the start of test. This ensures that the client is familiar with the test vocabulary, and the client’s responses can be accurately interpreted by the clinician. Care to be taken to eliminate the visual cues during familiarization.
- Based on the circumstances or individuals (age, language facility, physical condition), the standard word list can be modified; however, that the use of speech stimuli with less homogeneity than spondaic words may compromise the reliability of this measure.
- The test material used should be noted in reporting of the results.
Response Format / Mode of Speech Reception Thresholds:
- The usual response mode for obtaining the SRT is repetition of the stimulus item.
- For many patients it is not possible to obtain verbal responses, necessitating the use of alternative response modes such as writing down the responses or closed set of choices such as picture pointing, signing, or visual scanning etc.
- If picture pointing mode is to be used, then the clinician should be cautious in choosing the number of response items (e.g., between 6 and 12 words usually is appropriate).
Procedure of Speech Reception Thresholds:
There are different methods to obtain SRT – ascending or descending method.
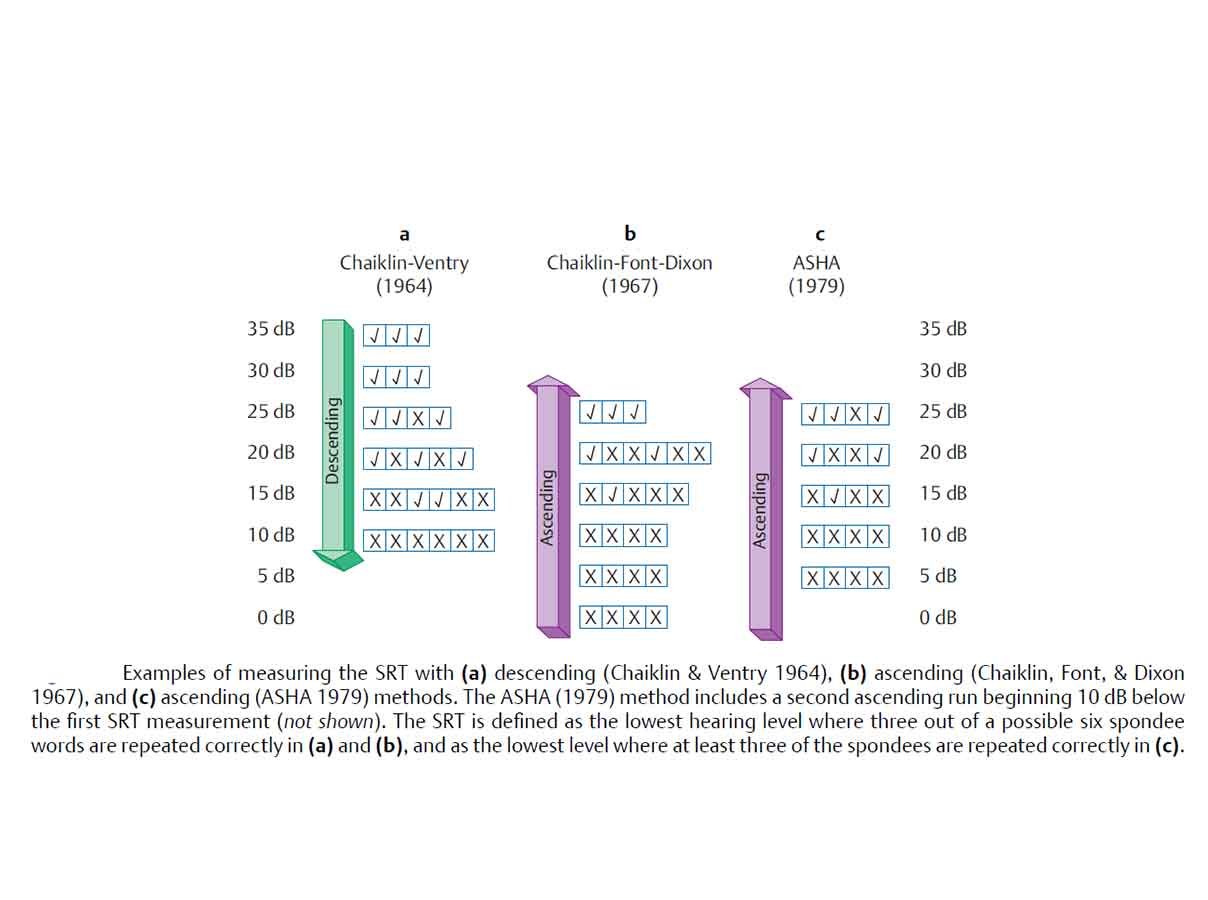
Generally, descending method (ASHA, 1988) is preferred and is described below.
- Obtain pure tone average (PTA) .
- Starting level for SRT: 30-40dB above anticipated SRT or 20 dBSL (with reference to PTA).
- Present one spondee at a time at this level. Decrease in 10 dB decrements, whenever the client response is correct. The 10 dB decrement continues until one word is missed/ until the client responds incorrectly.
- Now present a second spondaic word at the same level that the client responded incorrectly.
- If the second word is correctly identified by the client, the level is attenuated by 10 dB and two spondees are presented. This process is continued until two spondees are incorrectly identified at one level. This is the preliminary phase and the actual test phase begins, which can be performed in 5 dB step (Martin & Sides, 1985).
- If you get response for at least one spondee, reduce the intensity by 5 dB and present 03 spondees at that level.
- Continue the same procedure, until “no response” for all the 03 spondees obtained. Increase by 5 dB and continue it by presenting 03 spondees at each level till you get 2/3 spondees (>50%). That level can be considered as SRT.
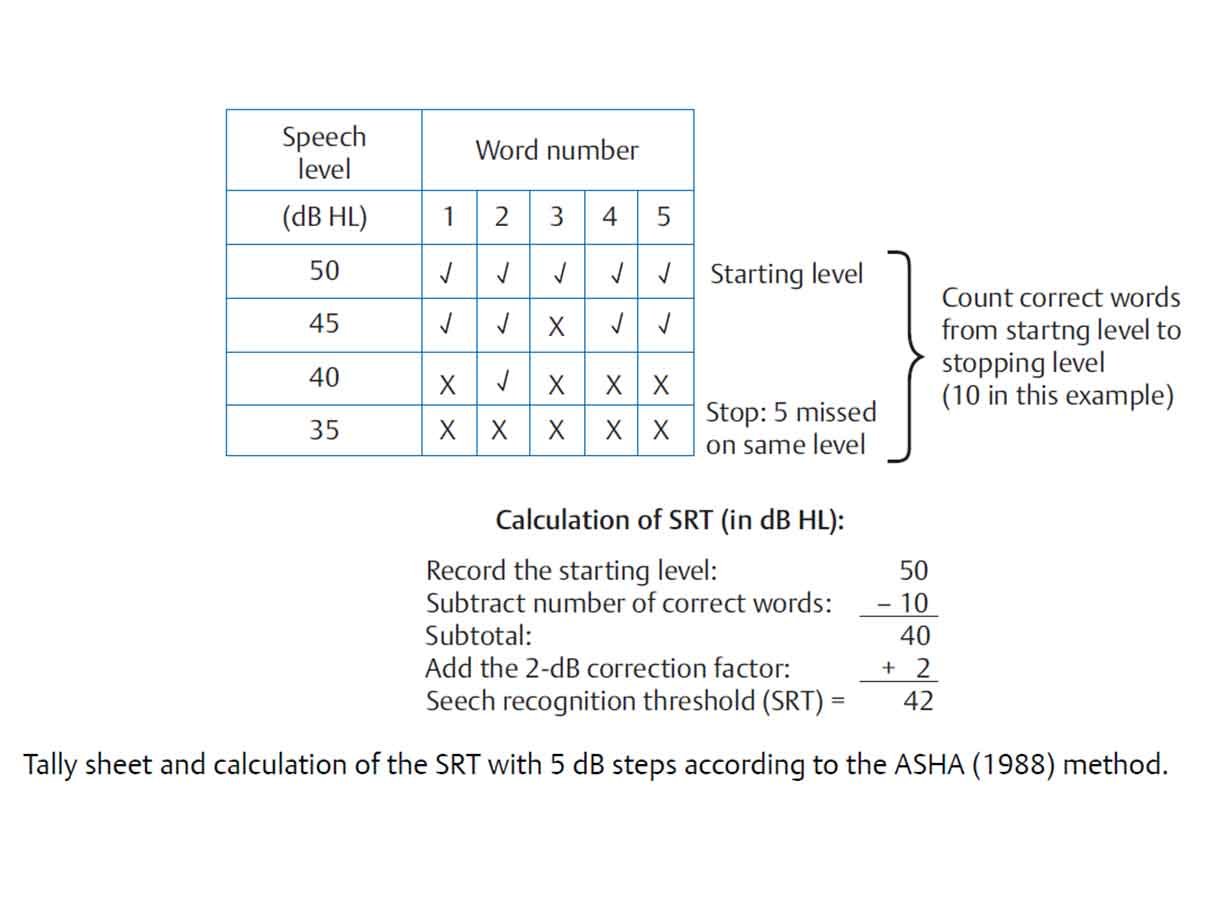
Interpretation of Speech Reception Thresholds:
- The SRT shall be recorded in dB HL. The results should be recorded for each ear on the same form that contains the client’s results for pure tone audiometry. Additional space should be available to report other pertinent information that describes the test situation, such as alternative materials or response modes
- The SRT & PTA correlation are usually within 6 – 12dB.
- If there is disagreement, it could indicate one of the possibilities: misunderstanding of the instructions, functional hearing loss (non-organic) , instrumentation malfunction, pathology along CANS including VIII nerve, cognitive and language difficulties etc. For e.g. the SRT can be poorer than PTA in elderly and auditory processing disorders; whereas the SRT can be better than PTA in cases of malingerers/functional hearing loss .
Masking of Speech Reception Thresholds:
- Masking should be applied to the non-test ear, when the obtained SRT in one ear exceeds the apparent SRT or a pure tone BC threshold at 500, 1000, 2000 or 4000 Hz in the contralateral ear by 40 dB or more.
- The masker used should have a wide band spectrum (white, pink or speech noise) to effectively mask the speech stimuli.
- The level of effective masking used should be sufficient to eliminate reception by the non-test ear without causing over masking and should be recorded on the same form as that used to record audiometric results.
Application of Speech Reception Thresholds:
Speech recognition measures have been used in every phase of audiology, such as
- To describe the extent of hearing impairment in terms of how it affects speech understanding,
- In the differential diagnosis of auditory disorders,
- For determining the needs for amplification and other forms of audiologic rehabilitation,
- For making comparisons between various hearing aids and amplification approaches,
- For verifying the benefits of hearing aid use and other forms of audiologic rehabilitation, and
- For monitoring patient performance over time for either diagnostic or rehabilitative purposes.
References :
⇒ https://www.ishaindia.org.in/pdf/Guidelines-Standard-Audiometric-Screening-Procedures.PDF ⇒ https://www.asha.org/PRPSpecificTopic.aspx?folderid=8589935335§ion=Assessment#Speech_Audiometry ⇒ Essentials of Audiology – Stanley A. Gelfand, PhD (Book)
You are reading about:
Share this:.
- Click to share on Twitter (Opens in new window)
- Click to share on Facebook (Opens in new window)
- Click to share on LinkedIn (Opens in new window)
- Click to share on Telegram (Opens in new window)
- Click to share on WhatsApp (Opens in new window)
- Click to print (Opens in new window)

Written by BASLPCOURSE.COM
June 11, 2020, audiologic testing materials | audiology | baslp 2nd semester audiology notes | baslp 2nd semester audiology unit 4 notes | baslp 2nd semester notes | baslp notes | blog | hearing assessment tests, 0comment(s), follow us on.
For more updates follow us on Facebook, Twitter, Instagram, Youtube and Linkedin
You may also like….
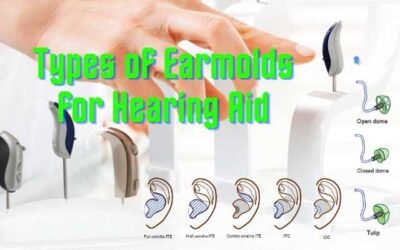
Types of Earmolds for Hearing Aid – Skeleton | Custom
Jan 18, 2024
Types of Earmolds for Hearing Aid - Skeleton | Custom: The realm of hearing aids is a diverse landscape with a myriad...
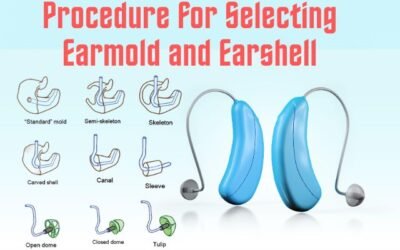
Procedure for Selecting Earmold and Earshell
Jan 17, 2024
Procedure for Selecting Earmold and Earshell: When it comes to optimizing the acoustic performance of hearing aids,...
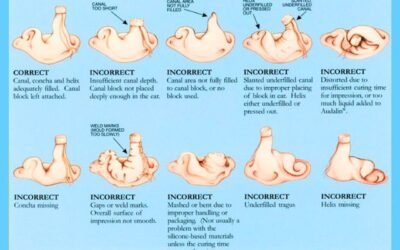
Ear Impression Techniques for Earmolds and Earshells
Jan 16, 2024
Ear Impression Techniques for Earmolds and Earshells: In the realm of audiology and hearing aid fabrication, the Ear...
If you have any Suggestion or Question Please Leave a Reply Cancel reply
- Audiometers
- Tympanometers
- Hearing Aid Fitting
- Research Systems
- Research Unit
- ACT Research
- Our History
- Distributors
- Sustainability
- Environmental Sustainability

