- Open access
- Published: 01 February 2017

Examining study habits in undergraduate STEM courses from a situative perspective
- Matthew T. Hora 1 &
- Amanda K. Oleson 2
International Journal of STEM Education volume 4 , Article number: 1 ( 2017 ) Cite this article
42k Accesses
37 Citations
15 Altmetric
Metrics details
A growing body of research in cognitive psychology and education research is illuminating which study strategies are effective for optimal learning, but little descriptive research focuses on how undergraduate students in STEM courses actually study in real-world settings. Using a practice-based approach informed by situated cognition theory, we analyzed data from 61 STEM students about their study habits.
Results indicate that studying is a multi-faceted process that is initiated by instructor- or self-generated cues, followed by marshaling resources and managing distractions, and then implementing study behaviors that include selecting a social setting and specific strategies. Variations in some study behaviors are also evident according to the timing of their studying (e.g., cramming), course level, discipline, and social setting. Three cases of individual student practices reveal how studying is also shaped by how the course is designed and taught, students’ own beliefs about studying, and aspects of their personal lives.
Conclusions
The results indicate that studying involves various social, digital, and curricular resources, that many students persist in utilizing low-impact study strategies (e.g., re-reading text), and that the use of study strategies varies across different situations. We suggest that the focus on changing teaching behaviors that is dominant within STEM education be broadened to include a focus on instructional design that supports student self-regulatory behaviors and the adoption of high-impact study strategies.
Graphical abstract
The stages of studying across three cases: Brianna, Larry, and Angelica.
As concerns mount regarding the quality of undergraduate education, particularly in the science, technology, engineering, and mathematics (STEM) fields, policymakers, educators, and student affairs professionals are increasingly focusing on how to support student learning throughout their academic careers. Given that students’ academic success is shaped by a complex matrix of psychological, cultural, and organizational factors, scholars are investigating a variety of issues that may impact student success including underlying psychological attributes such as engagement (Carini et al. 2006 ) and perseverance or “grit” (Duckworth et al. 2007 ), what instructors believe about teaching and learning (Hativa and Goodyear 2002 ), and the types of teaching methods used in the classroom (Freeman et al. 2014 ). However, while these areas of research shed light on key aspects of student learning, these foci overlook a key piece of the student learning puzzle—what students actually do when they leave the classroom and study.
A considerable body of literature exists on college student study skills and habits, with foci on students’ cognitive styles and approaches to learning (Biggs 1987 ; Riding and Cheema 1991 ), the use of specific study techniques (Karpicke et al. 2009 ) and the role of study habits and time spent studying on overall student achievement (Nonis and Hudson 2010 ; Robbins et al 2004 ). Investigating the nature of study habits is important because factors related to studying such as motivation and specific study techniques have been linked to academic success. In a meta-analysis of 72, 431 students, Credé and Kuncel ( 2008 ) found that motivation and study skills (e.g., time management) were positively associated with grade point average and grades in individual courses. Furthermore, a comprehensive review of research on specific study strategies found that some (e.g., distributed practice) led to learning gains whereas others (e.g., re-reading text) did not (Dunlosky et al. 2013 ) and that many college students are not employing these study habits (Hartwig and Dunlosky 2012 ), and understanding why students persist in using ineffective study practices and how to change this state of affairs, from a situative perspective, is of particular importance to the field of STEM education. Thus, knowing whether or not (and why) students are using these practices is important information for instructors and student affairs/academic advising professionals.
Yet for the field of postsecondary education in general, and STEM education in particular, relatively little is known about student study habits, largely due to the lack of robust descriptive research that accounts for students’ behaviors in real-world settings. The gaps in the literature are twofold. First, much of the research on studying is based on survey research or experimental studies of specific study strategies, with few qualitative, descriptive studies of how students actually study in real-world situations. Such an approach to research, that focuses on descriptive accounts of naturalistic behaviors in order to inform educational programming and reforms, is becoming increasingly important in research on reform implementation in both K-12 and postsecondary contexts (Hora 2016 ; Coburn and Turner 2012 ; Spillane et al. 2002 ). Second is view of study habits as decontextualized, not shaped by social, curricular, situation; given insights from situated cognition research on how activity and learning itself is “distributed, stretched over (and) not divided among” mind, tools, and social and organizational contexts (Lave 1988 , p. 1), and that decision-making and behavior cannot be properly understood without close attention to the naturalistic settings in which they unfold (Klein 2008 ), the reliance on decontextualized survey research for insights into study habits is no longer tenable.
In this exploratory study, we utilize a practice-based approach to focus on the actual study behaviors of 61 undergraduates at three research universities in the USA and Canada who were enrolled in biology, physics, earth science, and mechanical engineering courses. Drawing upon situated cognition theory to conceptualize studying as a behavior that encompasses individual study strategies as they unfold in specific social, technological, and institutional contexts, we analyze data using inductive thematic analysis from 22 focus groups, and these students provided detailed information about their study habits that allowed us to answer the following research questions: (1) What behaviors do students taking undergraduate STEM courses engage in when studying? (2) What underlying contextual factors, if any, influence these behaviors?
We pursued this line of research because while the question “How can we teach students if we do not know how they learn?” (Coffield et al. 2004 , p. 1) is important, we also wonder “How can we best support student success if we do not understand how they study?” Insights gleaned from the data presented in this paper, which indicate that studying is a complex, multi-dimensional practice that implicates cues, social resources, artifacts, and study strategies, can provide faculty and student affairs professionals with a new way to think about studying that extends the prior focus on specific, decontextualized study strategies.
Discussions regarding the state of undergraduate education in the early twenty-first century often focus on the role of the instructor and their pedagogical acumen in the classroom (e.g., Bok 2009 ). Indeed, much of the focus in the STEM education literature is on how to affect changes in faculty teaching practices and philosophies about student learning (PCAST 2012 ). While instructors certainly play an important role in facilitating student learning by crafting experiences that engage students in these ways (or not), researchers have long questioned whether enough attention has been placed on the other actor involved in the learning enterprise—the student. As Entwistle and Tait ( 1990 , p. 170) observed, student behaviors are “part of a broader academic environment which affects learning probably as much as, if not more than, the classroom skills of the lecturer.” According to this view, the student as an agent actively engaged in his or her own learning and overall experience in college is a central, if not primary, part of the teaching and learning equation that is too often overlooked.
Psychological approaches to understanding study habits and academic success
In early research on the change processes that young people undergo while in college (Pascarella and Terenzini 2005 ) and the factors that contribute to students’ lack of persistence (Tinto 1993 ), higher education scholars have paid particularly close attention to the psychological factors that shape students’ experiences and ultimate success (or lack thereof). For instance, attributes associated with academic success such as involvement (Astin 1984 ) and engagement have been used to explain students’ relative success in their academic coursework (Carini et al. 2006 ). An underlying assumption in this literature is that students’ mental stances or psychological attributes play a major role in their academic outcomes and that higher education professionals should support them by facilitating higher degrees of involvement and engagement to increase their prospects for success.
Another line of inquiry has focused on subconscious psychological traits associated with student learning including cognitive styles and approaches to learning (see Coffield et al. 2004 for a review). For example, researchers have argued that people have stable cognitive styles or “typical or habitual mode(s) of problem solving, thinking, perceiving, and remembering” that shape how they think and learn (Riding and Cheema 1991 , p. 194). Another commonly used construct is that of approaches to studying, which refers to more elastic, changeable approaches and preferences that learners have for studying and learning (Entwistle and Tait 1990 ). Early work in this area argued for the existence of two distinct approaches to learning whose basic outlines persist to the present time: deep approaches to learning that involve searching for meaning and surface approaches that involve rote memorization (Marton and Säljö 1976 ; Biggs 1987 ). While such approaches to learning are theorized as being relatively stable within an individual, they can change over time with concerted effort. Furthermore, these psychological attributes should not be considered as operating independently from the context in which studying occurs (Ramsden 1979 ). In fact, early research in this area found that some students actively sought information in the environment (e.g., textbooks, lecture content) and then studied using what was called “cue-seeking” behavior, whereas others were more “cue-deaf” or worked to succeed without seeking hints about exams (Miller and Parlett 1974 ).
This focus on the origins of student’s motivation to initiate studying is similar to a long-standing line of inquiry that examines the degree to which learners are able and willing to assume control of their own learning process or what is known as self-regulated learning (Zimmerman and Schunk 2001 ). A self-regulated learner engages in a process of initiating the learning process on their own, setting goals, identifying appropriate strategies, and reflecting on his or her own task performance—all of which ultimately leads to a decision to enact changes in future behaviors or to maintain current practices (Cassidy 2011 ). Self-regulated learning is a particularly valuable idea in college student success, with empirical research in this area indicating that students who exhibit high degrees of self regulation have higher rates of academic achievement as measured by persistence and grades (Boekaerts and Corno 2005 ).
Research on study habits and skills
Another body of literature that examines studying focuses directly on the study habits and skills that students utilize during the act of studying itself. However, what at first glance may appear to be a straightforward, easily defined term is operationalized in a variety of ways in the literature. For instance, Robbins et al. ( 2004 , p. 276) define study skills as “activities necessary to organize and complete schoolwork tasks and to prepare for and take tests” and operationalize the construct using measures including time management, leadership skills, communication skills, and the un-defined category of “study skills and habits” (see also Credé and Kuncel 2008 ; Lotkowski et al. 2004 ). Other scholars have defined study habits in different ways, including the ability to concentrate, the scheduling of regular review sessions, and hours spend studying (Nonis and Hudson 2010 ). Conceptualizing study habits in terms of time spent studying is rather common, and a widely cited report by Babcock and Marks ( 2010 ) found that hours spent studying has declined from 24 h a week in 1961 to 14 h a week in 2003. In 2009, the picture was bleaker, with over half of freshmen who took the Your First College Year Survey and over half of seniors who took the College Senior Survey spending 10 h or less per week studying or doing homework (Ruiz et al. 2010 ; Franke et al. 2010 ).
While these studies capture important facets of studying and the role that they play in student achievement and persistence, the specific strategies and actions students actually engage in during their study sessions remain obscured. Providing more clarity on specific study habits, Karpicke et al. ( 2009 ) found that the preferred study strategy of 84% of the surveyed undergraduates was re-reading textbooks and lecture notes. Unfortunately, a study examining the utility of 10 learning techniques in the empirical literature found that habits such as these considered low utility in regard their impact on student learning, in contrast to high-utility techniques such as practice testing and distributed practice (i.e., taking tests over time), thus suggesting that many undergraduates utilize study habits that are ineffective (Dunlosky et al 2013 ).
Given the ubiquity of the Internet and digital media in many people’s lives, researchers are also investigating how these artifacts are being used as study aids. In one study exploring student utilization of digital and “traditional” resources, researchers found that 39 and 44% of students search Wikipedia and Google, respectively, if they need help with coursework, with only 36% seeking out a faculty member (Morgan et al. 2012 ). Similarly, a 2010 study of 36,950 undergraduates found that 33% used wikis, 24% used video-sharing websites, and 12% used blogging tools (Smith and Caruso 2010 ). Besides these more traditional digital media, including course websites hosted on institutional learning management systems, some argue that other tools that facilitate personalized learning (Dabbagh and Kitsantas 2012 ) and digitally mediated social learning via open Internet-based resources (Seely Brown and Adler 2008 ) are under-utilized in higher education. Researchers are also examining how digital media can inhibit studying, however, and Rosen et al. ( 2013 ) found middle-school, high-school, and undergraduate students were unable to remain on task for even 6 min before being tempted by Facebook or texting when studying at home.
However, the literature on study skills, strategies, and habits is limited by a tendency to reduce the complex and multi-faceted behaviors that comprise studying to metrics that cannot capture how and why students study (i.e., hours spent studying) or focus on strategies (e.g., re-reading) at the expense of other possible behaviors or choices students make. Perhaps the single largest limitation, however, is the lack of attention paid to the contexts within which students actually study. While some scholars have focused on the environmental contexts of studying (Kuo et al. 2004 ) and the interaction among study habits and social factors (Treisman 1992 ; Robbins et al 2004 ), few recent studies have attempted to describe studying behaviors as a multi-faceted process that includes not only study strategies but also how situations and resources are implicated in these practices.
This is important because educational practice, whether a group of undergraduates studying for a biology course or an administrator finalizing a budget, should not be thought of solely in terms of an individual making decisions in isolation, as the context of decision-making as well as tools and other artifacts utilized as part of the process is critically important. In studying the practices of principals in K-12 settings, for example, researchers have utilized theoretical frameworks from situated and distributed cognition which assert that the institutional context is not a mere backdrop for activity but is instead an integral feature of individual cognition and decision-making as well as task performance itself (Halverson 2003 ; Hora 2012 ; Spillane et al 2002 ). In this study, we draw upon these frameworks to conceptualize studying as the discrete behaviors of individuals (e.g., reviewing notes) as they unfold within specific contexts and that implicate particular artifacts and resources.
Why does the lack of descriptive research on student study habits that adopt a situative perspective matter? Because fine-grained descriptions of people’s behaviors in specific contexts and situations illuminates the specific steps people take when solving problems or performing tasks—information that can then be used by instructors and educational leaders to improve their practices and design more locally attuned interventions (Coburn and Turner 2012 ; Spillane et al. 2001 ). Educational researchers across the K-16 spectrum have argued that more practice-based research should be conducted on the various behaviors associated with teaching and learning, so that how and why educators and students make decisions in “the wild” of schools, colleges, and universities can illuminate barriers and supports to effective practice, rather than simply prescribing how people should think and act regardless of the situation (Bastedo 2012 ). With such a comprehensive and multi-faceted approach to describing studying, we set out to document the study habits of 61 undergraduates taking STEM courses as a corrective to the focus on both teaching and study strategies alone, in the hopes that such accounts could inform ways that educators can improve student learning and academic success.
Exploratory research is intended to examine poorly understood phenomena and generate new insights and hypotheses that can guide future research on the topic (Slavin 2002 ; Stebbins 2001 ). In this exploratory study, we examine the study habits of a group of STEM students, with a focus on describing the lived experiences and subjective interpretations of individuals and groups or what cultural anthropologists call an “emic” account of social life (Merriam 2014 ). The study took place at three large, public research universities in the USA and Canada that had similar undergraduate populations (approximately 25,000 students). These sites were selected due to the presence of instructional reform initiatives, which was a criterion for the larger study on STEM instructors’ data driven decision-making upon which this analysis is based. The disciplines included in this study are biology, geology, physics, and mechanical engineering based on the STEM-related focus of the larger study. For this study, a non-random purposive sampling procedure was used to identify faculty study participants. Faculty were included in the study population if they were listed as instructors in each institution’s course listings for the 2013 spring semester. We contacted 165 instructors via email requesting their participation in the study, and 59 participated (36% response rate). Thus, the faculty whose classes were recruited into the study were unique in that they were self-selected and taught undergraduate STEM courses at large research universities.
These instructors represented the initial pool of courses from which we selected student participants for the focus groups. We selected the focus group technique in order to collect a large amount of qualitative, in-depth data in a shorter amount of time than would be possible with individual interviews (Bernard 2011 ). Of the 59 faculty who participated in the larger study, we asked 30 instructors they would recruit students for participation in focus groups, of which 22 instructors agreed. The 30 courses (and instructors) selected for recruitment represented the largest courses across all four of the disciplines included in the study, which increased the prospects of recruiting sufficient numbers of students. Those instructors sent email requests to their classes, and students contacted the research team if they were interested in participation. There was a $20 incentive, and 61 students participated (see Table 1 ).
Data collection
A team of four researchers conducted the student focus group interviews using a semi-structured interview protocol, with each group led by one or two moderators depending upon scheduling constraints. The key question posed to participants in the focus groups was: “Please imagine for a moment how you typically study for this course —can you describe in as much detail as possible your study situation?” This question was followed by probes regarding the types of materials used for studying, whether participants studied alone or with others, and any additional details not yet described. While the open-ended nature of the questions resulted in detailed observations about study practices, it also led to idiosyncratic accounts that were not always comparable across individuals. We also did not provide a definition for the act of “studying” during the focus groups, which was based on our goal of capturing students’ own unique perceptions about what behaviors and situations constituted a study session. Each focus group included between two and six students and lasted approximately 45 min. These focus groups were audio recorded and transcribed.
Data analysis
Transcripts were entered into NVivo qualitative analysis software and then segmented into manageable units or discrete statements by participants that encapsulated a single thought or idea (Gee 1986 ). First, a code list was created to segment the data that aligned with the research questions guiding the analysis. We were interested in segments related to “study strategies” and “study situations,” and thus, any utterances pertaining to these two categories were sought out. Both analysts reviewed five transcripts with these two codes in mind and highlighted text fragments related to both codes and then met to ensure a common understanding of the relationship between the codes and the raw data. Upon ensuring that the codes were being applied similarly, the second author then segmented the remainder of the dataset. Second, we followed a structured approach to grounded theory that involved using a combination of a pre-existing “coding paradigm” and the inductive analysis of transcripts to develop a code list with which to analyze the entire dataset. The second author developed a preliminary code list using an inductive open-coding approach where terms or ideas mentioned by study participants themselves (e.g., re-reading textbooks) were used to create code names (Glaser and Strauss 1967 ) while the research questions and theoretical framework were also kept in mind (Strauss and Corbin 1990 ). After developing the initial code list, we met to discuss the codes and revised them while reviewing text fragments and discussing the applicability of codes to the data. During this process, we attempted to derive codes that maintained as much fidelity to participants’ own language and descriptions of study behaviors as possible.
The second author then developed the final code list using the constant comparative method, where each occurrence of a code was compared to each previous instance of that code in order to confirm or alter the code and/or its definition (Glaser and Strauss 1967 ), after which the final code list was applied to the entire dataset. At this point in the analytic process, qualitative researchers have the option of reporting recurrent themes with or without numeric counts of their prevalence. In this paper, where all study participants responded to questions in a similar fashion (e.g., specific study strategies), we elected to report the number of times a code was applied to the raw data in order to convey to readers the frequency with which a particular behavior or observation was identified in the data. In other cases where responses were more ambiguous and/or where different respondents interpreted questions differently, we report recurrent themes instead of numeric counts.
The data were also entered into a data matrix with subjects as rows and study cues, resources, and strategies as columns. These data were analyzed using exploratory data reduction methods (i.e., hierarchical cluster analysis and multi-dimensional scaling) to see if patterns across the data could be discerned. Clear patterns were not discernable, so these data were then organized to report the frequency with which particular strategies were used according to different groups of students (e.g., discipline, social situation). The results reported in this paper depict the percentage of students within each group reporting each strategy, with results weighted according to the size of each respective group.
Next, we analyzed two students and one entire focus group who provided particularly rich details about their study habits in order to depict how studying unfolds in real-world settings at the individual level. These subjects were selected because of the level of detail they provided when self-reporting their conceptions of what studying means, the contexts in which their studying occurred, and their actual study behaviors. These case studies also highlight the situated nature of studying in the influence of peers, curricular artifacts, and other features of the environment on their study habits. Finally, we examined the resulting themes to explore any patterns in the data and identified a new way of thinking about studying that is reported in this paper.
Limitations to this study include the self-selected nature of the sample, both of the participating instructors and students, that limits generalizability of the findings to broader populations of undergraduates. Such limitations to generalizability are an inherent part of research using small, non-randomly selected samples, but their strength is in illuminating behaviors at a fine-grained level. While future research involving larger samples will be necessary to assess how widespread the behaviors reported in this paper truly are, the data do raise questions and considerations about studying that can be applied to different institutions. Another limitation is that the focus group method may introduce an element of self-censoring and social desirability bias by participants due to the public nature of the setting, which can result in incomplete or inaccurate answers to the facilitator’s questions. Finally, because participants discussed their studying with varying degrees of specificity it was difficult at times to ascertain whether similar behaviors were being reported. The limitations associated with social desirability and the veracity of students’ accounts could not be overcome with the current study, though future work should consider incorporating an observational component to corroborate self-reported behaviors with actual practice.
Before reporting data addressing the research questions guiding the study, we first discuss how respondents had differing notions of what activities constituted “studying.” For some, it meant any exposure to course material such as attending a class, whereas for others, studying implied completing assigned tasks. In yet other cases studying referred to activities that were not assigned and took place outside of class. As one student said, “I see studying more as something that I do separate from any assigned material.” In addition to these task-oriented conceptions, some reported “folk” theories of the learning or ideas about phenomena that are not necessarily grounded in evidence. For example, one student stated, “Studying to me means stressing out your brain so that it realizes that the information is significant.”
Thus, for the students in this study, “studying” was not easily distilled into a set of discrete strategies such as re-reading the textbook or hours spent engaged in discrete strategies. Further, as we discuss below, students’ views of studying also implicate a variety of strategies, social and physical settings, and resources as being involved in the studying process (Greeno 1998 ; Halverson 2003 ; Robbins et al 2004 ). Future research should delve more deeply into what students consider to be studying in terms of its physical, artifactual, and temporal boundaries. To maintain a consistent definition for this analysis, however, we defined studying as any interaction with course material outside of the classroom.
Cues to initiate studying and timing of study strategies
Prior to engaging in particular study activities, students frequently discussed why they started studying, which centered on the core idea of “cues” that trigger study behaviors. These cues were either provided by the instructor or were internally generated. While students were not explicitly asked about what cued their study sessions, descriptions of the study processes for many students provided information for this analysis. Another important aspect of these preliminary stages of studying is when students choose to study—either throughout the semester, several days before an assessment, or the day before a test or exam (i.e., cramming).
Instructor-generated cues
Throughout a given semester, 40 students reported that instructors often provided cues regarding when and what they should study. The most important cue for students tended to be the announcement of an upcoming assessment, thus initiating the process of studying. For some, an impending assessment was the only reason for studying. Similarly, instructors’ discussions about assessments (e.g., topics that would be covered) served as a primary rationale for some students to attend class. One participant said, “I go to class to (hear) the professor say this week on the exam you will see this subject or that subject.” Consequently, for some students, the classroom becomes a venue in which cues pertaining to assessments are sought and then applied to their studying.
Self-generated cues
Fewer (four) participants also discussed another cue for studying, that of recognizing that they were not sufficiently prepared or familiar with the course material. One participant explained that he studied after realizing that he did not understand a concept, which then set in motion a series of study behaviors that lasted until he felt conversant with the material. He said, “…and then I realize, ‘Oh man, I don’t understand pulleys so well,’ so last week I studied pulleys until I understood them.” Others reported a strong desire to learn certain skills and material so that they could reach their career goals.
Next, we discuss findings regarding when students reported engaging in study activities. For 11 respondents studying took place several days before an exam or test, while 14 reported waiting until the last day or even night before, popularly known as “cramming.” While the literature indicates that cramming is an ineffective way to study (e.g., Kornell 2009 ), and some students recognize its limitations (e.g., one student reported that after cramming “[the information] is not still in my brain”), this mode of preparation remains a common method. Finally, 15 respondents discussed studying throughout the term. In some cases, this practice was instigated by course-specific factors such as an instructor’s use of weekly quizzes, whereas in others, the student established a regular schedule of studying on their own.
Marshaling resources for studying
After discussing cues and timing for studying, the respondents then discussed collecting and utilizing a variety of resources with which to study. In describing students’ use of resources, we included references to commonly used tools such as course websites and textbooks as well as human resources that learners draw upon when studying. This represents a broader view of resources within organizations than is commonly used but captures knowledge and capabilities of instructors and staff within an educational organization (Gamoran et al. 2003 ). Understanding the resources used during studying is important because digital, print, and human resources and tools are used to enhance or even shape the studying act itself.
The resources discussed by the respondents included digital tools and media, print resources, and human resources, and those most commonly reported are depicted in Table 2 .
Digital resources
While the most commonly reported digital tool included laptops or desktop computers, we focus here instead on the applications used by students on these now ubiquitous resources for college students. The most widely reported resource was the course website (27 students), which operated on various learning management system (LMS) platforms. These websites were developed by instructors who posted a variety of learning resources including videotaped lectures, readings, practice exams, and course syllabi. One student described her professor’s course website as such, “So basically like any way you learn you can find it on [course website name] through all her resources and find a good way to study for you.”
The next most widely used digital resource included websites for seeking out new information including Google (24) and Wikipedia (13). These websites helped students expand upon lecture notes or clarify concepts or steps in solving problems. For example, one participant noted that in lecture, he listened for key words that could be included on exams and then looked them up online, because “With the Internet and Wikipedia you just need to know a few keywords and you can learn about anything.” Other resources included Facebook (9) which was used as an organizing tool and Youtube (5) for informational purposes. These results support prior research that found college students regularly utilize these online resources, even more so than their own instructors (e.g., Morgan et al. 2012 ).
However, the evidence suggests that technology also acts as a disruptive force in some students’ study habits. Nineteen respondents reported that some digital resources, usually cell phones and Facebook, regularly disrupted their studying yet they had no strategy for managing these distractions. One student noted, “[When studying] I look up sports stuff, any excuse not to be studying….at a computer I can just click on whatever I want.” To mitigate the potential distractions of the Internet or a buzzing phone, 21 students reported having developed strategies for managing distractions, often by deliberately removing them from their study “space.” The optimal studying situation for one student was in an isolated cubicle in the library basement with no cell phone reception, and he would turn off his laptop’s wireless Internet signal. In another case, a student went to her parent’s house on the weekends for a self-imposed “no devices zone” where her phone was confiscated so she could concentrate. Thus, digital resources can both enhance and detract from an individual’s studying, and students have varying degrees of success when it comes to managing the detrimental aspect of digital devices and media.
Print-based resources
Another type of resource that respondents regularly used was print-based resources such as textbooks (34) and lecture notes (33). Lecture notes took many forms including notes taken by student in class as well as notes and/or PowerPoint slides provided by the instructor, both of which were reported as important resources for studying. Another less utilized print resource discussed by five respondents was cue cards, which were mostly used to memorize key facts and formulas.
Human resources
The last type of resource reported by students pertained to the knowledge and content-expertise of people within their courses and/or departments. These included instructors (8) as well as teaching assistants (8) and tutors (4). In some cases, the participants reported approaching instructors or teaching assistants outside of class to obtain assistance with homework, upcoming or previous exams, and challenging concepts or problems. For students who were especially struggling with the course, tutors provided expertise and one-on-one instruction that these students viewed as an especially important form of academic support.
Setting and strategies
Next, we report data that speak to the studying process itself, particularly with whom students study and the specific strategies they employ.
The social setting in which studying occurs
When describing their actual study sessions, the respondents noted whether or not they studied alone or with others. For 39 respondents, studying was often a solitary affair. Some students noted that studying alone was an explicit strategy to reduce distracting conversations with others, while others stated that it was simply a habit. In contrast, 35 students described studying in groups. In these cases, the respondents stated that group-based studying was useful because peers could provide new insights or solutions. However, because 24 students reported studying both alone and in groups, depending on the proximity to an exam or the nature of the assignment, it is clear that for some students in the sample, the social setting in which studying occurred was rather flexible and not a fixed criterion or preference.
Employing specific study strategies
The studying process next involves the selection of specific strategies or techniques. While the participants often described these strategies using imprecise or idiosyncratic terminology such that it was often not possible to align them with those discussed in the literature (e.g., Dunlosky et al. 2013 ), it was possible to identify several core strategies utilized by this group of undergraduate students. In this section, we elaborate on the most commonly referenced strategies (see Table 3 ).
Thirty-eight participants re-read or reviewed course material or notes taken in class. This strategy was discussed as both a general practice that took place throughout the term as well as an initial step in preparing for exams. For example, one participant said that he re-read all of his lecture notes before working with old test materials “to try to understand what the professor had said fully” before attempting to take practice tests.
Given the broad conception of studying used in this analysis (i.e., any interaction students have with course material outside of class), we include the strategy of “doing homework” which 25 participants reported. As one participant put it: “My method of studying is pretty much to do any homework or review questions…” Homework also provided a litmus test of understanding—one participant explained how he learned a lot in class, but it really became clear when he answered the homework questions correctly.
While students reported reviewing lecture notes from class, this particular strategy involved 22 students creating their own artifacts such as cue cards, consolidating notes from different sources (some instructor-provided, others self-procured) into one set of notes, and so on. For example, one participant explained, “I write myself notes and everything is in my notes, including the textbook material and the prof’s slides and what the prof said or the stuff I found in Wikipedia or everything.” Others created study aids (e.g., games or cheat sheets) that were used throughout the term for study sessions.