Training in Speech Audiometry
- Why Perform Functional Hearing Tests?
- Performing aided speech testing to validate pediatric hearing devices
Speech Audiometry: An Introduction
Description, table of contents, what is speech audiometry, why perform speech audiometry.
- Contraindications and considerations
Audiometers that can perform speech audiometry
How to perform speech audiometry, results interpretation, calibration for speech audiometry.
Speech audiometry is an umbrella term used to describe a collection of audiometric tests using speech as the stimulus. You can perform speech audiometry by presenting speech to the subject in both quiet and in the presence of noise (e.g. speech babble or speech noise). The latter is speech-in-noise testing and is beyond the scope of this article.
Speech audiometry is a core test in the audiologist’s test battery because pure tone audiometry (the primary test of hearing sensitivity) is a limited predictor of a person’s ability to recognize speech. Improving an individual’s access to speech sounds is often the main motivation for fitting them with a hearing aid. Therefore, it is important to understand how a person with hearing loss recognizes or discriminates speech before fitting them with amplification, and speech audiometry provides a method of doing this.
A decrease in hearing sensitivity, as measured by pure tone audiometry, results in greater difficulty understanding speech. However, the literature also shows that two individuals of the same age with similar audiograms can have quite different speech recognition scores. Therefore, by performing speech audiometry, an audiologist can determine how well a person can access speech information.
Acquiring this information is key in the diagnostic process. For instance, it can assist in differentiating between different types of hearing loss. You can also use information from speech audiometry in the (re)habilitation process. For example, the results can guide you toward the appropriate amplification technology, such as directional microphones or remote microphone devices. Speech audiometry can also provide the audiologist with a prediction of how well a subject will hear with their new hearing aids. You can use this information to set realistic expectations and help with other aspects of the counseling process.
Below are some more examples of how you can use the results obtained from speech testing.
Identify need for further testing
Based on the results from speech recognition testing, it may be appropriate to perform further testing to get more information on the nature of the hearing loss. An example could be to perform a TEN test to detect a dead region or to perform the Audible Contrast Threshold (ACT™) test .
Inform amplification decisions
You can use the results from speech audiometry to determine whether binaural amplification is the most appropriate fitting approach or if you should consider alternatives such as CROS aids.
You can use the results obtained through speech audiometry to discuss and manage the amplification expectations of patients and their communication partners.
Unexpected asymmetric speech discrimination, significant roll-over , or particularly poor speech discrimination may warrant further investigation by a medical professional.
Non-organic hearing loss
You can use speech testing to cross-check the results from pure tone audiometry for suspected non‑organic hearing loss.
Contraindications and considerations when performing speech audiometry
Before speech audiometry, it is important that you perform pure tone audiometry and otoscopy. Results from these procedures can reveal contraindications to performing speech audiometry.
Otoscopic findings
Speech testing using headphones or inserts is generally contraindicated when the ear canal is occluded with:
- Foreign body
- Or infective otitis externa
In these situations, you can perform bone conduction speech testing or sound field testing.
Audiometric findings
Speech audiometry can be challenging to perform in subjects with severe-to-profound hearing losses as well as asymmetrical hearing losses where the level of stimulation and/or masking noise required is beyond the limits of the audiometer or the patient's uncomfortable loudness levels (ULLs).
Subject variables
Depending on the age or language ability of the subject, complex words may not be suitable. This is particularly true for young children and adults with learning disabilities or other complex presentations such as dementia and reduced cognitive function.
You should also perform speech audiometry in a language which is native to your patient. Speech recognition testing may not be suitable for patients with expressive speech difficulties. However, in these situations, speech detection testing should be possible.
Before we discuss speech audiometry in more detail, let’s briefly consider the instrumentation to deliver the speech stimuli. As speech audiometry plays a significant role in diagnostic audiometry, many audiometers include – or have the option to include – speech testing capabilities.
Table 1 outlines which audiometers from Interacoustics can perform speech audiometry.
Clinical audiometer | |
Diagnostic audiometer | |
Diagnostic audiometer | |
Equinox 2.0 | PC-based audiometer |
Portable audiometer | |
Hearing aid fitting system | |
Hearing aid fitting system |
Table 1: Audiometers from Interacoustics that can perform speech audiometry.
Because speech audiometry uses speech as the stimulus and languages are different across the globe, the way in which speech audiometry is implemented varies depending on the country where the test is being performed. For the purposes of this article, we will start with addressing how to measure speech in quiet using the international organization of standards ISO 8252-3:2022 as the reference to describe the terminology and processes encompassing speech audiometry. We will describe two tests: speech detection testing and speech recognition testing.
Speech detection testing
In speech detection testing, you ask the subject to identify when they hear speech (not necessarily understand). It is the most basic form of speech testing because understanding is not required. However, it is not commonly performed. In this test, words are normally presented to the ear(s) through headphones (monaural or binaural testing) or through a loudspeaker (binaural testing).
Speech detection threshold (SDT)
Here, the tester will present speech at varying intensity levels and the patient identifies when they can detect speech. The goal is to identify the level at which the patient detects speech in 50% of the trials. This is the speech detection threshold. It is important not to confuse this with the speech discrimination threshold. The speech discrimination threshold looks at a person’s ability to recognize speech and we will explain it later in this article.
The speech detection threshold has been found to correlate well with the pure tone average, which is calculated from pure tone audiometry. Because of this, the main application of speech detection testing in the clinical setting is confirmation of the audiogram.
Speech recognition testing
In speech recognition testing, also known as speech discrimination testing, the subject must not only detect the speech, but also correctly recognize the word or words presented. This is the most popular form of speech testing and provides insights into how a person with hearing loss can discriminate speech in ideal conditions.
Across the globe, the methods of obtaining this information are different and this often leads to confusion about speech recognition testing. Despite there being differences in the way speech recognition testing is performed, there are some core calculations and test parameters which are used globally.
Speech recognition testing: Calculations
There are two main calculations in speech recognition testing.
1. Speech recognition threshold (SRT)
This is the level in dB HL at which the patient recognizes 50% of the test material correctly. This level will differ depending on the test material used. Some references describe the SRT as the speech discrimination threshold or SDT. This can be confusing because the acronym SDT belongs to the speech detection threshold. For this reason, we will not use the term discrimination but instead continue with the term speech recognition threshold.
2. Word recognition score (WRS)
In word recognition testing, you present a list of phonetically balanced words to the subject at a single intensity and ask them to repeat the words they hear. You score if the patient repeats these words correctly or incorrectly. This score, expressed as a percentage of correct words, is calculated by dividing the number of words correctly identified by the total number of words presented.
In some countries, multiple word recognition scores are recorded at various intensities and plotted on a graph. In other countries, a single word recognition score is performed using a level based on the SRT (usually presented 20 to 40 dB louder than the SRT).
Speech recognition testing: Parameters
Before completing a speech recognition test, there are several parameters to consider.
1. Test transducer
You can perform speech recognition testing using air conduction, bone conduction, and speakers in a sound-field setup.
2. Types of words
Speech recognition testing can be performed using a variety of different words or sentences. Some countries use monosyllabic words such as ‘boat’ or ‘cat’ whereas other countries prefer to use spondee words such as ‘baseball’ or ‘cowboy’. These words are then combined with other words to create a phonetically balanced list of words called a word list.
3. Number of words
The number of words in a word list can impact the score. If there are too few words in the list, then there is a risk that not enough data points are acquired to accurately calculate the word recognition score. However, too many words may lead to increased test times and patient fatigue. Word lists often consist of 10 to 25 words.
You can either score words as whole words or by the number of phonemes they contain.
An example of scoring can be illustrated by the word ‘boat’. When scoring using whole words, anything other than the word ‘boat’ would result in an incorrect score.
However, in phoneme scoring, the word ‘boat’ is broken down into its individual phonemes: /b/, /oa/, and /t/. Each phoneme is then scored as a point, meaning that the word boat has a maximum score of 3. An example could be that a patient mishears the word ‘boat’ and reports the word to be ‘float’. With phoneme scoring, 2 points would be awarded for this answer whereas in word scoring, the word float would be marked as incorrect.
5. Delivery of material
Modern audiometers have the functionality of storing word lists digitally onto the hardware of the device so that you can deliver a calibrated speech signal the same way each time you test a patient. This is different from the older methods of testing using live voice or a CD recording of the speech material. Using digitally stored and calibrated speech material in .wav files provides the most reliable and repeatable results as the delivery of the speech is not influenced by the tester.
6. Aided or unaided
You can perform speech recognition testing either aided or unaided. When performing aided measurements, the stimulus is usually played through a loudspeaker and the test is recorded binaurally.
Global examples of how speech recognition testing is performed and reported
Below are examples of how speech recognition testing is performed in the US and the UK. This will show how speech testing varies across the globe.
Speech recognition testing in the US: Speech tables
In the US, the SRT and WRS are usually performed as two separate tests using different word lists for each test. The results are displayed in tables called speech tables.
The SRT is the first speech test which is performed and typically uses spondee words (a word with two equally stressed syllables, such as ‘hotdog’) as the stimulus. During this test, you present spondee words to the patient at different intensities and a bracketing technique establishes the threshold at where the patient correctly identifies 50% of the words.
In the below video, we can see how an SRT is performed using spondee words.
Below, you can see a table showing the results from an SRT test (Figure 1). Here, we can see that the SRT has been measured in each ear. The table shows the intensity at which the SRT was found as well as the transducer, word list, and the level at which masking noise was presented (if applicable). Here we see an unaided SRT of 30 dB HL in both the left and right ears.

Once you have established the intensity of the SRT in dB HL, you can use it to calculate the intensity to present the next list of words to measure the WRS. In WRS testing, it is common to start at an intensity of between 20 dB and 40 dB louder than the speech recognition threshold and to use a different word list from the SRT. The word lists most commonly used in the US for WRS are the NU-6 and CID-W22 word lists.
In word recognition score testing, you present an entire word list to the test subject at a single intensity and score each word based on whether the subject can correctly repeat it or not. The results are reported as a percentage.
The video below demonstrates how to perform the word recognition score.
Below is an image of a speech table showing the word recognition score in the left ear using the NU‑6 word list at an intensity of 55 dB HL (Figure 2). Here we can see that the patient in this example scored 90%, indicating good speech recognition at moderate intensities.
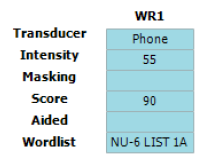
Speech recognition testing in the UK: Speech audiogram
In the UK, speech recognition testing is performed with the goal of obtaining a speech audiogram. A speech audiogram is a graphical representation of how well an individual can discriminate speech across a variety of intensities (Figure 3).

In the UK, the most common method of recording a speech audiogram is to present several different word lists to the subject at varying intensities and calculate multiple word recognition scores. The AB (Arthur Boothroyd) word lists are the most used lists. The initial list is presented around 20 to 30 dB sensation level with subsequent lists performed at quieter intensities before finally increasing the sensation level to determine how well the patient can recognize words at louder intensities.
The speech audiogram is made up of plotting the WRS at each intensity on a graph displaying word recognition score in % as a function of intensity in dB HL. The following video explains how it is performed.
Below is an image of a completed speech audiogram (Figure 4). There are several components.
Point A on the graph shows the intensity in dB HL where the person identified 50% of the speech material correctly. This is the speech recognition threshold or SRT.
Point B on the graph shows the maximum speech recognition score which informs the clinician of the maximum score the subject obtained.
Point C on the graph shows the reference speech recognition curve; this is specific to the test material used (e.g., AB words) and method of presentation (e.g., headphones), and shows a curve which describes the median speech recognition scores at multiple intensities for a group of normal hearing individuals.
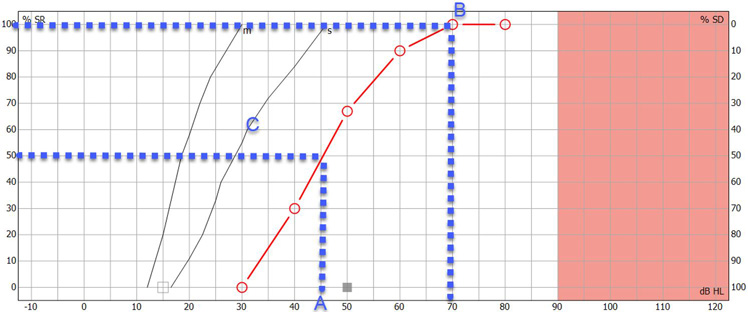
Having this displayed on a single graph can provide a quick and easy way to determine and analyze the ability of the person to hear speech and compare their results to a normative group. Lastly, you can use the speech audiogram to identify roll-over. Roll-over occurs when the speech recognition deteriorates at loud intensities and can be a sign of retro-cochlear hearing loss. We will discuss this further in the interpretation section.
Masking in speech recognition testing
Just like in audiometry, cross hearing can also occur in speech audiometry. Therefore, it is important to mask the non-test ear when testing monaurally. Masking is important because word recognition testing is usually performed at supra-threshold levels. Speech encompasses a wide spectrum of frequencies, so the use of narrowband noise as a masking stimulus is not appropriate, and you need to modify the masking noise for speech audiometry. In speech audiometry, speech noise is typically used to mask the non-test ear.
There are several approaches to calculating required masking noise level. An equation by Coles and Priede (1975) suggests one approach which applies to all types of hearing loss (sensorineural, conductive, and mixed):
- Masking level = D S plus max ABG NT minus 40 plus E M
It considers the following factors.
1. Dial setting
D S is the level of dial setting in dB HL for presentation of speech to the test ear.

2. Air-bone gap
Max ABG NT is the maximum air-bone gap between 250 to 4000 Hz in the non‑test ear.
3. Interaural attenuation
Interaural attenuation: The value of 40 comes from the minimum interaural attenuation for masking in audiometry using headphones (for insert earphones, this would be 55 dB).
4. Effective masking
E M is effective masking. Modern audiometers are calibrated in E M , so you don’t need to include this in the calculation. However, if you are using an old audiometer calibrated to an older calibration standard, then you should calculate the E M .
You can calculate it by measuring the difference in the speech dial setting presented to normal listeners at a level that yields a score of 95% in quiet and the noise dial setting presented to the same ear that yields a score less than 10%.
You can use the results from speech audiometry for many purposes. The below section describes these applications.
1. Cross-check against pure tone audiometry results
The cross-check principle in audiology states that no auditory test result should be accepted and used in the diagnosis of hearing loss until you confirm or cross-check it by one or more independent measures (Hall J. W., 3rd, 2016). Speech-in-quiet testing serves this purpose for the pure tone audiogram.
The following scores and their descriptions identify how well the speech detection threshold and the pure tone average correlate (Table 2).
6 dB or less | Good |
7 to 12 dB | Adequate |
13 dB or more | Poor |
Table 2: Correlation between speech detection threshold and pure tone average.
If there is a poor correlation between the speech detection threshold and the pure tone average, it warrants further investigation to determine the underlying cause or to identify if there was a technical error in the recordings of one of the tests.
2. Detect asymmetries between ears
Another core use of speech audiometry in quiet is to determine the symmetry between the two ears and whether it is appropriate to fit binaural amplification. Significant differences between ears can occur when there are two different etiologies causing hearing loss.
An example of this could be a patient with sensorineural hearing loss who then also contracts unilateral Meniere’s disease . In this example, it would be important to understand if there are significant differences in the word recognition scores between the two ears. If there are significant differences, then it may not be appropriate for you to fit binaural amplification, where other forms of amplification such as contralateral routing of sound (CROS) devices may be more appropriate.
3. Identify if further testing is required
The results from speech audiometry in quiet can identify whether further testing is required. This could be highlighted in several ways.
One example could be a severe difference in the SRT and the pure tone average. Another example could be significant asymmetries between the two ears. Lastly, very poor speech recognition scores in quiet might also be a red flag for further testing.
In these examples, the clinician might decide to perform a test to detect the presence of cochlear dead regions such as the TEN test or an ACT test to get more information.
4. Detect retro-cochlear hearing loss
In subjects with retro-cochlear causes of hearing loss, speech recognition can begin to deteriorate as sounds are made louder. This is called ‘roll-over’ and is calculated by the following equation:
- Roll-over index = (maximum score minus minimum score) divided by maximum score
If roll-over is detected at a certain value (the value is dependent on the word list chosen for testing but is commonly larger than 0.4), then it is considered to be a sign of retro-cochlear pathology. This could then have an influence on the fitting strategy for patients exhibiting these results.
It is important to note however that as the cross-check principle states, you should interpret any roll-over with caution and you should perform additional tests such as acoustic reflexes , the reflex decay test, or auditory brainstem response measurements to confirm the presence of a retro-cochlear lesion.
5. Predict success with amplification
The maximum speech recognition score is a useful measure which you can use to predict whether a person will benefit from hearing aids. More recent, and advanced tests such as the ACT test combined with the Acceptable Noise Level (ANL) test offer good alternatives to predicting hearing success with amplification.
Just like in pure tone audiometry, the stimuli which are presented during speech audiometry require annual calibration by a specialized technician ster. Checking of the transducers of the audiometer to determine if the speech stimulus contains any distortions or level abnormalities should also be performed daily. This process replicates the daily checks a clinicians would do for pure tone audiometry. If speech is being presented using a sound field setup, then you can use a sound level meter to check if the material is being presented at the correct level.
The next level of calibration depends on how the speech material is delivered to the audiometer. Speech material can be presented in many ways including live voice, CD, or installed WAV files on the audiometer. Speech being presented as live voice cannot be calibrated but instead requires the clinician to use the VU meter on the audiometer (which indicates the level of the signal being presented) to determine if they are speaking at the correct intensity. Speech material on a CD requires daily checks and is also performed using the VU meter on the audiometer. Here, a speech calibration tone track on the CD is used, and the VU meter is adjusted accordingly to the desired level as determined by the manufacturer of the speech material.
The most reliable way to deliver a speech stimulus is through a WAV file. By presenting through a WAV file, you can skip the daily tone-based calibration as this method allows you to calibrate the speech material as part of the annual calibration process. This saves the clinician time and ensures the stimulus is calibrated to the same standard as the pure tones in their audiometer. To calibrate the WAV file stimulus, the speech material is calibrated against a speech calibration tone. This is stored on the audiometer. Typically, a 1000 Hz speech tone is used for the calibration and the calibration process is the same as for a 1000 Hz pure tone calibration.
Lastly, if the speech is being presented through the sound field, a calibration professional should perform an annual sound field speaker calibration using an external free field microphone aimed directly at the speaker from the position of the patient’s head.
Coles, R. R., & Priede, V. M. (1975). Masking of the non-test ear in speech audiometry . The Journal of laryngology and otology , 89 (3), 217–226.
Graham, J. Baguley, D. (2009). Ballantyne's Deafness, 7th Edition. Whiley Blackwell.
Hall J. W., 3rd (2016). Crosscheck Principle in Pediatric Audiology Today: A 40-Year Perspective . Journal of audiology & otology , 20 (2), 59–67.
Katz, J. (2009). Handbook of Clinical Audiology. Wolters Kluwer.
Killion, M. C., Niquette, P. A., Gudmundsen, G. I., Revit, L. J., & Banerjee, S. (2004). Development of a quick speech-in-noise test for measuring signal-to-noise ratio loss in normal-hearing and hearing-impaired listeners . The Journal of the Acoustical Society of America , 116 (4), 2395–2405.
Stach, B.A (1998). Clinical Audiology: An Introduction, Cengage Learning.

Popular Academy Advancements
What is nhl-to-ehl correction, getting started: assr, what is the ce-chirp® family of stimuli, nhl-to-ehl correction for abr stimuli.
- Find a distributor
- Customer stories
- Made Magazine
- ABR equipment
- OAE devices
- Hearing aid fitting systems
- Balance testing equipment
Certificates
- Privacy policy
- Cookie Policy
Toggle Menu
Tous droits réservés © NeurOreille (loi sur la propriété intellectuelle 85-660 du 3 juillet 1985). Ce produit ne peut être copié ou utilisé dans un but lucratif.
Journey into the world of hearing
Speech audiometry
Authors: Benjamin Chaix Rebecca Lewis Contributors: Diane Lazard Sam Irving
Facebook Twitter Google+
Speech audiometry is routinely carried out in the clinic. It is complementary to pure tone audiometry, which only gives an indication of absolute perceptual thresholds of tonal sounds (peripheral function), whereas speech audiometry determines speech intelligibility and discrimination (between phonemes). It is of major importance during hearing aid fitting and for diagnosis of certain retrocochlear pathologies (tumour of the auditory nerve, auditory neuropathy, etc.) and tests both peripheral and central systems.
Speech audiogram
Normal hearing and hearing impaired subjects.
The speech recognition threshold (SRT) is the lowest level at which a person can identify a sound from a closed set list of disyllabic words.
The word recognition score (WRS) testrequires a list of single syllable words unknown to the patient to be presented at the speech recognition threshold + 30 dBHL. The number of correct words is scored out of the number of presented words to give the WRS. A score of 85-100% correct is considered normal when pure tone thresholds are normal (A), but it is common for WRS to decrease with increasing sensorineural hearing loss.
The curve 'B', on the other hand, indicates hypoacusis (a slight hearing impairment), and 'C' indicates a profound loss of speech intelligibility with distortion occurring at intensities greater than 80 dB HL.
It is important to distinguish between WRS, which gives an indication of speech comprehension, and SRT, which is the ability to distinguish phonemes.
Phonetic materials and testing conditions
Various tests can be carried out using lists of sentences, monosyllabic or dissyllabic words, or logatomes (words with no meaning, also known as pseudowords). Dissyllabic words require mental substitution (identification by context), the others do not.
A few examples
|
| ||
Laud Boat Pool Nag Limb Shout Sub Vine Dime Goose |
Pick Room Nice Said Fail South White Keep Dead Loaf | Greyhound Schoolboy Inkwell Whitewash Pancake Mousetrap Eardrum Headlight Birthday Duck pond |
The test stimuli can be presented through headphones to test each ear separately, or in freefield in a sound attenuated booth to allow binaural hearing to be tested with and without hearing aids or cochlear implants. Test material is adapted to the individual's age and language ability.
What you need to remember
In the case of a conductive hearing loss:
- the response curve has a normal 'S' shape, there is no deformation
- there is a shift to the right compared to the reference (normal threshold)
- there is an increase in the threshold of intelligibility
In the case of sensorineural hearing loss:
- there is an increased intelligibility threshold
- the curve can appear normal except in the higher intensity regions, where deformations indicate distortions
Phonetic testing is also carried out routinely in the clinic (especially in the case of rehabilitation after cochlear implantation). It is relatively long to carry out, but enables the evaluation of the real social and linguistic handicaps experienced by hearing impaired individuals. Cochlear deficits are tested using the “CNC (Consonant Nucleus Consonant) Test” (short words requiring little mental recruitment - errors are apparent on each phoneme and not over the complete word) and central deficits are tested with speech in noise tests, such as the “HINT (Hearing In Noise Test)” or “QuickSIN (Quick Speech In Noise)” tests, which are sentences carried out in noise.
Speech audiometry generally confirms pure tone audiometry results, and provides insight to the perceptual abilities of the individual. The intelligibility threshold is generally equivalent to the average of the intensity of frequencies 500, 1000 and 2000 Hz, determined by tonal audiometry (conversational frequencies). In the case of mismatch between the results of these tests, the diagnostic test used, equipment calibration or the reliability of the responses should be called into question.
Finally, remember that speech audiometry is a more sensitive indicator than pure tone audiometry in many cases, including rehabilitation after cochlear implantation.
Last update: 16/04/2020 8:57 pm
Connexion | Powered by eZPublish - Ligams
Masks Strongly Recommended but Not Required in Maryland, Starting Immediately
Due to the downward trend in respiratory viruses in Maryland, masking is no longer required but remains strongly recommended in Johns Hopkins Medicine clinical locations in Maryland. Read more .
- Vaccines
- Masking Guidelines
- Visitor Guidelines
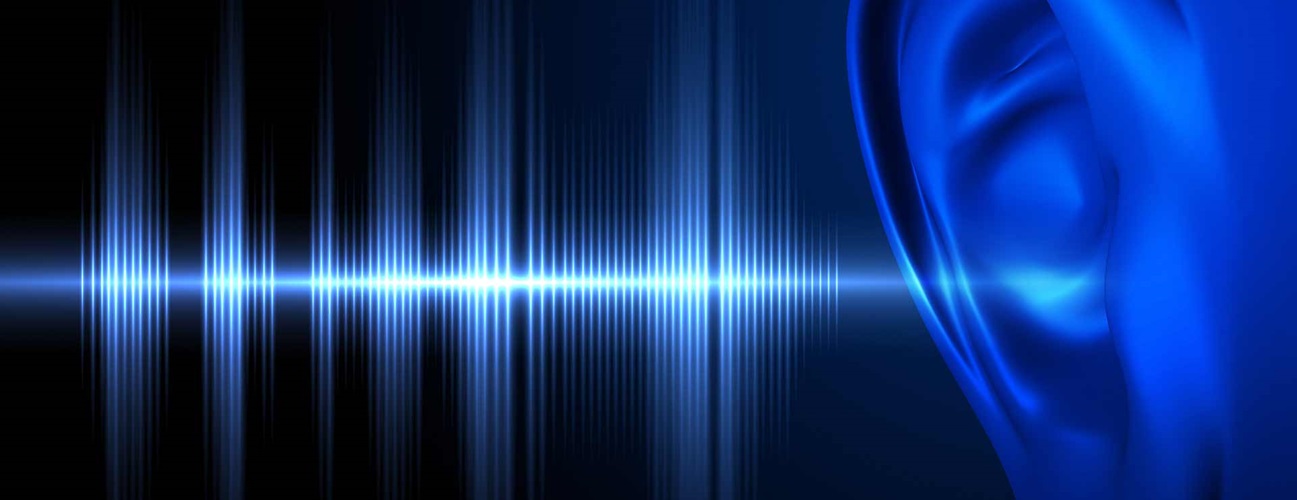
Understanding Your Audiogram
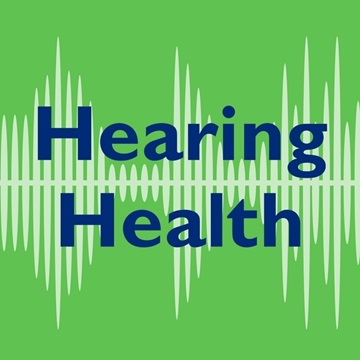
The audiogram is a chart that shows the results of a hearing test. It shows how well you hear sounds in terms of frequency (high-pitched sounds versus low-pitched sounds) and intensity, or loudness. The audiogram shows results for each ear and tells the audiologist the softest sound you can hear at each specific frequency.
Frequency or pitch is measured in Hertz (Hz). Frequencies range from low pitch to high pitch and read from left to right on the audiogram. Each vertical line represents a different frequency, such as 250, 500, 1000, 2000, 4000 and 8000 Hz.
The intensity is measured in decibels (dB). The intensity relates to how loud or soft a sound is. Each horizontal line represents a different intensity level. The softest sounds are at the top of the chart and the loudest sounds at the bottom. Each mark on your audiogram shows the softest sounds you can hear. The softest intensity tested is typically 0 dB and the loudest is 120 dB.
Right Ear vs Left Ear
For the part of the hearing test when you used headphones, results for your right ear appear on the audiogram as either a circle or triangle. The left ear is graphed with an X or a square. These responses represent the air conduction results of either the right or left ear.
Results for the part of the hearing test when you are listening through speakers or in the sound field are marked with “S.” This line on the audiogram represents the response of at least one ear, or the response of the better hearing ear.
Other symbols seen on the audiogram may show responses for the bone conduction testing. The right ear is graphed with < or [, and the left ear with > or ]. These responses can help determine whether a hearing loss is sensorineural or conductive .
Frequently Asked Questions About Hearing Aids
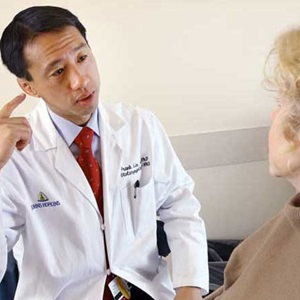
Speech Testing
Part of the speech test involves listening to spoken words through headphones, at a comfortable volume and with no background noise. Speech discrimination or word recognition ability is scored as a percentage and shows how often words need to be repeated for you to recognize them.
Degrees of Hearing Loss
Hearing loss ranges from none to profound, depending on your hearing threshold — the softest a sound was heard at a specific frequency.
Johns Hopkins Audiology
Our team of audiologists provides hearing testing, hearing aid selection and fitting, and implantable hearing devices for people of all ages.
Find a Doctor
Specializing In:
- Sudden Hearing Loss
- Hearing Aids
- Hearing Disorders
- Hearing Loss
- Hearing Restoration
- Cochlear Implantation
- Implantable Hearing Devices
Find a Treatment Center
- Otolaryngology-Head and Neck Surgery
Find Additional Treatment Centers at:
- Howard County Medical Center
- Sibley Memorial Hospital
- Suburban Hospital