Twenty participants reported reading the textbook in some capacity, either in full or in part, either assigned or unassigned, or they consulted the book when confronted with unfamiliar material. Often, the participants did not specify if they were re-reading, reading it for the first time, or if they were skimming. Importantly, the depth with which students read textbooks appeared to vary based on their intentions. In one case, a student explained, “Sometimes I just go through the chapter we’re going to go through in class and I just read all the captions for the images (to prepare for the lecture) so I know what we’re going to talk about and then afterwards I’ll read through the chapter.” In most cases, however, students spoke more ambiguously about reading.
Nineteen participants reported working with test materials provided by the instructor or students who had previously taken the course. One participant reported her routine as taking practice exams in a simulated test-taking environment, followed by an item-by-item analysis of her performance. Another talked about reviewing tests from previous years and randomly selecting problems to complete for practice. In both cases, the materials provided the students with an opportunity to monitor their level of understanding (or lack thereof) while also becoming attuned to the test-maker’s approach.
Working on problems was a strategy reported by 17 participants. Although ambiguous, the specific nature of the term “problems” likely refers to mathematical or computational problems given that many of these participants were enrolled in science or engineering courses. As one participant said, “I just find every single practice problem that I can get my hands on and do it.”
Eleven participants reported working on a variety of questions while studying. In one class, students worked on study questions or short essay prompts that review that day’s lecture. Further, instead of relying on practice exams, one student in that class reported, “I’ve found the best way to do well on the test is not to do all of her practice exams, but do (the) study questions.” Others reported working on end-of-chapter questions and completing discussion questions as an effective study strategy.
Taking quizzes related to course material outside of class was another method of studying reported by 11 participants. Sometimes the instructor provided the quiz to test comprehension after a reading assignment. One student who takes bi-weekly extra-credit quizzes provided by her instructor said, “I take them pretty seriously, I’ll prep a little bit before them even though they’re only five questions and if I get something wrong I’ll read (about it).”
Other factors influencing the study process
In addition to specific cues, resources, and study strategies, respondents also discussed various situations or factors that influenced their study behaviors.
Role of instructor in providing resources for studying
Student’s use of resources during their studying depends, in part, on the instructor and his/her provision of particular resources within the course. For example, some instructors provided their students with a variety of modalities and tools for learning (e.g., podcasts, supplementary readings, online lecture notes) that other students might not have had access to in other courses or with other instructors. These can be offered as in-class resources, or more commonly, embedded within the course’s website or LMS. Students can then select from the resources made available by their instructors, as well as resources that they find on their own, to construct their own unique study situation.
Course characteristics and discipline
The participants described how disciplinary content and course structure also influenced the strategies and resources they used. Some students perceived that different disciplines required different approaches to studying. One participant said, “You can’t study math how you would study biology, right?” The student followed up this observation by describing how studying for a math course entailed doing numerous problem sets, while a biology course required extensive reading, memorization, and understanding laboratory assignments. Other course characteristics that influenced teaching were the assessments and teaching methods used in the course. For instance, one student explained how her approach to preparing for multiple-choice exams emphasized a surface knowledge of selected topics: “Instead of looking at a topic and being able to discuss it for paragraphs at a time in like an essay format, I’ll try to memorize details that I feel are important.” Another respondent student noted that his studying “tends to match the style of the class” so that in a class taught with PowerPoint slides, his studying entails “a lot of time looking at slides,” whereas a more interactive class involves focusing on concepts and hands-on activities. This student’s approach to studying suggests that an instructor’s teaching style may have consequences for student learning not only through in-class comprehension of material but also by sending messages to students regarding the best way to study.
Personal situations and dispositions
The participants also alluded to personal factors that influenced their studying such as the lack of time due to heavy course loads and/or work schedules, family situations, and health-related issues. Additionally, the participants brought to a course pre-existing dispositions and experiences that influenced their approach to studying. One of these pertains to historic study habits from high school, where some students attempted to alter their “old” study behaviors to fit with the “new” expectations and demands of the university, while others simply continued using what had worked for them previously. Finally, student’s personal reasons for taking a course (e.g., to satisfy degree requirement, curiosity) also shaped how participants approached their studying.
Patterns in cues, resources, and strategies
Next, we sought to explore whether or not patterns in the data existed in regard to how cues, resources, and strategies were inter-related or not. Preliminary analyses using exploratory data techniques did not reveal discernable patterns, and no clear links were evident across the three primary components of studying identified in the data (i.e., cues, resource use, strategies). Instead, we chose to examine patterns in the use of study strategies considered effective in the literature (e.g., Dunlosky et al. 2013 ) according to two aspects of study behaviors (i.e., study timing, social setting) and two variables related to subject characteristics (i.e., course level and discipline). All analyses include weighted averages.
First, when looking at when studying occurs according to three groups of students (i.e., less than 1 week prior, cramming, throughout term), some data points stand out (see Fig. 1 ). Crammers review notes more often than others (94%), while those studying less than 1 week prior to exams use more textbooks (73%), study questions (55%), and video (36%) than other groups and those studying throughout the term or semester use problem sets (53%) more often than others. These data indicate that some variation in study strategies is evident depending on when students choose to study.
Selected study strategies by timing of study practices and social setting
Second, when organizing the data according to two groups (i.e., studying alone or studying in groups) differences in study strategies are also evident (see Fig. 2 ). Note that some students reporting doing both, hence, the large numbers in both groups that do not sum to 60 (42 and 36, respectively). Students studying alone tend to review notes (64%) and textbooks (52%) and also do practice tests (24%) and quizzes (19%) more than those studying in groups. In contrast, those studying in groups create study artifacts (47%), do problems (31%) and questions (31%), and use online materials such as video (14%) and the Mastering Physics/Anatomy videos (19%).
Third, when the data are organized according to the discipline of the course students were enrolled in at the time of data collection, additional points of variation are evident (see Fig. 3 ). Again, students may or may not be majors in these fields but discussed their study habits in relation to these disciplines. Students taking biology courses (26) report reviewing notes (69%) and textbooks (46%), doing practice tests (31%) and questions (35%), and reviewing videos (27%) more than students taking courses in other fields. Students in physics courses (11) reported creating artifacts (64%), doing problem sets (82%), and mastering resources (45%) more than others. Mechanical engineers and geology students did not report any study strategies more than other groups.
Selected study strategies by discipline and course level
Finally, the data indicate that study habits vary by course level, with students in upper division courses (18) reporting using certain study strategies more than those in lower division courses (43), including practice tests (33%), questions (39%), video (28%), and mastering physics or anatomy resources (33%). The students in lower division courses reported reviewing notes (58%), creating artifacts (37%), reviewing textbooks (51%), and doing problems (42%) and quizzes (16%) more than the students in upper division courses (see Fig. 4 ).
Case examples
Finally, to illustrate how each of these sets of findings is evident in students’ own real-world experiences, we present three in-depth analyses of students’ actual studying practices. The first two cases are those of individual students—Larry and Brianna—whose study behaviors reflect different sequences of decisions that link particular cues, resource use, and strategies. The final case is that of a group of five students in a single course (i.e., upper division anatomy and physiology). These cases illustrate how studying is a complex, idiosyncratic practice, while also being shaped by the social, institutional, and technological milieu in which students operate.
Larry. When we spoke with Larry he was studying for an upper level biology course that was required for his major. He first talked about his personal view of what studying means, stating that:
Studying to me means stressing out your brain so that it realizes that the information is significant. Basically, your brain can be lazy when it doesn’t think that something’s important and stressing it is what makes it retain information. So studying for exams is a lot about stressing your brain out.
This theory of how the brain and learning work thus set the stage for Larry’s subsequent study habits, which was a process that began in the classroom. There, he “frantically” wrote on the instructor’s PowerPoint slides that he printed off before class, labeling images, drawing arrows, and identifying mechanisms for cell signaling that were being discussed in class. Larry said that he did not necessarily understand the concepts at the time but took the notes down to reference later. In fact, it is not until he finds the time to sit in the library and read the relevant sections of the textbook that “it all comes together and finally makes sense.” While reading, he writes down key terms and their definitions in a notebook. For Larry, the library represented an important resource in his education because he does not own the book because he cannot afford it. Thus, he spent a lot of time in the library reading one of two copies on reserve. He also attended the optional recitation section for the course where he was able to speak with the instructor one-on-one and earn extra credit.
While he tried to study throughout the term, with his demanding course load and work schedule, he often only had time to study 3 or 4 days prior to an exam. Describing his study habits as “messy” and comprised of “lots of big stages,” Larry first gathered his notes from classroom sessions and his review of the textbook and then made flashcards for key concepts from the course. He also completed the end-of-chapter quizzes in the text and reviewed (and retakes) any old quizzes or exams from the course. The day before the exam, he tells himself “Wow Larry, you really have to get to it now,” and he sequestered himself at the library to review his notes and difficult concepts in the textbook and to re-watch videotaped lectures from the course website. All along, he deliberately studied alone because he had to maximize the limited time available for studying, such that he “cannot afford to sit around and have people talk about other stuff.” After several hours in the library reviewing these materials, Larry generally felt ready for the exam. Altogether, in Larry’s case, studying is an act that is instigated by instructor’s cues (i.e., upcoming exam), informed by a folk theory of the mind, involves a variety of curricular resources, and is strongly influenced by his personal situation.
Brianna. Next, we consider the case of Brianna who was enrolled in a lower division physics course when we met with her group. Her general approach to studying was to hope that the instructor was direct about expectations and guidelines because then, “the ball is in (her) court and (she) either learns the material or not.” Thus, Brianna was relatively self-motivated but relied on instructors to provide cues regarding when and how hard to study. This motivation is also sparked by her aspiration to attend medical school, which requires doing well on the Medical College Admission Test (MCAT). Brianna observed that “even if I get an A on a test but have no idea what is going on, it wouldn’t set me up to be in a good place for studying the MCAT in the future.”
In addition, she relied heavily on what she called her own preferred “learning style,” which centered on reading and re-reading text, whether it be the textbook or notes taken in class. Given her reliance on text and notes, Brianna observed that, “I pretty much show up to lecture just to write down what he’s saying.” The notes she took in class then became an important artifact for later studying, as she used them to create flashcards from her notes (and the textbook) and an outline for the course that is added to throughout the term. During her actual study sessions, Brianna either studied alone, reviewing her notes, scanning various digital resources, and doing practice problems, or with a group of friends in the library where she typically had on her headphones while surrounded by classmates who intermittently helped one another out on difficult problems.
Brianna’s use of digital technology is notable because she described online videos, the course website, the Internet, and social media as the “majority” of the resources she used to study. For example, she consulted free online tutoring videos (especially videos featuring one tutor in particular at the University of California at Berkeley), which helped her fill out her notes and summaries from the class and textbook. Overall she described the Internet as a “great resource” for finding course materials (e.g., slides, notes, exams, and videos) from other instructors teaching the same course at other colleges or universities. The questions these other instructors ask their students provides insights into what Brianna perceives her professor may ask, so she values their outsider’s perspective. Further, when doing her homework problems, she looked up the solutions online, even when she was confident about her answer. She does this to ensure that she is “approaching [the problem] in the right way” or to see if there are alternative methods to solving the problem. This, in turn, gives her a more “holistic grasp of the question.” Finally, in this and other courses, social media sites such as Facebook provided a place where she and her friends posted questions and shared approaches to different problems. Notably, most of the digital resources Brianna utilized were not part of the official course materials organized by her instructor and posted on the course website.
Thus, for Brianna studying involves a process of re-reading course materials and tools such as cue cards and digital media, largely in response to instructor’s cues about upcoming exams or homework. Driven by the desire to attend medical school introduced an element of motivation that made her take studying rather seriously.
Dr. Wells’ course. The final case is that of five female students taking an upper division anatomy and physiology course with Dr. Wells. In this course, which had an enrollment of 525 students across three sections, Dr. Wells had provided a rich array of learning resources on the course website that included weekly postings of videotaped lectures and PowerPoint slides, weekly practice questions, old exams, and links to other online resources. The students in the focus group noted that Dr. Wells did not simply post these resources and let students figure out how to utilize them but instead discussed in class how to use each tool and study with them. As Angelica noted, “She just does a really great job of giving us a lot of different ways to study.” Jacquie concurred, saying that while an online course she was taking was similarly well-resourced, “Dr. Wells provides more alternative methods to study which is what makes her course stand out.” Ultimately, in providing such a variety of resources for studying, Dr. Wells had crafted a learning experience that stood out for these students. For Bailey, who had little experience with the material, this was particularly important because “it’s really hard to stick your fingers in and get going,” and if you only have a textbook to work with, the entry points to the material are limited, often inaccessible and not particularly engaging.
In many ways, Dr. Wells was running a partially flipped classroom, in that students watched videotaped lectures online and came to a class that was highly interactive and engaging. During the class, Dr. Wells was constantly in motion, using her iPad to project slides on the screen while also writing and drawing using a stylus pen. Many questions were asked of students, including peer-based activities and small group discussions. Linda noted that Dr. Wells also emphasized important ideas across various formats such as clicker questions, practice tests, and study questions, such that “the repetition is awesome…even if you’re tired or distracted, eventually you’ll still get it.”
In terms of how these students actually studied in the course, weekly study questions (i.e., short essay questions that recap entire lectures) provided by Dr. Wells played an important role. For Jacquie, who said that she essentially crams before the exam—saying “well yeah, that’s why we study, for the exams”—her lecture notes, study questions, the mastering anatomy online resources, and old test materials were all utilized during study sessions. After discussing the course with her friends, however, she concluded that the best way to succeed in the course was “not to do all of her practice exams but to do those freaking (weekly) study questions.” Angelica said that “I noticed when I don’t do the study questions I don’t do very well.” This approach is similar to the technique of distributed practice, or regularly spaced testing of material over time, which is one of the high-impact study strategies identified by Dunlosky and colleagues ( 2013 ). Robin also spent 3–4 h after each class doing the study questions, along with a variety of other tools including the mastering anatomy activities, notes, lecture videotapes, and old test materials. In fact, while the group varied on the timing of their studying, all were cued by the instructor, used a variety of digital and print resources and between four and six study strategies. This reliance on multiple resources and study strategies is unsurprising given how Dr. Wells structured her course and guided her students in regard to studying. Within this learning environment, students then developed their own approach to studying but in ways strongly shaped by the resources and strategies Dr. Wells had embedded in the course structure.
The field of higher education in general and STEM education in particular continues to grapple with how to best facilitate learning, persistence, and retention throughout students’ postsecondary careers. Does the answer lie in changing teacher behaviors alone, such as the adoption of active learning techniques, structural responses such as reducing student debt and dealing with the rising price of college, or is success also dependent upon student attributes such as engagement and motivation? What these questions reveal is that students’ experiences in college are shaped by a variety of influences and that the intersection among policy, economics, organizations, and instruction provides a more accurate frame for thinking about student success than a search for a single “magic bullet” solution.
The same idea applies to thinking about the role that effective study strategies play in student learning. While the use of high-impact practices such as distributed practice is certainly a key ingredient in leading to student learning (Dunlosky et al. 2013 ), it is important to recognize that students’ adoption of these practices requires several antecedent conditions to be in place before this can happen. These include knowledge of these methods, time to study, access to the resources required to study in this manner, and so on. Similarly, studying is not simply about using strategies such as re-reading text or doing practice problems but is a process that involves cues about when to study, the timing of their actual study sessions, which resources to utilize, where to study, and which strategies to employ. How these stages unfold in practice are also shaped by a variety of factors such as a students’ personal life, the course material, and how instructors structure courses and make learning resources available. This is not to diminish the importance of high-impact study strategies but instead to point out that there are many steps taken by students to get to the point where they can sit down and utilize them with some regularity.
In the remainder of this paper, we discuss how this exploratory study contributes to the literature on college student study habits, particularly through the articulation of a multi-dimensional conception of studying that can provide instructors and administrators with a more nuanced account of how students engage in studying. In combination with the data reported in this paper as well as developments in educational technology and research, such an account also highlights the importance of instructional design that facilitates students’ use of high-impact strategies, diversified learning tools, and self-regulatory capabilities.
A new approach for thinking about undergraduates’ study behaviors
The results reported in this paper confirm and extend prior research on college student study habits. The data reinforce prior research that some of the most dominant study strategies utilized by students include reviewing notes and re-reading textbooks (Karpicke et al. 2009 ), utilize a variety of digital resources (Smith and Caruso 2010 ), and also rely on instructors to provide cues to begin studying (Miller and Parlett 1974 ). While the study described here is limited by a small sample size and lack of data on the impact of various study habits on learning outcomes, it was designed to shed light on fine-grained behaviors among a small group of students in order to advance our understanding of decision-making and action in specific social, organizational, technological contexts. In doing so, we extend the prior literature by offering an integrative multi-stage approach for thinking about study behaviors.
When interpreting the results from this exploratory study, we observed that students discussed their studying in terms of stages that began with cues to study and ended with their use of specific strategies. Along the way, they made decisions about who to study with and which resources to use, an account consistent with a situative theory of cognition, which posits that mental activity and social action is situated within specific socio-cultural and organizational contexts while also being distributed among mind, tool, and activity (Greeno 1998 ; Spillane et al. 2001 ). In other words, studying is not solely a matter of a “mind” sequestered with a book and highlighter pen, or a behavior that could be distilled into hours spent studying or the prevalence of a particular strategy, but instead involves people interacting with one another and various tools in specific situations (Seely Brown and Adler 2008 ). This is not to diminish the value of experimental work that does hone in on specific aspects of studying such as how students self-pace their study or time spent on specific tasks (Bjork, Dunlosky and Kornell 2013 ) but instead is an argument that a broader perspective of the act of studying itself is also useful.
Consequently, based on the data presented in this paper, we suggest that a new way of thinking about studying is warranted that includes the following components: (1) recognizing the situation and detecting cues to initiate studying, (2) marshaling resources and managing distractions (or not), (3) selecting a time and social setting to study, and then selecting specific strategies, and (4) engaging in a period of self-reflection. We illustrate this approach using the three cases reported earlier in the paper (see Fig. 1 ).
Some caveats are necessary when interpreting this figure. First, while the stages of cue detection and timing, resource use, and strategies are based on data from this paper, the self-regulative period is not. Instead, it is included as a post-assessment phase of reflection and commitment that the literature indicates is an important aspect of learning (Zimmerman and Schunk 2001 ). Second, we do not claim that all 61 participants in the study progressed through each of these steps, but instead that this conception of studying captures the broad range of behaviors and experiences students reported engaging in during a recent study session. As a result, we are not suggesting that this account of studying is generalizable to all students but is a heuristic device for thinking about studying in a more multi-dimensional manner than is common at the present time.
Thus, we argue that conceptions of the act of "studying" extend beyond a focus on discrete, decontextualized factors such as hours spent studying or the use of specific strategies (e.g., re-reading text). In making this argument, we highlight the importance of ecological validity when thinking about study habits in general and interpreting laboratory-based research in particular. In other words, understanding how findings from the literature about "effective" study habits may vary according to disciplinary, social, institutional, or personal situations will be important for future work in the area. We also suggest that a more multi-dimensional conception of studying can also be a useful interpretive framework for educators, instructional designers, and administrators to begin thinking more broadly and strategically about how their courses are designed (or not) to foster effective study habits. By recognizing that studying involves multiple states, resources, strategies and actors, it becomes necessary to move beyond simply providing “how-to” guides for studying or recommendations for students to use high-impact practices to instead think about the role that cue-seeking, resource acquisition, and distraction management play in shaping students’ study habits. With a more situative view of studying in mind, it is possible to consider how the course as a whole creates an environment that prompts particular study behaviors, such as Dr. Wells’ provision of various learning tools via her LMS that prompted students to study with them. Thinking of studying in these terms, in the remainder of this paper we highlight ways that educators can facilitate or support effective studying and learning practices: fostering self-regulated learning and using principles from instructional design to encourage high-impact studying.
Fostering self-regulated learners
One of the most pressing issues facing educators is the fact that many students continue to utilize ineffective study practices, such as re-reading textbooks or cramming the night before an exam. Informing some low-impact practices are “faulty mental models” (p. 417) about how memory and learning work, such as the view that information can be recalled and played back like a recording (Bjork, Dunlosky and Kornell 2013 ). Instead, the retrieval process involves reconstructing knowledge from various stored memories, is heavily dependent on specific cues, and that upon cueing information in memory becomes reinforced. Essentially, students need to understand that in order to create a library of information in their minds that is easily accessed and retained over the long term requires a “meaningful encoding of that information” which involves integrating information into a network of connected ideas and then regularly practicing retrieval of that information (Dunlosky et al. 2013 ).
Besides becoming more sophisticated learners and theorists about how the mind works, it is clear that students can also benefit from more guidance about how to more effectively study and learn. Educational psychologists argue that becoming a more adept learner is not simply about amassing tips and strategies about how to study but is based on becoming what is known as a self-regulated learner, which is the “self-directive process by which learners transform their mental abilities into academic skills” (Zimmerman 2002 , p. 65). Self-regulation is not just a quality or personal aptitude, however, but is best thought of as a sequence of states that include forethought (i.e., plans for studying), performance, and self-reflection. Motivation to initiate studying on one’s own is important, but perhaps more critical is the self-monitoring of performance, especially the ability to scrutinize and interpret failure and make corrections (Boekaerts and Corno 2005 ). Furthermore, while considerable barriers exist for students to develop self-regulative habits, such as a belief that intelligence is “fixed” and not malleable (Yeager and Dweck 2012 ) and assumptions that learning should be simple and unproblematic (Bjork, Dunlosky and Kornell, 2013 ), helping students to develop this aptitude is critical because it is a core aspect of success not only in school but also in life and the workplace (Pellegrino and Hilton 2012 ).
So how, if at all, can STEM educators embed self-regulatory skills into their courses? Setting aside for the moment the extent to which self-control, goal setting, and responsibility should be learned in the home, grade school, or various other cultural fields during childhood, the fact remains that it is possible to teach some aspect of self-regulatory competencies in the college classroom (Nilson 2013 ). One strategy is to create a classroom environment with high expectations and a low- to zero-tolerance policy for irresponsible behavior or late assignments, thereby encouraging if not forcing students to set goals for themselves and achieve them. Another strategy is the widely used instructional wrapper, which refers to prompts for students to reflect on their performance before and after an assignment or activity, which trains students to regularly reflect on their study habits and approach to learning (Lovett 2013 ). Other ideas include modeling learning strategies such as self-monitoring and summarizing in front of students in what is known as a “cognitive apprenticeship” (Palincsar and Brown 1984 ), using small group work tasks designed to spark self-regulation (Fitch et al. 2012 ) and assigning open-ended tasks and assessments requiring students to choose strategies and take control of their learning (Boekaerts and Corno 2005 ).
We conclude this discussion about self-regulation with a note about digital media. While online resources and digital devices can play an important and productive role in facilitating student learning (Dabbagh and Kitsantas 2012 ; Smith and Caruso 2010 ), it is evident from the data that they can detract from focused study. Thus, another aspect about self-regulation is the willingness and ability to remove digital distractions when they are not serving a productive purpose, such as the students in this study who deliberately went to libraries without wireless Internet or parents’ homes where devices were confiscated. As will be discussed in the next section, technology can and should be part of educators’ instructional toolkit, but students would be well served by adopting more self-regulated stances when it comes to the presence of technology in their study sessions.
Encouraging personalized learning and high-impact studying through course structure
Next, we turn to issue of how instructors, through the deliberate design of their courses, can facilitate effective study habits. Here, we focus on two aspects of effective studying: the use of multiple representations and modalities and the use of high-impact strategies. In both cases, we can look to the example of Dr. Wells’ course where she embedded within the structure of the course itself opportunities for students to draw upon various learning tools while also imposing a high-impact study habit (i.e., distributed practice) via weekly practice questions.
First, providing students with a variety of learning resources and tools offers them a variety of entry points with which to explore the material. The rationale for doing so is not to support students’ distinct learning styles, an idea that is popular but unsupported by the empirical evidence (Pashler et al. 2008 ), but instead is based on the fact that learners that engage with varied representations of an idea or concept demonstrate improved learning outcomes (Pellegrino and Hilton 2012 ). In addition, the provision of various learning tools is useful because today’s learners are broadly proficient in developing personalized learning pathways, whether for academic or personal purposes, using online resources and social media (Dabbagh and Kitsantas 2012 ). In doing so, many create social learning environments, or what some call “participatory cultures,” where people develop online learning communities where they collectively create, share, and learn from each other (Jenkins et al. 2006 ). Essentially, the idea is to make available a repertoire of learning tools and media for students that they can then select from to approach the material from multiple perspective and according to their own unique way of engaging with different learning modalities.
The second approach for facilitating effective study habits pertains to the structure of a course, from the timing and nature of assessments to the types of learning activities students are required to do. Again, consider the example of Dr. Wells, who embedded within her course an assessment strategy that forced her students to engage in the high-impact study habit of distributed practice. Through weekly practice questions, students were required to regularly take mini-exams on different topics (Dunlosky et al. 2013 ). Spacing out study sessions on distinct topics enhances learning through the spacing effect and also by introducing comparisons or “interference” across topics, which results in higher-order representations or complex mental models that not only are repositories of information but also facilitate transfer and retention (Bjork, Dunlosky, and Kornell 2013 ).
Similarly, the role of course structure in facilitating student learning has been well documented in STEM education, where pre-class reading quizzes and weekly practice exams have been linked to improved student learning (Freeman et al. 2011 ), and even in reducing the achievement gap between white and under-represented minority students, because highly structured courses with regular practice may introduce study and learning skills to students with little experience from high school (Haak et al. 2011 ). The takeaway here is that as educators, we can design our courses and teach our classes with explicit attention towards creating (and mandating) situations for students to engage in certain study habits.
The attention currently being placed on STEM instructors and their pedagogical acumen as key facilitators of student learning is well-placed, but the relationship between teaching and learning is anything but direct, linear, and unproblematic. What students decide to do in terms of when and how to study act as critical intermediaries between what instructors do in the classroom and students’ ultimate performance in college. As Entwistle and Tait suggested over 25 years ago, ( 1990 , p. 170), students’ behaviors and strategies “affects learning probably as much as, if not more than, the classroom skills of the lecturer.” On this point, there are both promising and troubling signs. While students are increasingly utilizing varied resources and media in a deliberate and creative manner to advance their studies, some study methods that are demonstrably ineffective continue to be widely used. The exploratory study reported in this paper offers a new, multi-dimensional way to think about studying that suggests future research directions exploring undergraduate study habits including similar descriptive research with larger samples and additional disciplines, experimental research focusing on specific strategies under different conditions (e.g., resource use, cues for studying), and examining the relationship between course structure and studying.
Ultimately, students must take responsibility for their learning and strategize ways to create situations—whether in a quiet library basement or a group study session online—where they can effectively study and learn. However, postsecondary educators must also be cognizant of the pressures facing today’s college students and the fact that many have not been taught how to engage in high-impact study habits but instead rely on re-reading highlighted text. One of the guiding principles for instructional design should be the idea that it is no longer tenable to assume that students have been taught how to effectively study and learn prior to their matriculation into a college or university. While students bring a wealth of new learning habits and technological acumen to the twenty-first century classroom—whether online or face-to-face—they still need guidance in how to study. This conclusion, however, should not lead to complaints about unprepared students or a failed K-12 sector but instead needs to spark postsecondary educators to carefully design of rich and engaging learning environments that sparks self-regulatory habits of mind and encourages high-impact studying, so that students are well positioned to succeed.
Astin, A. W. (1984). Student involvement: a developmental theory for higher education. Journal of College Student Development, 40 (5), 518–529.
Google Scholar
Babcock, P., & Marks, M. (2010). Leisure college, USA: the decline in student study time. American Enterprise Institute for Public Policy Research, 7 , 1–7.
Bastedo, M. N. (2012). Organizing higher education: a manifesto. In M. N. Bastedo (Ed.), The organization of higher education: managing colleges for a new era (pp. 3–17). Baltimore: The Johns Hopkins University Press.
Bernard, H. R. (2011). Research methods in anthropology: qualitative and quantitative approaches (5th ed.). Lanham: Altamira Press.
Biggs, J. (1987). Student approaches to learning . Hawthorn: Australian Council for Educational Research.
Bjork, R. A., Dunlosky, J., & Kornell, N. (2013). Self-regulated learning: Beliefs, techniques, and illusions. Annual review of psychology, 64, 417–444.