Request an Appointment
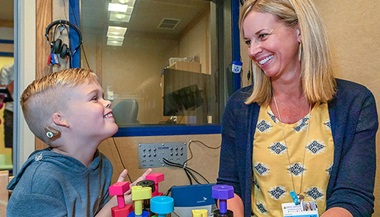
Microtia with Atresia: Theo's Story
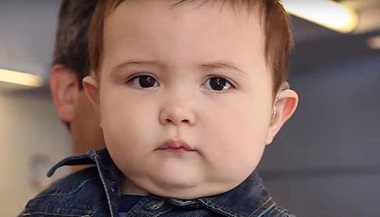
Pediatric Cochlear Implant: Mateo's Story
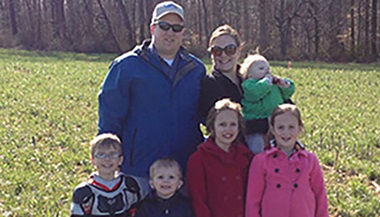
The Gift of Hearing: The Huegel Family Story
Related Topics
- Aging and Hearing
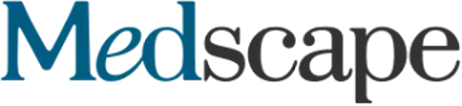
Speech Audiometry
- Author: Suzanne H Kimball, AuD, CCC-A/FAAA; Chief Editor: Arlen D Meyers, MD, MBA more...
- Sections Speech Audiometry
- Indications
- Contraindications
- Pediatric Speech Materials
Speech audiometry has become a fundamental tool in hearing-loss assessment. In conjunction with pure-tone audiometry, it can aid in determining the degree and type of hearing loss. Speech audiometry also provides information regarding discomfort or tolerance to speech stimuli and information on word recognition abilities.
In addition, information gained by speech audiometry can help determine proper gain and maximum output of hearing aids and other amplifying devices for patients with significant hearing losses and help assess how well they hear in noise. Speech audiometry also facilitates audiological rehabilitation management.
The Technique section of this article describes speech audiometry for adult patients. For pediatric patients, see the Pediatric Speech Materials section below.
Speech audiometry can be used for the following:
Assessment of degree and type of hearing loss
Examination of word recognition abilities
Examination of discomfort or tolerance to speech stimuli
Determination of proper gain and maximum output of amplifying devices
Speech audiometry should not be done if the patient is uncooperative.
No anesthesia is required for speech audiometry.
In most circumstances, speech audiometry is performed in a 2-room testing suite. Audiologists work from the audiometric equipment room, while patients undergo testing in the evaluation room. The audiometric equipment room contains the speech audiometer, which is usually part of a diagnostic audiometer. The speech-testing portion of the diagnostic audiometer usually consists of 2 channels that provide various inputs and outputs.
Speech audiometer input devices include microphones (for live voice testing), tape recorders, and CDs for recorded testing. Various output devices, including earphones, ear inserts, bone-conduction vibrators, and loudspeakers, are located in the testing suite. [ 1 ]
Tests using speech materials can be performed using earphones, with test material presented into 1 or both earphones. Testing can also be performed via a bone-conduction vibrator. In addition to these methods, speech material can be presented using loudspeakers in the sound-field environment.
Speech-awareness thresholds
Speech-awareness threshold (SAT) is also known as speech-detection threshold (SDT). The objective of this measurement is to obtain the lowest level at which speech can be detected at least half the time. This test does not have patients repeat words; it requires patients to merely indicate when speech stimuli are present.
Speech materials usually used to determine this measurement are spondees. Spondaic words are 2-syllable words spoken with equal emphasis on each syllable (eg, pancake, hardware, playground). Spondees are used because they are easily understandable and contain information within each syllable sufficient to allow reasonably accurate guessing.
The SAT is especially useful for patients too young to understand or repeat words. It may be the only behavioral measurement that can be made with this population. The SAT may also be used for patients who speak another language or who have impaired language function because of neurological insult.
For patients with normal hearing or somewhat flat hearing loss, this measure is usually 10-15 dB better than the speech-recognition threshold (SRT) that requires patients to repeat presented words. For patients with sloping hearing loss, this measurement can be misleading with regard to identifying the overall degree of loss.
If a patient has normal hearing in a low frequency, the SAT will be closely related to the threshold for that frequency, and it will not indicate greater loss in higher frequencies.
Speech-recognition threshold
The speech-recognition threshold (SRT) is sometimes referred to as the speech-reception threshold. [ 2 ] The objective of this measure is to obtain the lowest level at which speech can be identified at least half the time.
Spondees are usually used for this measurement. Lists of spondaic words commonly used to obtain the SRT are contained within the Central Institute for the Deaf (CID) Auditory List W-1 and W-2.
In addition to determining softest levels at which patients can hear and repeat words, the SRT is also used to validate pure-tone thresholds because of high correlation between the SRT and the average of pure-tone thresholds at 500, 1000, and 2000 Hz.
In clinical practice, the SRT and 3-frequency average should be within 5-12 dB. This correlation holds true if hearing loss in the 3 measured frequencies is relatively similar. If 1 threshold within the 3 frequencies is significantly higher than the others, the SRT will usually be considerably better than the 3-frequency average. In this case, a 2-frequency average is likely to be calculated and assessed for agreement with the SRT.
Other clinical uses of the SRT include establishing the sound level to present suprathreshold measures and determining appropriate gain during hearing aid selection.
Suprathreshold word-recognition testing
The primary purpose of suprathreshold word-recognition testing is to estimate ability to understand and repeat single-syllable words presented at conversational or another suprathreshold level. This type of testing is also referred to as word-discrimination testing or speech-discrimination testing.
Initial word lists compiled for word-recognition testing were phonetically balanced (PB). This term indicated that phonetic composition of the lists was equivalent and representative of connected English discourse.
The original PB lists were created at the Harvard Psycho-Acoustic Laboratory and are referred to as the PB-50 lists. The PB-50 lists contain 50 single-syllable words in 20 lists consisting of 1000 different monosyllabic words. Several years later, the CID W-22 word lists were devised, primarily using words selected from the PB-50 lists. Another word list (devised from a grouping of 200 consonant-nucleus-consonant [CNC] words) is called the Northwestern University Test No. 6 (NU-6). Recorded tape and CD versions of all these word-recognition tests are commercially available.
The PB-50, CID W-22, and NU-6 word lists each contain 50 words that are presented at specified sensation levels. Words can be presented via tape, CD, or monitored live voice. Patients are asked to repeat words to the audiologist. Each word repeated correctly is valued at 2%, and scores are tallied as a percent-correct value.
Varying the presentation level of monosyllabic words reveals a variety of performance-intensity functions for these word lists. In general, presenting words at 25-40 dB sensation level (refer to the SRT) allows patients to achieve maximum scores. Lowering the level results in lower scores. For individuals with hearing loss, words can be presented at a comfortable loudness level or at the highest reasonable level before discomfort occurs.
When words are presented at the highest reasonable level and the word-recognition score is 80% or better, testing can be discontinued. If the score is lower than 80%, further testing at lower presentation levels is recommended. If scores at lower levels are better than those obtained at higher presentation levels, "roll over" has occurred, and these scores indicate a possible retrocochlear (or higher) site of lesion.
Another use of suprathreshold word-recognition testing is to verify speech-recognition improvements achieved by persons with hearing aids . Testing can be completed at conversational levels in the sound field without the use of hearing aids and then again with hearing aids fitted to the patient. Score differences can be used as a method to assess hearing with hearing aids and can be used as a pretest and posttest to provide a percent-improvement score
Sentence testing
To evaluate ability to hear and understand everyday speech, various tests have been developed that use sentences as test items. Sentences can provide information regarding the time domain of everyday speech and can approximate contextual characteristics of conversational speech.
Everyday sentence test
This is the first sentence test developed at the CID in the 1950s.
Clinical use of this test is limited, because its reliability as a speech-recognition test for sentences remains undemonstrated.
Synthetic-sentence identification test
The synthetic-sentence identification (SSI) test was developed in the late 1960s. SSI involves a set of 10 synthetic sentences. Sentences used in this test were constructed so that each successive group of 3 words in a sentence is itself meaningful but the entire sentence is not.
Because the sentences are deemed insufficiently challenging in quiet environments, a recommendation has been made that sentences be administered in noise at a signal-to-noise (S/N) ratio of 0 dB, which presents both sentences and noise at equal intensity level.
Speech perception in noise test
The speech perception in noise (SPIN) test is another sentence-identification test. The SPIN test was originally developed in the late 1970s and was revised in the mid 1980s.
The revised SPIN test consists of 8 lists of 50 sentences. The last word of each sentence is considered the test item. Half of listed sentences contain test items classified as having high predictability, indicating that the word is very predictable given the sentence context. The other half of listed sentences contain test items classified as having low predictability, indicating that the word is not predictable given sentence context. Recorded sentences come with a speech babble-type noise that can be presented at various S/N ratios.
Speech in noise test
The speech in noise (SIN) test, developed in the late 1990s, contains 5 sentences with 5 key words per test condition. Two signal levels (70 and 40 dB) and 4 S/N ratios are used at each level. A 4-talker babble is used as noise. This recorded test can be given to patients with hearing aids in both the unaided and aided conditions.
Results are presented as performance-intensity functions in noise. A shorter version of the SIN, the QuickSIN, was developed in 2004. The QuickSIN has been shown to be effective, particularly when verifying open-fit behind-the-ear hearing aids.
Hearing in noise test
The hearing in noise test (HINT) is designed to measure speech recognition thresholds in both quiet and noise. The test consists of 25 lists of 10 sentences and noise matched to long-term average speech.
Using an adaptive procedure, a reception threshold for sentences is obtained while noise is presented at a constant level. Results can be compared with normative data to determine the patient's relative ability to hear in noise.
Words in noise test
The Words-in-Noise Test (WIN), developed in the early 2000s, provides an open set word-recognition task without linguistic context. The test is composed of monosyllabic words from the NU-6 word lists presented in multitalker babble. The purpose of the test is to determine the signal-to-babble (S/B) ratio in decibels for those with normal and impaired hearing. The WIN is similar to the QuickSIN in providing information about speech recognition performance.
The WIN is used to measure performance of basic auditory function when working memory and linguistic context is reduced or eliminated. This measure, by using monosyllabic words in isolation, evaluates the listener's ability to recognize speech using acoustic cues alone and by eliminating syntactical and semantic cues founds in sentences. The WIN materials allow for the same words to be spoken by the same speaker for both speech-in-quiet and speech-in-noise data collection.
Bamford-Kowal-Bench speech in noise test
Bamford-Kowal-Bench Speech-in-Noise Test (BKB-SIN) was developed by Etymotic Research in the early to mid 2000s. The primary population for this test include children and candidates or recipients of cochlear implants .
Like the HINT, the BKB-SIN uses Americanized BKB sentences. [ 3 ] These words are characterized as short, and the sentences are highly redundant; they contain semantic and syntactic contextual cues developed at a first grade reading level. Compared to the HINT, which uses speech-spectrum noise, the BKB-SIN uses multitalker babble. Clinicians can expect better recognition performance on the BKB-SIN and HINT in comparison to the QuickSIN and WIN because of the additional semantic context provided by the BKB sentences.
Selecting proper speech in noise testing
QuickSIN and WIN materials are best for use in discriminating those who have hearing loss from normal hearing individuals. The BKB-SIN and HINT materials are less able to identify those with hearing loss. [ 4 , 5 ] Therefore, the QuickSIN or WIN is indicated as part of the routine clinical protocol as a speech in noise task. The choice of QuickSIN or WIN is strictly a matter of clinician preference; however, the clinician must also consider whether or not the patient can handle monosyllabic words (WIN) or needs some support from sentence context (QuickSIN).
The BKB-SIN and HINT materials are easier to recognize because of the semantic content, making them excellent tools for young children or individuals with substantial hearing loss, including cochlear implant candidates and new recipients.
Most comfortable loudness level and uncomfortable loudness level
Most comfortable loudness level
The test that determines the intensity level of speech that is most comfortably loud is called the most comfortable loudness level (MCL) test.
For most patients with normal hearing, speech is most comfortable at 40-50 dB above the SRT. This sensation level is reduced for many patients who have sensorineural hearing loss (SNHL). Because of this variation, MCL can be used to help determine hearing aid gain for patients who are candidates for amplification.
MCL measurement can be obtained using cold running or continuous speech via recorded or monitored live-voice presentation. Patients are instructed to indicate when speech is perceived to be at the MCL. Initial speech levels may be presented at slightly above SRT and then progressively increased until MCL is achieved. Once MCL is achieved, a speech level is presented above initial MCL and reduced until another MCL is obtained. This bracketing technique provides average MCL.
Uncomfortable loudness level
One reason to establish uncomfortable loudness level (UCL) is to determine the upper hearing limit for speech. This level provides the maximum level at which word-recognition tests can be administered. UCL can also indicate maximum tolerable amplification.
Another reason to establish UCL is to determine the dynamic speech range. Dynamic range represents the limits of useful hearing in each ear and is computed by subtracting SRT from UCL. For many patients with SNHL, this range can be extremely limited because of recruitment or abnormal loudness perception.
UCL speech materials can be the same as for MCL. The normal ear should be able to accept hearing levels of 90-100 dB. Patients are instructed to indicate when presented speech is uncomfortably loud. Instructions are critical, since patients must allow speech above MCL before indicating discomfort.
While the use of speech testing in general has not necessarily been shown to predict hearing aid satisfaction, [ 6 ] the use of loudness discomfort levels (UCLs) has been shown to be useful in successful hearing aid outcomes. [ 7 ]
The Acceptable Noise Level (ANL) test is a measure of the amount of background noise that a person is willing to tolerate. [ 8 ] In recent years it has gained interest among researchers and hearing-care professionals because of its ability to predict, with 85% accuracy, who will be successful with hearing aids. [ 9 ]
For very young children with limited expressive and receptive language skills, picture cards representing spondaic words can be used to establish the SRT. Before testing, the tester must ensure that the child understands what the card represents. Once the child has been taught to point to the correct picture card, 4-6 cards are chosen and presented to the child. Then, the softest level at which the child can select the correct card at least half the time is established.
For children with typical kindergarten or first-grade language skills, the Children's Spondee Word List can be used instead of adult word lists. The CID W-1 list is appropriate for use with older children.
Word-recognition testing for children can be classified as open-message response testing or closed-response testing. Closed-response testing uses the picture-pointing technique.
Word intelligibility by picture identification test
One of the more popular closed-response tests is the word intelligibility by picture identification (WIPI) test. This test consists of 25 pages; on each page are 6 colored pictures representing an item named by a monosyllabic word. Four pictures represent a test item, while the other 2 serve to decrease probability of a correct guess.
WIPI was developed for use with children with hearing impairment and can be used for children aged 4 years and older.
Northwestern University children's perception of speech test
Another popular closed-response test is the Northwestern University children's perception of speech (NU-CHIPS) test. NU-CHIPS consists of 50 pages with 4 pictures per page.
This test was developed for use with children aged 3 years and older.
Pediatric speech intelligibility test
The pediatric speech intelligibility (PSI) test uses both monosyllabic words and sentence test items. The PSI test consists of 20 monosyllabic words and 10 sentences. Children point to the appropriate picture representing the word or sentence presented.
Test materials are applicable for children aged as young as 3 years.
Phonetically balanced kindergarten test
One of the more popular open-message response tests for children is the phonetically balanced kindergarten (PBK) test, which contains 50 monosyllabic words that the child repeats.
The PKB test is most appropriate for children aged 5-7 years.
Bamford-Kowal-Bench Speech-in-Noise Test
As mentioned prior, the BKB-SIN materials are easier due to the amount of semantic content utilized which makes it an excellent tool for use with young children. [ 10 ]
Lewis MS, Crandell CC, Valente M, Horn JE. Speech perception in noise: directional microphones versus frequency modulation (FM) systems. J Am Acad Audiol . 2004 Jun. 15(6):426-39. [QxMD MEDLINE Link] .
Harris RW, McPherson DL, Hanson CM, Eggett DL. Psychometrically equivalent bisyllabic words for speech recognition threshold testing in Vietnamese. Int J Audiol . 2017 Aug. 56 (8):525-537. [QxMD MEDLINE Link] .
Bench J, Kowal A, Bamford J. The BKB (Bamford-Kowal-Bench) sentence lists for partially-hearing children. Br J Audiol . 1979 Aug. 13(3):108-12. [QxMD MEDLINE Link] .
Wilson RH, McArdle RA, Smith SL. An Evaluation of the BKB-SIN, HINT, QuickSIN, and WIN Materials on Listeners With Normal Hearing and Listeners With Hearing Loss. J Speech Lang Hear Res . 2007 Aug. 50(4):844-56. [QxMD MEDLINE Link] .
Carlson ML, Sladen DP, Gurgel RK, Tombers NM, Lohse CM, Driscoll CL. Survey of the American Neurotology Society on Cochlear Implantation: Part 1, Candidacy Assessment and Expanding Indications. Otol Neurotol . 2018 Jan. 39 (1):e12-e19. [QxMD MEDLINE Link] .
Killion MC, Gudmundsen GI. Fitting hearing aids using clinical prefitting speech measures: an evidence-based review. J Am Acad Audiol . 2005 Jul-Aug. 16(7):439-47. [QxMD MEDLINE Link] .
Mueller HG, Bentler RA. Fitting hearing aids using clinical measures of loudness discomfort levels: an evidence-based review of effectiveness. J Am Acad Audiol . 2005 Jul-Aug. 16(7):461-72. [QxMD MEDLINE Link] .
Nabelek AK, Tucker FM, Letowski TR. Toleration of background noises: relationship with patterns of hearing aid use by elderly persons. J Speech Hear Res . 1991 Jun. 34 (3):679-85. [QxMD MEDLINE Link] .
Nabelek AK, Freyaldenhoven MC, Tampas JW, Burchfiel SB, Muenchen RA. Acceptable noise level as a predictor of hearing aid use. J Am Acad Audiol . 2006 Oct. 17 (9):626-39. [QxMD MEDLINE Link] .
Neave-DiToro D, Rubinstein A, Neuman AC. Speech Recognition in Nonnative versus Native English-Speaking College Students in a Virtual Classroom. J Am Acad Audiol . 2017 May. 28 (5):404-414. [QxMD MEDLINE Link] .
- Speech audiogram. Video courtesy of Benjamin Daniel Liess, MD.
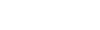
Contributor Information and Disclosures
Suzanne H Kimball, AuD, CCC-A/FAAA Assistant Professor, University of Oklahoma Health Sciences Center Suzanne H Kimball, AuD, CCC-A/FAAA is a member of the following medical societies: American Academy of Audiology , American Speech-Language-Hearing Association Disclosure: Nothing to disclose.
Francisco Talavera, PharmD, PhD Adjunct Assistant Professor, University of Nebraska Medical Center College of Pharmacy; Editor-in-Chief, Medscape Drug Reference Disclosure: Received salary from Medscape for employment. for: Medscape.
Peter S Roland, MD Professor, Department of Neurological Surgery, Professor and Chairman, Department of Otolaryngology-Head and Neck Surgery, Director, Clinical Center for Auditory, Vestibular, and Facial Nerve Disorders, Chief of Pediatric Otology, University of Texas Southwestern Medical Center; Chief of Pediatric Otology, Children’s Medical Center of Dallas; President of Medical Staff, Parkland Memorial Hospital; Adjunct Professor of Communicative Disorders, School of Behavioral and Brain Sciences, Chief of Medical Service, Callier Center for Communicative Disorders, University of Texas School of Human Development Peter S Roland, MD is a member of the following medical societies: Alpha Omega Alpha , American Academy of Otolaryngic Allergy , American Academy of Otolaryngology-Head and Neck Surgery , American Auditory Society , American Neurotology Society , American Otological Society , North American Skull Base Society , Society of University Otolaryngologists-Head and Neck Surgeons , The Triological Society Disclosure: Received honoraria from Alcon Labs for consulting; Received honoraria from Advanced Bionics for board membership; Received honoraria from Cochlear Corp for board membership; Received travel grants from Med El Corp for consulting.
Arlen D Meyers, MD, MBA Emeritus Professor of Otolaryngology, Dentistry, and Engineering, University of Colorado School of Medicine Arlen D Meyers, MD, MBA is a member of the following medical societies: American Academy of Facial Plastic and Reconstructive Surgery , American Academy of Otolaryngology-Head and Neck Surgery , American Head and Neck Society Disclosure: Serve(d) as a director, officer, partner, employee, advisor, consultant or trustee for: Cerescan; Neosoma; MI10;<br/>Received income in an amount equal to or greater than $250 from: Neosoma; Cyberionix (CYBX)<br/>Received ownership interest from Cerescan for consulting for: Neosoma, MI10 advisor.
Cliff A Megerian, MD, FACS Medical Director of Adult and Pediatric Cochlear Implant Program, Director of Otology and Neurotology, University Hospitals of Cleveland; Chairman of Otolaryngology-Head and Neck Surgery, Professor of Otolaryngology-Head and Neck Surgery and Neurological Surgery, Case Western Reserve University School of Medicine Cliff A Megerian, MD, FACS is a member of the following medical societies: American Academy of Otolaryngology-Head and Neck Surgery , American College of Surgeons , American Neurotology Society , American Otological Society , Association for Research in Otolaryngology , Massachusetts Medical Society , Society for Neuroscience , Society of University Otolaryngologists-Head and Neck Surgeons , Triological Society Disclosure: Nothing to disclose.
Medscape Reference thanks Benjamin Daniel Liess, MD, Assistant Professor, Department of Otolaryngology, University of Missouri-Columbia School of Medicine, for the video contributions to this article.
What would you like to print?
- Print this section
- Print the entire contents of
- Print the entire contents of article
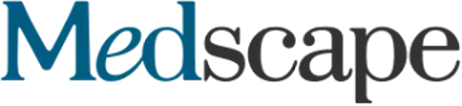
- Skill Checkup: Peritonsillar Abscess Drainage
- Biologic Therapies in Refractory Chronic Rhinosinusitis With Nasal Polyps
- Otolaryngologic Manifestations of Granulomatosis With Polyangiitis
- Pierre Robin Syndrome
- Fast Five Quiz: How Much Do You Know About Bell Palsy?
- Common Comorbidities of Chronic Rhinosinusitis With Nasal Polyps
- Targeted Treatment in Chronic Rhinosinusitis With Nasal Polyps
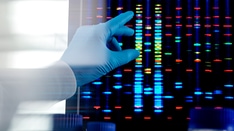
- Drug Interaction Checker
- Pill Identifier
- Calculators
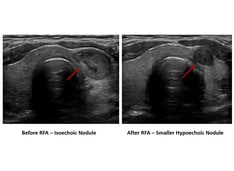
- 2001/viewarticle/980727 Private Equity Firms Increasing Purchases of Otolaryngology Practices

- 2001/viewarticle/1001142 Primary Care Focus: Obstructive Sleep Apnea-Hypopnea Syndrome
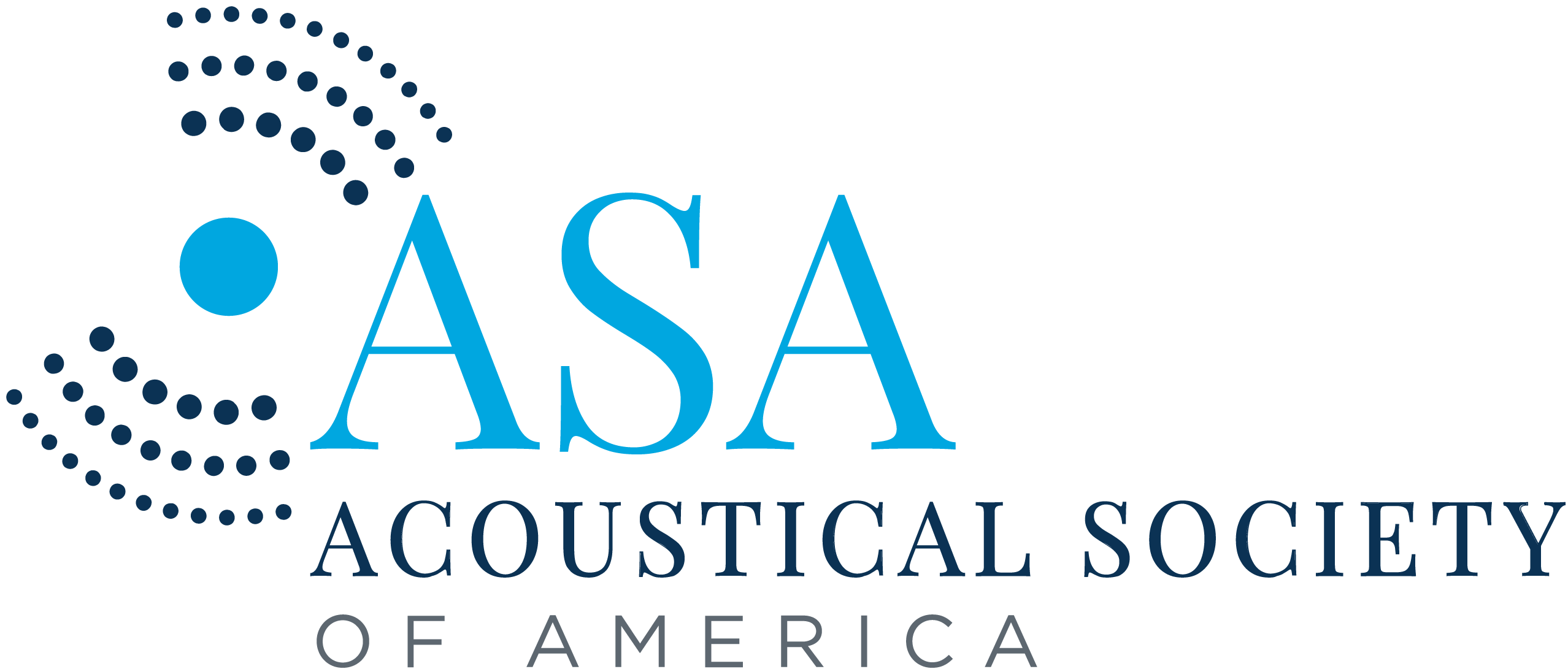
The interpretation of speech reception threshold data in normal-hearing and hearing-impaired listeners: Steady-state noise
Author to whom correspondence should be addressed. Electronic mail: [email protected]
- Article contents
- Figures & tables
- Supplementary Data
- Peer Review
- Reprints and Permissions
- Cite Icon Cite
- Search Site
Cas Smits , Joost M. Festen; The interpretation of speech reception threshold data in normal-hearing and hearing-impaired listeners: Steady-state noise. J. Acoust. Soc. Am. 1 November 2011; 130 (5): 2987–2998. https://doi.org/10.1121/1.3644909
Download citation file:
- Ris (Zotero)
- Reference Manager
Speech-in-noise-measurements are important in clinical practice and have been the subject of research for a long time. The results of these measurements are often described in terms of the speech reception threshold (SRT) and SNR loss. Using the basic concepts that underlie several models of speech recognition in steady-state noise, the present study shows that these measures are ill-defined, most importantly because the slope of the speech recognition functions for hearing-impaired listeners always decreases with hearing loss. This slope can be determined from the slope of the normal-hearing speech recognition function when the SRT for the hearing-impaired listener is known. The SII-function (i.e., the speech intelligibility index (SII) against SNR) is important and provides insights into many potential pitfalls when interpreting SRT data. Standardized SNR loss, sSNR loss, is introduced as a universal measure of hearing loss for speech in steady-state noise. Experimental data demonstrates that, unlike the SRT or SNR loss, sSNR loss is invariant to the target point chosen, the scoring method or the type of speech material.
Citing articles via
- Online ISSN 1520-8524
- Print ISSN 0001-4966
- For Researchers
- For Librarians
- For Advertisers
- Our Publishing Partners
- Physics Today
- Conference Proceedings
- Special Topics
pubs.aip.org
- Privacy Policy
- Terms of Use
Connect with AIP Publishing
This feature is available to subscribers only.
Sign In or Create an Account