Article Google Scholar
Boekaerts, M., & Corno, L. (2005). Self-regulation in the classroom: a perspective on assessment and intervention. Applied Psychology, 54 (2), 199–231.
Bok, D. (2009). Our underachieving colleges: a candid look at how much students learn and why they should be learning more . Princeton: Princeton University Press.
Carini, R. M., Kuh, G. D., & Klein, S. P. (2006). Student engagement and student learning: testing the linkages. Research in Higher Education, 47 (1), 1–32.
Cassidy, S. (2011). Self-regulated learning in higher education: identifying key component processes. Studies in Higher Education, 36 (8), 989–1000.
Coburn, C. E., & Turner, E. O. (2012). The practice of data use: an introduction. American Journal of Education, 118 (2), 99–111.
Coffield, F., Moseley, D., Hall, E., & Ecclestone, K. (2004). Learning styles and pedagogy in post-16 learning: a systematic and critical review . London: Learning Skills Network.
Credé, M., & Kuncel, N. R. (2008). Study habits, skills, and attitudes: the third pillar supporting collegiate academic performance. Perspectives on Psychological Science, 3 (6), 425–453.
Dabbagh, N., & Kitsantas, A. (2012). Personal learning environments, social media, and self-regulated learning: a natural formula for connecting formal and informal learning. The Internet and higher education, 15 (1), 3–8.
Duckworth, A. L., Peterson, C., Matthews, M. D., & Kelly, D. R. (2007). Grit: perseverance and passion for long-term goals. Journal of Personality and Social Psychology, 92 (6), 1087–1101.
Dunlosky, J., Rawson, K. A., Marsh, E. J., Nathan, M. J., & Willingham, D. T. (2013). Improving students’ learning with effective learning techniques: promising directions from cognitive and educational psychology. Psychological Science in the Public Interest, 14 (1), 4–58.
Entwistle, N. J., & Tait, H. (1990). Approaches to learning, evaluations of teaching, and preferences for contrasting academic environments. Higher Education, 19 (2), 169–194.
Fitch, T., Marshall, J., & McCarthy, W. (2012). The effect of solution-focused groups on self-regulated learning. Journal of College Student Development, 53 (4), 586–595.
Franke, R., Ruiz, S., Sharkness, J., DeAngelo, L., & Pryor, J. (2010). Findings from the 2009 administration of the College Senior Survey (CSS): National aggregates. Chicago: Higher Education Research Institute at the University of California Los Angeles.
Freeman, S., Haak, D., & Wenderoth, M. P. (2011). Increased course structure improves performance in introductory biology. CBE-Life Sciences Education, 10 (2), 175–186.
Freeman, S., Eddy, S. L., McDonough, M., Smith, M. K., Okoroafor, N., Jordt, H., & Wenderoth, M. P. (2014). Active learning increases student performance in science, engineering, and mathematics. Proceedings of the National Academy of Sciences, 111 (23), 8410–8415.
Gamoran, A., Anderson, C. W., Quiroz, P. A., Secada, W. G., Williams, T., & Ashman, S. (2003). Transforming teaching in math and science: how schools and districts can support change . New York: Teachers College Press.
Gee, J. P. (1986). Units in the production of narrative discourse. Discourse Processes, 9 , 391–422.
Glaser, B. G., & Strauss, A. L. (1967). The discovery of grounded theory: strategies for qualitative research . New Brunswick: Aldine Transaction.
Greeno, J. G. (1998). The situativity of knowing, learning, and research. American psychologist, 53 (1), 5–26.
Haak, D. C., HilleRisLambers, J., Pitre, E., & Freeman, S. (2011). Increased structure and active learning reduce the achievement gap in introductory biology. Science, 332 (6034), 1213–1216.
Halverson, R. (2003). Systems of practice: how leaders use artifacts to create professional community in schools. Educational Policy Analysis Archives, 11 (37), 1–35.
Hartwig, M. K., & Dunlosky, J. (2012). Study strategies of college students: are self-testing and scheduling related to achievement? Psychonomic Bulletin & Review, 19 (1), 126–134.
Hativa, N., & Goodyear, P. (Eds.). (2002). Teacher thinking, beliefs, and knowledge in higher education . Norwell: Kluwer Academic Publishers.
Hora, M. T. (2012). Organizational factors and instructional decision-making: A cognitive perspective. The Review of Higher Education, 35(2), 207–235.
Hora, M. T. (2016). Navigating the problem space of academic work: How workload and curricular affordances shape STEM faculty decisions about teaching and learning. AERA Open, 2(1), 1–19.
Jenkins, H., Clinton, K., Purushotma, R., Robison, A. J., and Weigel, M. (2006). Confronting the challenges of participatory culture: media education for the 21 st century. Chicago: The John D. and Catherine T. MacArthur Foundation.
Karpicke, J. D., Butler, A. C., & Roediger, H. L., III. (2009). Metacognitive strategies in student learning: do students practice retrieval when they study on their own? Memory, 17 (4), 471–479.
Klein, G. (2008). Naturalistic decision making. Human Factors, 50 (3), 456–460.
Kornell, N. (2009). Optimising learning using flashcards: spacing is more effective than cramming. Applied Cognitive Psychology, 23 , 1297–1317.
Kuo, J., Hagie, C., & Miller, M. T. (2004). Encouraging college student success: the instructional challenges, response strategies, and study skills of contemporary undergraduates. Journal of Instructional Psychology, 31 (1), 60–67.
Lave, J. (1988). Cognition in practice: mind, mathematics and culture in everyday life . Cambridge: Cambridge University Press.
Book Google Scholar
Lotkowski, V. A., Robbins, S. B., & Noeth, R. J. (2004). The role of academic and non-academic factors in improving college retention . ACT Policy Report, Retrieved from http://ww.cfder.org/uploads/3/0/4/9/3049955/the_role_of_academic_and_non_academic_factors_in_improving_college_retention.pdf .
Lovett, M.C. (2013). Make exams worth more than the grade: using exam wrappers to promote metacognition (pp. 18-52), in Using reflection and metacognition to improve student learning: across the disciplines, across the academy, ed. Kaplan, M, Silver, N., LaVague-Manty, D., & Meizlish, D. Sterling, VA: Stylus Publishing.
Marton, F., & Säljö, R. (1976). On qualitative differences in learning: I. Outcome and process. British Journal of Educational Psychology, 46 , 4–11.
Merriam, S. B. (2014). Qualitative research: a guide to design and implementation . San Francisco: John Wiley & Sons.
Miller, C. M., & Parlett, M. R. (1974). Up to the mark: a study of the examination game . London: SRHE.
Morgan, G., Moskal, P., Wolf, A., Dziuban, C., McMartin, F., & Morrill, J. (2012). Understanding student use of digital learning resources. [Powerpoint slides]. Retrieved from http://bit.ly/1vjZRsY
Nilson, L. (2013). Creating self-regulated learners: strategies to strengthen students self-awareness and learning skills . Sterling: Stylus Publishing.
Nonis, S. A., & Hudson, G. I. (2010). Performance of college students: impact of study time and study habits. The Journal of Education for Business, 85 , 229–238.
Palincsar, A. S., & Brown, A. L. (1984). Reciprocal teaching of comprehension-fostering and comprehension-monitoring activities. Cognition and Instruction, 1 , 117–175.
Pascarella, E., & Terenzini, P. (2005). How college affects students: a third decade of research . San Francisco: Jossey-Bass.
Pashler, H., McDaniel, M., Rohrer, D., & Bjork, R. (2008). Learning styles concepts and evidence. Psychological science in the public interest, 9 (3), 105–119.
Pellegrino, J. W., & Hilton, M. L. (Eds.). (2012). Education for life and work: developing transferable knowledge and skills in the 21 st century . Washington, DC: National Academies Press.
President’s Council of Advisors on Science and Technology (2012). Report to the President. Engage to excel: Producing one million additional college graduates with degrees in science, technology, engineering and mathematics. Washington, D.C.: Executive Office of the President.
Ramsden, P. (1979). Student learning and perceptions of the academic environment. Higher Education, 8 (4), 411–427.
Riding, R., & Cheema, I. (1991). Cognitive styles—an overview and integration. Educational Psychology, 11 (3-4), 193–215.
Robbins, S. B., Lauver, K., Le, H., Davis, D., Langley, R., & Carlstrom, A. (2004). Do psychosocial and study skill factors predict college outcomes? A meta-analysis. Psychological Bulletin, 130 (2), 261–288.
Rosen, L. D., Carrier, L. M., & Cheever, N. A. (2013). Facebook and texting made me do it: media-induced task-switching while studying. Computers in Human Behavior, 29 , 948–958.
Ruiz, S., Sharkness, J., Kelly, K., DeAngelo, L., & Pryor, J. (2010). Findings from the 2009 administration of the Your First College Year (YFCY): national aggregates. Los Angeles: Higher Education Research Institute at the University of California Los Angeles.
Seely Brown, J., & Adler, R. P. (2008). Open education, the long tail, and learning 2.0. Educause Review, 43 (1), 16–20.
Slavin, R. E. (2002). Evidence-based education policies: transforming educational practice and research. Educational researcher, 31 (7), 15–21.
Smith, S. D., & Caruso, J. B. (2010). Key findings: the ECAR study of undergraduate students and information technology . Boulder: EDUCAUSE Center for Applied Research.
Spillane, J. P., Halverson, R., & Diamond, J. B. (2001). Investigating school leadership practice: a distributed perspective. Educational Researcher, 30 (3), 23–28.
Spillane, J. P., Reiser, B. J., & Reimer, T. (2002). Policy implementation and cognition: reframing and refocusing implementation research. Review of Educational Research, 72 (3), 387–431.
Stebbins, R. A. (2001). Exploratory research in the social sciences. Qualitative research methods in the social sciences, 48 . Thousand Oaks: Sage Publications.
Strauss, A., & Corbin, J. M. (1990). Basics of qualitative research: grounded theory procedures and techniques . Thousand Oaks: Sage Publications, Inc.
Tinto, V. (1993). Leaving college: Rethinking the causes and cures of student attrition. (2nd ed.). Chicago: University of Chicago Press.
Treisman, U. (1992). Studying students studying calculus: a look at the lives of minority mathematics students in college. The College Mathematics Journal, 23 (5), 362–372.
Yeager, D. S., & Dweck, C. S. (2012). Mindsets that promote resilience: when students believe that personal characteristics can be developed. Educational Psychologist, 47 (4), 302–314.
Zimmerman, B. J. (2002). Becoming a self-regulated learner: an overview. Theory into Practice, 41 (2), 64–70.
Zimmerman, B. J., & Schunk, D. H. (Eds.). (2001). Self-regulated learning and academic achievement: theoretical perspectives . Hillsdale: Lawrence Erlbaum Associates.
Download references
Acknowledgements
The authors would also like to thank Jana Bouwma-Gearhart and Jennifer Collins for their involvement in this study and collecting data reported in this paper.
This research was supported by a grant from the National Science Foundation (DUE#1224624) for the Tracking the Processes of Data Driven Decision-Making Study ( http://tpdm.wceruw.org ).
Authors’ contributions
AO participated in the design of the study and data collection, led the data analysis, and collaborated with MH to draft the manuscript. MH conceived of the study, led the design, participated in data collection and analysis, and finalized the manuscript. Both authors read and approved the final manuscript.
Competing interests
The authors declare that they have no competing interests.
Author information
Authors and affiliations.
Department of Liberal Arts and Applied Studies, University of Wisconsin-Madison, 21 N. Park St., Madison, WI, 53715, USA
Matthew T. Hora
Wisconsin Center for Education Research, University of Wisconsin-Madison, Madison, USA
Amanda K. Oleson
You can also search for this author in PubMed Google Scholar
Corresponding author
Correspondence to Matthew T. Hora .
Rights and permissions
Open Access This article is distributed under the terms of the Creative Commons Attribution 4.0 International License ( http://creativecommons.org/licenses/by/4.0/ ), which permits unrestricted use, distribution, and reproduction in any medium, provided you give appropriate credit to the original author(s) and the source, provide a link to the Creative Commons license, and indicate if changes were made.
Reprints and permissions
About this article
Cite this article.
Hora, M.T., Oleson, A.K. Examining study habits in undergraduate STEM courses from a situative perspective. IJ STEM Ed 4 , 1 (2017). https://doi.org/10.1186/s40594-017-0055-6
Download citation
Received : 01 August 2016
Accepted : 06 January 2017
Published : 01 February 2017
DOI : https://doi.org/10.1186/s40594-017-0055-6
Share this article
Anyone you share the following link with will be able to read this content:
Sorry, a shareable link is not currently available for this article.
Provided by the Springer Nature SharedIt content-sharing initiative
- Learn Management System
- Study Strategy
- Study Session
- Digital Resource
- Medical College Admission Test
The challenge of change: understanding the role of habits in university students’ self-regulated learning
- Open access
- Published: 10 April 2024
Cite this article
You have full access to this open access article
- Louise David ORCID: orcid.org/0000-0003-1973-4568 1 ,
- Felicitas Biwer ORCID: orcid.org/0000-0003-4211-7234 1 ,
- Rik Crutzen ORCID: orcid.org/0000-0002-3731-6610 2 &
- Anique de Bruin ORCID: orcid.org/0000-0001-5178-0287 1
2685 Accesses
23 Altmetric
Explore all metrics
Study habits drive a large portion of how university students study. Some of these habits are not effective in fostering academic achievement. To support students in breaking old, ineffective habits and forming new, effective study habits, an in-depth understanding of what students’ study habits look like and how they are both formed and broken is needed. Therefore, in this study, we explored these aspects among first-year university students in six focus group discussions ( N = 29). Using a thematic analysis approach, we clustered the data in five themes: Goals Matter , Balancing Perceived Efficiency and Effectiveness when Studying , Navigating Student Life: from Structured Routines to Self-Regulation Challenges , the Quest for Effective Habits with Trying to Break Free From the Screen as subtheme, and the Motivation Roller Coaster . Findings suggest that students had different study habits depending on their goals. Students had quite accurate metacognitive knowledge about effective learning strategies for long-term learning, but often used other learning strategies they deemed most efficient in reaching their goals. Students indicated intentions to change, but did not prioritize change as their current habits enabled them to pass exams and change was not perceived as adding value. Fluctuations in motivation and transitioning to a self-regulated life hampered students’ intentions to form new and break old habits. Next to insights into factors affecting students’ behavioral change intentions, the findings suggest the importance of aligning assessment methods with life-long learning and supporting students in their long-term academic goal setting to prioritize study habits which target lasting learning to optimally foster their self-regulated learning.
Similar content being viewed by others
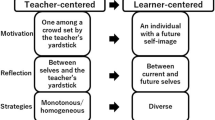
Does changing from a teacher-centered to a learner-centered context promote self-regulated learning: a qualitative study in a Japanese undergraduate setting
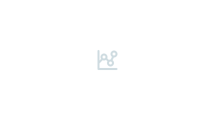
Using multiple, contextualized data sources to measure learners’ perceptions of their self-regulated learning
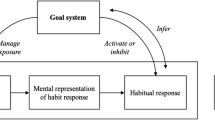
The Science of Habit and Its Implications for Student Learning and Well-being
Explore related subjects.
- Artificial Intelligence
Avoid common mistakes on your manuscript.
Introduction
In higher education, students are required to plan, monitor, and execute their learning autonomously (Dresel et al., 2015 ; Zimmerman, 1986 ). Therefore, effective self-regulated learning (SRL), facilitated by the use of effective learning strategies, is essential for students’ academic achievement and lifelong learning (e.g., Dunlosky et al., 2013 ). However, many students struggle to use effective learning strategies optimal for long-term learning, such as practice testing, and often rely on passive strategies such as re-reading (Morehead et al., 2016 ; Rea et al., 2022 ). While training programs are successful in increasing students’ knowledge regarding these effective learning strategies, many students struggle to sustainably change their behavior and apply these learning strategies (Biwer et al., 2020a , b ; Foerst et al., 2017 ; Rea et al., 2022 ). This gap between students’ knowledge and behavior is partially due to students’ strong habits of using ineffective study strategies (Blasiman et al., 2017 ; Rea et al., 2022 ). However, so far, this gap between students’ knowledge and behavior and their study habits remained largely unexplored. To explore the gap between students’ knowledge and behavior further, we deem it essential to first gain a thorough understanding of students’ current study habits, how they usually form new, and break old habits.
Using a qualitative approach, we add to the current field of research by targeting an in-depth exploration of students’ study habits, which is currently lacking. Insight into these are essential to tailor educational strategies and training to better align with students' behaviors and needs. Awareness of potential struggles students might encounter when breaking old or forming new habits provides important insights into hurdles students face when attempting to modify their study behavior and how they can be supported in achieving their goals.
Self-regulated learning in higher education
In higher education, learning is happening mostly in teacher-absent environments, which requires students to effectively self-regulate their learning (Dresel et al., 2015 ). However, many students struggle to self-regulate effectively. They monitor and control their learning inaccurately, which has a negative impact on their academic achievement (Hartwig & Dunlosky, 2012 ). One way of fostering self-regulated learning is by helping students to use learning strategies which are optimal for long-term learning (e.g., “desirably difficult” learning strategies, Bjork & Bjork, 2011 ). Examples of desirably difficult learning strategies are practice testing, interleaving items of various categories, and spacing learning sessions over time (Dunlosky et al., 2013 ). A shared commonality of these effective strategies is that they require an active learning process with repeated memory retrieval of information (Bjork & Bjork, 2011 ).
While essential for self-regulated learning, students often avoid desirable difficulties or fail to use them in the long-run, even when being aware of their benefits (Biwer et al., 2020a , b ; Rea et al., 2022 ). One reason for not using effective learning strategies is that students often have strong habits of using surface processing strategies. Instead of using desirable difficulties, students rely on passive learning strategies and mass their study sessions close to the exam instead of spacing the sessions over time (Blasiman et al., 2017 ; Dembo & Seli, 2004 ; Foerst et al., 2017 ). Often students start using these strategies in high school already (Dirkx et al., 2019 ).
Next to struggling to use effective learning strategies, students often face additional regulation issues during their self-study. Learners also need to employ resource management strategies such as effort and motivation management to optimize learning conditions (Dresel et al., 2015 ) by, for example, planning their study sessions or asking for help if necessary. While resource management strategies have been identified as an essential factor for academic performance (Grunschel et al., 2016 ; Waldeyer et al., 2020 ), many students struggle with time management (Basila, 2014 ; Thibodeaux et al., 2017 ) and encounter motivational problems leading to for example procrastination (Grunschel et al., 2016 ).
Metacognition and resource-management strategies are often indicated as important factors in self-regulated learning models (for an overview, see Panadero, 2017 ). However, these factors might not fully explain how students maintain recurring study behaviors over the long term (Fiorella, 2020 ). Instead, habits, which, once formed, are usually not guided by conscious intentions and goals, have been suggested crucial for academic behaviors that need to be repeated consistently over an extended period (Fiorella, 2020 ). Therefore, to foster students’ self-regulated learning, it has been suggested to support students in building new study habits, which, for example, incorporate desirable difficult learning strategies (Fiorella, 2020 ).
Behavioral change and study habits
In the last years, interventions have been developed to enhance students’ knowledge and initial use of effective learning strategies (e.g., Ariel & Karpicke, 2018 ; Biwer et al., 2020b ; Broeren et al., 2021 ). While showing positive effects, the actual transition of this knowledge and application to students’ self-study in the long run remain limited (Dignath & Veenman, 2021 ). The majority of these interventions focus more on increasing students’ knowledge and less on building new habits or changing the actual study behavior consistently potentially explaining the limited application. Habits, however, are an important predictor of behavior in addition to, for example, attitudinal and control beliefs (Verhoeven et al., 2012 ; Verplanken, 2018 ), and are seen as a key factor in behavior maintenance (Rothman et al., 2009 ). To ultimately support students in actually applying effective learning strategies in the long run, it is essential to first understand the factor that drives most behavior: habits (Rothman et al., 2009 ; Verplanken, 2018 ).
Habits are behaviors that reoccur in stable contexts. Once a habit forms, behavior is led by automatic, effortless actions instead of deliberate intentions (Gardner, 2015 ; Lally, et al., 2010 ). According to our conceptualization, study habits can relate to behaviors such as the usual length of a student’s study session, learning strategies used, or study timing and environment. Study habits can play an essential role in harming or helping students to achieve long-term academic goals. Irrespective of whether these habits help or hinder goal achievement, they initiate behavior automatically and effortlessly. Habits usually form when an association between a certain behavior and context is established by consistently repeating a behavior in that context (Gardner, 2015 ; Lally & Gardner, 2013 ). Due to the association, the habitual behavior is automatically and sometimes unconsciously activated (Aarts & Dijksterhuis, 2000 ) while alternative behaviors become less accessible (Danner et al., 2007 ). Often, the effort required to initiate the habitual behavior decreases over time and initiation becomes effortless (Lally et al., 2011 ). Even when intentions change, engagement in habitual behavior commonly persists (Adriaanse & Verhoeven, 2018 ).
According to the goal–habit interface model (Wood & Rünger, 2016 ), many habits are initiated by goal-directed behavior. Initially, before habit formation, goals drive individuals to repeat a certain action in a specific context. Once a habit is formed, the habitual behavior shifts from goal-dependent to goal-independent behavior. In this case, behaviors are enacted based on context cues and irrespective of goals (Mazar & Wood, 2018 ). Nevertheless, as many individuals are unaware of this habit formation process, they might misattribute their habitual behavior to goals or intentions instead of habits (Loersch & Payne, 2011 ; Mazar & Wood, 2018 ).Wood & Neal ( 2016 ) suggest various essential components for habit-based interventions that target initiation and maintenance of health behavior change. They suggest the importance of habit-forming approaches for fostering repeated engagement in healthy behaviors while also disrupting undesirable behavior by habit-breaking approaches. As main components for habit formation, they suggest frequent repetition of the desirable behavior, creating a re-occurring context and context-cues, and administering rewards randomly (Wood & Neal, 2016 ). To break undesirable habits, context-cue disruption, re-structuring of one’s environment, and monitoring one’s behavior are suggested as efficient (Wood & Neal, 2016 ).
While there has been quite some research showing the beneficial effects of habits in health behavior change (e.g., exercising habit, healthy eating habit), only a few studies have focused on how study habits could improve students’ study behavior (e.g., Galla & Duckworth, 2015 ). Students’ ineffective study habits such as ineffective learning strategy use (Biwer et al., 2020a , b ; Foerst et al., 2017 ; Rea et al., 2022 ), high smartphone use (Chen & Yan, 2016 ; Lepp et al., 2015 ), or poor time management (Basila, 2014 ; Thibodeaux et al., 2017 ) seem to play a role in their study behavior and academic achievement. Breaking these ineffective habits and forming effective study habits instead by, for example, incorporating more desirably difficult learning strategies could help students to optimize their self-regulated learning and academic achievement.
In the present study, we aim to gain a better understanding of the nature of university students’ study habits, how students usually form new habits, and how they (try) to break ineffective habits. We explored this using a qualitative approach via focus group discussions with first- and second-year university students. As our goal was to dive beyond surface-level observations and gain an in-depth understanding of students’ experiences rather than generalizing to a larger population, a qualitative approach seemed most appropriate (Morse, 2008 ). Compared to a quantitative approach, a qualitative approach via focus groups or interviews offers various benefits (for a more general overview of focus group discussions in medical education, see Stalmeijer et al., 2014 ). First, they offer a relatively time- and cost-effective access to students’ thoughts and experiences. Second, by being able to directly engage with students, it is possible to ask students for further elaboration or clarification, which would not be possible using for example questionnaires and therefore ensuring an in-depth understanding. Third, due to the flexibility of this approach and our semi-structured question guide, we were able to capture students’ opinions while limiting imposing pre-existing assumptions about their study habits. Most importantly though, we anticipated that the interactions between students would be essential when discussing study habits and focus group discussions would thus allow a more diverse and multifaceted picture of students’ study habits. While habitual behaviors could be unconscious, we expected that hearing other students’ experiences would facilitate students’ reflections on similar or dissimilar experiences. Insights from our study can help to inform potential future study habit interventions to overcome students’ knowledge-behavior gap.
Research setting
This study was conducted at the Faculty of Health, Medicine, and Life Sciences (FHML) at Maastricht University, which is a public research university in the Netherlands. At Maastricht University (UM), the academic year is divided into six different blocks (i.e., teaching periods), including different thematic courses lasting between 4 and 8 weeks. Courses usually consist of lectures and tutorial sessions and are finalized with an exam. The tutorial sessions are structured according to the problem-based learning (PBL) approach. Within this approach, authentic scenarios are central to learning (Dolmans et al., 2005 ). In small tutorial groups, ranging from 10 to 12 students and one tutor, students are introduced to these scenarios according to a seven-step model (Moust et al., 2005 ). The first five steps, (1) term clarification, (2) problem definition, (3) brainstorming explanations, (4) structuring and analyzing the identified explanations, and (5) formulation of learning goals, take place during the tutorial session. Students then individually study literature to answer their learning goals during (6) self-study. Lastly, during a next tutorial session, students integrate and discuss findings from their self-study in a (7) post-discussion.
Admission to the medical program at UM is a selective procedure, with only limited places available. Students are only eligible if having followed a certain combination of STEM courses during high school. Furthermore, potential candidates have to undergo two selection rounds. In the first selection round, students’ high school transcripts, curriculum vitae, and a written assignment are evaluated. If of sufficient quality, candidates are invited to a second selection round, which is an assessment day at the university, where potential candidates have to fulfill various assignments during which their cognitive and (inter)personal characteristics and skills are assessed. Based on these criteria, students are ranked and selected. Admission to the biomedical program at UM was not selective for the cohort participating in this study. The only eligibility criterion was that students had followed a certain combination of STEM courses during high school and were proficient enough in English.
The majority of first-year FHML students at UM are assigned to a mentor and receive a formal learning strategy training in their first weeks (“Study Smart”; Biwer & De Bruin, 2023 ) to support their self-regulated learning and professional development. The learning strategy training consists of three 90-min small-group training sessions with different goals. In the first session, the main goal is to increase students’ awareness about effective and ineffective learning strategies, why certain strategies work better, and possibly misleading experiences that students might encounter when using (in)effective learning strategies. During the second session, students are asked to try-out different effective learning strategies and think about a concrete plan, how they could incorporate these during their self-regulated learning. During the third session, which is held a few weeks after the previous sessions, students are asked to reflect on their learning strategy use during their self-regulated learning and how they could improve. Throughout the year, students meet with their mentor and write a portfolio reflecting on their competencies related to program content, professional- and study behavior.
Participants
Twenty-nine first- and second-year medicine ( n = 14) and biomedical sciences ( n = 15) bachelor students participated in one of six focus group discussions. Students were on average 20.1 years old (14% male). Students were recruited via multiple channels. The first author contacted the mentor and course coordinators of the respective bachelor programs to share the study information with their students. Additionally, students were recruited via posters and approached after tutorials and lectures. During the recruitment phase, the study goal was advertised as exploration of students’ study methods and habits. We obtained written informed consent from all participants prior to the study. Participants completed a demographic questionnaire inquiring about their age, study program and year, and grade point average (GPA). Students’ GPA ranged from 5.8 to 9, with the mean GPA being 7.4 (on a scale from 1 to 10, with 10 being the highest). Two students did not indicate their GPA. The faculty’s ethical review board (FHML-REC/2022/049) approved the study. Students received a small monetary reimbursement to compensate for their participation.
Focus groups
Students who completed the demographic questionnaire and indicated their availability were invited to the focus group discussions at university. The focus groups lasted approximately 80 min ( M = 78.4 min, SD = 4.2 min) and were moderated and observed by the first and second author. Based on a semi-structured focus group guide (see supplementary materials), students were asked which study methods they usually used during their self-study, what a typical study day looked like, and about their experiences with forming and breaking habits. The clarity of the questions within the focus group guide was piloted with two students prior to the focus group discussions. Based on their feedback, the wording of the questions was slightly adjusted to improve clarity. The first four focus groups were held at the end of students’ first academic year. Two additional focus groups were held at the beginning of students’ second bachelor year. The focus group guide was adjusted before the second part of data collection as we noticed that students had difficulties reflecting in-depth on their experiences with forming and breaking habits. Therefore, we created three vignettes describing concrete examples of how a habit was broken or formed based on students’ reflections during the first focus groups. Data was collected iteratively and occurred simultaneously with data analysis. In line with Morse ( 2015 ), as soon as we estimated our data to be sufficiently rich to gain a deep understanding of students’ study experiences and their habit-breaking and habit-forming experiences, we determined data saturation and stopped data collection. Data saturation is commonly an interpretive judgment in thematic analysis (for a critical stance regarding the concept “data saturation,” see Braun & Clarke, 2019 ). In our case, we started data analysis by descriptive line-by-line coding of the so far collected transcripts, clustering these codes, and searching for patterns, simultaneously to data collection. Based on the understanding we gained during these first analytic steps, we determined to have collected sufficient data to understand students’ experiences after six focus groups.