An official website of the United States government
The .gov means it’s official. Federal government websites often end in .gov or .mil. Before sharing sensitive information, make sure you’re on a federal government site.
The site is secure. The https:// ensures that you are connecting to the official website and that any information you provide is encrypted and transmitted securely.
- Publications
- Account settings
Preview improvements coming to the PMC website in October 2024. Learn More or Try it out now .
- Advanced Search
- Journal List
- Trends Hear
- v.23; Jan-Dec 2019
Efficient Adaptive Speech Reception Threshold Measurements Using Stochastic Approximation Algorithms
This study examines whether speech-in-noise tests that use adaptive procedures to assess a speech reception threshold in noise ( SRT50n ) can be optimized using stochastic approximation (SA) methods, especially in cochlear-implant (CI) users. A simulation model was developed that simulates intelligibility scores for words from sentences in noise for both CI users and normal-hearing (NH) listeners. The model was used in Monte Carlo simulations. Four different SA algorithms were optimized for use in both groups and compared with clinically used adaptive procedures. The simulation model proved to be valid, as its results agreed very well with existing experimental data. The four optimized SA algorithms all provided an efficient estimation of the SRT50n . They were equally accurate and produced smaller standard deviations (SDs) than the clinical procedures. In CI users, SRT50n estimates had a small bias and larger SDs than in NH listeners. At least 20 sentences per condition and an initial signal-to-noise ratio below the real SRT50n were required to ensure sufficient reliability. In CI users, bias and SD became unacceptably large for a maximum speech intelligibility score in quiet below 70%. In conclusion, SA algorithms with word scoring in adaptive speech-in-noise tests are applicable to various listeners, from CI users to NH listeners. In CI users, they lead to efficient estimation of the SRT50n as long as speech intelligibility in quiet is greater than 70%. SA procedures can be considered as a valid, more efficient, and alternative to clinical adaptive procedures currently used in CI users.
Many cochlear-implant (CI) recipients and hearing-impaired people experience difficulties with understanding speech in a noisy environment. To characterize a subjects’ ability to listen in noise, speech-in-noise tests have been developed in many languages. For clinical use of a test, it is important that the test is accurate in the sense that the test should have a small test–retest variance and bias. With an accurate test, a clinician is able to measure differences between amplification and signal processing settings. Furthermore, the test should be efficient to be applicable in a busy clinic and to prevent fatigue. Efficiency here means that a sufficient accuracy is reached within a limited number of trials.
A frequently used measure of speech perception in noise is the speech reception threshold in noise ( SRT50n ), defined by the signal-to-noise ratio (SNR) that yields an average response of 50% correctly recognized items over a number of trials ( Plomp & Mimpen, 1979 ). This SRT50n can be measured with an adaptive procedure that varies the SNR based on previous responses of the listener to track the 50% score. The SNR and the percent correct score are related by a psychometric curve, which is often referred to as the intelligibility function. The slope of this curve is steepest around the 50% correct score in normal-hearing (NH) listeners. The adaptive procedure keeps the trials in this steep part of the curve and avoids potential floor and ceiling effects. In general, tests of sentence recognition in steady-state speech-spectrum noise have intelligibility functions with steep slopes, giving the advantage that the SRT50n estimate is accurate, because the test–retest variance is inversely related to the slope (e.g., Kollmeier et al., 2015 ). The slope of the intelligibility function is often increased by optimizing the homogeneity of the sentences with respect to their SRT50n and slope.
For CI users, speech-in-noise tests may not be optimally designed. First, the just-mentioned optimization of the homogeneity of the sentences is usually done in a group of NH listeners, and it is unknown whether this homogeneity also applies to CI users. Second, the slope is often less steep in CI recipients. Dingemanse and Goedegebure (2015) found an average slope of 6.4%/dB around 50% for CI recipients, which is much lower than the typical slope of 10%/dB to 15%/dB obtained with NH listeners (e.g., Versfeld et al., 2000 ). However, the step sizes used in adaptive speech tests are often the same in CI recipients as in NH listeners (e.g., Chan et al., 2008 ; Dawson et al., 2011 ; Zhang et al., 2010 ), which may result in different step size to slope ratios for CI recipients compared with NH listeners. This can reduce the accuracy of the adaptive procedure. Third, the maximum proportion correct score (measured in quiet) is lowered and may range from 1 to 0.1 (e.g., Gifford et al., 2008 ), making the proportion correct score of 0.5 no longer the point with the steepest slope. Consequently, the accuracy of the SRT50n measure may be insufficient for CI listeners, or an adaptive estimation of the SRT50n is not even feasible if the maximum proportion correct score of a CI listener approaches 0.5. Given these concerns, there is a need to address the accuracy of SRT50n measures in CI listeners and to explore if SRT50n measurements need special procedures in CI listeners to enhance accuracy.
Several researchers have attempted to modify the simple up-down procedure for use in CI recipients because of their reduced speech intelligibility. The Hearing in Noise Test procedure was modified by allowing one or more errors in repeating a sentence ( Chan et al., 2008 ) or allowing a maximum error of 20%, 40%, or 60% ( Wong & Keung, 2013 ). Wong and Keung showed that adaptive procedures based on these criteria could be used in a greater percentage of CI users. These modifications of the scoring may improve the accuracy because of the increase in maximum proportion correct score and the slope at SRT50n .
Another well-known option to enhance the accuracy of the SRT50n estimate is to score the correctly repeated sentence elements (often words, so-called word scoring; Brand & Kollmeier, 2002 ; Terband & Drullman, 2008 ). The test–retest reliability is inversely proportional to the square root of the number of sentences and for word scoring also to the number of statistically independent elements per sentence. The effective number of statistically independent elements in a sentence is typically around two words per sentence. This is less than the number of words in the sentence because the words in a sentence are related by the contextual information of the sentence ( Boothroyd & Nittrouer, 1988 ). In CI users having a lowered maximum proportion correct score, word scoring is a good option, because this type of scoring can still be used, while sentence scoring is not feasible.
If word scoring is used, an adaptive procedure has to prescribe how the step size depends on the proportion of correct words. Hagerman and Kinnefors (1995) described such a procedure. They used small step sizes if only some of the words were recognized and larger steps if all words or none of the words were recognized. Brand and Kollmeier (2002) proposed a generalization of the Hagerman and Kinnefors procedure based on the difference between the proportion of correct words in the previous trial and the target proportion correct. This difference was divided by the slope of the intelligibility function and scaled by a scaling function that governed the step size sequence. A concern with this adaptive procedure is that the optimal step size is related to the slope of the intelligibility curve, which is most often unknown and can vary considerably in CI users and hearing-impaired listeners.
The accuracy of an SRT50n estimate also depends on the adaptive procedures themselves and the way in which the SRT50n is calculated. Often, adaptive procedures use a fixed step size to govern SNR placement and the average SNR over the trials as the SRT50n estimate ( Nilsson et al., 1994 ; Plomp & Mimpen, 1979 ). These simple up-down procedures are nonparametric. Several researchers used a parametric maximum-likelihood estimation of the SRT50n and the slope, with the aim of improving accuracy ( Brand & Kollmeier, 2002 ; Versfeld et al., 2000 ). However, Versfeld et al. showed that maximum-likelihood estimates were not systematically different from an estimate based on the average of the last 10 sentences of the nonparametric simple up-down procedure. Others have proposed Bayesian methods to estimate the parameters of the psychometric function ( King-Smith & Rose, 1997 ; Kontsevich & Tyler, 1999 ). Such methods can also be used to control SNR placement (e.g., Doire et al., 2017 ; Shen & Richards, 2012 ). In general, both maximum-likelihood estimation and Bayesian estimation require some prior knowledge of the intelligibility function. Most studies have assumed the maximum proportion correct near 1 and did not test the performance of an estimation method for a lower maximum proportion correct score (but cf. Green, 1995 ). Shen and Richards (2012) proposed a method that includes an estimation of the maximum proportion correct. A disadvantage of their method is that all parameters of the psychometric function must be estimated concurrently, which requires a larger number of trials at well-distributed SNRs. In contrast, nonparametric methods only assume a monotonic increasing intelligibility function (cf. Robbins & Monro, 1951 ) and are able to estimate the SRT50n as the only parameter. Although some prior knowledge of the mean and slope may help to optimize nonparametric adaptive procedures, this knowledge is not a fundamental requirement. Furthermore, nonparametric methods are easier in concept and calculation.
The nonparametric adaptive procedures are in fact stochastic approximation (SA) methods that try to approximate the SRT50n based on scores from earlier trials, which are stochastic in nature. SA algorithms were originally developed to find the roots of a function if only noisy observations are available ( Robbins & Monro, 1951 ). In the context of this study, it means to find the root of the function f(SNR) – 0.5 , in which f is the intelligibility function. Nowadays, there is a large body of literature on SA describing a variety of recursive SA algorithms with different step size sequences (for an overview, see Kushner & Yin, 2003 ).
SA algorithms often have step size sequences that decrease with increasing trial number n . The rationale is that the estimation of the root (or target proportion correct) is more accurate if the step size decreases during the recursive approximation ( Kushner & Yin, 2003 ). Decreasing step size sequences have also sometimes been used for speech-in-noise measurements ( Brand & Kollmeier, 2002 ; Keidser et al., 2013 ).
A concern of using a decreasing step size sequence in speech tests is that it makes an adaptive threshold estimation algorithm more prone to bias due to nonstationary behavior of the listener, such as lapses in attention. Fatigue can also occur, although Dingemanse and Goedegebure (2015) have found no effect of fatigue in a typical experiment with CI users. A second concern regarding the use of decreasing step sizes is that there is a risk of bias if the SNR of the first trial is relatively far from the real SRT50n . So, when using SA algorithms with decreasing step sizes, consideration should be given to possible effects of nonstationary behavior of the listener and the selection of the initial SNRs.
The aim of this study is to find an efficient SA algorithm for SRT50n estimation in CI users, using word scoring, and taking into account intelligibility functions with less steep slopes and a lower maximum intelligibility score in quiet.
The research questions are as follows:
- Is there an SA algorithm based on word scoring that provides a more efficient estimate of the SRT50n than clinically used procedures in CI users?
- What are the conditions for reliable use of adaptive measurements of SRT50n in CI users, with respect to the speech intelligibility score in quiet and the initial SNR?
To answer these questions, we selected several SA algorithms from the literature. We used Monte Carlo simulations to investigate the efficiency and accuracy of the SA algorithms. The main outcome measures were the standard deviation (SD) and the bias of the estimated SRT50n . Simulations with NH subjects were included to get insight into possible differences in optimal algorithms or parameters between CI recipients and NH listeners.
Materials and Methods
Sa algorithms.
To find the root of a function f(SNR) – P t , with P t the target proportion correct, SA algorithms use an adaptive up-down procedure of the form:
where x n is the stimulus value (the SNR) of the n th trial, y n the proportion of correctly recognized words as a noisy measurement of the value f(x n ), P t the target proportion correct, and a n the step size parameter of the n th trial. Robbins and Monro (1951) proved that a decreasing step size sequence of a n = b/n implies convergence of x n to x t with f(x t ) = P t , where b is the step size constant, and f a monotonically increasing function. In the literature on SA, many other step size sequences and their convergence are described, and even other recursive formulas have been proposed ( Kushner & Yin, 2003 ).
For our purpose, we need SA algorithms that have the following properties: (a) a good small-sample convergence because sentence lists have a relatively small number of trials (10–30 sentences) for reasons of test efficiency; (b) good rejection of the noise in the y n because the variance of the noise in y n is large; (c) insensitivity to badly chosen initial values or large deviations of y n from P t early in the procedure to prevent bias; and (d) tolerance with respect to some nonstationarity in the intelligibility function due to nonstationary behavior of the participants, such as varying attention. Note that these four requirements describe different aspects but are not independent of each other. In general, smaller step sizes are better for noise rejection, and larger step sizes lead to faster forgetting of initial conditions.
In the SA literature, four algorithms were found that may meet the aforementioned criteria. The first algorithm is the accelerated SA ( Kesten, 1958 ). Kesten proved that the convergence of the SA sequence can be accelerated compared with the original form ( Equation 1 ) if the step size decreases on reversals of the direction of the iterates.
where n rev is the number of reversals. The last iterate x n + 1 is the estimate of the x t for which f(x t ) = P t . The accelerated SA has good small-sample convergence. We need to determine the optimal value of b for speech tests.
A second algorithm is the averaged SA with decreasing step size (dss) sequence (averaged dss SA). It uses the original algorithm of Equation 1 together with averaging of the iterates:
with step size decrease rates α. Because x has to converge to the target, it is likely that the first trials are not close to the target. Therefore, the first n e trials may be left out of the average. The result of the average x ¯ n + 1 gives the estimate of x t . In the SA literature, this is known as Polyak–Ruppert averaging ( Polyak, 1990 ; Polyak & Juditsky, 1992 ; Ruppert, 1988 ). It was shown by Polyak and Juditsky (1992) that this average is preferable if the step size sequence [ a n ] goes to zero slower than order 1/n . The idea is that relative large step sizes [ a n ] lead to faster forgetting of initial conditions, while use of the average reduces noise. In the original form, n e = 0, but it is also possible to introduce exclusion of the initial values with n e > 0. For this algorithm, we need to determine the optimal step size sequence parameters b , α, and n e .
A third option is the use of a not decreasing step size (ndss) sequence together with averaging (averaged ndss SA). In fact, this is the Polyak–Ruppert averaging from Equation 3 with α = 0 and a n = b. This option was used in speech recognition tests by Hagerman and Kinnefors (1995) . They proposed a procedure with P t = 0.4 and a n = b = 5 for five-word sentences. If applied to six-word sentences, as in this study, the procedure is implemented by choosing P t = 0.5 and a n = b = 6.
A fourth algorithm that may be suitable to use with a speech test is the so-called smoothed SA that was first described by Bather (1989) and was further considered by Schwabe (1994 ; Schwabe & Walk, 1996 ). In this algorithm, the average of both the iterates x n and the noisy observations y n are used in the recursive equation:
The average of the iterates x ¯ n + 1 is the estimate of x t , also with the possibility to exclude the first n e trials. Schwabe and Walk (1996) showed that for step sizes with ½ < α < 1, the influence of inappropriate starting points decays faster than in Polyak–Ruppert averaging.
Simulation Model of a Listener
To be able to test the accuracy of the proposed SA algorithms with Monte Carlo simulations, we have made a simulation model of speech recognition that generates a listener’s response for a given SNR.
The first element of the listener model is an intelligibility function that describes the average proportion correct words in a sentence as a function of the SNR. The intelligibility function was modeled as
with p the proportion of correctly recognized words in a sentence, λ the lapse rate, p max the proportion correct in quiet, SRT m the x where p(x) is half (1– λ )· p max , and s the nominal slope (the slope of p at SRT m is (1– λ )· p max · s ). For higher p , lapses may occur due to moments of inattentiveness, and for low p , there may be some lapsing because the listener gives up ( Bronkhorst et al., 1993 ).
The intelligibility function was fitted to the data of a group of 20 CI users from a study of Dingemanse and Goedegebure (2015) . In that study, speech intelligibility in noise was measured at three SNRs, with three corresponding performance levels: adaptively estimated SRTs at 50% and 70% words correct and performance level at a fixed SNR of SRT50% + 11 dB. The performance was measured with and without activation of a noise reduction algorithm. Furthermore, speech intelligibility in quiet was measured. For each of the participants, the intelligibility function was fitted to all the data because the noise reduction algorithm had no measurable effect on the speech performance. Table 1 shows mean, SD, and range of the group for the different parameters of the intelligibility function. Only relatively high-performing CI users were included. SRT m and s were not significantly correlated.
Values of the Parameters of the Intelligibility Function (see Text at Equation 6 ) for a Group of CI Recipients and a Group of NH Listeners.
Range | Range | |||||||
---|---|---|---|---|---|---|---|---|
(dB) | 3.7 | 3.4 | 2.7 | –1.0–10.7 | ||||
(dB) | 4.2 | 3.4 | 3.3 | –1.0–12.7 | –5.5 | –5.5 | 0.6 | –6.6 – –4.6 |
(pc/dB) | 0.067 | 0.065 | 0.021 | 0.029–0.125 | ||||
(pc/dB) | 0.064 | 0.064 | 0.021 | 0.026–0.122 | 0.151 | 0.146 | 0.025 | 0.116–0.192 |
(pc) | 0.947 | 0.965 | 0.062 | 0.740–1.0 | 1.0 | 1.0 | 0 | 1.0–1.0 |
Note . The mean, median, SD, and range are given. For the NH group, the SRT m and the SRT50n are the same, and s and s50 are the same.pc = proportion correct; SRT50n = the speech reception threshold at a proportion of correctly recognized words of 0.5; s50 = the slope at the 0.5 point; CI = cochlear implant; NH = normal hearing; SD = standard deviation.
The intelligibility function was also fitted to the data of a reference group of 16 NH subjects with a mean age of 22 years, described by Dingemanse and Goedegebure (2019) . In that study, the SRT50n was adaptively measured using word scoring and the ndss SA algorithm with b = 4, along with the proportion of correct words at four SNRs around the individual SRT50n . The intelligibility function was fitted to the performance at these four SNRs, assuming that λ ≈ 0. Table 1 shows the parameter values found. In both studies, Vrije Universiteit (VU) sentences (2 lists of 13 sentences for each condition) and steady-state speech-spectrum noise were used ( Versfeld et al., 2000 ).
In practice, variation in intelligibility from trial to trial occurs due to variability in the SRT and slope of sentences, differences between listeners, and variability in listening effort and attention. We modeled variability in sentences by adding a normal distribution of SRT m values with a small SD SD_SRT m = 0.5 dB and a normal distribution of variation is slopes with SD_slope = 0.01. These values were in accordance with Versfeld et al. (2000) . To incorporate differences between subjects, variation of SRT50n between subjects was modeled as a normal distribution with an SD of 1 dB for the NH group (based on Versfeld et al., 2000 ) and 3 dB for the CI group (based on Table 1 ). The variation in slope between listeners was varied according to a normal distribution with an SD of 0.02, according to Table 1 . To account for variability in attention, the lapse rate (λ in Equation 6 ) was set to 0.02 independent of the proportion correct score. This means that in 2% of the trials the listener is not attentive.
The second element of the listener model models the response of a listener in a trial. For this element, a multinomial distribution is used, giving the probabilities that k out of l words ( k = 0, … , l ) of a sentence were correctly recognized as a function of the average proportion correct word score. The multinomial distribution was obtained from a model of Bronkhorst et al. (1993 , 2002 ) for context effects in speech recognition. This model gives predictions of the probabilities p w,k that k elements ( k = 0, … , l ) of wholes containing l elements are recognized. These probabilities p w,k are a function of a set of context parameters c i ( i = 1, … , l ) and the recognition probabilities of the elements if presented in isolation (no context) p i,nc .
The context parameters c i give the probabilities of correctly guessing a missing element given that i of the l elements were missed. They quantify the amount of contextual information used by the listener. The maximum value of 1 means that a missing element is available from context information without uncertainty. The minimum value is the guessing rate if the whole contains no context information. For details of the model, we refer to Bronkhorst et al. (1993 , 2002 ). From the array of p w,k values, we can calculate the average proportion of correctly recognized words in sentences:
This model was fitted to speech recognition data of a group of CI users and a group of NH listeners from the study of Dingemanse and Goedegebure (2019) , resulting in a set context parameters for each group (see their Figure 4 ). In the study of Dingemanse and Goedegebure, VU sentences ( Versfeld et al., 2000 ) were used as speech material in both groups.