Data analysis and coding procedure
All focus groups were audio recorded and transcribed verbatim. Based on the thematic analysis approach (Braun & Clarke, 2006 ), we analyzed the focus group discussions inductively. Our analysis was data-driven and thus, the theme development did not mirror our question guide nor do the themes fully align with our potential pre-existing assumptions about students’ study habits (Braun & Clarke, 2006 ; Kiger & Varpio, 2020 ). We used pre-existing theories to establish our focus group guide and kept this knowledge as sensitizing concepts during the coding procedure (Bowen, 2006 ). We conducted a semantic analysis of participants’ accounts by interpreting their explicit meanings as recorded and transcribed. After thoroughly listening to the audio recordings and reading the transcripts in-depth multiple times, they were descriptively coded line-by-line using Atlas.ti (version 23.0.7.) by the first author (an extract of our codebook is included in the supplementary materials). Using these descriptive codes, the second author coded 50% of two transcripts without insights into the first coder’s line-by-line coding. The outcomes and coding alignments of these partial transcripts were discussed and definitions of codes were further specified, if necessary. Then, patterns within and across focus groups were discussed within the whole research team based on summaries of the transcripts and clustering of the descriptive codes done by the first and second author. Based on this discussion, the first and second author formulated themes, which were reviewed and refined with the entire research team. Additionally, the formulated themes were checked against the coded data and original transcripts. Throughout the analytic procedure, our research team engaged in regular discussions and asked for feedback from scholars outside of the research team during roundtable sessions and conference presentations to critically examine interpretations and findings. We followed a pragmatist approach in analyzing the data, prioritizing the practical relevance of knowledge instead of attempting to explain “reality” (Cherryholmes, 1992 ). The pragmatist approach offered us the flexibility and adaptability of addressing our research questions as well as generating practical implications from the identified themes while interpreting the data within our specific context.
Reflexivity statement
LD is a PhD student who completed a Bachelor’s degree at Maastricht University and is therefore familiar with the UM system also from a student perspective. FB is an educational psychologist with research interests in self-regulated learning and teacher professionalization and knows the PBL system in their role as mentor and tutor. RC is a cognitive psychologist with research expertise in behavior change and technology. AdB is an educational psychologist with research expertise in self-regulated learning in higher education. RC and AdB know the PBL system in various roles (student, teacher, mentor). We are aware that the findings of this study are co-created by interactions between the authors and participants. We thoroughly discussed each process step with all team members and discussed intermediate findings with educational scientists outside of the team and continuously reflected on the extent to which our individual personal experiences and beliefs shaped the research project.
We clustered students’ experiences into five themes: Goals Matter , Balancing Perceived Efficiency and Effectiveness when Studying , Navigating Student Life: from Structured Routines to Self-Regulation Challenges , the Quest for Effective Habits with Trying to Break Free From the Screen as subtheme, and the Motivation Roller Coaster .
Goals matter
Students mentioned various goals, which influenced their study behavior. Many students had a clear short-term goal of passing the exam in mind. For them, studying during the course served the goal of “collecting all the information” (student studying medicine (MED), focus group (FG) 2) to prepare for tutorial sessions. Studying for the exam served the goal of “actual studying” (student studying biomedical sciences (BMS), FG2), during which information is memorized or learned. Students experienced more pressure when studying for an exam compared to studying during the course. Approximately 1.5 weeks before an exam, students started intense studying. During this period, they dedicated extensive time to studying, often sacrificing a work-life balance and reducing time for activities like exercising, socializing, and hobbies.
Other students indicated having clear long-term academic goals such as receiving a high GPA or wanting to become a good doctor. These clear long-term goals helped them to study consistently, be less exam-oriented, and prioritize their studying also during the course, even if having to sacrifice other activities. Instead of studying solely to pass exams, students explained to be more consistent in their study behavior and tried to understand and learn the content continuously. They mentioned achieving this by planning and spreading their study load throughout the course. For example, one student mentioned:
I don’t study for exams anymore, I study because I want to be a good doctor. And I think that mindset also helped me change. […]. So it’s harder to find time around [studying], but I also prioritise [studying], university is the most important thing for me. If it means I don’t go to a party or I don’t go to the gym, which I also want to do, then that’s just how it is. (Student MED, FG5)
Balancing perceived efficiency and effectiveness when studying
Students generally had quite accurate metacognitive knowledge and were aware that active learning strategies and distributed learning sessions were more effective for long-term learning. Contrastingly, students did not necessarily use those strategies as they experienced that their own study strategies “work” (multiple students in multiple FGs) and were most efficient in reaching their short-term goal to pass the exam or having a strong habit of using them. For example, one student mentioned:
Well, I know it’s not really useful. But I still [summarize] a lot. But I know that it’s […] been proved that it’s not really effective. But I still, I don’t know, it’s just a habit. […] And it works. (Student MED, FG 3)
Students differed in which strategies they deemed to “work” (multiple students in multiple FGs) for themselves. For tutorial preparations, students usually read and summarized the provided sources. For exam preparations, students used various active and passive learning strategies such as re-reading, summarizing, creating mind maps, watching videos, recalling information, testing themselves by using past exams or creating flashcards, and explaining content to others or themselves. The amount of practice testing students used during their self-study depended on the availability of past exam questions in their study program as students experience creating practice questions themselves as too time consuming:
Some of our professors, they made […] really extensive kind of quizzes with lots of questions. And that was really helpful. I wish they did that for everything. (Student BMS, FG6)
To optimize their study efficiency, next to using cognitive learning strategies, students tried various resource management strategies, such as creating a planning for their tasks or studying with friends to stay motivated but often faced challenges in accurately managing their time and effort. Students usually started their study session by creating a planning or to-do list, but it was difficult to accurately know how long a task would take or to “really stick to it” (Student BMS, FG3). Instead of studying until a certain time, students usually studied until the task was finished or they could not focus anymore.
Navigating student life: from structured routines to self-regulation challenges
Students reported basing their study habits on what they had learned during high school, despite university being experienced as different. Next to the content being perceived as more in volume and difficulty, students also mentioned having to adjust to the change of setting. During high school, students often had a structured daily routine. When transitioning to university, they had to adjust to the lack of structure and external control, but also higher flexibility associated with student life. While students appreciated this increased freedom, it also came with the challenge of managing themselves and self-regulating various aspects of life: starting to study, moving to a new city, looking for new hobbies and friends, or managing their household.
When I lived with my parents in high school […], you have like a whole routine. […]. Now you can do things whenever you want to do it. And […] sometimes you have parties on Monday or on Wednesdays. So, it’s on random days. Yeah. And then you’re tired on Tuesdays. So, then you don’t do as much as other days. […] I think, it’s also part of student life. (Student MED, FG2)
Some students solved the lack of structure by actively creating more external structure in their daily life by joining committees, making appointments with others, or creating a clear separation between work and “personal” life. For other students, the lack of external structure seemed to negatively influence their willingness to form habits as they experienced habits as restricting flexibility. Some students appreciated the flexibility that they associated with student life and did not report actively looking for more structure. In search for more structure, students differed in their habit and preference of study location. Some students preferred to study at home to avoid distractions, whereas others preferred to study at university as they found it easier to initiate studying with others around and appreciated a separation between their studying and living space. During courses, students were more flexible in their study setting.
The quest for effective habits
Even though students experienced their current strategies to “work” for the exam, they reported intentions to change their current study behavior. These intentions targeted improving time management, such as following their planning, procrastinating less, cramming less, limiting screen time, and changing their sleeping schedule. They mentioned making study appointments with others, which helped to feel more accountable, and promoted adherence to intended behavior. Students also mentioned wanting to add steps to their current study habits such as completing their study notes or creating flashcards after each tutorial. For example, a student mentioned “I first had to […] talk about this topic, answer the question, then I was allowed, for example, to go to the bathroom” (student BMS, FG4) or working through flashcards when traveling. While students had intentions to change, they mentioned not necessarily prioritizing working on realizing their intentions since their current habits were sufficient to pass their exams but also encountering difficulties when trying to realize them. Students identified various reasons for this, for example, difficulties in creating a feasible planning, following their intention when not being motivated, or falling back into old habits when experiencing a setback. While having good intentions, students perceived change to be effortful, time-consuming, or being insecure about whether a change would actually lead to an improved outcome. For example, one student mentioned:
It’s not enough to have motivation to change that one point. Because it’s what you say, how do you make the change? By doing it daily. […]. That’s, you know, what I’m trying to do. Like be more consistent. […]. I’ll do great for the first couple of days, maybe two weeks, if I was really motivated. But then after I wake up on a bad day, I really don’t feel like it. And it’s this boring topic. I’ll push it back. […]. I’ll do something else. Then time runs out. Then we’re already kind of back in the bad cycle of procrastinating on everything. […] If there’s not the stress of a deadline, then it comes down purely to motivation every day. (Student BMS, FG3)
Trying to break free from the screen
One particularly challenging habit students tried to break was their smartphone habit. Students mentioned turning to their phones or study unrelated websites automatically when lacking focus or procrastinating. This was experienced as distracting and negatively influencing students’ studying. They tended to spend more time on their phone than anticipated, for example, if wanting to briefly check something. To reduce this automatic use, students tried to discontinue their habit by re-structuring their environment so that the distraction would be less accessible or interchanging their phone use with another activity such as reading. Instead of having their phone on the table when studying, they put their phone out of sight, blocked or deleted apps in the morning, or exchanged their phone with a friend’s phone. Even though students used various strategies to reduce the distraction from these devices, students continuously struggled in limiting their screen time.
I am so done with my phone being the hugest distraction in my life at this point, it just annoys the hell out of me. I’m actually breaking a habit right now, it’s called ‘no Instagram anymore’. Yes, it’s very bad, but I am... I’m not going to say I am improving because I am not, but I am trying to improve. (Student BMS, FG6)
The motivation roller coaster
Students described experiencing a fluctuation in motivation throughout a course and academic year, which influenced their study behavior and behavioral change intentions. With their past stressful exam preparation in mind, students reported beginning a new course with many resolutions wanting to improve what went “wrong,” such as aiming to study more consistently or to start exam preparations earlier. Students often managed to realize their resolutions for the first few weeks of a new course. However, after approximately 2 weeks, students lost motivation and started to slack with their studying (e.g., studying less consistently). They experienced less pressure to study, and thus experienced more motivational conflict when initiating studying due to tempting alternative activities, other course obligations, or interferences outside of university. For example, one student, being well aware of the consequences of procrastination, mentioned:
I can relax now and do this fun thing. And then maybe have to do like, a quick version of this assignment late at night. (Student BMS, FG3)
Three weeks prior to the exam, students felt increased pressure when realizing how close the exam and how much work was left. This pressure motivated students to catch up on the coursework and spend more time studying. Often, students focused on getting the studying done (e.g., creating summaries for each case) and cramming the content using methods that previously worked to pass the exam. For example, one student mentioned:
I’m also motivated in the beginning of a new block. […] I have this motivation over me. Like, well, now I’m going to keep up with it. And then sometimes, […] I lose track of the course that we’re in. And I’m behind again. And then I lose it a bit. So, when I get behind, and I’m like, okay, now I just do the minimal. And then two, a week and a half, before the exam, then I get my motivation back. (Student MED, FG2)
This paper outlines the findings of a study exploring university students’ study habits during self-regulated learning. We held focus groups aiming to explore university students’ current study habits, habit formation, and experiences with breaking habits. A thematic analysis of the transcribed data revealed five themes and one subtheme, which offer insights into our research aims regarding how students usually form new habits, and how they (try) to break ineffective habits. Our study offers a detailed exploration of university students’ study habits. Using a qualitative approach, we were able to dive beyond surface-level observations by exploring students’ experiences and factors, which influence these habits, their formation, and the challenges associated with breaking habits in-depth. While our chosen approach allows for a rich and nuanced understanding of the multifaceted nature of students’ study habits, the goal of a qualitative approach is commonly not to generalize findings to a larger population (Morse, 2008 ). The findings of this study are experiences from a specific student population rooted within a specific educational and cultural context. Including a thick description of this context, we try to establish transferability of our findings by indicating how they could apply to other contexts and student populations.
Students’ study habits
Students had different study habits depending on their goals. Students with clear short-term goals of passing the exam focused on collecting information during the course and started intense studying shortly before the exam. Students with long-term academic or career goals planned their study sessions continuously and focused more on learning the study content. Students differed in their preferred study setting when preparing for exams, but usually had a habit and preference of where and how to study. During courses, students were more flexible in their study setting. These findings suggest that long-term academic goal setting helps students to prioritize long-term learning by spreading out study sessions and focusing on learning the study content continuously. Long-term academic goal setting might be more evident for some study programs than for others. In study programs such as medicine, many first-year students have a clear goal of becoming a good doctor with long-term retention of knowledge and skills as important factors to reach that goal. Study programs with more diverse career paths might make it difficult for students to envision clear long-term goals. This lack of long-term goal setting might hamper students’ motivation to ensure more effortful studying in a manner that ensures long-term retention or best possible grades. Previous research indicates that students’ long-term perspectives are related to better self-regulation strategies (Bembenutty & Karabenick, 2004 ; De Bilde et al., 2011 ).
Students tried to be strategic in their learning strategy use by balancing perceived efficiency and effectiveness of the methods in reaching their goals. For tutorial preparations, students usually read and summarized the provided sources. For exam preparations, students combined active and passive learning strategies such as summarizing, watching videos, or explaining content to themselves. Unlike often assumed in the literature (e.g., Morehead et al., 2016 ; Yan et al., 2016 ), students in the current sample had quite accurate metacognitive knowledge. This could be related to the fact that they followed a formal learning strategy training at the beginning of their studies or the PBL system employed at UM, which aims to foster an active learning approach (Dolmans et al., 2005 ). Students might have been aware of effective and ineffective learning strategies and received suggestions on how to incorporate as many active learning approaches as possible within their self-regulated learning. Nevertheless, they used learning strategies, which are not the most effective for long-term learning but ones that they deemed most time-efficient to pass exams. While students were often aware of this discrepancy, assessment forms were an important driver for learning strategy choices. This is in line with prior research indicating that students adapt their learning strategies to examination requirements (Broekkamp & Van Hout-Wolters, 2007 ; Rovers et al., 2018 ). Bachelor students are often assessed using multiple choice questions, which focus on recognizing information instead of retention. They thus use learning strategies to maximize success for that assessment type. In order to nudge students to use learning strategies essential for long-term learning, it is crucial to align assessment by using methods that stimulate long-term learning and prioritize testing understanding instead of recognition (Van der Vleuten et al., 2010 ).
Furthermore, students’ willingness to incorporate active learning strategies such as retrieval practice seemed dependent on the available resources in their study program. In the medicine program, students had access to past exam questions and thus used them during exam preparations. Students in the biomedical sciences program, having no access to previous exam questions, found it difficult and time-consuming to create practice questions themselves and appreciated course coordinators who provided example questions. This suggests that more support from educators, such as providing practice questions or training sessions on formulating practice questions, might further increase students’ use of active learning strategies.
Forming and breaking habits
In line with previous research, students reported basing their study methods on what they had learned during high school (Dirkx et al., 2019 ). They updated these methods if they did not help them to achieve their goals by trying to embed additional steps in pre-existing habits (e.g., if going to bed, then reading notes). This method of specific if–then plans also called implementation intentions (Adriaanse & Verhoeven, 2018 ; Gollwitzer, 1993 ) is commonly suggested to foster habit formation and habit breaking. By pre-selecting a situation and mentally rehearsing the if–then link, individuals are more likely to automatically trigger the desired behavior when encountering the situation. Formulating these if–then plans could create new associations that compete with existing habits. If the new cue-action link is stronger than the habitual pattern, the desired action could potentially override the habitual response. While research across various domains supports the effectiveness of implementation intentions in promoting goal-directed actions (Adriaanse et al., 2011 ; Gollwitzer & Sheeran, 2006 ), it is unclear whether implementation intentions influence learners’ learning outcomes or metacognition (Hoch et al., 2020 ).
Students reported intentions to change their study behavior. While their intentions targeted mainly improving time management aspects or wanting to add steps to their current study habits, disentangling the extent to which students wanted to realize these intentions was difficult. Additionally, detailed reflections of how students broke past ineffective habits and whether they actually changed their behavior once their habits did not “work” anymore remained scarce, making it difficult to fully target our third research aim. Students did not see change as a priority because their current method “worked” to pass exams. As mentioned above, assessment was an important driver for students’ learning strategy choices but also a key factor in their behavioral change intentions. Students were successful in passing exams and thus did not perceive change as necessity or added benefit. However, they also indicated not knowing how to change, or experiencing change as effortful and time-consuming. The difference between not changing because perceiving change as having no additional value or because not knowing how to has important implications for behavioral change interventions. Students who do not perceive change as beneficial might profit from interventions that highlight the utility value of effective learning strategies (e.g., McDaniel & Einstein, 2020 ) in achieving a sufficient grade to pass a course as efficiently as possible. Students who do not know how to change might profit from interventions that provide them with information on how behavioral change could be achieved. More research is necessary to disentangle the extent to which students are willing to form new and break old habits and if so, how they could be best supported in this.
Another factor influencing students’ intention to change and breaking old habits was their fluctuating motivation throughout the course. Students began a new course with many resolutions wanting to improve what went “wrong” the block before but motivational conflict and their usual context quickly disabled them from realizing their intentions and instead continuing with unwanted behavior. In line with Verplanken and colleagues ( 2018 ), this finding suggests a potential importance of temporal considerations in behavioral change interventions in transition periods. Interventions held at the beginning of a new course might benefit from students’ increased motivation to change.
Additionally, students’ capacity to form new and break old habits seems to be influenced by their transition to a more independent environment. During this transition, they did not solely focus on establishing effective study habits but also had to navigate their flexible schedule during which they encountered other self-regulation challenges. According to the habit discontinuity hypothesis (Verplanken & Wood, 2006 ), major life events or disruptions can serve as catalysts for behavior change, indicating that the transition to university could potentially serve as a great opportunity to break existing habits and form more adaptive ones. As the transitions create a habit discontinuity, people change their habits and adopt new behaviors as old cues and routines that support existing habits are weakened or disrupted (Verplanken et al, 2018 ). As a result, individuals are more receptive to new opportunities to establish new habits or modify existing ones. This suggests that it might be valuable to support students in building effective habits as soon as they start university to make use of the discontinuity of existing habits, or, as mentioned above, at the beginning of a new course later in the semester. Contrastingly, our findings suggest that the new context and flexibility also challenge the formation of new habits, since it is difficult to “piggy-bag” new habits onto existing ones and because the needs for what makes learning effective to pass exams in the new context are not clear yet. Students solved this by actively looking for more structure. More research is necessary to investigate how the transition to university influences students’ capacity to form new habits.
Another factor, which could be important in students’ study habits and breaking and formation hereof, is co-regulation. The latter describes how learners’ cognition, emotions, and motivation during learning are influenced by interaction with others (Bransen et al., 2022 ; Hadwin & Oshige, 2011 ). Students mentioned making study appointments with others, which helped them to feel more accountable for their actions and thus promoted adherence to their intended behavior, suggesting that co-regulation could be an important factor in supporting students to break ineffective habits and form new habits. Furthermore, by observing how peers study, students could learn to model adaptive behavior, enabling them to form habits that incorporate more effective learning strategies. Furthermore, being able to exchange experiences with peers during the habit formation and breaking process, which might be associated with negative emotions such as stress and frustration, could help students to cope and stay motivated during their behavior change journey. However, more research is necessary to investigate how co-regulation could influence students’ study habits.
Strengths and limitations
Our findings highlight the central role of students’ goals in shaping their study habits. Knowing how goals influence study habits offers valuable insights into the importance of tailoring educational strategies to better align with students’ goals and to shed light onto goal-driven behavior in education. Furthermore, the discrepancy between students’ knowledge and behavior when it comes to the limited application of effective learning strategies seems to stem from the low perceived efficiency of these strategies, highlighting a need to increase students’ perceived efficiency of effective learning strategies to close the gap between knowledge and behavior. Furthermore, the identified challenges related to motivation fluctuations within and throughout a course and the transitioning from high school to a self-regulated learning environment in university present original perspectives, which so far remain underexamined in the context of self-regulated learning. Our study offers valuable insights into the dynamic hurdles that students face when attempting to modify their study habits and highlights the importance of aligning assessment methods with life-long learning goals. However, this study has various limitations. First, we conducted convenience sampling. We did not target a specific target population but all first-year biomedical sciences and medicine students were eligible to participate. As the study goal was advertised as an exploration of students’ study methods and habits, it might have been possible that especially students who were more confident in their study methods or habits participated leading to a biased perspective. While we cannot rule this out, students were openly sharing that they engaged in methods that they thought were not the most effective and also mention not currently wanting to change their habits. Furthermore, students’ self-reported GPA ranged from 5.8 to 9, suggesting a mix between low- and high-achieving students. Additionally, the students’ in our sample received a formal learning strategy training in their first weeks to support their self-regulated learning and professional development, which potentially shaped their study behaviors and perspectives and thus outcomes of this study. Next to this, we did not split students from the two different study programs but mixed them within our focus groups. As mentioned above, the structure of the study programs might have shaped the results and further research is necessary to investigate to what extent these findings transfer to other study programs. Second, we held four focus groups at the end of students’ first academic year and two focus groups at the beginning of students’ second academic year. This change in context might have influenced students’ perspectives on their study methods and habits. Future research is necessary to investigate to what extent students’ study methods and habits change throughout their study program. Third, we asked students to reflect on their habitual behavior. As habits are often unconscious, we did not explicitly ask students about their habits but instead asked them to reflect on behavior they usually engaged in. The fact that we did not offer any definition of what counts as a usual behavior might have led to different interpretations hereof. While not the goal of this study, a quantitative research design capturing students’ habitual behavior could give more systematic insights by clearly defining and operationalizing the concepts of “usual behavior” in measurable terms. For example, it could be operationalized as behaviors that participants engage in at least three times a week or behaviors that they have engaged in consistently for the past six months or using the Self-Report Habit Index (SRHI, Verplanken & Orbell, 2003 ).
Overall, this study contributes to the literature by providing a qualitative exploration of university students’ study habits, formation hereof, and difficulties they experience when breaking habits. A thematic analysis of six focus groups indicated that students had different study habits depending on their goals. While showing accurate metacognitive knowledge of what are effective learning strategies, students often used other learning strategies they deemed most efficient in reaching their goals. Students indicated intentions to change but did not prioritize change as their current habits enabled them to pass exams and change was not perceived as adding value. Fluctuations in motivation across courses and transitioning to a self-regulated life hampered students’ intentions to form new and break old habits. The findings give insights into influential factors affecting students’ behavioral change intentions and suggest the importance of aligning assessment methods with life-long learning and supporting students in their long-term academic goal setting to prioritize study habits that target lasting learning to optimally foster students’ self-regulated learning.
Data Availability
Due to the nature of the research, supporting data is not available to ensure privacy of participants.
Aarts, H., & Dijksterhuis, A. (2000). Habits as knowledge structures: Automaticity in goal-directed behavior. Journal of Personality and Social Psychology, 78 (1), 53–63. https://doi.org/10.1037/0022-3514.78.1.53
Article Google Scholar
Adriaanse, M. A., & Verhoeven, A. (2018). Breaking habits using implementation intentions. In B. Verplanken (Ed.), The Psychology of Habit (pp. 169–188). Springer International Publishing. https://doi.org/10.1007/978-3-319-97529-0_10
Adriaanse, M. A., Vinkers, C. D. W., De Ridder, D. T. D., Hox, J. J., & De Wit, J. B. F. (2011). Do implementation intentions help to eat a healthy diet? A systematic review and meta-analysis of the empirical evidence. Appetite, 56 (1), 183–193. https://doi.org/10.1016/j.appet.2010.10.012
Ariel, R., & Karpicke, J. D. (2018). Improving self-regulated learning with a retrieval practice intervention. Journal of Experimental Psychology: Applied, 24 (1), 43–56. https://doi.org/10.1037/xap0000133
Basila, C. (2014). Good time management and motivation level predict student academic success in college online courses. International Journal of Cyber Behavior Psychology and Learning, 4 (3), 45–52. https://doi.org/10.4018/ijcbpl.2014070104
Bembenutty, H., & Karabenick, S. A. (2004). Inherent association between academic delay of gratification, future time perspective, and self-regulated learning. Educational Psychology Review, 16 (1), 35–57. https://doi.org/10.1023/B:EDPR.0000012344.34008.5c
Biwer, F., & De Bruin, A. B. H. (2023). Teaching students to ‘Study Smart’ – A training program based on the science of learning. In In their own words: What scholars and teachers want you to know about why and how to apply the science of learning in your academic setting (pp. 411–425). Society for the Teaching of Psychology. https://teachpsych.org/ebooks/itow
Biwer, F., De Bruin, A. B. H., Schreurs, S., & Oude Egbrink, M. G. A. (2020a). Future steps in teaching desirably difficult learning strategies: Reflections from the study smart program. Journal of Applied Research in Memory and Cognition, 9 (4), 439–446. https://doi.org/10.1016/j.jarmac.2020.07.006
Biwer, F., Egbrink, M. G. A. O., Aalten, P., & De Bruin, A. B. H. (2020b). Fostering effective learning strategies in higher education—A mixed-methods study. Journal of Applied Research in Memory and Cognition, 9 (2), 186–203. https://doi.org/10.1016/j.jarmac.2020.03.004
Bjork, E. L., & Bjork, R. A. (2011). Making things hard on yourself, but in a good way: Creating desirable difficulties to enhance learning (pp. 56–64). Essays Illustrating Fundamental Contributions to Society.
Google Scholar
Blasiman, R. N., Dunlosky, J., & Rawson, K. A. (2017). The what, how much, and when of study strategies: Comparing intended versus actual study behaviour. Memory, 25 (6), 784–792. https://doi.org/10.1080/09658211.2016.1221974
Bowen, G. A. (2006). Grounded theory and sensitizing concepts. International Journal of Qualitative Methods, 5 (3), 12–23. https://doi.org/10.1177/160940690600500304
Bransen, D., Govaerts, M. J. B., Panadero, E., Sluijsmans, D. M. A., & Driessen, E. W. (2022). Putting self-regulated learning in context: Integrating self-, co-, and socially shared regulation of learning. Medical Education, 56 (1), 29–36. https://doi.org/10.1111/medu.14566
Braun, V., & Clarke, V. (2006). Using thematic analysis in psychology. Qualitative Research in Psychology, 3 (2), 77–101. https://doi.org/10.1191/1478088706qp063oa
Braun, V., & Clarke, V. (2019). To saturate or not to saturate? Questioning data saturation as a useful concept for thematic analysis and sample-size rationales. Qualitative Research in Sport, Exercise and Health., 13 (2), 201–216. https://doi.org/10.1080/2159676X.2019.1704846
Broekkamp, H., & Van Hout-Wolters, B. (2007). The gap between educational research and practice: A literature review, symposium, and questionnaire. Educational Research and Evaluation, 13 (3), 203–220. https://doi.org/10.1080/13803610701626127
Broeren, M., Heijltjes, A., Verkoeijen, P., Smeets, G., & Arends, L. (2021). Supporting the self-regulated use of retrieval practice: A higher education classroom experiment. Contemp Educ Psychol, 64 , Article 101939. https://doi.org/10.1016/j.cedpsych.2020.101939
Chen, Q., & Yan, Z. (2016). Does multitasking with mobile phones affect learning? A review. Computers in Human Behavior, 54 , 34–42. https://doi.org/10.1016/j.chb.2015.07.047
Cherryholmes, C. H. (1992). Notes on pragmatism and scientific realism. Educational Researcher, 21 (6), 13–17. https://doi.org/10.3102/0013189X021006013
Danner, U. N., Aarts, H., & De Vries, N. K. (2007). Habit formation and multiple means to goal attainment: Repeated retrieval of target means causes inhibited access to competitors. Personality and Social Psychology Bulletin, 33 (10), 1367–1379. https://doi.org/10.1177/0146167207303948
De Bilde, J., Vansteenkiste, M., & Lens, W. (2011). Understanding the association between future time perspective and self-regulated learning through the lens of self-determination theory. Learning and Instruction, 21 (3), 332–344. https://doi.org/10.1016/j.learninstruc.2010.03.002
Dembo, M. H., & Seli, H. P. (2004). Students’ resistance to change in learning strategies courses. Journal of Developmental Education, 27 (3), 2–11.