Left panel: RMS within-staircase SDs for the SA methods as a function of the step size constant b for the CI group. Each data point is calculated from 2,000 simulations. Only results for 26 trials were shown. Right panel: SRT50n estimates minus the true SRT50n plotted together with the SD and bias of the data as a function of the within-staircase SD. The data originate from 2,000 simulations with 26 trials and the averaged ndss SA algorithm, with b = 4.
SA = stochastic approximation; dss = decreasing step size; ndss = not decreasing step size; RMS = root mean square; SD = standard deviation; SRT50n = speech reception threshold in noise.
Figure 1 shows in the left panel the probabilities p w , k as a function of p e for the CI group. For example, at the 50% correct point of the intelligibility function ( p e = 0.5) in 25% of the trials, the whole sentence is recognized ( k = 6), but in another 25%, no words are recognized ( k = 0); this is illustrated in the right panel of Figure 1 .

Left panel: Probabilities to recognize k words of a sentence correctly as a function of the average proportion of correctly recognized words p e . Center panel: Cumulative probabilities to correctly recognize k words or less as a function of p e . Right panel: Example of the multinomial distribution for an average word score of p e . = 0.5 that gives the probability to recognize k words from a sentence.
In the Monte Carlo simulations, the response of a listener in a trial was obtained following the next steps: First, the average word recognition probability was calculated from the intelligibility function ( Equation 6 ) for the SNR of the trial, resulting in value p x . Next, a random number from a continuous uniform distribution with a minimum value of 0 and a maximum value of 1 was taken, giving value p y . Third, point ( p x , p y ) was compared with the cumulative probabilities shown in the center panel of Figure 1 . For example, the point of p x = 0.5 and p y = 0.7 fell in the area of k = 5. That is, five out of six words were correctly recognized in this trial.
We added some variation in the context parameters using a normal distribution with an SD of 0.01 for c 1 to 0.016 for c 5 to simulate differences between listeners ( Dingemanse & Goedegebure, 2019 ).
Validation of the Simulation Model
The validity of the model for the description of averaged speech recognition scores has already been demonstrated by Bronkhorst et al. (1993 , 2002 ). To verify if the model not only describes speech recognition on average but also produces reliable word scores for single trials in adaptive procedures, we used the within-staircase SD as a measure to compare simulation outcomes with experimental data. The within-staircase SD shows whether the simulation model produces realistic variations within a staircase. As the model parameters were tuned to the CI group of Dingemanse and Goedegebure (2015) , the model should produce the same within staircases as found in the experimental data. The SRT50n staircases were measured in two conditions in Dingemanse and Goedegebure (2015) . The mean within-staircase SD was calculated as the root mean square (RMS) of the individual within-staircase SDs from the two conditions and resulted in a value of 2.0 dB. The adaptive procedure used was the averaged ndss SA, with b = 4. Simulations with this procedure resulted in a within-staircase SD of 2.1 dB. This corresponds very well with the experimental value of 2.0 dB.
When parameters of the NH group were applied, a within-staircase SD of 1.5 dB was found, which is in good agreement with the 1.4 dB found from the SRT50n measure in Dingemanse and Goedegebure (2019) . From the same study, a within-staircase SD of 1.9 dB for sentence scoring combined with a fixed step size of 2 dB and 13 trials was available. The within-staircase SD of the simulation of this condition was also 1.9 dB.
Versfeld et al. (2000) reported that the within-subjects SD of the SRT50n was 1.1 dB for sentence scoring and an adaptive up-down procedure with a 2 dB step size. A simulation of this condition resulted in a within-subjects SD of 1.1 dB.
These results confirmed the validity of the used listener model for use in simulations of adaptive procedures.
Calculation of Reference SDs at SRT50n
The listener model was used to generate 4,000 responses based on word scoring at an SNR of SRT50n . The SD of these responses was calculated and served as a reference measure of the variability in proportion correct speech recognition at the SRT50n due to the stochastic nature of the speech recognition process. Table 2 presents the reference SDs of the simulations at a fixed SNR of SRT50n . The calculated SD was divided by the slope of the intelligibility function at the SRT50n point to obtain a reference SD of the SRT50n measure. The SDs of the SRT50n estimates of the SA algorithms were compared with these reference SDs to get a measure of the variability introduced by the SA algorithms itself.
Reference Standard Deviations of Proportion Correct Words From Sentences P t and SRT50n Values, Resulting From Simulations of CI and NH Listeners at a Fixed SNR of SRT50n .
Sentence list length | ||||
---|---|---|---|---|
13 | 0.137 | 2.33 | 0.121 | 0.824 |
20 | 0.104 | 1.77 | 0.091 | 0.616 |
26 | 0.089 | 1.52 | 0.078 | 0.528 |
Note . SRT50n = speech reception threshold in noise; CI = cochlear implant; NH = normal hearing; SD = standard deviation.
In the simulation model, small variations in SRT50n and slope between sentences and between subjects were included, as mentioned in the model description. By comparing the simulation results with and without applying variations, it turned out that the effect of the variations in model parameters was a 4% to 6% increase of the SDs in CI users and a 0.5% to 1.3% increase in NH users.
The SDs of the P t estimates in the CI group were slightly greater than the SDs of the NH group due to the fact that the model for CI users had higher values for the context parameters. The SDs of SRT50n are higher in CI users because the slope of the intelligibility function is less steep. SDs decreased approximately with the square root of the list length, bearing in mind that the first four sentences were excluded in the calculations for all list lengths.
Simulation Procedures
In the simulations, we used a slope of 0.15 dB –1 for NH users and half that value for the CI group ( Equation 6 ). The parameter p max was set to 1 for NH listeners. For relatively high-performing CI users, the value was 0.95 according to Table 1 . To represent a broader range of performance values between 0.6 and 1, p max was set to 0.8 for CI users. The initial SNR (the SNR of the first trial) relative to the mean SRT50n was taken from a normal distribution with mean = –3 dB (NH) or –6 dB (CI) and SD = 1 dB (NH) or 3 dB (CI). The first trial was repeated at increasing SNRs (+2 dB) until at least half of the words were recognized correctly or the sentence was three times repeated.
In the simulations, independent streams of random numbers were generated for each variable for which a probability distribution was defined. For each condition, 2,000 simulations of staircases were generated, and each staircase consisted of 26 trials. For each simulation, the SRT50n estimate was the average or the end value of the staircase, depending of the SA algorithm. For each condition, three outcome measures were calculated: the SD and bias of SRT50n , and the within-staircase SD calculated as the RMS average of the 2,000 SDs of the SNRs within each staircase. We calculated the three outcome measures for sentence list lengths of 13, 20, and 26 sentences, as the minimum list length is 13 sentences for the speech material used in the model. A length of 26 sentences (two lists) is around a maximum length that can be used in clinical settings, in our opinion. A length of 20 sentences is included because this list length is used in other speech material (e.g., Soli & Wong, 2008 ), and it is in the middle of the clinically feasible range of the number of sentences to be used. All simulations and analyzes were performed with MATLAB (9.6.0, The MatWorks Inc., Natick, Massachusetts, USA).
Finding Optimal Parameters for SA Algorithms
To find optimal values of the parameters in the SA algorithms, simulations were performed while varying the relevant parameters. The step size constant b was varied from 2 to 14 dB in steps of 2 dB for the CI group and from 1 to 7 dB in steps of 1 dB for the NH group. Because the maximum of ( y n – p t ) in the Equations 1 to 4 is 0.5, b = 4 corresponded to the often used step size of 2 dB. For the averaged dss SA and the smoothed SA, optimal parameters were determined by simulations for step size decrease rates α from 0.1 to 0.5 with a step of 0.1 for the averaged dss SA and from 0.5 to 1 (step 0.1) for the smoothed SA. For the averaging SA algorithms, the number of excluded trials n e was 4, 6, or 8 trials.
To find the best parameter set of b , α, and n e , we looked for minimum SD and bias of SRT50n for each combination of b , α, and n e . However, the minima of SD and bias were often not reached at the same parameter values. We regarded a minimum SD as the most important criterion (i.e., for test–retest purposes), but we did not allow differences in intelligibility greater than 5% due to bias because that may become a clinically relevant difference. Based on this criterion, the mean bias should be ≤0.85 dB in the CI group and ≤0.33 dB in the NH group. The parameter set that produced the smallest SD of SRT50n within these bias criteria was chosen as the optimal parameter set of b , α, and n e . The optimization was done for each of the three list lengths.
Simulations With the Optimal SA Algorithms and Clinical Procedures
In the simulations, we also included some clinically used procedures. First, sentence scoring with a fixed step size of 2 dB was included ( Nilsson et al., 1994 ; Plomp & Mimpen, 1979 ). Second, a procedure of modified sentence scoring was added, allowing 2 errors per sentence (66.67%) such as in Chan et al. (2008) and Wong and Keung (2013) . In this procedure, the SNR was varied adaptively as in Chan et al., that is, in 5 dB steps for the first four sentences and in 3 dB steps for the remaining sentences of the list for the CI group. For the NH group, the steps were 4 dB for the first four sentences and 2 dB for the remaining trials as in the Hearing in Noise Test procedure ( Soli & Wong, 2008 ). Because the psychometric curve of Equation 6 applies to word scoring, we calculated the change of the psychometric curve from the context model ( Equations 7 and 8 ) for sentence scoring and modified sentence scoring. Figure 2 shows the resulting curves.

Intelligibility Functions of Correctly Recognized Words From Sentences, Sentence Scoring, and Modified Sentence Scoring. The three leftmost curves represent the functions of the NH group, and the three rightmost curves represent the functions of the CI group. Dots show the target proportion correct of 0.5.
NH = normal hearing; CI = cochlear implant; SNR = signal-to-noise ratio.
Furthermore, we included a third clinically used procedure based on word scoring: the procedure of Brand and Kollmeier (2002) . They proposed the following formula:
We used p max · s as slope value. Brand and Kollmeier used a maximum-likelihood estimate of the SRT50n , but because only nonparametric methods are investigated in this study, the last iterate x n + 1 was used as an estimate of the threshold x t . Henceforth, this procedure will be referred as the npBK SA procedure.
We performed simulations with each optimized SA algorithm and the clinical procedures to investigate how their accuracy depends on the relative initial SNR by varying this SNR from –8 dB to +8 dB relative to the real SRT50n value. In these simulations, the first trial was not repeated. In addition, we examined the effect of the maximum intelligibility in quiet. The parameter p max was varied in five steps from 0.6 to 1 for each optimized algorithm, and the relative initial SNR was taken from a normal distribution, as described earlier.
Simulations With SA Algorithms to Find Optimal Parameters
Based on all simulations, we selected optimal parameters for each SA algorithm for both listener groups according to the criteria given in the Methods section. Exclusion of the first four trials ( n e = 4) in the averaging resulted in the smallest SD and bias values of SRT50n for all list lengths, compared with 6 or 8 ignored trials, although differences were small (between 0 and 0.15 dB). Therefore, only results for n e = 4 were presented throughout the Results section.
For the smoothed SA, we found that the last iterate was a better estimate for SRT50n with smaller SDs than the average of the iterates. So this end value was used instead of the average value.
Regarding the step size decrease rate α, it was found that a midrange value together with a moderate initial step size b resulted into the smallest SD and bias in CI users. A small initial step size and a large decrease rate resulted in a large bias. A large initial step size and a large decrease rate resulted in lower SD and bias, but even lower values were found for a moderate decrease rate and initial step size. Table 3 shows the optimal parameters and the SD and bias that were obtained with these parameters. The optimal step size decrease rate α was the same for CI and NH listeners, but the step size constant b was larger for the CI group. In CI users, the parameters given in Table 3 resulted in a bias smaller than the criterion value of 0.85 dB in the range of –8 to +4 dB for a staircase length of 26 sentences. For relative initial SNRs > 4 dB, the bias exceeded the criterion value for any set of parameter values. For a staircase length of 20 sentences, the bias exceeded the criterion value for a relative initial SNR > 3 dB. A list length of 13 sentences resulted in relatively high SDs and/or large bias (see also Figure 3 ) and was therefore not suitable.
Optimal Values for the Step Size Constant b and the Step Size Decrease Rate α for the Accelerated SA Algorithm, the Averaged SA Algorithm With Decreasing Step Size (dss) or Not Decreasing Step Size (ndss), and the Smoothed SA Algorithm if Applied in CI Recipients and in NH Listeners.
SA algorithm | ||||||||
---|---|---|---|---|---|---|---|---|
α | α | |||||||
Accelerated SA | 6 | – | 1.77 | –0.40 | 4 | – | 0.55 | –0.06 |
Averaged dss SA | 6 | 0.3 | 1.65 | –0.23 | 5 | 0.3 | 0.55 | –0.02 |
Averaged ndss SA | 4 | – | 1.71 | –0.02 | 4 | – | 0.58 | 0.01 |
Smoothed SA | 6 | 0.7 | 1.71 | –0.30 | 4 | 0.7 | 0.55 | –0.06 |
Note . For each optimized SA algorithm, the SD and bias of the SRT50n estimates are provided. SA = stochastic approximation; CI = cochlear implant; NH = normal hearing; SD = standard deviation.

Estimated Values of SD (Solid Lines) and Bias (Dashed Lines) of SRT50n as a Function of the Step Size Constant b From Simulations With the Different SA Algorithms. The upper row of panels shows the results of the CI group, and the second row shows the results of the NH group. Downward-pointing triangles: 13 sentences, squares: 20 sentences, and upward-pointing triangles: 26 sentences.
SRT50n = speech reception threshold in noise; CI = cochlear implant; NH = normal hearing; SD = standard deviation; SA = stochastic approximation; dss = decreasing step size; ndss = not decreasing step size.
Figure 3 shows the effect of the step size constant b on the SDs and biases of SRT50n for the different SA algorithms (with optimal α value). The panels on top of the figure show the results for the CI group, and the bottom panels show the results for the NH group. We observed that the SD of SRT50n was much greater in CI recipients than in NH listeners for all SA algorithms. In CI users, the SD was smallest for b = 4, except for the averaged ndss SA that had the smallest SD for b = 2. But for these b values, too much negative bias was found. Therefore, b = 6 (4 for the averaged ndss SA) was found to be optimal. In the NH group, the SDs of SRT50n were small and almost independent of b , indicating that the step size constant is not critical. The bias was close to zero for all algorithms and b values. Using a larger number of sentences resulted in smaller SD and bias for all conditions. It is remarkable that the different SA algorithms resulted in comparable minimum SDs.
The Within-Staircase SD
The left panel of Figure 4 shows the RMS within-staircase SD as a function of the step size factor b for the CI group. The RMS within-staircase SD increased for increasing b , as expected, but differed in size between SA algorithms. The smallest values were found for algorithms with decreasing step size. The right panel shows the SRT50n estimates minus the true SRT50n as a function of the within-staircase SD for the averaged ndss SA algorithm, with b = 4. The data points were grouped in bins of 1 SD width, and the mean (which is the bias) and SD were calculated for each bin and then plotted. Figure 4 shows that no clear relationship exists between the within-staircase SD and the SD or bias of the SRT50n estimates. This holds also for a list length of 20 sentences, for the other SA algorithms with optimized parameters, and for the NH listeners.
The Effect of the Initial SNR
Figure 5 shows the effect of the initial SNR (the SNR of the first trial relative to the true SRT50n of the intelligibility function) on the SD and bias of the SRT50n estimate. The simulations were performed with the optimal parameters given in Table 3 . Figure 5 only shows results for a staircase length of 26 trials because the pattern of results for 20 trials (CI and NH) or 13 trials (NH) was very similar.

SD and Bias of SRT50n Estimates as a Function of the Initial SNR Relative to the True SRT50n for the SA Methods and Clinical Procedures. In the top left panel, the SD of sentence scoring is out of range. At an initial SNR of –8 dB, this SD is 4.5 dB, and it increases almost linearly to 6.5 dB at +6 and +8dB.
NH = normal hearing; CI = cochlear implant; SNR = signal-to-noise ratio; SA = stochastic approximation; dss = decreasing step size; ndss = not decreasing step size; npBK = nonparametric Brand & Kollmeijer; SD = standard deviation; SRT50n = speech reception threshold in noise.
The SD and bias were very similar between the different SA algorithms over the entire SNR range. A relatively high bias was found for positive initial SNR values for the CI group. The bias was around zero, and the SDs were smallest for initial SNRs below the true SRT50n . From these results, it is clear that an initial SNR below the true SRT50n would be preferable. In the NH group, the SD was almost independent of the initial SNR, and the bias was within ±0.2 dB.
As a validation, we compared the simulation of the ndss SA algorithm with b = 4 with data of the NH group from Dingemanse and Goedegebure (2019) . In that study, the SRT50n was adaptively measured using the same algorithm and an initial relative SNR of 1 dB on average. In addition, an intelligibility function was fitted to the proportion of correct words at four fixed SNRs around the individual SRT50n. The SD of the individual differences between the SRT50n of the adaptive procedure and the SRT50n of the fitted intelligibility function was 0.55 dB. The SD of the simulations was 0.58 ( Figure 5 ) and is in good agreement with the experimental SD.
The clinical algorithms had higher SDs of SRT50n than the SA algorithms over the entire SNR range. For the CI group, sentence scoring resulted in a high SD and a bias that showed that the adaptive procedure was hardly able to move the SNR value away from the initial SNR. This is in accordance with the almost flat intelligibility function around a proportion correct of 0.5 (see Figure 2 ). The modified sentence scoring resulted in a much better SD around 2.8 dB and a positive bias between 0.7 and 1.4 dB. The SD of the npBK SA algorithm is nearly as small as the SDs of the SA algorithms in the NH group. But in the CI group, the SD is clearly greater than that of the SA algorithms, and the bias is positive.
The SA algorithms using word scoring resulted in the smallest SD and bias. For the NH group, sentence scoring resulted in an SD of 0.92 dB and only a small bias for all initial SNRs. The modified sentence scoring resulted in a smaller SD of around 0.73 dB due to the steeper slope of the intelligibility function ( Figure 2 ), but it was still higher than the SDs of the SA algorithms that were around 0.58 dB.
The Effect of Reduced Maximum Intelligibility
The effect of p max was investigated for the CI group with each of the optimal algorithms and the three clinical algorithms. Figure 6 shows that p max had a large effect on the SD and bias of the SRT50n estimates. The SD increased for decreasing p max . This effect was most apparent for sentence scoring, modified sentence scoring, and the npBK SA algorithm. For the range of p max between 0.7 and 1, the SA algorithms were efficient, that is, close to the reference SD from Table 2 that serves as a theoretical minimum. At p max = 0.6, bias values become more negative on average. Only the results for a staircase length of 26 trials were shown because the pattern of results for 20 trials was very similar, with small bias and efficient estimation for p max ≥ 0.7.