Dignath, C., & Veenman, M. V. J. (2021). The role of direct strategy instruction and indirect activation of self-regulated learning—Evidence from classroom observation studies. Educational Psychology Review, 33 (2), 489–533. https://doi.org/10.1007/s10648-020-09534-0
Dirkx, K. J. H., Camp, G., Kester, L., & Kirschner, P. A. (2019). Do secondary school students make use of effective study strategies when they study on their own? Applied Cognitive Psychology, 33 (5), 952–957. https://doi.org/10.1002/acp.3584
Dolmans, D. H. J. M., De Grave, W., Wolfhagen, I. H. A. P., & Van Der Vleuten, C. P. M. (2005). Problem-based learning: Future challenges for educational practice and research. Medical Education, 39 (7), 732–741. https://doi.org/10.1111/j.1365-2929.2005.02205.x
Dresel, M., Schmitz, B., Schober, B., Spiel, C., Ziegler, A., Engelschalk, T., Jöstl, G., Klug, J., Roth, A., Wimmer, B., & Steuer, G. (2015). Competencies for successful self-regulated learning in higher education: Structural model and indications drawn from expert interviews. Studies in Higher Education, 40 (3), 454–470. https://doi.org/10.1080/03075079.2015.1004236
Dunlosky, J., Rawson, K. A., Marsh, E. J., Nathan, M. J., & Willingham, D. T. (2013). Improving students’ learning with effective learning techniques: Promising directions from cognitive and educational psychology. Psychological Science in the Public Interest, 14 (1), 4–58. https://doi.org/10.1177/1529100612453266
Fiorella, L. (2020). The science of habit and its implications for student learning and well-being. Educational Psychology Review, 32 (3), 603–625. https://doi.org/10.1007/s10648-020-09525-1
Foerst, N. M., Klug, J., Jöstl, G., Spiel, C., & Schober, B. (2017). Knowledge vs. Action: Discrepancies in university students’ knowledge about and self-reported use of self-regulated learning strategies. Frontiers in Psychology , 8, Article 1288. https://www.frontiersin.org/articles/10.3389/fpsyg.2017.01288
Galla, B. M., & Duckworth, A. L. (2015). More than resisting temptation: Beneficial habits mediate the relationship between self-control and positive life outcomes. Journal of Personality and Social Psychology, 109 (3), 508–525. https://doi.org/10.1037/pspp0000026
Gardner, B. (2015). A review and analysis of the use of ‘habit’ in understanding, predicting and influencing health-related behaviour. Health Psychology Review, 9 (3), 277–295. https://doi.org/10.1080/17437199.2013.876238
Gollwitzer, P. M., & Sheeran, P. (2006). Implementation intentions and goal achievement: A meta‐analysis of effects and processes. In M.P. Zanna (Ed.), Advances in Experimental Social Psychology , Vol. 38, Elsevier Academic Press, pp. 69–119. https://doi.org/10.1016/S0065-2601(06)38002-1
Gollwitzer, P. M. (1993). Goal achievement: The role of intentions. European Review of Social Psychology, 4 (1), 141–185. https://doi.org/10.1080/14792779343000059
Grunschel, C., Schwinger, M., Steinmayr, R., & Fries, S. (2016). Effects of using motivational regulation strategies on students’ academic procrastination, academic performance, and well-being. Learning and Individual Differences, 49 , 162–170. https://doi.org/10.1016/j.lindif.2016.06.008
Hadwin, A., & Oshige, M. (2011). Self-regulation, coregulation, and socially shared regulation: Exploring perspectives of social in self-regulated learning theory. Teachers College Record, 113 (2), 240–264. https://doi.org/10.1177/016146811111300204
Hartwig, M. K., & Dunlosky, J. (2012). Study strategies of college students: Are self-testing and scheduling related to achievement? Psychonomic Bulletin & Review, 19 (1), 126–134. https://doi.org/10.3758/s13423-011-0181-y
Hoch, E., Scheiter, K., & Schüler, A. (2020). Implementation intentions related to self-regulatory processes do not enhance learning in a multimedia environment. Frontiers in Psychology, 11 , Article 46. https://doi.org/10.3389/fpsyg.2020.00046
Kiger, M. E., & Varpio, L. (2020). Thematic analysis of qualitative data: AMEE Guide No. 131. Medical Teacher, 42 (8), 846–854. https://doi.org/10.1080/0142159x.2020.1755030
Lally, P., & Gardner, B. (2013). Promoting habit formation. Health Psychology Review, 7 (1), 137–158. https://doi.org/10.1080/17437199.2011.603640
Lally, P., Van Jaarsveld, C. H. M., Potts, H. W. W., & Wardle, J. (2010). How are habits formed: Modelling habit formation in the real world. European Journal of Social Psychology, 40 (6), 998–1009. https://doi.org/10.1002/ejsp.674
Lally, P., Wardle, J., & Gardner, B. (2011). Experiences of habit formation: A qualitative study. Psychology, Health & Medicine, 16 (4), 484–489. https://doi.org/10.1080/13548506.2011.555774
Lepp, A., Barkley, J. E., & Karpinski, A. C. (2015). The relationship between cell phone use and academic performance in a sample of US college students. SAGE Open, 5 (1), Article 215824401557316. https://doi.org/10.1177/2158244015573169
Loersch, C., & Payne, B. K. (2011). The situated inference model: An integrative account of the effects of primes on perception, behavior, and motivation. Perspectives on Psychological Science, 6 (3), 234–252. https://doi.org/10.1177/1745691611406921
Mazar A., Wood W. (2018). Defining habit in psychology. In Verplanken B. (Ed.), The psychology of habit: Theory, mechanisms, change, and context (pp. 13–29). Springer. https://doi.org/10.1007/978-3-319-97529-0_2
McDaniel, M. A., & Einstein, G. O. (2020). Training learning strategies to promote self-regulation and transfer: The knowledge, belief, commitment, and planning framework. Perspectives on Psychological Science, 15 (6), 1363–1381. https://doi.org/10.1177/1745691620920723
Morehead, K., Rhodes, M. G., & DeLozier, S. (2016). Instructor and student knowledge of study strategies. Memory, 24 (2), 257–271. https://doi.org/10.1080/09658211.2014.1001992
Morse, J. M. (2008). “It’s only a qualitative study!” Considering the qualitative foundations of social sciences. Qualitative Health Research, 18 , 147–148. https://doi.org/10.1177/1049732307310262
Morse, J. M. (2015). Data Were Saturated. …. Qualitative Health Research, 25 (5), 587–588. https://doi.org/10.1177/1049732315576699
Moust, J. H. C., Van Berkel, H. J. M., & Schmidt, H. G. (2005). Signs of erosion: Reflections on three decades of problem-based learning at Maastricht University. Higher Education, 50 (4), 665–683. https://doi.org/10.1007/s10734-004-6371-z
Panadero, E. (2017). A review of self-regulated learning: Six models and four directions for research. Frontiers in Psychology , 8, Article 422. https://www.frontiersin.org/articles/10.3389/fpsyg.2017.00422
Rea, S. D., Wang, L., Muenks, K., & Yan, V. X. (2022). Students can (mostly) recognize effective learning, so why do they not do it? Journal of Intelligence, 10 (4), Article 127. https://doi.org/10.3390/jintelligence10040127
Rothman, A. J., Sheeran, P., & Wood, W. (2009). Reflective and automatic processes in the initiation and maintenance of dietary change. Annals of Behavioral Medicine, 38 (S1), 4–17. https://doi.org/10.1007/s12160-009-9118-3
Rovers, S. F. E., Stalmeijer, R. E., Van Merriënboer, J. J. G., Savelberg, H. H. C. M., & De Bruin, A. B. H. (2018). How and why do students use learning strategies? A mixed-methods study on learning strategies and desirable difficulties with effective strategy users. Frontiers in Psychology, 9 , Article 2501. https://doi.org/10.3389/fpsyg.2018.02501
Stalmeijer, R. E., McNaughton, N., & Van Mook, W. N. K. A. (2014). Using focus groups in medical education research: AMEE Guide No. 91. Medical Teacher, 36 (11), 923–939. https://doi.org/10.3109/0142159X.2014.917165
Thibodeaux, J., Deutsch, A., Kitsantas, A., & Winsler, A. (2017). First-year college students’ time use: Relations with self-regulation and GPA. Journal of Advanced Academics, 28 (1), 5–27. https://doi.org/10.1177/1932202X16676860
Van Der Vleuten, C. P. M., Schuwirth, L. W. T., Scheele, F., Driessen, E. W., & Hodges, B. (2010). The assessment of professional competence: Building blocks for theory development. Best Practice & Research Clinical Obstetrics & Gynaecology, 24 (6), 703–719. https://doi.org/10.1016/j.bpobgyn.2010.04.001
Verhoeven, A. A. C., Adriaanse, M. A., Evers, C., & De Ridder, D. T. D. (2012). The power of habits: Unhealthy snacking behaviour is primarily predicted by habit strength. British Journal of Health Psychology, 17 (4), 758–770. https://doi.org/10.1111/j.2044-8287.2012.02070.x
Verplanken, B., Roy, D., & Whitmarsh, L. (2018). Cracks in the wall: Habit discontinuities as vehicles for behaviour change. In B. Verplanken (Ed.), The Psychology of Habit (pp. 189–205). Springer International Publishing. https://doi.org/10.1007/978-3-319-97529-0_11
Verplanken, B. (2018). Introduction. In B. Verplanken (Ed.), The Psychology of Habit (pp. 1–10). Springer International Publishing. https://doi.org/10.1007/978-3-319-97529-0_1
Verplanken, B., & Orbell, S. (2003). Reflections on past behavior: A self-report index of habit strength. Journal of Applied Social Psychology, 33 (6), 1313–1330. https://doi.org/10.1111/j.1559-1816.2003.tb01951.x
Verplanken, B., & Wood, W. (2006). Interventions to break and create consumer habits. Journal of Public Policy & Marketing, 25 (1), 90–103. https://doi.org/10.1509/jppm.25.1.90
Waldeyer, J., Fleischer, J., Wirth, J., & Leutner, D. (2020). Validating the resource-management inventory (ReMI): Testing measurement invariance and predicting academic achievement in a sample of first-year university students. European Journal of Psychological Assessment, 36 (5), 777–786. https://doi.org/10.1027/1015-5759/a000557
Wood, W., & Neal, D. T. (2016). Healthy through habit: Interventions for initiating & maintaining health behavior change. Behavioral Science & Policy, 2 (1), 71–83. https://doi.org/10.1177/237946151600200109
Wood, W., & Rünger, D. (2016). Psychology of habit. Annual Review of Psychology, 67 , 289–314. https://doi.org/10.1146/annurev-psych-122414-033417
Yan, V. X., Bjork, E. L., & Bjork, R. A. (2016). On the difficulty of mending metacognitive illusions: A priori theories, fluency effects, and misattributions of the interleaving benefit. Journal of Experimental Psychology: General, 145 (7), 918–933. https://doi.org/10.1037/xge0000177
Zimmerman, B. J. (1986). Becoming a self-regulated learner: Which are the key subprocesses? Contemporary Educational Psychology, 11 (4), 307–313. https://doi.org/10.1016/0361-476X(86)90027-5
Download references
Acknowledgements
We thank our participants for their time-commitment and sharing their experiences openly. Additionally, we would like to thank the members of the EARLI Emerging Field Group 3 “Monitoring and Regulation of Effort” for the ongoing discussions on the topic of effort monitoring and regulation that contributed to this research.
This work was funded by the Dutch Research Council (NWO) (VIDI grant number VI.Vidi.195.135) awarded to Anique de Bruin.
Author information
Authors and affiliations.
Department of Educational Development and Research, School of Health Professions Education (SHE), Maastricht University, P.O. Box 616, 6200 MD, Maastricht, the Netherlands
Louise David, Felicitas Biwer & Anique de Bruin
Department of Health Promotion, School of Care and Public Health Research Institute (CAPHRI), Maastricht University, Maastricht, the Netherlands
Rik Crutzen
You can also search for this author in PubMed Google Scholar
Contributions
All authors contributed to the study concept and design. Material preparation, data collection, and analysis were performed by LD and FB in close consultation with AdB and RC. The first draft of the manuscript was written by LD. All authors commented on previous versions of the manuscript and read and approved the manuscript.
Corresponding author
Correspondence to Louise David .
Ethics declarations
The artificial intelligence tool Chat GPT 3.5 was used to refine the titles of four of the identified (sub-)themes. Based on these suggestions, the titles were adapted. We kept a list of the tools’ original title suggestions.
Conflict of interest
The authors declare no competing interests.
Additional information
Publisher's note.
Springer Nature remains neutral with regard to jurisdictional claims in published maps and institutional affiliations.
Supplementary Information
Below is the link to the electronic supplementary material.
Supplementary file1 (DOCX 20 KB)
Supplementary file2 (docx 22 kb), rights and permissions.
Open Access This article is licensed under a Creative Commons Attribution 4.0 International License, which permits use, sharing, adaptation, distribution and reproduction in any medium or format, as long as you give appropriate credit to the original author(s) and the source, provide a link to the Creative Commons licence, and indicate if changes were made. The images or other third party material in this article are included in the article's Creative Commons licence, unless indicated otherwise in a credit line to the material. If material is not included in the article's Creative Commons licence and your intended use is not permitted by statutory regulation or exceeds the permitted use, you will need to obtain permission directly from the copyright holder. To view a copy of this licence, visit http://creativecommons.org/licenses/by/4.0/ .
Reprints and permissions
About this article
David, L., Biwer, F., Crutzen, R. et al. The challenge of change: understanding the role of habits in university students’ self-regulated learning. High Educ (2024). https://doi.org/10.1007/s10734-024-01199-w
Download citation
Accepted : 12 February 2024
Published : 10 April 2024
DOI : https://doi.org/10.1007/s10734-024-01199-w
Share this article
Anyone you share the following link with will be able to read this content:
Sorry, a shareable link is not currently available for this article.
Provided by the Springer Nature SharedIt content-sharing initiative
- Desirable difficulties
- Self-regulated learning
- Behavioral change
- Higher education
- Find a journal
- Publish with us
- Track your research

Change Password
Your password must have 8 characters or more and contain 3 of the following:.
- a lower case character,
- an upper case character,
- a special character
Password Changed Successfully
Your password has been changed
- Sign in / Register
Request Username
Can't sign in? Forgot your username?
Enter your email address below and we will send you your username
If the address matches an existing account you will receive an email with instructions to retrieve your username
To What Extent Do Study Habits Relate to Performance?
- Elise M. Walck-Shannon
- Shaina F. Rowell
- Regina F. Frey
*Address correspondence to: Elise M. Walck-Shannon ( E-mail Address: [email protected] ).
Biology Department, Washington University in St. Louis, St. Louis, MO 63130
Search for more papers by this author
Center for Integrative Research on Cognition, Learning, and Education (CIRCLE), Washington University in St. Louis, St. Louis, MO 63130
Department of Chemistry, University of Utah, Salt Lake City, UT 84112
Students’ study sessions outside class are important learning opportunities in college courses. However, we often depend on students to study effectively without explicit instruction. In this study, we described students’ self-reported study habits and related those habits to their performance on exams. Notably, in these analyses, we controlled for potential confounds, such as academic preparation, self-reported class absences, and self-reported total study time. First, we found that, on average, students used approximately four active strategies to study and that they spent about half of their study time using active strategies. In addition, both the number of active strategies and the proportion of their study time using active strategies positively predicted exam performance. Second, on average, students started studying 6 days before an exam, but how early a student started studying was not related to performance on in-term (immediate) or cumulative (delayed) exams. Third, on average, students reported being distracted about 20% of their study time, and distraction while studying negatively predicted exam performance. These results add nuance to lab findings and help instructors prioritize study habits to target for change.
INTRODUCTION
One of our goals in college courses is to help students develop into independent, self-regulated learners. This requires students to perform several metacognitive tasks on their own, including setting goals, choosing strategies, monitoring and reflecting on performance, and modifying those steps over time ( Zimmerman, 2002 ). There are many challenges that learners encounter in developing self-regulation. One such challenge is that students often misjudge their learning during the monitoring and reflection phases ( Kornell and Bjork, 2007 ). Often, students feel that they learn more from cognitively superficial tasks than from cognitively effortful tasks. As one example, students may feel that they have learned more if they reread a text passage multiple times than if they are quizzed on that same material ( Karpicke and Roediger, 2008 ). In contrast to students’ judgments, many effortful tasks are highly effective for learning. R. A. Bjork defines these effective, effortful tasks as desirable difficulties ( Bjork, 1994 ). In the present study, we investigated the frequency with which students reported carrying out effortful (active) or superficial (passive) study habits in a large introductory biology course. Additionally, we examined the relationship between study habits and performance on exams while controlling for prior academic preparation and total study time.
THEORETICAL FRAMEWORK
Why would difficulties be desirable.
During learning, the goal is to generate knowledge or skills that are robustly integrated with related knowledge and easily accessible. Desirable difficulties promote cognitive processes that either aid forming robust, interconnected knowledge or skills or retrieving that knowledge or skill ( Bjork, 1994 ; also see Marsh and Butler, 2013 , for a chapter written for educators). Learners employing desirable difficulties may feel that they put in more effort and make more mistakes, but they are actually realizing larger gains toward long-term learning than learners using cognitively superficial tasks.
Which Study Habits Are Difficult in a Desirable Way?
Study habits can include a wide variety of behaviors, from the amount of time that students study, to the strategies that they use while studying, to the environment in which they study. The desirable difficulties framework ( Bjork and Bjork, 2011 ), describes two main kinds of effective habits that apply to our study: 1) using effortful study strategies or techniques that prompt students to generate something or test themselves during studying and 2) distributing study time into multiple sessions to avoid “cramming” near the exam. In the following two paragraphs, we expand upon these study habits of interest.
The desirable difficulties framework suggests that study strategies whereby students actively generate a product or test themselves promote greater long-term learning than study strategies whereby students passively consume presentations. This is supported by strong evidence for the “generation effect,” in which new knowledge or skills are more robustly encoded and retrieved if you generate a solution, explanation, or summary, rather than looking it up ( Jacoby, 1978 ). A few generative strategies that are commonly reported among students—summarization, self-explanation, and practice testing—are compared below. Summarization is a learning strategy in which students identify key points and combine them into a succinct explanation in their own words. As predicted by the generation effect, evidence suggests that summarization is more effective than rewriting notes (e.g., laboratory study by Bretzing and Kulhavy, 1979 ) or reviewing notes (e.g., classroom study by King, 1992 ). Self-explanation is a learning strategy wherein students ask “how” and “why” questions for material as they are being exposed to the material or shortly after ( Berry, 1983 ). This is one form of elaborative interrogation, a robust memory technique in which learners generate more expansive details for new knowledge to help them remember that information ( Pressley et al. , 1987 ). Self-explanation requires little instruction and seems to be helpful for a broad array of tasks, including recall, comprehension, and transfer. Further, it is more effective than summarization (e.g., classroom study by King, 1992 ), perhaps because it prompts students to make additional connections between new and existing knowledge. Practice testing is supported by evidence of the “testing effect,” for which retrieving information itself actually promotes learning ( Karpicke and Roediger, 2008 ). The memory benefits of the “testing effect” can be achieved with any strategy in which students complete problems or practice retrieval without relying on external materials (quizzing, practice testing, flashcards, etc.). In this study, we refer to these strategies together as “self-quizzing.” Self-quizzing is especially effective at improving performance on delayed tests, even as long as 9–11 months after initial learning ( Carpenter, 2009 ). Additionally, in the laboratory, self-quizzing has been shown to be effective on a range of tasks from recall to inference ( Karpicke and Blunt, 2011 ). Overall, research suggests that active, more effortful strategies—such as self-quizzing, summarization, and self-explanation—are more effective for learning than passive strategies—such as rereading and rewriting notes. In this study, we asked whether these laboratory findings would extend to students’ self-directed study time, focusing especially on the effectiveness of effortful (herein, “active”) study strategies.
The second effective habit described by the desirable difficulties framework is to avoid cramming study time near exam time. The “spacing effect” describes the phenomenon wherein, when given equal study time, spacing study out into multiple sessions promotes greater long-term learning than massing (i.e., cramming) study into one study session. Like the “testing effect,” the “spacing effect” is especially pronounced for longer-term tests in the laboratory ( Rawson and Kintsch, 2005 ). Based on laboratory studies, we would expect that, in a course context, cramming study time into fewer sessions close to an exam would be less desirable for long-term learning than distributing study time over multiple sessions, especially if that learning is measured on a delay.
However, estimating spacing in practice is more complicated. Classroom studies have used two main methodologies to estimate spacing, either asking the students to report their study schedules directly ( Susser and McCabe, 2013 ) or asking students to choose whether they describe their pattern of study as spaced out or occurring in one session ( Hartwig and Dunlosky, 2012 ; Rodriguez et al. , 2018 ). The results from these analyses have been mixed; in some cases, spacing has been a significant, positive predictor of performance ( Rodriquez et al. , 2018 ; Susser and McCabe, 2013 ), but in other cases it has not ( Hartwig and Dunlosky, 2012 ).
In the present study, we do not claim measure spacing directly. Lab definitions of spacing are based on studying the same topic over multiple sessions. But, because our exams have multiple topics, some students who start studying early may not revisit the same topic in multiple sessions. Rather, in this study, we measure what we refer to as “spacing potential.” For example, if students study only on the day before the exam, there is little potential for spacing. If, instead, they are studying across 7 days, there is more potential for spacing. We collected two spacing potential measurements: (1) cramming , or the number of days in advance that a student began studying for the exam; and (2) consistency , or the number of days in the week leading up to an exam that a student studied. Based on our measurements, students with a higher spacing potential would exhibit less cramming and study more consistently than students with lower spacing potential. Because not every student with a high spacing potential may actually space out the studying of a single topic into multiple sessions, spacing potential is likely to underestimate the spacing effect; however, it is a practical way to indirectly estimate spacing in practice.
Importantly, not all difficult, or effortful, study tasks are desirable ( Bjork and Bjork, 2011 ). For example, in the present study, we examined students’ level of distraction while studying. Distraction can come in many forms, commonly “multitasking,” or splitting one’s attention among multiple tasks (e.g., watching lectures while also scrolling through social media). However, multitasking has been shown to decrease working memory for the study tasks at hand ( May and Elder, 2018 ). Thus, it may make a task more difficult, but in a way that interferes with learning rather than contributing to it.
In summary, available research suggests that active, effortful study strategies are more effective than passive ones; that cramming is less effective than distributing studying over time; and that focused study is more effective than distracted study. Whether students choose to use these more effective practices during their independent study time is a separate question.
How Do Students Actually Study for Their Courses?
There have been several studies surveying students’ general study habits. When asked free-response questions about their study strategies in general, students listed an average of 2.9 total strategies ( Karpicke et al. , 2009 ). In addition, few students listed active strategies, such as self-quizzing, but many students listed more passive strategies, such as rereading.
There have also been studies asking whether what students actually do while they are studying is related to their achievement. Hartwig and Dunlosky (2012) surveyed 324 college students about their general study habits and found that self-quizzing and rereading were positively correlated with grade point average (GPA). Other studies have shown that using Facebook or texting during study sessions was negatively associated with college GPA ( Junco, 2012 ; Junco and Cotten, 2012 ). While these findings are suggestive, we suspect that the use of study strategies and the relationship between study strategies and achievement may differ from discipline to discipline. The research we have reviewed thus far has been conducted for students’ “general” study habits, rather than for specific courses. To learn about how study habits relate to learning biology, it is necessary to look at study habits within the context of biology courses.
How Do Students Study for Biology Courses?
Several prior qualitative studies carried out within the context of specific biology courses have shown that students often report ineffective habits, such as favoring passive strategies or cramming. Hora and Oleson ( 2017 ) found that, when asked about study habits in focus groups, students in science, technology, engineering, and mathematics (STEM) courses (including biology) used predominantly passive strategies such as reviewing notes or texts, practices that in some cases were unchanged from high school. Tomanek and Montplaisir (2004) found that the majority of 13 interviewed students answered questions on old exams (100% of students) and reread lecture slides (92.3% of students) or the textbook (61.5% of students) to study for a biology exam, but only a small minority participated in deeper tasks such as explaining concepts to a peer (7.7% of students) or generating flashcards for retrieval practice (7.7% of students). We can also learn indirectly about students’ study habits by analyzing what they would change upon reflection. For example, in another study within an introductory biology classroom, Stanton and colleagues ( 2015 ) asked students what they would change about their studying for the next exam. In this context, 13.5% of students said that using active strategies would be more effective for learning, and 55.5% said that they wanted to spend more time studying, many of whom reported following through by studying earlier for the next exam ( Stanton et al. , 2015 ). In the current study, we extended prior research by exploring the prevalence of multiple study habits simultaneously, including the use of active study strategy and study timing, in a large sample of introductory biology students.
In addition to characterizing students’ study habits, we also aimed to show how those study habits were related to performance in a biology classroom. In one existing study, there were positive associations between exam performance and some (but not all) active strategies—such as completing practice exams and taking notes—but no significant associations between performance and some more passive strategies—such as reviewing notes/screencasts or reviewing the textbook ( Sebesta and Bray Speth, 2017 ). In another study, both self-reported study patterns (e.g., spacing studies into multiple sessions or one single session) and self-quizzing were positively related to overall course grade in a molecular biology course ( Rodriguez et al. , 2018 ). We build on this previous work by asking whether associations between performance and a wide variety of study habits still hold when controlling for confounding variables, such as student preparation and total study time.
In this study, we asked whether students actually use cognitive psychologists’ recommendations from the desirable difficulty framework in a specific biology course, and we investigated whether students who reported using those recommendations during studying performed differently on exams than those who did not. We wanted to focus on how students spend their study time, rather than the amount of time that they study, their level of preparation, or engagement. Therefore, we used regression analyses to hold preparation (i.e., ACT math and the course pretest scores), self-reported class absences, and overall study time equal. In this way, we estimated the relationship between particular study habit variables—including the strategies that students use, their timing of using those strategies, and their level of distraction while studying—and exam performance.
Students would use a combination of active and passive strategies, but those who used more active study strategies or who devoted more of their study time to active strategies would perform higher on their exams than those who used fewer active strategies or devoted less time to active strategies.
Students would vary in their study timing, but those with less spacing potential (e.g., crammed their study time or studied less consistently) would perform worse, especially on long-term tests (final exam and course posttest), than students with more spacing potential.
Students would report at least some distraction during their studying, but those who reported being distracted for a smaller percent of their study time would score higher on exams than students who reported being distracted for a larger percent of their study time.
Context and Participants
Data for this study were gathered from a large-enrollment introductory biology course (total class size was 623) during the Spring 2019 semester at a selective, private institution in the Midwest. This course covers basic biochemistry and molecular genetics. It is the first semester of a two-semester sequence. Students who take this course are generally interested in life science majors and/or have pre-health intentions. The data for this study came from an on-campus repository; both the repository and this study have been approved by our internal review board (IRB ID: 201810007 for this study; IRB ID: 201408004 for the repository). There were no exclusion criteria for the study. Anyone who gave consent and for whom all variables were available was considered for the analyses. However, because the variables were different in each analysis, the sample differed slightly from analysis to analysis. When we compared students who were included in the first hypothesis’s analyses to students who gave consent but were not included, we found no significant differences between participants and nonparticipants for ACT math score, pretest score, year in school, sex, or race (Supplemental Table 1). This suggested that our sample did not dramatically differ from the class as a whole.
Other than those analyses labeled “post hoc,” analyses were preplanned before data were retrieved.
Timeline of Assignments Used in This Study
Figure 1 shows a timeline of the assignments analyzed in this study, which included the exam 1 and 2 reflections (both online), exams 1 and 2 (both in person), the course pre and post knowledge tests (both online), and a cumulative final exam (in person). As shown in the text boxes within Figure 1 , the majority (85.7% [430/502] or greater) of students completed each of the assignments that were used in this study.
FIGURE 1. Timeline of assignments used in this study organized by mode of submission (online vs. in person) and grading (completion vs. accuracy). Exam days are indicated by thick lines. There were other course assignments (including a third exam), but they are not depicted here, because they were not analyzed in this study. Exam return is indicated by dotted lines. Light gray boxes represent weeks that class was in session. The number of consenting students who completed each assignment is indicated in the corresponding assignment box; the total number of consenting students was 502.
Exam Reflections
Students’ responses to exam 1 and 2 study habits reflections were central to all of our hypotheses. In these reflection assignments, students were asked to indicate their study habits leading up to the exam (see Supplemental Item 1 for prompts), including the timing of studying and type of study strategies. The list of strategies for students to choose from came from preliminary analysis of open-response questions in previous years. To increase the likelihood that students accurately remembered their study habits, we made the exercise available online immediately after each exam for 5 days. The reflection assignment was completed before exam grades were returned to students so that their performance did not bias their memory of studying. Students received 0.20% of the total course points for completion of each reflection.
Exams in this course contained both structured-response (multiple-choice, matching, etc.) and free-response questions. The exams were given in person and contained a mixture of lower-order cognitive level (i.e., recall and comprehension) and higher-order cognitive level (i.e., application, analysis, synthesis, or evaluation) questions. Two independent (A.B and G. Y.) raters qualitatively coded exam questions by cognitive level using a rubric slightly modified from Crowe et al. (2008) to bin lower-order and higher-order level questions. This revealed that 38% of exam points were derived from higher-order questions. Each in-term exam was worth 22.5% of the course grade, and the cumulative final exam was worth 25% of the course grade. To prepare for the exams, students were assigned weekly quizzes and were given opportunities for optional practice quizzing and in-class clicker questions as formative assessment. Students were also provided with weekly learning objectives and access to the previous year’s exams. None of the exam questions were identical to questions presented previously in problem sets, old exams, or quizzes. Additionally, in the first week of class, students were given a handout about effective study strategies that included a list of active study techniques along with content-specific examples. Further, on the first quiz, students were asked to determine the most active way to use a particular resource from a list of options. The mean and SD of these exams, and all other variables used in this analysis, can be found in Supplemental Table 2. Pairwise correlations for all variables can be found in Supplemental Table 3
Pre and Post Knowledge Test
As described previously ( Walck-Shannon et al. , 2019 ), the pre/posttest is a multiple-choice test that had been developed by the instructor team. The test contained 38 questions, but the percentage of questions correct is reported here for ease of interpretation. The same test was given online in the first week of classes and after class sessions had ended. One percent extra credit was given to students who completed both tests. To encourage students to participate fully, we presented the pre and posttests as learning opportunities in the course to foreshadow topics for the course (pretest) or review topics for the final (posttest). Additionally, we told students that “reasonable effort” was required for credit. Expressing this rationale seemed to be effective for participation rates. While others have found that participation is low when extra credit is offered as an incentive (38%, Padilla-Walker et al. , 2005 ), we found participation rates for the pre- and posttests to be high; 97.4% of students completed the pretest and 85.9% of students completed the posttest.
Statistical Analyses
To test our three hypotheses, we used hierarchical regression. We controlled for potential confounding variables in step 1 and factored in the study variable of interest at step 2 for each model. We performed the following steps to check that the assumptions of linear regression were met for each model: first, we made scatter plots and found that the relationship was roughly linear, rather than curved; second, we plotted the histogram of residuals and found that they were normally distributed and centered around zero; and finally, we checked for multicollinearity by verifying that no two variables in the model were highly correlated (greater than 0.8). All statistical analyses were performed in JMP Pro (SAS Institute).
Base Model Selection
The purpose of the base model was to account for potential confounding variables. Thus, we included variables that we theoretically expected to explain some variance in exam performance based on previous studies. First, based on a meta-analysis ( Westrick et al. , 2015 ) and our own previous study with a different cohort in this same course ( Walck-Shannon et al. , 2019 ), we expected academic preparation to predict performance. Therefore, we included ACT math and biology pretest scores in our base model. Second, the negative relationship between self-reported class absences and exam or course performance is well documented ( Gump, 2005 ; Lin and Chen, 2006 ; Credé et al. , 2010 ). Therefore, we included the number of class sessions missed in our base model. Finally, our research questions focus on how students use their study time, rather than the relationship between study time itself and performance. Because others have found a small but significant relationship between total study time and performance ( Credé and Kuncel, 2008 ), we controlled for the total number of hours spent studying in our base model. In summary, theoretical considerations of confounds prompted us to include ACT math score, biology pretest score, self-reported class absences, and self-reported exam study time as the base for each model.
Calculated Indices
In the following sections we provide descriptions of variables that were calculated from the reported data. If variables were used directly as input by the student (e.g., class absences, percent of study time distracted) or directly as reported by the registrar (e.g., ACT score), they are not listed below.
Total Exam Study Time.
In students’ exam reflections, they were asked to report both the number of hours that they studied each day in the week leading up to the exam and any hours that they spent studying more than 1 week ahead of the exam. The total exam study time was the sum of these study hours.
Number of Active Strategies Used.
To determine the number of active strategies used, we first had to define which strategies were active. To do so, all authors reviewed literature about desirable difficulties and effective study strategies (also reviewed in Bjork and Bjork, 2011 , and Dunlosky et al. , 2013 , respectively). Then, each author categorized the strategies independently. Finally, we met to discuss until agreement was reached. The resulting categorizations are given in Table 1 . Students who selected “other” and wrote a text description were recoded into existing categories. After the coding was in place, we summed the number of active strategies that each student reported to yield the number of active strategies variable.
Exam 1 | Exam 2 | |||||
---|---|---|---|---|---|---|
Study strategy abbreviation | Study strategy prompt | Type | % | % | ||
Read notes | Read lecture slides or class notes | Passive | 401 | 94.58 | 445 | 94.48 |
Completed problem sets | Answered the problem set questions | Active | 365 | 86.08 | 393 | 83.44 |
Completed old exams | Answered the old exam questions | Active | 348 | 82.08 | 341 | 72.4 |
Self-quizzed | Quizzed myself using ungraded weekly quizzes, quizlets, flashcards | Active | 321 | 75.71 | 317 | 67.3 |
Explained concepts | Explained concepts to myself or others | Active | 275 | 64.86 | 301 | 63.91 |
Synthesized notes | Paraphrased or outlined class notes | Active | 257 | 60.61 | 326 | 69.21 |
Made diagrams | Made my own diagrams or comparison tables from lecture notes | Active | 232 | 54.72 | 312 | 66.24 |
Reviewed online outside content | Reviewed online content from sources outside of the course (e.g., videos) | Passive | 172 | 40.57 | 240 | 50.96 |
Watched lecture | Watched lecture videos | Passive | 171 | 40.33 | 248 | 52.65 |
Read textbook | Read textbook | Passive | 162 | 38.21 | 97 | 20.59 |
Attended review session/help sessions/office hours | Attended the exam review session, study hall, office hours, etc. | Mixed | 101 | 23.82 | 59 | 12.53 |
Rewrote notes | Rewrote my class notes word for word | Passive | 69 | 16.27 | 81 | 17.2 |
a The classification of the strategy into active and passive is stated in “type.” Prevalences for exam 1 ( n = 424) and exam 2 ( n = 471) are reported.
Proportion of Study Time Using Active Strategies.
In addition to asking students which strategies they used, we also asked them to estimate the percentage of their study time they spent using each strategy. To calculate the proportion of study time using active strategies, we summed the percentages of time using each of the active strategies, then divided by the sum of the percentages for all strategies. For most students (90.0% for exam 1 and 92.8% for exam 2), the sum of all percentages was 100%. However, there were some students whose reported percentages did not add to 100%. If the summed percentages added to between 90 and 110%, they were still included in analyses. If, for example, the sum of all percentages was 90%, and 40% of that was using active strategies, this would become 0.44 (40/90). If the summed percentages were lower than 90% or higher than 110%, students were excluded from the analyses involving the proportion of active study time index.
Number of Days in Advance Studying Began.
In the exam 2 reflection, we asked students to report: 1) their study hours in the week leading up to the exam; and 2) if they began before this time, the total number of hours and date that they began studying. If students did not report any study hours earlier than the week leading up to the exam, we used their first reported study hour as the first day of study. If students did report study time before the week before the exam, we used the reported date that studying began as the first day of study. To get the number of days in advance variable, we counted the number of days between the first day of study and the day of the exam. If a student began studying on exam day, this would be recorded as 0. All students reported some amount of studying.
Number of Days Studied in Week Leading Up to the Exam.
As a measure of studying consistency, we counted the number of days that each student reported studying in the week leading up to exam 2. More specifically, the number of days with nonzero reported study hours were summed to give the number of days studied.
The study strategies that students selected, the timing with which they implemented those strategies, and the level of distraction they reported while doing so are described below. We depict the frequencies with which certain study variables were reported and correlate those study variables to exam 1 and exam 2 scores. For all performance analyses described in the Results section, we first controlled for a base model described below.
We attempted to control for some confounding variables using a base model, which included preparation (ACT math and course pretest percentage), self-reported class absences, and self-reported total study hours. For each analysis, we included all consenting individuals who responded to the relevant reflection questions for the model. Thus, the sample size and values for the variables in the base model differed slightly from analysis to analysis. For brevity, only the first base model is reported in the main text; the other base models included the same variables and are reported in Supplemental Tables 5A, 7A, and 8A.
The base model significantly predicted exam 1 score and exam 2 score for all analyses. Table 2 shows these results for the first analysis; exam 1: R 2 = 0.327, F (4, 419) = 51.010, p < 0.0001; exam 2: R 2 = 0.219, F(4, 466) = 32.751, p < 0.0001. As expected, all individual predictor terms were significant for both exams, with preparation and study time variables positively associated and absences negatively associated. For means and SDs of all continuous variables in this study, see Supplemental Table 2. We found that the preparatory variables were the most predictive, with the course pretest being more predictive than ACT math score. Total study time and class absences were predictive of performance to a similar degree. In summary, our base model accounted for a substantial proportion (32.7%) of the variance due to preparation, class absences, and study time, which allowed us to interpret the relationship between particular study habits and performance more directly.
Exam 1 | Exam 2 | |||||||
---|---|---|---|---|---|---|---|---|
Base model | β | SE | β | SE | ||||
Intercept | – | 36.441*** | 5.502 | 0.327 | – | 17.235* | 7.949 | 0.219 |
ACT [0–36] | 0.240*** | 0.965*** | 0.240 | 0.223*** | 1.248*** | 0.243 | ||
Bio. pretest % [0–100] | 0.419*** | 0.339*** | 0.035 | 0.333*** | 0.364*** | 0.047 | ||
Number of classes missed before exam 1 (0–15) or between exams 1 and 2 (0–12) | −0.160*** | −0.630*** | 0.158 | −0.101* | −0.450* | 0.183 | ||
Total exam 1 or 2 study hours (≥0) | 0.126** | 0.132** | 0.042 | 0.154*** | 0.203*** | 0.055 |
a Standardized β values, unstandardized b values, and standard errors (SE) are reported. Each model’s R 2 is also reported. Ranges of possible values for each variable are shown in brackets. The values for the base models corresponding to Tables 4 – 6 vary slightly depending on the students included in that analysis (see Supplemental Table 4). The following symbols indicate significance: * p ≤ 0.05; ** p ≤ 0.01; *** p ≤ 0.001.
Did Students Who Used More Active Study Strategies Perform Better on Exams?
We first investigated the specific study strategies listed in Table 1 . Then, we examined the total amount of time spent on active strategies to test our hypothesis that students who spent more time actively studying performed better on exams. Further, we counted the number of different types of active strategies that students used to test whether students who used a more diverse set of active strategies performed better on exams than those who used fewer active strategies.
Study Strategies Differed in Their Frequency of Use and Effectiveness.
The frequency with which specific study strategies were employed is reported in Table 1 . Almost all students reported reading notes. The next most prevalent strategies were active in nature, including that students (in order of prevalence) completed problem sets, completed old exams, self-quizzed, synthesized notes, explained concepts, and made diagrams. Surprisingly, each of these active strategies was used by the majority of students (54.7–86.1%) for both exams 1 and 2 ( Table 1 ). Less frequently used strategies included those more passive in nature, including that students (in order of prevalence) watched lectures, reviewed online content, read the textbook, and rewrote notes. A relatively infrequent strategy was attending review sessions, office hours, and help sessions. Because student engagement varied dramatically in these different venues, we classified this category as mixed. In summary, our results showed that, after reading notes, the most frequently used strategies were active strategies.
Next, we wondered whether the types of strategies that students reported using were related to exam performance. For these analyses, we added whether a student used a specific strategy (0 or 1) into the model, after controlling for the base model reported in Table 2 . When holding preparation, class absences, and total study time equal, we found that, on average, students who reported having completed problem sets, explained concepts, self-quizzed, or attended review sessions earned 4.0–7.7% higher on average on both exams 1 and 2 than students who did not report using the strategy (see b unstd. in Table 3 ). Notably, these strategies were active in nature, except for the category attending review session, which was mixed in nature. The remaining active strategies were positively correlated to performance for only one of the exams. Additionally, we observed that the strategies categorized as passive were either nonsignificant or negatively related to performance on at least one exam. Together, these results suggest that active strategies tended to be positively related to exam performance. In our sample, each of these active strategies was used by the majority (more than half) of the students.
Exam 1 | Exam 2 | ||||||
---|---|---|---|---|---|---|---|
Study strategy | Type | SE | ΔR | SE | Δ | ||
Read notes | Passive | −1.225 | 1.954 | 0.001 | −2.634 | 2.705 | 0.002 |
Completed problem sets | Active | 4.320*** | 1.292 | 0.018*** | 7.678*** | 1.637 | 0.034*** |
Completed old exams | Active | 1.444 | 1.177 | 0.003 | 5.847*** | 1.373 | 0.029*** |
Self-quizzed | Active | 4.377*** | 1.042 | 0.028*** | 5.395*** | 1.317 | 0.027*** |
Explained concepts | Active | 5.538*** | 0.917 | 0.054*** | 6.288*** | 1.280 | 0.038*** |
Synthesized notes | Active | 1.402 | 0.957 | 0.004 | 2.813* | 1.375 | 0.007* |
Made diagrams | Active | 1.855* | 0.926 | 0.007* | 2.102 | 1.341 | 0.004 |
Reviewed online outside content | Passive | −1.839* | 0.910 | 0.007* | −1.731 | 1.256 | 0.003 |
Watched lecture | Passive | 0.194 | 0.968 | 0.001 | −2.660* | 1.305 | 0.006* |
Read textbook | Passive | −1.352 | 0.911 | 0.004 | −1.425 | 1.525 | 0.003 |
Attended review session/help sessions/office hours | Mixed | 4.600*** | 1.028 | 0.031*** | 3.961* | 1.900 | 0.007* |
Rewrote notes | Passive | −0.853 | 1.232 | 0.001 | −4.177* | 1.659 | 0.011* |
All strategies | – | – | – | 0.114*** | – | – | 0.115*** |
a This table summarizes 12 two-step hierarchical models per exam. The first step adjusted for the base model (see Table 2 ), and the second step included one specific study strategy. Unstandardized b values, standard errors (SE), and the change in R 2 relative to the base model are reported. Full model outputs are reported in Supplemental Table 4. Unstandardized b coefficients correspond to the average difference in exam score between the people who did and did not use the strategy. The following symbols indicate significance: * p ≤ 0.05; ** p ≤ 0.01; *** p ≤ 0.001.
b Relative to a base model with R 2 = 0.327 that included ACT score, bio. pretest %, number of classes missed before exam 1, and total exam 1 study hours. See Table 2 for base model details.
c Relative to a base model with R 2 = 0.219 that included ACT score, bio. pretest %, number of classes missed between exams 1 and 2, and total exam 2 study hours. See Table 2 for base model details.
The Proportion of Time Spent Using Active Strategies Positively Predicted Exam Score.
To further understand how active strategies related to performance, we investigated the proportion of study time that students spent using active strategies. On average, students spent about half of their study time using active strategies for exam 1 (M = 0.524, SD = 0.244) and exam 2 (M = 0.548, SD = 0.243), though values varied from 0 to 1 ( Figure 2 ). Importantly, students who spent a larger proportion of their study time on active strategies tended to perform better on exams 1 and 2. More specifically, after accounting for our base model (Supplemental Table 5A), the proportion of time students spent using active strategies added significant additional predictive value for exam 1, F (1, 416) = 8.770, p = 0.003, Δ R 2 = 0.014; and exam 2, F (1, 450) = 14.848, p = 0.0001, Δ R 2 = 0.024. When holding preparation, class absences, and total study time equal, we found that students who spent all of their study time on active strategies scored 5.5% higher and 10.0% higher on exams 1 and 2, respectively, than those who spent none of their study time on active strategies ( Table 4 ). Overall, these two results suggested that, on average, students spent about half of their study time using active strategies and students who devoted more study time to active strategies tended to perform better on exams.
FIGURE 2. Distribution of the proportion of time that students devoted to active study for exam 1 ( n = 422) and exam 2 ( n = 456). Percentages of students in each bin are indicated.
Exam 1 | Exam 2 | |||||
---|---|---|---|---|---|---|
Study strategy | SE | Δ | SE | Δ | ||
Proportion of exam study time using active strategies (0–1) | 5.499** | 1.857 | 0.014** | 9.967*** | 2.587 | 0.024*** |
Number of active strategies used for exam studying (0–6) | 1.858*** | 0.320 | 0.051*** | 2.759*** | 0.420 | 0.066*** |
a This table summarizes two two-step hierarchical models per exam. The first step adjusted for the base model, and the second step included either proportion of active study time or the number of active strategies. Full model outputs are reported in Supplemental Table 5. Unstandardized b values, standard errors (SE), and the change in R 2 from the base model (see Supplemental Table 5A) are reported. Ranges of possible values for each variable are given in brackets. The following symbols indicate significance: * p ≤ 0.05; ** p ≤ 0.01; *** p ≤ 0.001.
b Relative to a base model with R 2 = 0.322 that included ACT score, bio. pretest %, number of classes missed before exam 1, and total exam 1 study hours. See Supplemental Table 5A for base model details.
c Relative to a base model with R 2 = 0.238 that included ACT score, bio. pretest %, number of classes missed between exams 1 and 2, and total exam 2 study hours. See Supplemental Table 5A for base model details.
The Number of Active Strategies Used Positively Predicted Exam Score.
We next investigated the number of active strategies used by each student. On average, students used approximately four active strategies for exam 1 (M = 4.212, SD = 1.510) and exam 2 (M = 4.239, SD = 1.501). Very few students used no active strategies and most students (73%) used four or more active strategies ( Figure 3 ). Further, those students who used more active strategies tended to perform higher on exams 1 and 2. More specifically, after accounting for our base model, the number of active strategies students used added significant additional predictive value for exam 1, ( F (1, 416) = 33.698, p < 0.0001 Δ R 2 = 0.024; and exam 2, F (1, 450) = 91.083, p < 0.0001, Δ R 2 = 0.066. When holding preparation, class absences, and total study time equal, we found that, for each additional active strategy used, students scored 1.9% and 2.8% higher on exams 1 and 2, respectively. Students who used all six active strategies scored 11.1% higher and 16.6% higher on exams 1 and 2, respectively, than those who used no active strategies ( Table 4 , See Supplemental Table 5A for base model). In summary, students who used a greater diversity of active strategies tended to perform better on exams.
FIGURE 3. Distribution of the number of active strategies that each student used for exam 1 ( n = 422) and exam 2 ( n = 456). Percentages of students in each bin are indicated.
Post Hoc Analysis 1: Are Certain Active Strategies Uniquely Predictive of Performance?
Though it was not part of our planned analyses, the previous finding that the number of active strategies is predictive of performance made us question whether certain active strategies are uniquely predictive or whether they each have overlapping benefits. To test this, we added all six of the active strategies into the model as separate variables in the same step. When doing so, we found that the following active strategies were distinctly predictive for both exams 1 and 2: explaining concepts, self-quizzing, and completing problem sets (Supplemental Table 6). In other words, the portion of exam-score variance explained by certain active strategies was non-overlapping.
Did Study Timing Predict Performance on Immediate or Delayed Exams?
We next characterized students’ spacing potential using two indices: 1) the number of days in advance that studying began (cramming) and 2) the number of days in the week leading up to the exam that a student studied (consistency). Notably, in these results, we adjusted for our base model, which included total study time. In this way, we addressed the timing of studying while holding the total amount of studying equal. We examined outcomes at two different times: exam 2, which came close after studying; and the cumulative final exam and the posttest, which came after about a 5-week delay.
Cramming Was Not a Significant Predictor of Exam 2, the Final Exam, or the Posttest.
While there was variation in the degree of cramming among students, this was not predictive of exam score on either immediate or delayed tests. On average, students began studying 5.842 d in advance of exam 2 (SD = 4.377). About a third of students began studying 0–3 days before the exam, and another third began studying 4–6 days before the exam ( Figure 4 A). When holding preparation, class absences, and total study time equal, we found that the number of days in advance that studying began was not a significant predictor of in-term exam 2, the posttest, or the cumulative final ( Table 5 ; see Supplemental Table 7A for base model).
FIGURE 4. Distributions of spacing potential variables for exam 2 ( n = 450). (A) The distribution of the days in advance that exam 2 studying began (cramming); (B) the distribution of the number of days studied in the week before exam 2 (consistency). Percentages of students in each bin are indicated.
Exam 2 | Posttest | Final exam | |||||||
---|---|---|---|---|---|---|---|---|---|
Study habit | SE | Δ | SE | Δ | SE | Δ | |||
Days in advance exam 2 studying began (≥0) | 0.011 | 0.168 | 0.000 | 0.252 | 0.162 | 0.003 | −0.184 | 0.140 | 0.002 |
Number of days studied in week before exam 2 (0–8) | −0.464 | 0.418 | 0.002 | −0.589 | 0.413 | 0.003 | −0.608 | 0.348 | 0.004 |
a This table summarizes two two-step hierarchical regression models per assessment. The first step adjusted for our base model, and second step included either days in advance, or number of days studied in week before exam. Full model outputs are reported in Supplemental Table 7. Unstandardized b values, standard errors (SE), and the change in R 2 from the base model (Supplemental Table 7A) are reported. Ranges of possible values for each variable are given in brackets. The following symbols indicate significance, * p ≤ 0.05; ** p ≤ 0.01; *** p ≤ 0.001.
b Relative to a base model with R 2 = 0.231 that included ACT score, bio. pretest %, number of classes missed before exam 2, and total exam 2 study hours. See Supplemental Table 7A for base model details.
c Relative to a base model with R 2 = 0.396 that included ACT score, bio. pretest %, number of classes missed before exam 2, and total exam 2 study hours. See Supplemental Table 7A for base model details.
d Relative to a base model with R 2 = 0.273 that included ACT score, bio. pretest %, number of classes missed before exam 2, and total exam 2 study hours. See Supplemental Table 7A for base model details.
Studying Consistency Was Not a Significant Predictor of Exam 2, the Final Exam, or the Posttest.
While there was variation in how consistently students studied in the week leading up to exam 2, this consistency was not predictive of exam score either immediately or on delayed tests. On average, students studied 5 of the 8 days leading up to the exam (M = 5.082, SD = 1.810 ). Sixteen percent of students studied every day, and no students studied fewer than 2 days in the week leading up to the exam ( Figure 4 B). When holding preparation, class absences, and total study time equal, we found that the number of days studied in the week leading up to the exam was not a significant predictor of in-term exam 2, the posttest, or the cumulative final ( Table 5 ; see Supplemental Table 7A for base model).
In summary, our students varied in both the degree of cramming and the consistency of their studying. Even so, when holding preparation, class absences, and study time equal as part of our base model, neither of these spacing potential measures were predictive of performance on immediate or delayed tests.
Did Students Who Reported Being Less Distracted while Studying Perform Better on Exams?
In addition to the timing of studying, another factor that contextualizes the study strategies is how focused students are during study sessions. In the exam reflections, we asked students how distracted they were while studying. Here, we relate those estimates to exam scores while controlling for our base model of preparation, class absences, and total study time.
Distraction while Studying Was a Negative Predictor of Exam Score.
On average, students reported being distracted during 20% of their exam 1 and exam 2 study time (exam 1: M = 20.733, SD = 16.478; exam 2: M = 20.239, SD = 15.506) . Sixty-one percent of students reported being distracted during more than 10% of their study time ( Figure 5 ). Further, students who were more distracted while studying tended to perform lower on exams 1 and 2. After accounting for our base model, the percent of study time that students reported being distracted added significant additional predictive value for exam 1 and exam 2; exam 1: F (1, 429) = 12.365, p = 0.000, Δ R 2 = 0.019; exam 2: F (1, 467) = 8.942, p = 0.003, Δ R 2 = 0.015. When holding preparation, class absences, and total study time equal, we found that students who reported being distracted 10% more than other students scored about 1% lower on exams 1 and 2 ( Table 6 ; see Supplemental Table 8A for base model). In summary, this suggests that not only was it common for students to be distracted while studying, but this was also negatively related to exam performance.
FIGURE 5. Distribution of the percent of time students reported being distracted while studying for exam 1 ( n = 435) and exam 2 ( n = 473). Percentages of students in each bin are indicated.
Exam 1 | Exam 2 | |||||
---|---|---|---|---|---|---|
Study strategy | SE | Δ | SE | Δ | ||
Percent of time distracted while studying (0–100) | −0.093*** | 0.027 | 0.019*** | −0.119** | 0.040 | 0.015** |
a This table summarizes one two-step hierarchical regression model per exam. The first step adjusted for our base model, and second step included the percent of study time that students reported being distracted. Full model outputs are reported in Supplemental Table 8. Unstandardized b values, standard errors (SE), and the change in R 2 from the base model (see Supplemental Table 8A) are reported. The range of possible values is given in brackets. The following symbols indicate significance: * p ≤ 0.05; ** p ≤ 0.01; *** p ≤ 0.001.
b Relative to a base model with R 2 = 0.328 that included ACT score, bio. pretest %, number of classes missed before exam 1, and total exam 1 study hours. See Supplemental Table 8A for base model details.
c Relative to a base model with R 2 = 0.219 that included ACT score, bio. pretest %, number of classes missed between exams 1 and 2, and total exam 2 study hours. See Supplemental Table 8A for base model details.
Students’ independent study behaviors are an important part of their learning in college courses. When holding preparation, class absences, and total study time equal, we found that students who spent more time on effortful, active study strategies and used a greater number of active strategies had higher scores for exams. Yet neither students who started studying earlier nor those who studied over more sessions scored differently than students who started later or studied over fewer sessions. Additionally, students who were more distracted while studying tended to perform worse than students who were less distracted. In other words, both the degree to which students employed desirably difficult strategies while studying and their level of focus when doing so were important for performance.
Specific Study Strategies (Hypothesis 1)
Our finding that more time and diversity of active study strategies were associated with higher exam grades was consistent with our hypothesis based on the desirable difficulties framework, laboratory, and classroom research studies ( Berry, 1983 ; King, 1992 ; Bjork, 1994 ; Karpicke and Roediger, 2008 ; Karpicke and Blunt, 2011 ; Hartwig and Dunlosky, 2012 ). Our study brought together lab research about effective strategies with what students did during self-directed study in an actual course. In doing so, we affirmed the lab findings that active strategies are generally effective, but also uncovered further nuances that highlight the value of investigating course-specific study strategies.
First, our study, when combined with other work, may have revealed that certain study strategies are more common than course-nonspecific surveys would predict. For example, compared with surveys of general study habits, our students reported relatively high use of active strategies. We found that the majority of students (73%) reported using four or more active strategies, which was more than the 2.9 average total strategies listed by students in a survey about general study habits at this same institution ( Karpicke et al. , 2009 ). In particular, we found that two-thirds of students reported the active study strategy of self-quizzing. This was considerably higher than what was found in a free-response survey about general habits not focused on a specific course at the same institution ( Karpicke et al. , 2009 ). In this survey, only 10.7% reported self-testing and 40.1% reported using flashcards. This higher frequency of self-quizzing behaviors may be due to a combination of factors in the course, the measures, and/or the students. In this course, we attempted to make self-quizzing easier by reopening the weekly quiz questions near exam time ( Walck-Shannon et al. , 2019 ). We also used a course-specific survey rather than the more general, course-nonspecific surveys used in the previous research. Additionally, it is possible that, in recent years, more students have become more aware of the benefits of self-testing and so are using this strategy with greater frequency. When we compared our frequencies of several categories to analogous categories from course-specific surveys of introductory biology students ( Sebesta and Bray Speth, 2017 ) and molecular biology students ( Rodriguez et al. , 2018 ), we saw similar results. Combined with our work, these studies suggest that when students focused on a particular course, they reported more active strategies than when prompted about studying in general.