SD and Bias of SRT50n Estimates as a Function of p max for the SA Methods and Clinical Procedures Applied in the CI Group. Only results of the conditions with 26 trials were shown. The dash-dotted line with asterisks gives the minimum SD based on the reference SD in Table 2 as a function of p max .
SA = stochastic approximation; dss = decreasing step size; ndss = not decreasing step size; npBK = nonparametric Brand & Kollmeijer; SD = standard deviation; SRT50n = speech reception threshold in noise.
SA Methods Versus Clinical Procedures
The four SA algorithms proposed in this study provide more efficient estimates of the SRT50n than clinically used adaptive procedures in CI users, as can be observed from Figures 5 and and6. 6 . The SD estimates of the four SA algorithms were close to the reference SDs from Table 2 , indicating that the SA algorithms add little variance to the SRT50n estimate, compared with the variability due to the stochastic nature of the speech recognition process. Even with the more shallow intelligibility functions found in CI users, the algorithms remain efficient, provided that p max ≥ 0.7 and the initial SNR is within –8 to +4 dB of the real SRT50n .
Several researchers recognized the inaccuracy of sentence scoring in CI users and proposed a modified sentence scoring that allows some errors per sentence ( Chan et al., 2008 ; Wong & Keung, 2013 ). Indeed, the modified sentence scoring resulted in better accuracy. But the SA algorithms had both smaller SD and bias, especially when p max is below 1 ( Figure 6 ). This can be explained by their use of word scoring that has a higher number of statistically independent elements per sentence, as explained in the Introduction section.
The new proposed SA algorithms also performed better than the npBK SA algorithm. The main reason is that this algorithm has relatively large steps early in the staircase and a high decrease rate. Especially in the CI group, having a lowered p max , this combination resulted in a larger SD and bias. The large steps early in the staircase may result in high SNR values, were the intelligibility function is already flat. In this flat part of the function, the SNR may jump randomly up and down at high SNRs, while the step size is decreasing. As a result, the staircase ends with a large positive bias.
The four SA algorithms proposed in this study resulted in comparable SD and bias if parameters were used that were optimal for the group that was tested. There is no clear winner. It is noteworthy that a more complex SA method, such as the smoothed SA, did not result in better performance than the simpler ndss SA method. The optimal step size decrease rate α was the same in CI and NH listeners, both for the averaged dss SA and for the smoothed SA algorithm. The only difference between groups is the step size constant b , except for the averaged ndss SA algorithm, where b = 4 applies to both groups. The NH group and the CI group represent the extremes of the intelligibility function. The group of people with sensorineural hearing loss, using hearing aids or not, is expected to have intelligibility functions with slopes in between the slopes of the NH group and the CI group. So, the averaged ndss SA algorithm with a step size constant of 4 is applicable to a wide range of hearing-impaired listeners. This algorithm was already used in speech recognition tests by Hagerman and Kinnefors (1995) . Furthermore, it was used in several studies with CI recipients and provided highly reproducible and consistent data (cf. Dingemanse & Goedegebure, 2015 , Figure 3 ; 2018, Figure 3 ; Vroegop et al., 2017 ).
The use of simulations gave the possibility to gain insight into the occurrence of a bias. Because the true SRT50n of the listener model is known, the bias can be calculated, which is impossible in real subjects with unknown SRT50n . In NH listeners, the bias was close to zero for all SA algorithms if initial SNRs were within –8 to +8 dB relative to SRT50n . If in the first trials a large step in the wrong direction is made due to the stochastic behavior of the speech recognition process, then the average proportion correct at the next SNR is much higher or lower because of the steep slope of the intelligibility function. This leads to a high chance that a reversal occurs and that is why no bias occurs. Furthermore, the intelligibility function is symmetrical in the SRT50n point in NH listeners, making that steps from above or from below the SRT50n point on average have equal but opposite effects that are averaged out. In CI users, only a small bias (<0.85 dB) was present if optimal parameters are used. The bias depended on the relative initial SNR. An SNR more than 4 dB above the SRT50n resulted in a relatively large positive bias. The explanation is that the slope of the intelligibility function well above SRT50n becomes very shallow, making the adaptive procedure not very effective, as already explained for the npBK SA algorithm.
The within-staircase SD was dependent on the step size constant, the decrease rate of the step size, the number of trials, and the intelligibility function ( s and p max ) of the group of listeners. As a consequence, the within-staircase SD cannot be used as a measure of the reliability of a single SRT50n measurement in combination with a fixed criterion (cf. Keidser et al., 2013 ). We analyzed if the SD and bias of the SRT50n estimates was dependent on the within-staircase SD. In the stimulations, within-staircase SDs up to approximately twice the RMS within-staircase SD of the group were seen. For this range, no relationship was found for the averaged ndss SA with b = 4, neither in the CI group ( Figure 4 ), nor in the NH group. This means that the within-staircase SD is not really suitable as a measure for the reliability of an individual staircase. Only if a single staircase has a very large within-staircase SD compared with the group value (as a rule of thumb: more than twice the RMS within-staircase SD of the group), one may decide to reject this measurement.
Influence of Maximum Intelligibility on Accuracy
A decrease of the maximum intelligibility in quiet p max caused an increase in the SD of the SRT50n estimates. This was as expected and was mainly caused by the decrease of the slope of the intelligibility function to p max times the original slope at p = ½ p max . At p = 0.5, the slope is reduced even more because at this point the slope is no longer at its maximum value. For a smaller part, the increase in the SD of the SRT50n estimates was caused by a decreasing efficiency of the adaptive procedure for decreasing p max . As can be seen from Figure 6 , if p max decreases, the difference between the SDs of the SA algorithms and the theoretical minimum SD increases. There was also some bias in the SRT50n estimate, but this remained acceptable small (< 0.5 dB) if the initial SNR was not too far from the true SRT50n value.
For CI users with p max ≥ 0.7, but < 1, it is advantageous to start at an SNR that is below the real SRT50n . Then, the trials are in the steepest part of the intelligibility function, which makes the SA algorithms converge better toward the target. As a result, both bias and SD were smaller ( Figure 5 ). According to Figure 6 , the minimum p max required for reliable use of adaptive estimation of SRT50n is p max = 0.7 provided that at least 20 sentences are used.
The Simulation Model
The development and application of a realistic and detailed simulation model of speech recognition was an important part of this study. The usefulness of the model for single trials in adaptive procedures was verified by comparing the within-staircase SDs of the simulations with the within-staircase SDs of the participants in the studies that were used to determine the model parameters. They matched very well. Furthermore, simulation of sentence scoring was in good agreement with the data of Versfeld et al. (2000) , and simulations of word scoring with the ndss SA for NH listeners agreed well with results of Dingemanse and Goedegebure (2019) . These findings show that the model appears to be a valid tool for evaluation of adaptive speech-in-noise algorithms.
The good agreement between simulations and experimental data is based on the detailed and already validated model of Bronkhorst et al. (1993) that predicts the proportions correct of k out of l words correctly. In the model, the effect of contextual information is incorporated. Due to the contextual information, a listener has a higher chance to predict initial missed words correctly from the words that were already understood. Brand and Kollmeier (2002) also used Monte Carlo simulations to examine adaptive procedures for sentences-in-noise tests with word scoring. To account for the effect of the contextual information, they used the j factor of Boothroyd and Nittrouer (1988) , a factor that quantifies the number of statistically independent words in a sentence. In their simulations, each trial consisted of j Bernoulli trials, and the proportion correct score for each trial was calculated by dividing the sum of the results of the Bernoulli trials by j. However, the resulting distribution of proportion correct scores is not in accordance with the distribution that is found in sentence recognition, having a relatively large proportion of 0 and 1 values (see Figure 1 and also Hu et al., 2015 ). Furthermore, only integer values of j can be used. In contrast, the multinomial distribution of proportions from the model of Bronkhorst et al. (1993) as shown in Figure 1 was in good agreement with experimentally found distributions for all percent correct values. Also noninteger values of j that were dependent of the proportion correct value were a result of this model ( Dingemanse & Goedegebure, 2019 ).
We added small stochastic between-sentence variations in SRT50n and slope that exist within speech materials and individual listeners. We also added between-subject variations in context parameters and slopes. Addition of these stochastic variations has made the model more realistic, but the effects of these variations were small. This is in accordance with the finding of Smits and Houtgast (2006) , who also reported that variations in SRT50n and slope had only a small effect in a digit-in-noise test.
In the simulation model, some lapsing was included, but the lapse rate was kept constant over time. In future use of simulation models, it is worth to consider more variation in this lapse rate to simulate variations in attention and/or fatigue. These variations should be based on experimental data on attention variations and fatigue effects. However, we expect that the effect of lapsing on the accuracy is limited. The effect of lapsing is comparable with a reduction of p max (see Equation 6 ). Figure 6 shows that for a reduction of p max from 1 to 0.9, the increase of the SD and bias of SRT50n was limited. So, for lapse rates smaller than 10%, the effect of lapsing on the SRT50n estimate is small.
Usefulness of Adaptive Speech-in-Noise Tests in CI Recipients
Although SA algorithms provide relatively accurate estimations of the SRT50n in CI users, the SD of the SRT50n estimate was still much larger in the CI group than in the NH group, depending on p max and the slope of the intelligibility function. The decreased slope in CI users (even for p max = 1) is due to difficulties in understanding the sentences in this open-set speech material with relatively good real-life similarity. In contrast, if a closed-set speech material is used, such as a matrix sentence test ( Kollmeier et al., 2015 ), the difference in slope between CI and NH listeners is much smaller ( Hey et al., 2014 ; Theelen-van den Hoek et al., 2014 ), and the j factor is higher: approximately 4 ( Wagener et al., 1999 ). This may be of help to obtain a more reliable SRT50n value, but the ecological validity of the speech material is much less than the sentences used in this study.
The question is whether a larger SD of the SRT50n estimate in CI users is problematic. From the perspective of CI recipients, a perceived increase in speech intelligibility is more important than a change in SRT50n . If the slope of the intelligibility curve at 50% is shallow, a larger shift in SNR is needed to obtain a relevant increase in speech intelligibility. This allows a less accurate estimate of the SNR. A typical SD value for the SA procedures is 1.7 dB for 26 sentences of the speech material used in this study. An SNR difference of 1.7 dB corresponds to an intelligibility difference of 10%. In NH listeners, the SD of the SA methods is 0.6 dB, corresponding to an intelligibility difference of 9%. So, in terms of intelligibility, the accuracy of the speech-in-noise test in CI users is comparable with the accuracy in NH listeners.
Because of the relatively large SDs in the CI group, it is often not possible to compare two conditions or two algorithms within an individual. The test–retest SD is √2 times the SD of a single measurement. A significant difference at the .05 level requires a difference of at least 1.96 · √2 · SD. In our example, 1.96 · √2 · 1.7 = 4.7 dB. Therefore, only differences in conditions that result in large SRT differences can be reliably detected in individuals. If one wants to compare two conditions in a research setting, the relatively high SD can be compensated by the group size.
General Discussion and Limitations
In clinical practice, often the first sentence is presented repeatedly with increasing SNR until the sentence is recognized ( Plomp & Mimpen, 1979 ). We also used this procedure in the simulations, but we used a relatively small step of 2 dB and restricted the number of repetitions to a maximum of 3. This restriction prevented for initial SNRs that are (much) greater than the SRT50n because these SNRs would have resulted in more variability in the SRT50n estimate (according to Figure 5 ). We recommend to make an educated guess of the SRT50n and to use this guessed SRT50n minus 2 to 4 dB as initial SNR. Such an educated guess may be based on norm data, preliminary data, a familiarization run, or on known relationships of the SRT50n with other clinically available speech recognition data, such as word scores (e.g., Gifford et al., 2008 ). Only if one has too little knowledge for an educated guess, it is better to use the procedure of repeating the initial trials at higher SNR (+2dB) with a maximum of three repetitions.
In this study, the target proportion correct was 0.5, regardless of the maximum speech intelligibility in quiet. Another option is to choose the target as half the maximum speech intelligibility in quiet. Then, the target is at the steepest part of the intelligibility function, and the function is more symmetrical around the target. This would lead to a smaller SD and bias for SRT50n . However, this option has three drawbacks: First, each participant is tested at his own target level, making it impossible to compare the SRT50n values among participants; second, the perceived difficulty of the test would become too high, which increases the risk that a participant gives up; third, the individual p max must be measured beforehand.
This study has some limitations. First, the VU sentences were selected for equal intelligibility at sentence level in NH listeners and not at word level in CI listeners. We have taken this into account by making variations in SRT and slope per sentence in the simulation model, but this is only an approximation. Second, the search for the best adaptive procedure was only done with use of parameters for the context model and the intelligibility function which were derived from data obtained with the VU sentences. However, the context parameters of the VU sentences are expected to be comparable with other open-set sentence materials. For example, they are comparable with the context parameters of the Göttingen sentence test reported by Bronkhorst et al. (2002) . Only if a very different speech type is used, such as a matrix test ( Kollmeier et al., 2015 ), it would be safer to repeat the simulations with a context model and an intelligibility function that are suitable to these materials.
To test if the results of this study are applicable to the matrix test, we did some simulations for matrix tests. The simulations were based on the context parameters of the Olsa test that were reported by Bronkhorst et al. (2002) . For the intelligibility function, we used p max = 0.82, and a slope of 13.5 ± 4.6%/dB at P t = 0.5, based on values of Hey et al. (2014) . Simulations for a list length of 30 trials with the averaged ndss SA algorithm with b = 4 resulted in a test–retest SD of 0.75 dB, giving a 95% confidence interval of about 3 dB. This agrees well with the range of test–retest differences reported by Hey et al. in their Figure 3 . This indicates that SA algorithms work well for the matrix test. In matrix tests, a maximum-likelihood estimation of SRT50n is used. This estimation is computationally complex and may sometimes produce more than one maximum, especially if the number of sentences is small ( Pedersen & Juhl, 2017 ). As an alternative, an SA algorithm could be used because SA algorithms are nonparametric and provide easy to calculate estimations of the SRT50n .
In this study, nonparametric SA algorithms were used to estimate the SRT50n . However, as discussed in the Introduction section, maximum-likelihood and Bayesian methods are also valuable options to estimate the SRT50n . Doire et al. (2017) reported on a robust Bayesian method and compared this method with the estimation methods of Brand and Kollmeier (2002) and Shen and Richards (2012) . They reported simulation results for several psychometrical functions. One of these functions, having a slope of 0.075 dB –1 and a lapse rate of 0.1, is comparable with the simulations of the CI group in this study. In our study, the number of statistically independent trials for 26 sentences is 52 because the effective number of independent words in the VU sentences is 2 ( Dingemanse & Goedegebure, 2019 ). Results of this study can therefore be compared with 52 trials in the Doire et al. study. For 52 trials, Doire et al. reported an SD of 2 dB and a bias of –1 dB for SRT50n for all methods used. In this study, the values are better: SD = 1.3 – 1.5 dB, and the bias is around –0.5 to –0.3 dB ( Figure 6 at p max = 0.9). On the other hand, the method of Doire et al. may be more robust for initial SNRs that are relatively far from the true SRT50n . For future research, we recommend a comparison between the nonparametric SA methods, parametric maximum-likelihood-based methods, and Bayesian methods, all with the same listener simulation model as used in this study. Furthermore, more research is needed on how to extend the different methods to measure threshold, slope, and p max concurrently.
Conclusions
In conclusion, this study showed that SA methods based on word scoring provide efficient estimations of the SRT50n in sentence-in-noise measurements, both in CI recipients and in NH listeners, if used with optimized parameters that govern the step size sequence. Although intelligibility functions in CI users have less steep slopes and a lower maximum intelligibility score in quiet, SA algorithms are capable to estimate the SRT50n efficiently. They have the advantage that knowledge of the maximum intelligibility score in silence and slope is not needed in the estimation of SRT50n .
The SA algorithms proposed in this study provided more efficient SRT50n estimates than clinical used adaptive procedures. Therefore, they are recommended for clinical use. They may also lead to more statistical power of speech-in-noise tests if used in research or equivalently in a smaller number of participants that is needed to achieve sufficient statistical power.
The different SA algorithms used in this study provide equally accurate estimations of the SRT50n . This was found both for CI users and NH listeners. The averaged SA algorithm with a step size factor of 4 is recommended for clinical use because it is relatively easy, and it is applicable to a wide range of hearing-impaired listeners. In CI users, the most accurate estimate of SRT50n is obtained if the initial SNR is chosen below the SRT50n , the step size is relatively small, and at least 20 sentences per condition are used. The within-staircase SD turned out not to be suitable as a measure for test reliability.
The SD of the SRT50n estimate increases with decreasing maximum intelligibility in quiet. The score of words from sentences in quiet should be at least 70% correct for reliable use of adaptive estimation of SRT50n .
Author Contributions
G.D. designed the study and did the simulations. Both authors did the interpretation of the data. G.D. drafted the article, and A.G. revised the article. Both authors approved the final version of the article for submission.
Declaration of Conflicting Interests
The authors declared no potential conflicts of interest with respect to the research, authorship, and/or publication of this article.
The authors received no financial support for the research, authorship, and/or publication of this article.
Gertjan Dingemanse https://orcid.org/0000-0001-8837-3474
- Bather J. A. (1989). Stochastic approximation: A generalisation of the Robbins-Monro procedure . Mathematical Sciences Institute, Cornell University.
- Boothroyd A., Nittrouer S. (1988). Mathematical treatment of context effects in phoneme and word recognition . Journal of the Acoustical Society of America , 84 ( 1 ), 101–114. 10.1121/1.396976 [ PubMed ] [ CrossRef ] [ Google Scholar ]
- Brand T., Kollmeier B. (2002). Efficient adaptive procedures for threshold and concurrent slope estimates for psychophysics and speech intelligibility tests . Journal of the Acoustical Society of America , 111 ( 6 ), 2801–2810. 10.1121/1.1479152 [ PubMed ] [ CrossRef ] [ Google Scholar ]
- Bronkhorst A. W., Bosman A. J., Smoorenburg G. F. (1993). A model for context effects in speech recognition . The Journal of the Acoustical Society of America , 93 ( 1 ), 499–509. 10.1121/1.406844 [ PubMed ] [ CrossRef ] [ Google Scholar ]
- Bronkhorst A. W., Brand T., Wagener K. C. (2002). Evaluation of context effects in sentence recognition . The Journal of the Acoustical Society of America , 111 ( 6 ), 2874–2886. 10.1121/1.1458025 [ PubMed ] [ CrossRef ] [ Google Scholar ]
- Chan J. C., Freed D. J., Vermiglio A. J., Soli S. D. (2008). Evaluation of binaural functions in bilateral cochlear implant users . International Journal of Audiology , 47 ( 6 ), 296–310. 10.1080/14992020802075407 [ PubMed ] [ CrossRef ] [ Google Scholar ]
- Dawson P. W., Mauger S. J., Hersbach A. A. (2011). Clinical evaluation of signal-to-noise ratio-based noise reduction in Nucleus® cochlear implant recipients . Ear and Hearing , 32 ( 3 ), 382–390. 10.1097/AUD.0b013e318201c200 [ PubMed ] [ CrossRef ] [ Google Scholar ]
- Dingemanse J. G., Goedegebure A. (2015). Application of noise reduction algorithm ClearVoice in cochlear implant processing: Effects on noise tolerance and speech intelligibility in noise in relation to spectral resolution . Ear and Hearing , 36 ( 3 ), 357–367. 10.1097/AUD.0000000000000125 [ PubMed ] [ CrossRef ] [ Google Scholar ]
- Dingemanse J. G., Goedegebure A. (2018). Optimising the effect of noise reduction algorithm ClearVoice in cochlear implant users by increasing the maximum comfort levels . International Journal of Audiology , 57 ( 3 ), 230–235. 10.1080/14992027.2017.1390267 [ PubMed ] [ CrossRef ] [ Google Scholar ]
- Dingemanse J. G., Goedegebure A. (2019). The important role of contextual information in speech perception in cochlear implant users and its consequences in speech tests . Trends in Hearing , 23 , 2331216519838672 10.1177/2331216519838672 [ PMC free article ] [ PubMed ] [ CrossRef ] [ Google Scholar ]
- Doire C. S. J., Brookes M., Naylor P. A. (2017). Robust and efficient Bayesian adaptive psychometric function estimation . Journal of the Acoustical Society of America , 141 ( 4 ), 2501 10.1121/1.4979580 [ PubMed ] [ CrossRef ] [ Google Scholar ]
- Gifford R. H., Shallop J. K., Peterson A. M. (2008). Speech recognition materials and ceiling effects: Considerations for cochlear implant programs . Audiology and Neuro-Otology , 13 ( 3 ), 193–205. 10.1159/000113510 [ PubMed ] [ CrossRef ] [ Google Scholar ]
- Green D. M. (1995). Maximum-likelihood procedures and the inattentive observer . Journal of the Acoustical Society of America , 97 ( 6 ), 3749–3760. 10.1121/1.412390 [ PubMed ] [ CrossRef ] [ Google Scholar ]
- Hagerman B., Kinnefors C. (1995). Efficient adaptive methods for measuring speech reception threshold in quiet and in noise . Scandinavian Audiology , 24 ( 1 ), 71–77. 10.3109/01050399509042213 [ PubMed ] [ CrossRef ] [ Google Scholar ]
- Hey M., Hocke T., Hedderich J., Muller-Deile J. (2014). Investigation of a matrix sentence test in noise: Reproducibility and discrimination function in cochlear implant patients . International Journal of Audiology , 53 ( 12 ), 895–902. 10.3109/14992027.2014.938368 [ PubMed ] [ CrossRef ] [ Google Scholar ]
- Hu W., Swanson B. A., Heller G. Z. (2015). A statistical method for the analysis of speech intelligibility tests . PLoS One , 10 ( 7 ), e0132409 10.1371/journal.pone.0132409 [ PMC free article ] [ PubMed ] [ CrossRef ] [ Google Scholar ]
- Keidser G., Dillon H., Mejia J., Nguyen C.V. (2013). An algorithm that administers adaptive speech-in-noise testing to a specified reliability at selectable points on the psychometric function . International Journal of Audiology , 52 ( 11 ), 795–800. 10.3109/14992027.2013.817688 [ PubMed ] [ CrossRef ] [ Google Scholar ]
- Kesten H. (1958). Accelerated stochastic approximation . The Annals of Mathematical Statistics , 29 ( 1 ), 41–59. 10.1214/aoms/1177706705 [ CrossRef ] [ Google Scholar ]
- King-Smith P. E., Rose D. (1997). Principles of an adaptive method for measuring the slope of the psychometric function . Vision Research , 37 ( 12 ), 1595–1604. 10.1016/s0042-6989(96)00310-0 [ PubMed ] [ CrossRef ] [ Google Scholar ]
- Kollmeier B., Warzybok A., Hochmuth S., Zokoll M. A., Uslar V., Brand T., Wagener K. C. (2015). The multilingual matrix test: Principles, applications, and comparison across languages: A review . International Journal of Audiology , 54 ( Suppl 2 ), 3–16. 10.3109/14992027.2015.1020971 [ PubMed ] [ CrossRef ] [ Google Scholar ]
- Kontsevich L. L., Tyler C. W. (1999). Bayesian adaptive estimation of psychometric slope and threshold . Vision Research , 39 ( 16 ), 2729–2737. 10.1016/s0042-6989(98)00285-5 [ PubMed ] [ CrossRef ] [ Google Scholar ]
- Kushner H. J., Yin G. (2003). Stochastic approximation and recursive algorithms and applications . Springer. [ Google Scholar ]
- Nilsson M., Soli S. D., Sullivan J. A. (1994). Development of the Hearing in Noise Test for the measurement of speech reception thresholds in quiet and in noise . Journal of the Acoustical Society of America , 95 ( 2 ), 1085–1099. 10.1121/1.408469 [ PubMed ] [ CrossRef ] [ Google Scholar ]
- Pedersen E. R., Juhl P. M. (2017). Simulated critical differences for speech reception thresholds . Journal of Speech, Language, and Hearing Research , 60 ( 1 ), 238–250. 10.1044/2016_JSLHR-H-15-0445 [ PubMed ] [ CrossRef ] [ Google Scholar ]
- Plomp R., Mimpen A. (1979). Improving the reliability of testing the speech reception threshold for sentences . International Journal of Audiology , 18 ( 1 ), 43–52. 10.3109/00206097909072618 [ PubMed ] [ CrossRef ] [ Google Scholar ]
- Polyak B. T. (1990). New stochastic approximation type procedures . Automation and Remote Control , 7 ( 2 ), 98–107. [ Google Scholar ]
- Polyak B. T., Juditsky A. B. (1992). Acceleration of stochastic approximation by averaging . SIAM Journal on Control and Optimization , 30 ( 4 ), 838–855. 10.1137/0330046 [ CrossRef ] [ Google Scholar ]
- Robbins H., Monro S. (1951). A stochastic approximation method . The Annals of Mathematical Statistics , 22 ( 3 ), 400–407. 10.1214/aoms/1177729586 [ CrossRef ] [ Google Scholar ]
- Ruppert D. (1988). Efficient estimations from a slowly convergent Robbins-Monro process (Technical Report No. 781). School of Operations Research and Industrial Engineering, Cornell University.
- Schwabe R. (1994). On Bather’s stochastic approximation algorithm. Kybernetika , 30 ( 3 ), 301–306. [ Google Scholar ]
- Schwabe R., Walk H. (1996). On a stochastic approximation procedure based on averaging . Metrika , 44 ( 1 ), 165–180. 10.1007/bf02614063 [ CrossRef ] [ Google Scholar ]
- Shen Y., Richards V. M. (2012). A maximum-likelihood procedure for estimating psychometric functions: Thresholds, slopes, and lapses of attention . Journal of the Acoustical Society of America , 132 ( 2 ), 957–967. 10.1121/1.4733540 [ PMC free article ] [ PubMed ] [ CrossRef ] [ Google Scholar ]
- Smits C., Houtgast T. (2006). Measurements and calculations on the simple up-down adaptive procedure for speech-in-noise tests . Journal of the Acoustical Society of America , 120 ( 3 ), 1608–1621. 10.1121/1.2221405 [ PubMed ] [ CrossRef ] [ Google Scholar ]
- Soli S. D., Wong L. L. (2008). Assessment of speech intelligibility in noise with the Hearing in Noise Test . International Journal of Audiology , 47 ( 6 ), 356–361. 10.1080/14992020801895136 [ PubMed ] [ CrossRef ] [ Google Scholar ]
- Terband H., Drullman R. (2008). Study of an automated procedure for a Dutch sentence test for the measurement of the speech reception threshold in noise . Journal of the Acoustical Society of America , 124 ( 5 ), 3225–3234. 10.1121/1.2990706 [ PubMed ] [ CrossRef ] [ Google Scholar ]
- Theelen-van den Hoek F. L., Houben R., Dreschler W. A. (2014). Investigation into the applicability and optimization of the Dutch matrix sentence test for use with cochlear implant users . International Journal of Audiology , 53 ( 11 ), 817–828. 10.3109/14992027.2014.922223 [ PubMed ] [ CrossRef ] [ Google Scholar ]
- Versfeld N. J., Daalder L., Festen J. M., Houtgast T. (2000). Method for the selection of sentence materials for efficient measurement of the speech reception threshold . Journal of the Acoustical Society of America , 107 ( 3 ), 1671–1684. 10.1121/1.428451 [ PubMed ] [ CrossRef ] [ Google Scholar ]
- Vroegop J. L., Dingemanse J. G., Homans N. C., Goedegebure A. (2017). Evaluation of a wireless remote microphone in bimodal cochlear implant recipients . International Journal of Audiology , 56 (9), 643--649. 10.1080/14992027.2017.1308565 [ PubMed ] [ CrossRef ] [ Google Scholar ]
- Wagener K., Brand T., Kollmeier B. (1999). Entwicklung und evaluation eines satztests für die deutsche sprache III: Evaluation des oldenburger satztests (Development and evaluation of a German sentence test Part III: Evaluation of the Oldenburg sentence test). Zeitschrift Audiologie , 38 , 86–95. [ Google Scholar ]
- Wong L. L. N., Keung S. K. H. (2013). Adaptation of scoring methods for testing cochlear implant users using the Cantonese Hearing In Noise Test (CHINT) . Ear & Hearing , 34 ( 5 ), 630–636. 10.1097/AUD.0b013e31828e0fbb [ PubMed ] [ CrossRef ] [ Google Scholar ]
- Zhang N., Liu S., Xu J., Liu B., Qi B., Yang Y., . . Han D. (2010). Development and applications of alternative methods of segmentation for Mandarin Hearing in Noise Test in normal-hearing listeners and cochlear implant users . Acta Otolaryngologica , 130 ( 7 ), 831–837. 10.3109/00016480903493758 [ PubMed ] [ CrossRef ] [ Google Scholar ]