Second, the opportunity to control for potential confounding variables in our study, including total study time, allowed us to better estimate the relationships between specific strategies and performance. This approach was important, given concerns raised by others that in classroom studies, benefits of certain strategies, such as explanation, could simply have been due to greater total study time ( Dunlosky et al. , 2013 ). Our results showed that, even when controlling for total study time, self-explanation and other strategies were still significant predictors of performance. This helped illustrate that the strategies themselves, and not just the time on task, were important considerations of students’ study habits.
Third, we were surprised by how predictive the diversity of active strategies was of performance. While we found that the proportion of active study time and the number of active strategies were both important predictors of performance, we found that the latter was a stronger predictor. This suggests that, if total study time was held equal, students who used a larger number of active strategies tended to perform better than those that used a smaller number of active strategies. This finding also deserves to be followed up in subsequent study to determine whether any of the active strategies that students use tend to co-occur in a “suite,” and whether any of those suites are particularly predictive of performance. We suspect that there is some limit to the benefit of using diverse strategies, as some strategies take a considerable amount of time to master ( Bean and Steenwyk, 1984 ; Armbruster et al. , 1987 ; Wong et al. , 2002 ), and students need to devote enough time to each strategy to learn how to use it well.
Additionally, we found that particular active study strategies—explanation, self-quizzing, and answering problem sets—were uniquely predictive of higher performance in a biology course context. Undergraduate biology courses introduce a large amount of discipline-specific terminology, in addition to requiring the higher-order prediction and application skills found among STEM courses ( Wandersee, 1988 ; Zukswert et al. , 2019 ). This is true for the course studied here, which covers biochemistry and molecular genetics, and the assessments that we used as our outcomes reflect this combination of terminology, comprehension, prediction, and application skills. Our results support the finding that active, effortful strategies can be effective on a variety of cognitive levels ( Butler, 2010 ; Karpicke and Blunt, 2011 ; Smith and Karpicke, 2014 ); and this work extends support of the desirable difficulties framework into biology by finding unique value for distinct generative or testing strategies.
Study Timing (Hypothesis 2)
Inconsistent with our second hypothesis that students with less spacing potential would perform worse than students with more spacing potential, we found no relationship between study timing and performance on in-term or cumulative exams. Because we knew that spacing was difficult to estimate, we analyzed two spacing potential indices, the degree of cramming (i.e., the number of days in advance that students started studying) and the consistency of studying (i.e., the number of days studied in the week leading up to the exam). We controlled for total study time, because the spacing effect is defined as identical study time spread over multiple sessions rather than fewer, massed sessions. When doing so, neither of these measures were significantly related to performance.
There are a few possible explanations why we may not have observed a “spacing effect.” First, as explained in the Introduction , we measured spacing potential. It could be that students with high spacing potential may have arranged their studies to mass studying each topic, rather than spacing it out, which would lead us to underestimate the spacing effect. Second, students likely studied again before our cumulative final. This delayed test is where we expected to see the largest effect, and restudying may have masked any spacing effect that did exist. Third, we asked students to directly report their study time, and some may have struggled to remember the exact dates that they studied. While this has the advantage that it results in more sensitive and direct measures of students’ spacing potential than asking students to interpret for themselves whether they binarily spaced their studies or crammed ( Hartwig and Dunlosky, 2012 ; Rodriguez et al. , 2018 ), students who did not remember their study schedules may have reported idealized study schedules with greater spacing, rather than realistic schedules with more cramming ( Susser and McCabe, 2013 ), thus minimizing the expected spacing effect.
Despite the lack of a spacing effect in our data, we certainly do not advocate that students cram their studying, as we find it likely that students who started studying earlier may also have tended to study more. Also, those same students who studied earlier may have felt less stressed and gotten more sleep. In other words, even though our estimation of spacing potential did not capture performance benefits, benefits of spacing for well-being may be multifaceted and not wholly captured by our study.
Distraction (Hypothesis 3)
Consistent with our third hypothesis, we found a negative relationship between distraction while studying and performance. This finding agreed with the few available studies that related distraction during self-directed out-of-class studying and grade, but differs in that our students reported a lower level of distraction than other published studies ( Junco, 2012 ; Junco and Cotten, 2012 ). One possible reason for our low distraction estimate may have been that students were inadvertently underestimating their distraction, as has been reported ( Kraushaar and Novak, 2010 ). In addition, some students may not have been including multitasking as a type of distraction, and this habit of multitasking while studying will likely be difficult to change, as students tend to underestimate how negatively it will affect performance ( Calderwood et al. , 2016 ).
Implications for Instruction
To encourage students to use more active study strategies, try asking students to turn in the output of the strategy as a low-stakes assignment. For example, to encourage self-explanation, you could ask students to turn in a short video of themselves verbally explaining a concept for credit. To encourage practice quizzing, try to publish or reopen quizzes near exam time ( Walck-Shannon et al. , 2019 ) and ask students to complete them for credit.
To encourage students to use active study strategies effectively, model those strategies during class. For example, when doing a clicker question, explicitly state your approach to answering the question and self-explain your reasoning out loud. This also gives you an opportunity to add the rationale for why certain strategies are effective or provide advice about carrying them out. In addition to modeling a strategy, remind students to do it often. Simply prompting students to explain their reasoning to their neighbors or themselves during a clicker question helps shifts students’ conversations toward explanation ( Knight et al. , 2013 ).
To encourage students to stay focused during studying, provide voluntary, structured study sessions. These could include highly structured peer-led team-learning sessions during which students work through a packet of new questions ( Hockings et al. , 2008 ; Snyder et al. , 2015 ) or more relaxed sessions during which students work through problems that have already been provided ( Kudish et al. , 2016 ).
Limitations and Future Directions
There are multiple caveats to these analyses, which may be addressed in future studies. First, our data about study behaviors were self-reported. While we opened the reflection exercise immediately after the exam to mitigate students forgetting their behaviors, some may still have misremembered. Further, some students may not have forgotten, but rather were unable to accurately self-report certain behaviors. As stated earlier, one behavior that is especially prone to this is distraction. But, similarly, we suspect that some students had trouble estimating the percent of study time that they spent using each strategy, while their binary report of whether they used it or not may be more accurate. This may be one reason why the number of active strategies has more explanatory power than the percent of time using an active strategy. Separately, although students were told that we would not analyze their responses until after the semester had ended, some may have conformed their responses to what they thought was desirable. However, there is not strong evidence that students conform their study habit responses to their beliefs about what is effective. For example, Blasiman and colleagues found that, even though students believed rereading was an ineffective strategy, they still reported using it more than other strategies ( Blasiman et al. , 2017 ). Another limitation due to self-reporting is that we lack knowledge of the exact, nuanced behaviors that a student carried out. Thus, a student who chose a strategy that we defined as active—such as “completing problem sets”—may have actually performed more passive behaviors. Specifically, while we did use verbal reminders and delay the release of a key when encouraging students to complete the problem sets and old exams before looking at the answers, some students may have looked up answers prematurely or may have read passively through portions of the key. These more passive behaviors may have underestimated the importance of active strategies. A second limitation is that these data were collected from a course at a selective research-intensive institution and may not be applicable to all student populations. A third limitation is that our analyses are correlational. While we have carefully selected potential confounds, there may be other important confounding variables that we did not account for. Finally, it was beyond the scope of this study to ask whether certain subgroups of students employed different strategies or whether strategies were more or less predictive of performance for different subgroups of students.
Despite these caveats, the main point is clear. Students’ course-specific study habits predict their performance. While many students in our sample reported using effective strategies, some students still had room to improve, especially with their level of distraction. One open question that remains is how we can encourage these students to change their study habits over time.

ACKNOWLEDGMENTS
We would like to thank April Bednarski, Kathleen Weston-Hafer, and Barbara Kunkel for their flexibility and feedback on the exam reflection exercises. We would also like to acknowledge Grace Yuan and Ashton Barber for their assistance categorizing exam questions. This research was supported in part by an internal grant titled “Transformational Initiative for Educators in STEM,” which aimed to foster the adoption of evidence-based teaching practices in science classrooms at Washington University in St. Louis.
- Armbruster, B. B., Anderson, T. H., & Ostertag, J. ( 1987 ). Does text structure/summarization instruction facilitate learning from expository text? Reading Research Quarterly , 22 (3), 331. Google Scholar
- Bean, T. W., & Steenwyk, F. L. ( 1984 ). The effect of three forms of summarization instruction on sixth graders’ summary writing and comprehension . Journal of Reading Behavior , 16 (4), 297–306. Google Scholar
- Berry, D. C. ( 1983 ). Metacognitive experience and transfer of logical reasoning . Quarterly Journal of Experimental Psychology Section A , 35 (1), 39–49. Google Scholar
- Bjork, E. L., & Bjork, R. A. ( 2011 ). Making things hard on yourself, but in a good way: Creating desirable difficulties to enhance learning . In Gernsbacher, M. A.Pew, R. W.Hough, L. M.Pomerantz, J. R. (Eds.) & FABBS Foundation, Psychology and the real world: Essays illustrating fundamental contributions to society (pp. 56–64). New York, NY: Worth Publishers. Google Scholar
- Bjork, R. A. ( 1994 ). Memory and metamemory considerations in the training of human beings . In Metcalfe, J.Shimamura, A. P. (Eds.), Metacognition: Knowing about knowing (pp. 185–205). Cambridge, MA: Worth Publishers. Google Scholar
- Blasiman, R. N., Dunlosky, J., & Rawson, K. A. ( 2017 ). The what, how much, and when of study strategies: Comparing intended versus actual study behaviour . Memory , 25 (6), 784–792. Medline , Google Scholar
- Bretzing, B. H., & Kulhavy, R. W. ( 1979 ). Notetaking and depth of processing . Contemporary Educational Psychology , 4 (2), 145–153. Google Scholar
- Butler, A. C. ( 2010 ). Repeated testing produces superior transfer of learning relative to repeated studying . Journal of Experimental Psychology: Learning, Memory, and Cognition , 36 (5), 1118–1133. Medline , Google Scholar
- Calderwood, C., Green, J. D., Joy-Gaba, J. A., & Moloney, J. M. ( 2016 ). Forecasting errors in student media multitasking during homework completion . Computers and Education , 94 , 37–48. Google Scholar
- Carpenter, S. K. ( 2009 ). Cue strength as a moderator of the testing effect: The benefits of elaborative retrieval . Journal of Experimental Psychology: Learning, Memory, and Cognition , 35 (6), 1563–1569. Medline , Google Scholar
- Credé, M., & Kuncel, N. R. ( 2008 ). Study habits, skills, and attitudes: The third pillar supporting collegiate academic performance . Perspectives on Psychological Science , 3 (6), 425–453. Medline , Google Scholar
- Credé, M., Roch, S. G., & Kieszczynka, U. M. ( 2010 ). Class attendance in college . Review of Educational Research , 80 (2), 272–295. Google Scholar
- Crowe, A., Dirks, C., & Wenderoth, M. P. ( 2008 ). Biology in Bloom: Implementing Bloom’s taxonomy to enhance student learning in biology . CBE—Life Sciences Education , 7 (4), 368–381. Link , Google Scholar
- Dunlosky, J., Rawson, K. A., Marsh, E. J., Nathan, M. J., & Willingham, D. T. ( 2013 ). Improving students’ learning with effective learning techniques . Psychological Science in the Public Interest , 14 (1), 4–58. Medline , Google Scholar
- Gump, S. E. ( 2005 ). The cost of cutting class: Attendance as a predictor of success . College Teaching , 53 (1), 21–26. Google Scholar
- Hartwig, M. K., & Dunlosky, J. ( 2012 ). Study strategies of college students: Are self-testing and scheduling related to achievement? Psychonomic Bulletin & Review , 19 (1), 126–134. Medline , Google Scholar
- Hockings, S. C., DeAngelis, K. J., & Frey, R. F. ( 2008 ). Peer-led team learning in general chemistry: Implementation and evaluation . Journal of Chemical Education , 85 (7), 990. Google Scholar
- Hora, M. T., & Oleson, A. K. ( 2017 ). Examining study habits in undergraduate STEM courses from a situative perspective . International Journal of STEM Education , 4 (1), 1. Google Scholar
- Jacoby, L. L. ( 1978 ). On interpreting the effects of repetition: Solving a problem versus remembering a solution . Journal of Verbal Learning and Verbal Behavior , 17 (6), 649–667. Google Scholar
- Junco, R. ( 2012 ). Too much face and not enough books: The relationship between multiple indices of Facebook use and academic performance . Computers in Human Behavior , 28 (1), 187–198. Google Scholar
- Junco, R., & Cotten, S. R. ( 2012 ). No A 4 U: The relationship between multitasking and academic performance . Computers & Education , 59 (2), 505–514. Google Scholar
- Karpicke, J. D., & Blunt, J. R. ( 2011 ). Retrieval practice produces more learning than elaborative studying with concept mapping . Science , 331 (6018), 772–775. Medline , Google Scholar
- Karpicke, J. D., Butler, A. C., & Roediger, H. L. ( 2009 ). Metacognitive strategies in student learning: Do students practise retrieval when they study on their own? Memory , 17 (4), 471–479. Medline , Google Scholar
- Karpicke, J. D., & Roediger, H. L. ( 2008 ). The critical importance of retrieval for learning . Science , 319 (5865), 966–968. Medline , Google Scholar
- King, A. ( 1992 ). Comparison of self-questioning, summarizing, and notetaking-review as strategies for learning from lectures . American Educational Research Journal , 29 (2), 303. Google Scholar
- Knight, J. K., Wise, S. B., & Southard, K. M. ( 2013 ). Understanding clicker discussions: Student reasoning and the impact of instructional cues . CBE—Life Sciences Education , 12 (4), 645–654. Link , Google Scholar
- Kornell, N., & Bjork, R. A. ( 2007 ). The promise and perils of self-regulated study . Psychonomic Bulletin & Review , 14 (2), 219–224. Medline , Google Scholar
- Kraushaar, J. M., & Novak, D. ( 2010 ). Examining the effects of student multitasking with laptops during the lecture . Journal of Information Systems Education , 21 (2), 241–251. Google Scholar
- Kudish, P., Shores, R., McClung, A., Smulyan, L., Vallen, E. A., & Siwicki, K. K. ( 2016 ). Active learning outside the classroom: Implementation and outcomes of peer-led team-learning workshops in introductory biology . CBE—Life Sciences Education , 15 (3), ar31. Link , Google Scholar
- Lin, T. F., & Chen, J. ( 2006 ). Cumulative class attendance and exam performance . Applied Economics Letters , 13 (14), 937–942. Google Scholar
- Marsh, E. J., & Butler, A. C. ( 2013 ). Memory in educational settings . In Reisberg, D. (Ed.), Oxford library of psychology. The Oxford handbook of cognitive psychology (pp. 299–317). Washington, DC: Oxford University Press. Google Scholar
- May, K. E., & Elder, A. D. ( 2018 ). Efficient, helpful, or distracting? A literature review of media multitasking in relation to academic performance . International Journal of Educational Technology in Higher Education , 15 (1), 13. Google Scholar
- Padilla-Walker, L. M., Thompson, R. A., Zamboanga, B. L., & Schmersal, L. A. ( 2005 ). Extra credit as incentive for voluntary research participation . Teaching of Psychology , 32 (3), 150–153. Google Scholar
- Pressley, M., McDaniel, M. A., Turnure, J. E., Wood, E., & Ahmad, M. ( 1987 ). Generation and precision of elaboration: Effects on intentional and incidental learning . Journal of Experimental Psychology: Learning, Memory, and Cognition , 13 (2), 291–300. Google Scholar
- Rawson, K. A., & Kintsch, W. ( 2005 ). Rereading effects depend on time of test . Journal of Educational Psychology , 97 (1), 70–80. Google Scholar
- Rodriquez, F., Rivas, M. J., Matsumura, L. H., Warschauer, M., & Sato, B. K. ( 2018 ). How do students study in STEM courses? Findings from a light-touch intervention and its relevance for underrepresented students . PLoS ONE , 13 (7), e0200767. Medline , Google Scholar
- Sebesta, A. J., & Bray Speth, E. ( 2017 ). How should I study for the exam? Self-regulated learning strategies and achievement in introductory biology . CBE—Life Sciences Education , 16 (2), ar30. Link , Google Scholar
- Smith, M. A., & Karpicke, J. D. ( 2014 ). Retrieval practice with short-answer, multiple-choice, and hybrid tests . Memory , 22 (7), 784–802. Medline , Google Scholar
- Snyder, J. J., Elijah Carter, B., & Wiles, J. R. ( 2015 ). Implementation of the peer-led team-learning instructional model as a stopgap measure improves student achievement for students opting out of laboratory . CBE—Life Sciences Education , 14 (1), ar2. Link , Google Scholar
- Stanton, J. D., Neider, X. N., Gallegos, I. J., & Clark, N. C. ( 2015 ). Differences in metacognitive regulation in introductory biology students: When prompts are not enough . CBE—Life Sciences Education , 14 (2), ar15. Link , Google Scholar
- Susser, J. A., & McCabe, J. ( 2013 ). From the lab to the dorm room: Metacognitive awareness and use of spaced study . Instructional Science , 41 (2), 345–363. Google Scholar
- Tomanek, D., & Montplaisir, L. ( 2004 ). Students’ studying and approaches to learning in introductory biology . Cell Biology Education , 3 (4), 253–262. Link , Google Scholar
- Walck-Shannon, E. M., Cahill, M. J., McDaniel, M. A., & Frey, R. F. ( 2019 ). Participation in voluntary re-quizzing is predictive of increased performance on cumulative assessments in introductory biology . CBE—Life Sciences Education , 18 (2), ar15. Link , Google Scholar
- Wandersee, J. H. ( 1988 ). The terminology problem in biology education: A reconnaissance . American Biology Teacher , 50 (2), 97–100. Google Scholar
- Westrick, P. A., Le, H., Robbins, S. B., Radunzel, J. M. R., & Schmidt, F. L. ( 2015 ). College performance and retention: A meta-analysis of the predictive validities of ACT® scores, high school grades, and SES . Educational Assessment , 20 (1), 23–45. Google Scholar
- Wong, R. M. F., Lawson, M. J., & Keeves, J. ( 2002 ). The effects of self-explanation training on students’ problem solving in high-school mathematics . Learning and Instruction , 12 (2), 233–262. Google Scholar
- Zimmerman, B. J. ( 2002 ). Becoming a self-regulated learner: An overview . Theory into Practice , 41 (2), 64–70. Google Scholar
- Zukswert, J. M., Barker, M. K., & McDonnell, L. ( 2019 ). Identifying troublesome jargon in biology: Discrepancies between student performance and perceived understanding . CBE—Life Sciences Education , 18 (1), ar6. Link , Google Scholar
- Causes and outcomes of at-risk underperforming pharmacy students: implications for policy and practice 19 April 2024 | BMC Medical Education, Vol. 24, No. 1
- The impact of study habits and personal factors on the academic achievement performances of medical students 19 August 2024 | BMC Medical Education, Vol. 24, No. 1
- Analyzing the Impact of Time Spent on Practice Questions on General Chemistry Students’ Problem-Solving Performance 30 July 2024 | Journal of Chemical Education, Vol. 101, No. 8
- Study strategy use among elementary school students: Is use of specific study strategies related to academic performance? 2 March 2024 | Metacognition and Learning, Vol. 19, No. 2
- Riding the wave towards flourishing in STEM education: Enhancing teaching efficacy through a K-12 training program 1 Jun 2024 | Teaching and Teacher Education, Vol. 143
- Medical Students’ Study Habits Through a Sociocultural Lens: A Systematic Literature Review 12 April 2024 | International Journal of Medical Students, Vol. 12, No. 1
- Exploring the link between perceived physical literacy and academic performance outcomes: insights from the EHDLA study 24 January 2024 | Frontiers in Sports and Active Living, Vol. 6
- Factors that influence general chemistry students’ decision making in study strategies 1 January 2024 | Chemistry Education Research and Practice
- Breaking the mold: Study strategies of students who improve their achievement on introductory biology exams 3 July 2023 | PLOS ONE, Vol. 18, No. 7
- The impact of effective study strategy use in an introductory anatomy and physiology class 31 May 2023 | Frontiers in Education, Vol. 8
- Assessing Learner Engagement and the Impact on Academic Performance within a Virtual Learning Environment 15 February 2023 | Pharmacy, Vol. 11, No. 1
- Which course resources and student approaches to learning are related to higher grades in introductory biology? 1 Jan 2023 | Journal of Research in Science, Mathematics and Technology Education
- Sleep Deprivation and Study Habits Effects toward Medical Imaging Students, UiTM Puncak Alam 30 September 2022 | Environment-Behaviour Proceedings Journal, Vol. 7, No. 21
- Evaluating open-note exams: Student perceptions and preparation methods in an undergraduate biology class 18 August 2022 | PLOS ONE, Vol. 17, No. 8
- Eight Recommendations to Promote Effective Study Habits for Biology Students Enrolled in Online Courses 29 Apr 2022 | Journal of Microbiology & Biology Education, Vol. 23, No. 1
- Analysis of Learning and Academic Performance of Education Students Before and During the Coronavirus Disease Pandemic 15 October 2021 | European Journal of Educational Research, Vol. volume-10-2021, No. volume-10-issue-4-october-2021
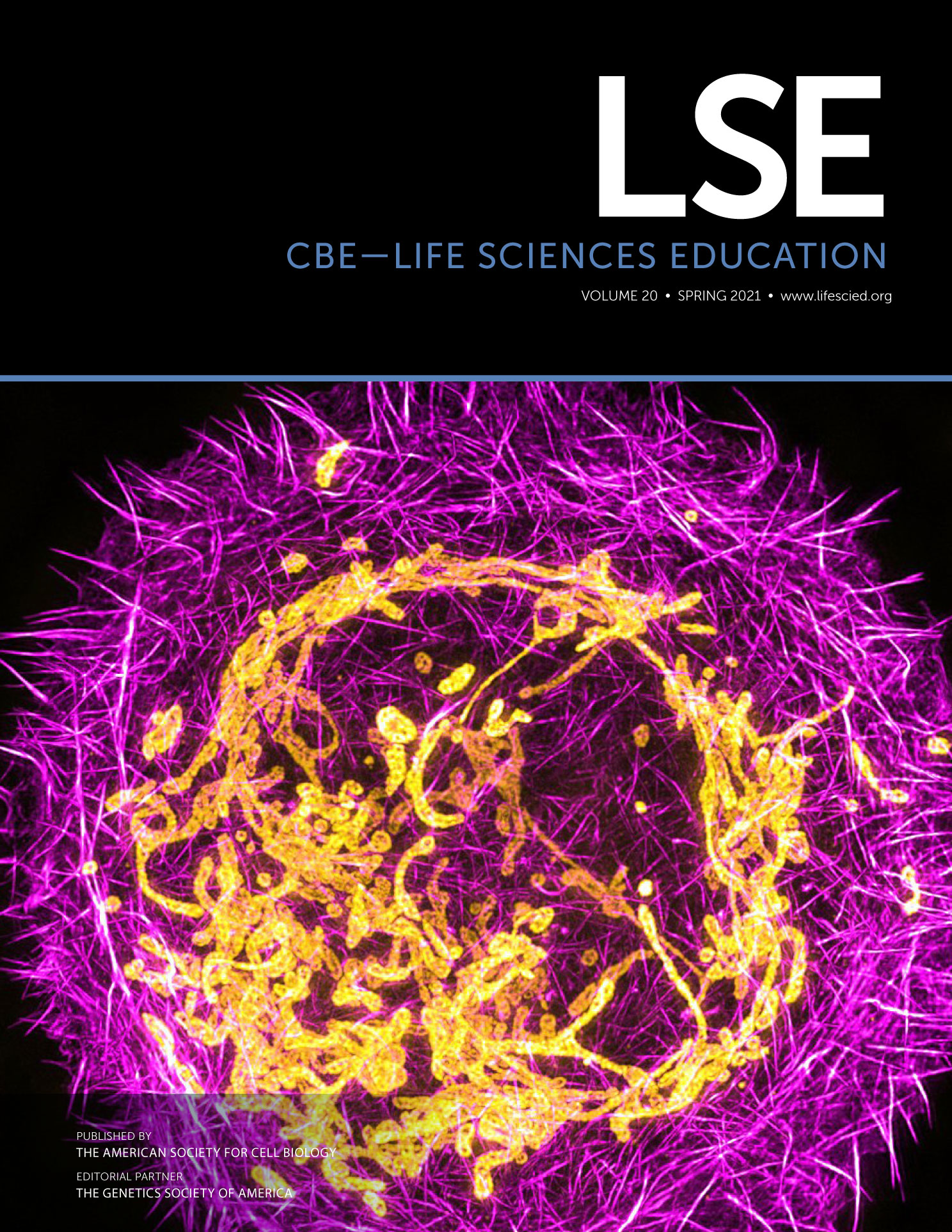
Submitted: 19 May 2020 Revised: 19 October 2020 Accepted: 22 October 2020
© 2021 E. M. Walck-Shannon et al. CBE—Life Sciences Education © 2021 The American Society for Cell Biology. This article is distributed by The American Society for Cell Biology under license from the author(s). It is available to the public under an Attribution–Noncommercial–Share Alike 3.0 Unported Creative Commons License (http://creativecommons.org/licenses/by-nc-sa/3.0).
- DOI: 10.31305/rrijm.2021.v06.i05.019
- Corpus ID: 242541756
Study Habits and Academic Performance among Students: A Systematic Review
- Published in RESEARCH REVIEW International… 15 June 2021
- Education, Psychology
2 Citations
Study habits and academic performance among public junior high school pupils in the ekumfi district: investigating the controlling effect of learning styles, analysis of learning and academic performance of education students before and during the coronavirus disease pandemic, 32 references, factors affecting the student’s study habit, study habits, skills, and attitudes: the third pillar supporting collegiate academic performance, relationship among self-concept , study habits and academic achievement of prence students in zamfara state college of education , nigeria, influence of study habits on the academic performance of physics students in federal university of agriculture makurdi, nigeria, academic stress and self efficacy in relation to study habits among adolescents, a handbook of educational technology, handbook of college reading and study strategy research, impact of family climate, mental health, study habits and self confidence on the academic achievement of senior secondary students, influence of study habits on academic achievement of senior secondary school students in relation to gender and community, a study of emotional intelligence school adjustment and study habits of secondary school students in relation to their academic achievement in social science, related papers.
Showing 1 through 3 of 0 Related Papers
- Open access
- Published: 19 August 2024
The impact of study habits and personal factors on the academic achievement performances of medical students
- Mohammed A. Aljaffer 1 ,
- Ahmad H. Almadani 1 ,
- Abdullah S. AlDughaither 2 ,
- Ali A. Basfar 2 ,
- Saad M. AlGhadir 2 ,
- Yahya A. AlGhamdi 2 ,
- Bassam N. AlHubaysh 2 ,
- Osamah A. AlMayouf 2 ,
- Saleh A. AlGhamdi 3 ,
- Tauseef Ahmad 4 &
- Hamza M. Abdulghani 5
BMC Medical Education volume 24 , Article number: 888 ( 2024 ) Cite this article
61 Accesses
2 Altmetric
Metrics details
Academic achievement is essential for all students seeking a successful career. Studying habits and routines is crucial in achieving such an ultimate goal.
This study investigates the association between study habits, personal factors, and academic achievement, aiming to identify factors that distinguish academically successful medical students.
A cross-sectional study was conducted at the College of Medicine, King Saud University, Riyadh, Saudi Arabia. The participants consisted of 1st through 5th-year medical students, with a sample size of 336. The research team collected study data using an electronic questionnaire containing three sections: socio-demographic data, personal characteristics, and study habits.
The study results indicated a statistically significant association between self-fulfillment as a motivation toward studying and academic achievement ( p = 0.04). The results also showed a statistically significant correlation between recalling recently memorized information and academic achievement ( p = 0.05). Furthermore, a statistically significant association between preferring the information to be presented in a graphical form rather than a written one and academic achievement was also found ( p = 0.03). Students who were satisfied with their academic performance had 1.6 times greater chances of having a high-grade point average (OR = 1.6, p = 0.08).