IMAGES
COMMENTS
Speech threshold audiometry is the procedure used in the assessment of an individual's threshold of hearing for speech. There are differing opinions regarding the clinical utility of this measure.
Word recognition score = % of words discerned at threshold Speech discrimination = % single syllabic words identified and repeated at suprathreshold levels (generally 30 dB above SRT)
The topics that I will address today are an overview of speech threshold testing, suprathreshold speech recognition testing, the most comfortable listening level testing, uncomfortable listening level, and a brief mention of some new directions that speech testing is taking.
Your audiologist will ask you to repeat a list of words to determine your speech reception threshold (SRT) or the lowest volume at which you can hear and recognize speech. Then, the audiologist will measure speech discrimination — also called word recognition ability.
Speech Audiometry In addition to pure tone audiometry, speech audiometry is usually conducted as part of a comprehensive audiological evaluation. Two types of speech audiometry are usually performed: speech awareness or detection threshold (SAT or SDT) testing and speech reception threshold (SRT) testing.
In the previous issue of Hearing Loss Magazine, I provided an over-view concerning hearing threshold results as recorded on the audiogram and an explanation of the pure-tone audiogram. In this article, I will describe various test procedures that are typically administered in an audiometric evaluation and what information the tests provide.
About Speech Testing. An audiologist may do a number of tests to check your hearing. Speech testing will look at how well you listen to and repeat words. One test is the speech reception threshold, or SRT. The SRT is for older children and adults who can talk. The results are compared to pure-tone test results to help identify hearing loss.
Speech audiometry is an important component of a comprehensive hearing evaluation. There are several kinds of speech audiometry, but the most common uses are to verify the pure tone thresholds, determine speech understanding, and determine most comfortable and uncomfortable listening levels. The results are used with the other tests to develop a diagnosis and treatment plan.
Speech Reception Thresholds - Procedure and Application Speech Reception Thresholds - Procedure and Application: The speech reception threshold is the minimum hearing level for speech (ANSI, 2010) at which an individual can recognize 50% of the speech material. Speech reception thresholds are achieved in each ear. The term speech reception threshold is synonymous with speech recognition ...
Speech testing is the measurement of a patient's ability to hear and understand speech. The speech reception threshold (SRT) is the lowest decibel level at which a patient can correctly repeat 50% of test words. The speech threshold should be within ± 10 dB of the pure tone average at frequencies of 500, 1000, and 2000 Hz.
What is the Speech Reception Threshold (SRT)? Speech reception threshold (SRT) is a measure of hearing ability that is used to assess the lowest intensity level at which an individual can repeat familiar two-syllable words, known as spondee words, more than half of the time. Spondee words are chosen because they are easy to understand and are ...
Speech audiometry is an umbrella term used to describe a collection of audiometric tests using speech as the stimulus. You can perform speech audiometry by presenting speech to the subject in both quiet and in the presence of noise (e.g. speech babble or speech noise).
Word recognition Word recognition (formerly called speech discrimination) is the ability to repeat correctly an open set of monosyllabic words at suprathreshold intensity. Word lists are phonetically balanced (PB), meaning that the speech sounds used occur with the same frequency as in the whole language.
Speech audiogram Normal hearing and hearing impaired subjects. The speech recognition threshold (SRT) is the lowest level at which a person can identify a sound from a closed set list of disyllabic words.
Understanding Your Audiogram. Aging and Hearing Hearing Loss. The audiogram is a chart that shows the results of a hearing test. It shows how well you hear sounds in terms of frequency (high-pitched sounds versus low-pitched sounds) and intensity, or loudness. The audiogram shows results for each ear and tells the audiologist the softest sound ...
Overview Speech audiometry has become a fundamental tool in hearing-loss assessment. In conjunction with pure-tone audiometry, it can aid in determining the degree and type of hearing loss. Speech audiometry also provides information regarding discomfort or tolerance to speech stimuli and information on word recognition abilities.
The speech discrimination score (also called word recognition score) is the most commonly used test of supra-threshold speech perception. This is an important test in the audiological test battery, as it indicates the patient's ability to hear and understand speech at typical conversational levels.
5 of the best threshold Recognition or understanding of the speech stimuli does not occur until about 7 -9 dB above the level of detection Speech Recognition Threshold (SRT): It is the lowest hearing level (intensity) at which the patient can correctly RECOGIZE (REPEAT, PERCIVE) the speech stimuli 50 % of the time.
Speech-in-noise-measurements are important in clinical practice and have been the subject of research for a long time. The results of these measurements are often described in terms of the speech reception threshold (SRT) and SNR loss. Using the basic concepts that underlie several models of speech recognition in steady-state noise, the present study shows that these measures are ill-defined ...
Speech audiometry is performed to obtain the speech recognition (reception) threshold (SRT) or speech detection (awareness) thresholds (SDTs) using spondee (bisyllabic) words and suprathreshold speech recognition. The SRT measures the lowest dB HL at which a patient can correctly repeat or identify spondee 50% of the time, while the SDT ...
The starting level is 40 dB re: SRT (speech reception threshold). The starting level will be adjusted upward to obtain a level at least 5 dB above the threshold at 2000 Hz, if not above the patient's tolerance level. Present 25 words at 6 dB above and 6 dB below the starting level. If recognition performance improves less than 6%, then ...
Pure-tone audiometry is the main hearing test used to identify hearing threshold levels of an individual, enabling determination of the degree, type and configuration of a hearing loss [ 1][ 2] and thus providing a basis for diagnosis and management.
This study examines whether speech-in-noise tests that use adaptive procedures to assess a speech reception threshold in noise (SRT50n) can be optimized using stochastic approximation (SA) methods, especially in cochlear-implant (CI) users. A simulation ...