The results of this study support the available literature, indicating a correlation between study habits and high academic performance. Further multicenter studies are warranted to differentiate between high-achieving students and their peers using qualitative, semi-structured interviews. Educating the students about healthy study habits and enhancing their learning skills would also be of value.
Peer Review reports
Introduction
Academic performance is a common indicator used to measure student achievement [ 1 , 2 ]. It is a compound process influenced by many factors, among which is study habits [ 2 , 3 ]. Study habit is defined as different individual behavior in relation to studying, and is a combination of study methods and skills [ 2 , 3 , 4 ]. Put differently, study habits involve various techniques that would increase motivation and transform the study process into an effective one, thus enhancing learning [ 5 ]. Students’ perspectives and approaches toward studying were found to be the key factors in predicting their academic success [ 6 , 7 ]. However, these learning processes vary from one student to another due to variations in the students’ cognitive processing [ 8 ].
The study habits of students are the regular practices and habits they exhibit during the learning process [ 9 , 10 ]. Over time, several study habits have been developed, such as time management, setting appropriate goals, choosing a comfortable study environment, taking notes effectively, choosing main ideas, and being organized [ 11 ]. Global research shows that study habits impact academic performance and are the most important predictor of it [ 12 ]. It is difficult for medical students to organize and learn a lot of information, and they need to employ study skills to succeed [ 1 , 2 , 5 , 13 ].
Different lifestyle and social factors could affect students’ academic performance. For instance, Jafari et al. found that native students had better study habits compared to dormitory students [ 1 ]. This discrepancy between native and dormitory students was also indicated by Jouhari et al. who illustrated that dormitory students scored lower in attitude, test strategies, choosing main ideas, and concentration [ 10 ]. Regarding sleeping habits, Curcio G et al. found that students with a regular and adequate sleeping pattern had higher Grade Point Average (GPA) scores [ 14 ]. Lifestyle factors, such as watching television and listening to music, were shown to be unremarkable in affecting students’ grades [ 15 , 16 ]. Social media applications, including WhatsApp, Facebook, and Twitter, distract students during learning [ 16 , 17 ].
Motivation was found to be a major factor in students’ academic success. Bonsaksen et al. found that students who chose “to seek meaning” when studying were associated with high GPA scores [ 18 ]. In addition, low scores on “fear of failure” and high scores on “achieving” correlated with a higher GPA [ 8 , 18 ].
Resource-wise, Alzahrani et al. found that 82.7% of students relied on textbooks assigned by the department, while 46.6% mainly relied on the department’s lecture slides [ 19 ]. The study also indicated that 78.8% perceived that the scientific contents of the lectures were adequate [ 19 ]. Another study found that most students relied on the lecture slides (> 83%) along with their notes, followed by educational videos (76.1%), and reference textbooks (46.1%) [ 20 ]. Striking evidence in that study, as well as in another study, indicated that most students tended to avoid textbooks and opted for lecture slides, especially when preparing for exams [ 20 , 21 ].
Several researchers studied the association between different factors and academic performance; however, more is needed to know about this association in the process of education among medical students [ 15 , 20 , 22 ], with some limitations to the conducted studies. Such limitations include the study sample and using self-reported questionnaires, which may generate inaccurate results. Moreover, in Saudi Arabia in particular, the literature concerning the topic remains limited. Since many students are unsatisfied with their performance and seek improvement [ 10 ], the present study was designed and conducted.
Unlike other studies in the region, this study aims to investigate the relationship between study habits and personal factors and measure their influence on academic achievement. The results of this study could raise awareness regarding the effect of study habits and personal factors on students’ performance and would also guide them toward achieving academic success. The study also seeks to identify the factors that distinguish academically successful students from their peers.
Study design, setting, and participants
This observational cross-sectional study, which took place between June and December 2022, was conducted among students attending the College of Medicine at King Saud University (KSU), Riyadh, Saudi Arabia. Its targeted population included all male and female medical students (first to fifth years) attending KSU during the academic year 2021/2022. Whereas, students at other colleges and universities, those who failed to complete the questionnaire, interns (the students who already graduated), and those who were enrolled in the university’s preparatory year, were all excluded from the current study. The sample size was calculated based on a study conducted in 2015 by Lana Al Shawwa [ 15 ]. Using the sample size formula for a single proportion (0.79), the required sample size was 255 using a confidence interval of 95% and a margin of error of 5%. After adding a 20% margin to accommodate non-responses and incomplete responses, the calculated sample size required for this study was 306. However, our research team collected a total of 336 participants for this study to ensure complete representation.
Study instrument
The research team developed and used an electronic questionnaire. The rationale is that no standardized questionnaire measuring the study objectives was found in the literature. However, the questionnaire was tested on a pilot of 15 students to test its clarity and address any possible misconceptions and ambiguity. The study questionnaire was distributed randomly to this cohort, who were asked to fill out the questionnaire. The students reported a complete understanding of the questionnaire’s contents, so the same questionnaire was used without any modifications. The questionnaire, written in English, consisted of three parts. The first part included eleven questions about the socio-demographic status of the participants. The second part contained twenty-one questions examining personal factors such as sleep and caffeine consumption. The last part included twenty-one questions regarding students’ study habits. The questionnaire was constructed based on an ordinal Likert scale which had: strongly agree, agree, neutral, disagree, and strongly disagree as possible answers. The questionnaire was sent to participants through email and social media applications like Twitter and WhatsApp to increase the study response. An informed consent that clearly states the study’s purpose was taken from all participants at the beginning of the questionnaire. In addition, all participants were assured that the collected data would be anonymous and confidential. Each participant was represented by a code for the sole purpose of analyzing the data. Furthermore, no incentives or rewards were given to the participants for their participation.
Study variables
Socio-demographic information (such as age, gender, and academic year), and personal factors (such as motivation, sleeping status, caffeine consumption, and self-management) were the independent variables. Study habits such as attendance, individual versus group study, memorization techniques, revision, learning style, and strategies were also independent variables.
Academic achievement refers to a student’s success in gaining knowledge and understanding in various subjects, as well as the ability to apply that knowledge effectively [ 23 ]. It is a measure of the student’s progress throughout the educational journey, encompassing both academic achievements and personal growth [ 3 , 24 ]. Academic achievement is judged based on the student’s GPA or performance score. In this study, students’ GPA scores, awareness, and satisfaction regarding their academic performance were the dependent variables.
We divided the study sample into two groups based on the GPA. We considered students with high GPAs to be exposed (i.e. exposed to the study habits we are investigating), and students with low GPAs to be the control group. The purpose of this study was to determine why an exposed group of students gets high grades and what study factors they adopt. Based on this exposure (high achieving students), we concluded what methods they used to achieve higher grades. Those in the first group had a GPA greater or equal to 4.5 (out of 5), while those in the second group had a GPA less than 4.5. The students’ data were kept confidential and never used for any other purpose.
Data analysis
The data collected were analyzed by using IBM SPSS Statistical software for Windows version 24.0. Descriptive statistics such as frequency and percentage were used to describe the socio-demographic data in a tabular form. Furthermore, data for categorical variables, including different study habits, motivation factors, memorizing and revising factors, and lifestyle factors, were tabulated and analyzed using the odds ratio test. Finally, we calculated the odds ratio statistic and a p-value of 0.05 to report the statistical significance of our results.
Ethical approval and consent to Participate
Before conducting the study, the research team obtained the Ethics Committee Approval from the Institutional Review Board of the College of Medicine, KSU, Riyadh, Saudi Arabia (project No. E-22-7044). Participants’ agreement/consent to participate was guaranteed by choosing “agree” after reading the consent form at the beginning of the questionnaire. Participation was voluntary, and consent was obtained from all participants. The research team carried out all methods following relevant guidelines and regulations.
The total 336 medical students participated in the study. All participants completed the study questionnaire, and there were no missing or incomplete data, with all of them being able to participate. As shown in Table 1 9.3% of participants were between 18 and 20, 44.9% were between the ages of 21 and 22, and 35.8% were 23–28 years old. In the current study, 62.5% of the participants were males and 37.5% were females. The proportion of first-year students was 21.4%, 20.8% of second-year students, 20.8% of third-year students, 18.2% of fourth-year students, and 18.8% of fifth-year students, according to academic year levels. Regarding GPA scores, 36.9% scored 4.75-5 and 32.4% scored 4.5–4.74. 23.8% achieved 4-4.49, 6.5% achieved 3-3.99, and only 0.4% achieved 2.99 or less. Participants lived with their families in 94.6% of cases, with friends in 1.2% of cases, and alone in 4.2% of cases. For smoking habits, 86.3% did not smoke, 11% reported using vapes, 2.1% used cigarettes, and 0.6% used Shisha. 91.4% of the participants did not report any chronic illnesses; however, 8.6% did. In addition, 83% had no mental illness, 8.9% had anxiety, 6% had depression, and 2.1% reported other mental illnesses.
Table 2 shows motivational factors associated with academic performance. There was a clear difference in motivation factors between students with high and low achievement in the current study. Students with high GPAs were 1.67 times more motivated toward their careers (OR = 1.67, p = 0.09) than those with low GPAs. Furthermore, significant differences were found between those students who had self-fulfillment or ambitions in life they had ~ 2 times higher (OR = 1.93, p = 0.04) GPA scores than low GPA students. Exam results did not motivate exposed or high GPA students (46%) or control students with low GPA students (41%), but the current study showed test results had little impact on low achiever students (OR = 1.03, p = 0.88). Furthermore, 72.6% of high achievers were satisfied with their academic performance, while only 41% of low achiever students were satisfied. Therefore, students who were satisfied with their academic performance had 1.6 times greater chances of a higher GPA (OR = 1.6, p = 0.08). Students who get support and help from those around them are more likely to get high GPAs (OR = 1.1, p = 0.73) than those who do not receive any support. When students reported feeling a sense of family responsibility, the odds (odds ratio) of their receiving higher grades were 1.15 times higher (OR = 1.15, p = 0.6) compared to those who did not feel a sense of family responsibility. The p-value, which indicates the level of statistical significance, was 0.6.
Table 3 shows the study habits of higher achiever students and low achiever students. Most of the high-achieving students (79.0%) attended most of the lectures and had 1.6 times higher chances of getting higher grades (OR = 1.6, p = 0.2) than those who did not attend regular lectures. The current study found that studying alone had no significant impact on academic achievement in either group. However, those students who had studied alone had lower GPAs (OR = 1.07, p = 0.81). The current study findings reported 29.8% of students walk or stand while studying rather than sit, and they had 1.57 times higher GPA chances compared to students with lower GPAs (OR = 0.73, p = 0.27). High achievers (54.0%) preferred studying early in the morning, and these students had higher chances of achieving good GPAs (OR = 1.3, p = 0.28) than low achiever groups of students. The number of students with high achievement (39.5%) went through the lecture before the lesson was taught. These students had 1.08 times higher chances of achieving than low achiever groups of students. Furthermore, students who made a weekly study schedule had 1.3 times higher chances of being good academic achievers than those who did not (OR = 1.3, p = 0.37). Additionally, high-achieving students paid closer attention to the lecturer (1.2 times higher). In addition, students with high GPAs spent more time studying when exam dates approached (OR = 1.3, p = 0.58).
Table 4 demonstrates the relationship between memorizing and revising with high and low GPA students. It was found that high achiever students (58.9%) studied lectures daily and had 1.4 times higher chances of achieving high grades (OR = 1.4, p = 0.16) than the other group. It was found that most of the high achievers (62.1%) skim the lecture beforehand before memorizing it, which led to 1.8 times higher chances of getting good grades in this exam (OR = 1.8, p = 0.06). One regular activity reported by high GPA students (82.3%) was recalling what had just been memorized. For this recalling technique, we found a significant difference between low-achieving students (OR = 0.8, p = 0.63) and high-achieving students (OR = 1.83, p = 0.05). A high achiever student writes notes before speaking out for the memorizing method, which gives 1.2 times greater chances of getting high grades (OR = 1.2, p = 0.55) than a student who does not write notes. A major difference in the current study was that high GPA achievers (70.2%) revise lectures more frequently than low GPA achievers (57.1%). They had 1.5 times more chances of getting high grades if they practiced and revised this method (OR = 1.5, p = 0.13).
Table 5 illustrates the relationship between negative lifestyle factors and students’ academic performance. The current study found that students are less likely to get high exam grades when they smoke. Students who smoke cigarettes and those who vape are 1.14 and 1.07 times respectively more likely to have a decrease in GPA than those who do not smoke. Those students with chronic illnesses had 1.22 times higher chances of a downgrade in the exam (OR = 1.22, p = 0.49). Additionally, students with high GPAs had higher mental pressures (Anxiety = 1.2, Depression = 1.18, and other mental pressures = 1.57) than those with low GPAs.
Learning is a multifaceted process that evolves throughout our lifetimes. The leading indicator that sets students apart is their academic achievement. Hence, it is crucial to investigate the factors that influence it. The present study examined the relationship between different study habits, personal characteristics, and academic achievement among medical students. In medical education, and more so in Saudi Arabia, there needs to be more understanding regarding such vital aspects.
Regarding motivational factors, the present study found some differences between high and low achievers. Students with high GPA scores were more motivated toward their future careers (OR = 1.67, p = 0.09). The study also indicated that students who had ambitions and sought self-fulfillment were more likely to have high GPA scores, which were statistically significant (OR = 1.93, p = 0.04). This was consistent with Bin Abdulrahman et al. [ 20 ], who indicated that the highest motivation was self-fulfillment and satisfying family dreams, followed by a high educational level, aspirations to join a high-quality residency program, and high income. Their study also found that few students were motivated by the desire to be regarded as unique students. We hypothesize that this probably goes back to human nature, where a highly rewarding incentive becomes the driving force of our work. Hence, schools should utilize this finding in exploring ways to enhance students’ motivation toward learning.
The present study did not find a significant effect of previous exam results on academic performance (OR = 1.03, p = 0.88). However, some studies reported that more than half of the high-achieving students admitted that high scores acquired on previous assessments are an important motivational factor [ 15 , 25 , 26 ]. We hypothesize that as students score higher marks, they become pleased and feel confident with their study approach. This finding shows how positive measurable results influence the students’ mentality.
The present study also explored the social environment surrounding medical students. The results indicated that those who were supported by their friends or family were slightly more likely to score higher GPAs (OR = 1.1, p = 0.73); however, the results did not reach a statistical significance. We hypothesize that a supportive and understanding environment would push the students to be patient and look for a brighter future. Our study results were consistent with previous published studies, which showed an association [ 3 , 27 , 28 , 29 , 30 ]. We hypothesize that students who spend most of their time with their families had less time to study, which made their study time more valuable. The findings of this study will hopefully raise awareness concerning the precious time that students have each day.
The association of different study habits among medical students with high and low GPAs was also studied in our study. It was noted that the high-achieving students try to attend their lectures compared to the lower achievers. This was in line with the previous published studies, which showed that significant differences were observed between the two groups regarding the attendance of lectures, tutorials, practical sessions, and clinical teachings [ 31 , 32 ]. The present study found that most students prefer to study alone, regardless of their level of academic achievement (82.1%). This finding is consistent with the study by Khalid A Bin Abdulrahman et al., which also showed that most students, regardless of their GPA, favored studying alone [ 20 ].
The present study findings suggest that a small number of students (29.8%) prefer to walk or stand while studying rather than sit, with most being high achievers (OR = 1.57, P = 0.15). A study reported that 40.3% of students with high GPAs seemed to favor a certain posture or body position, such as sitting or lying on the floor [ 15 ]. These contradictory findings might indicate that which position to adopt while studying should come down to personal preference and what feels most comfortable to each student. The present study also found that high achievers are more likely to prefer studying early in the morning (OR = 1.3, P = 0.28). The authors did not find similar studies investigating this same association in the literature. However, mornings might allow for more focused studying with fewer distractions, which has been shown to be associated with higher achievement in medical students [ 3 , 15 , 33 ].
Our study also found that 39.5% of the academically successful students reviewed pre-work or went through the material before they were taught it (OR = 1.08, p = 0.75), and 25% were neutral. Similar findings were reported in other studies, showing that academically successful students prepared themselves by doing their pre-work, watching videos, and revising slides [ 3 , 9 , 34 ]. Our study showed that 75% of high-achieving students tend to listen attentively to the lecturer (OR = 1.2, p = 0.48). Al Shawa et al. found no significant differences between the high achievers and low achievers when talking about attending lectures [ 15 ]. This could be due to the quality of teachers and the environment of the college or university.
Regarding the relationship between memorizing and revising with high and low GPA students, the present study found that students who study lectures daily are more likely to score higher than those who do not (OR = 1.4, p = 0.16). This finding is consistent with other studies [ 3 , 19 , 35 ]. For skimming lectures beforehand, an appreciable agreement was noted by high GPA students (62.1%), while only (42%) of low GPA students agreed to it. Similarly, previous published studies also found that highlighting and reading the content before memorization were both common among high-achieving students [ 15 , 36 ]. Furthermore, the present study has found recalling what has just been memorized to be statistically significantly associated with high GPA students (OR = 1.83, p = 0.05). Interestingly, we could not find any study that investigated this as an important factor, which could be justified by the high specificity of this question. Besides, when it comes to writing down/speaking out what has just been memorized, our study has found no recognizable differences between high-achieving students (75%) and low-achieving students (69%), as both categories had remarkably high percentages of reading and writing while studying.
The present study has found no statistical significance between regularly revising the lectures and high GPA ( p > 0.05), unlike the study conducted by Deborah A. Sleight et al. [ 37 ]. The difference in findings between our study and Deborah A. Sleight et al. might be due to a limitation of our study, namely the similar backgrounds of our participants. Another explanation could be related to curricular differences between the institutions where the two studies were conducted. Moreover, a statistically significant correlation between not preferring the data being presented in a written form instead of a graphical form and high GPA scores have been found in their study ( p < 0.05). However, a study conducted by Deborah A. Sleight et al. indicated that 66% of high achievers used notes prepared by other classmates compared to 84% of low achievers. Moreover, their study showed that only 59% of high achievers used tables and graphs prepared by others compared to 92% of low achievers. About 63% and 61% of the students in their study reported using self-made study aids for revision and memory aids, respectively [ 37 ].
The present study also examined the effects of smoking and chronic and mental illness, but found no statistical significance; the majority of both groups responded by denying these factors’ presence in their life. A similar finding by Al Shawwa et al. showed no statistical significance of smoking and caffeine consumption between low GPA and high GPA students [ 15 ]. We hypothesize that our findings occurred due to the study’s broad approach to examining such factors rather than delving deeper into them.
High-achieving students’ habits and factors contributing to their academic achievement were explored in the present study. High-achieving students were found to be more motivated and socially supported than their peers. Moreover, students who attended lectures, concentrated during lectures, studied early in the morning, prepared their weekly schedule, and studied more when exams approached were more likely to have high GPA scores. Studying techniques, including skimming before memorizing, writing what was memorized, active recall, and consistent revision, were adopted by high-achievers. To gain deeper insight into students’ strategies, it is recommended that qualitative semi-structured interviews be conducted to understand what distinguishes high-achieving students from their peers. Future studies should also explore differences between public and private university students. Additionally, further research is needed to confirm this study’s findings and provide guidance to all students. Future studies should collect a larger sample size from a variety of universities in order to increase generalizability.
Limitations and recommendations
The present study has some limitations. All the study’s findings indicated possible associations rather than causation; hence, the reader should approach the results of this study with caution. We recommend in-depth longitudinal studies to provide more insight into the different study habits and their impact on academic performance. Another limitation is that the research team created a self-reported questionnaire to address the study objectives, which carries a potential risk of bias. Hence, we recommend conducting interviews and having personal encounters with the study’s participants to reduce the risk of bias and better understand how different factors affect their academic achievement. A third limitation is that the research team only used the GPA scores as indicators of academic achievement. We recommend conducting other studies and investigating factors that cannot be solely reflected by the GPA, such as the student’s clinical performance and skills. Lastly, all participants included in the study share one background and live in the same environment. Therefore, the study’s findings do not necessarily apply to students who do not belong to such a geographic area and point in time. We recommend that future studies consider the sociodemographic and socioeconomic variations that exist among the universities in Saudi Arabia.
Availability of data materials
The datasets used and/or analyzed during the current study are available from the corresponding author on reasonable request.
Abbreviations
Grade Point Average
King Saud University
Institutional review board
Statistical package for the social sciences
Jafari H, Aghaei A, Khatony A. Relationship between study habits and academic achievement in students of medical sciences in Kermanshah-Iran. Adv Med Educ Pract. 2019;10:637–43.
Article Google Scholar
Abid N, Aslam S, Alghamdi AA, Kumar T. Relationships among students’ reading habits, study skills, and academic achievement in English at the secondary level. Front Psychol. 2023;14:1020269.
Abdulghani HM, Al-Drees AA, Khalil MS, Ahmad F, Ponnamperuma GG, Amin Z. What factors determine academic achievement in high achieving undergraduate medical students? A qualitative study. Med Teach. 2014;36(Suppl 1):S43–48.
Muntean LM, Nireștean A, Sima-Comaniciu A, Mărușteri M, Zăgan CA, Lukacs E. The relationship between personality, motivation and academic performance at Medical students from Romania. Int J Environ Res Public Health 2022, 19(15).
Reza HM, Alireza HJIJME. Investigating study Habits of Library and Information Sciences Students of Isfahan University and Isfahan University of Medical Sciences. 2014, 14:751–757.
Kurtz SM, Silverman JD. The Calgary-Cambridge Referenced Observation guides: an aid to defining the curriculum and organizing the teaching in communication training programmes. Med Educ. 1996;30(2):83–9.
Pun J, Kong B. An exploratory study of communication training for Chinese medicine practitioners in Hong Kong to integrate patients’ conventional medical history. BMC Complement Med Ther. 2023;23(1):10.
İlçin N, Tomruk M, Yeşilyaprak SS, Karadibak D, Savcı S. The relationship between learning styles and academic performance in TURKISH physiotherapy students. BMC Med Educ. 2018;18(1):291.
McKeirnan KC, Colorafi K, Kim AP, Stewart AS, Remsberg CM, Vu M, Bray BS. Study behaviors Associated with Student pharmacists’ academic success in an active Classroom Pharmacy Curriculum. Am J Pharm Educ. 2020;84(7):ajpe7695.
Jouhari Z, Haghani F, Changiz T. Assessment of medical students’ learning and study strategies in self-regulated learning. J Adv Med Educ Professionalism. 2016;4(2):72–9.
Google Scholar
Proctor BE, Prevatt FF, Adams KSS, Reaser A, Petscher Y. Study skills profiles of normal-achieving and academically-struggling College students. J Coll Student Dev. 2006;47(1):37–51.
Kyauta AMASY, Garba HS. The role of guidance and counseling service on academic performance among students of umar suleiman college of education, Gashua, Yobe State, Nigeria. KIU J Humanit. 2017;2(2):59–66.
Eva KW, Bordage G, Campbell C, Galbraith R, Ginsburg S, Holmboe E, Regehr G. Towards a program of assessment for health professionals: from training into practice. Adv Health Sci Education: Theory Pract. 2016;21(4):897–913.
Curcio G, Ferrara M, De Gennaro L. Sleep loss, learning capacity and academic performance. Sleep Med Rev. 2006;10(5):323–37.
Al Shawwa L, Abulaban AA, Abulaban AA, Merdad A, Baghlaf S, Algethami A, Abu-Shanab J, Balkhoyor A. Factors potentially influencing academic performance among medical students. Adv Med Educ Pract. 2015;6:65–75.
Ibrahim NK, Baharoon BS, Banjar WF, Jar AA, Ashor RM, Aman AA, Al-Ahmadi JR. Mobile Phone Addiction and its relationship to Sleep Quality and Academic Achievement of Medical students at King Abdulaziz University, Jeddah, Saudi Arabia. J Res Health Sci. 2018;18(3):e00420.
Alkhalaf AM, Tekian A, Park YS. The impact of WhatsApp use on academic achievement among Saudi medical students. Med Teach. 2018;40(sup1):S10–4.
Bonsaksen T, Brown T, Lim HB, Fong K. Approaches to studying predict academic performance in undergraduate occupational therapy students: a cross-cultural study. BMC Med Educ. 2017;17(1):76.
Alzahrani HA, Alzahrani OH. Learning strategies of medical students in the surgery department, Jeddah, Saudi Arabia. Adv Med Educ Pract. 2012;3:79–87.
Bin Abdulrahman KA, Khalaf AM, Bin Abbas FB, Alanazi OT. Study habits of highly effective medical students. Adv Med Educ Pract. 2021;12:627–33.
Jameel T, Gazzaz ZJ, Baig M, Tashkandi JM, Alharenth NS, Butt NS, Shafique A, Iftikhar R. Medical students’ preferences towards learning resources and their study habits at King Abdulaziz University, Jeddah, Saudi Arabia. BMC Res Notes. 2019;12(1):30.
Abdulghani HM, Alrowais NA, Bin-Saad NS, Al-Subaie NM, Haji AM, Alhaqwi AI. Sleep disorder among medical students: relationship to their academic performance. Med Teach. 2012;34(Suppl 1):S37–41.
Hwang G-J, Wang S-Y, Lai C-L. Effects of a social regulation-based online learning framework on students’ learning achievements and behaviors in mathematics. Comput Educ. 2021;160:104031.
Gamage KAA, Dehideniya D, Ekanayake SY. The role of personal values in learning approaches and student achievements. Behav Sci (Basel Switzerland) 2021, 11(7).
Linn Z, Tashiro Y, Morio K, Hori H. Peer evaluations of group work in different years of medical school and academic achievement: how are they related? BMC Med Educ. 2022;22(1):102.
Avonts M, Michels NR, Bombeke K, Hens N, Coenen S, Vanderveken OM, De Winter BY. Does peer teaching improve academic results and competencies during medical school? A mixed methods study. BMC Med Educ. 2022;22(1):431.
Topor DR, Keane SP, Shelton TL, Calkins SD. Parent involvement and student academic performance: a multiple mediational analysis. J Prev Interv Community. 2010;38(3):183–97.
Veas A, Castejón JL, Miñano P, Gilar-Corbí R. Relationship between parent involvement and academic achievement through metacognitive strategies: a multiple multilevel mediation analysis. Br J Educ Psychol. 2019;89(2):393–411.
Núñez JC, Regueiro B, Suárez N, Piñeiro I, Rodicio ML, Valle A. Student Perception of teacher and parent involvement in Homework and Student Engagement: the mediating role of motivation. Front Psychol. 2019;10:1384.
Abdulghani AH, Ahmad T, Abdulghani HM. The impact of COVID-19 pandemic on anxiety and depression among physical therapists in Saudi Arabia: a cross-sectional study. BMC Med Educ. 2022;22(1):751.
Park KH, Park JH, Kim S, Rhee JA, Kim JH, Ahn YJ, Han JJ, Suh DJ. Students’ perception of the educational environment of medical schools in Korea: findings from a nationwide survey. Korean J Med Educ. 2015;27(2):117–30.
Ahrberg K, Dresler M, Niedermaier S, Steiger A, Genzel L. The interaction between sleep quality and academic performance. J Psychiatr Res. 2012;46(12):1618–22.
Dikker S, Haegens S, Bevilacqua D, Davidesco I, Wan L, Kaggen L, McClintock J, Chaloner K, Ding M, West T, et al. Morning brain: real-world neural evidence that high school class times matter. Soc Cognit Affect Neurosci. 2020;15(11):1193–202.
Pittenger AL, Dimitropoulos E, Foag J, Bishop D, Panizza S, Bishop JR. Closing the Classroom Theory to practice gap by simulating a Psychiatric Pharmacy Practice Experience. Am J Pharm Educ. 2019;83(10):7276.
Walck-Shannon EM, Rowell SF, Frey RF. To what extent do Study habits relate to performance? CBE Life Sci Educ. 2021;20(1):ar6.
Abdulghani HM, Alanazi K, Alotaibi R, Alsubeeh NA, Ahmad T, Haque S. Prevalence of potential dropout thoughts and their influential factors among Saudi Medical Students. 2023, 13(1):21582440221146966.
Sleight DA, Mavis BE. Study skills and academic performance among second-Year Medical students in Problem-based learning. Med Educ Online. 2006;11(1):4599.
Download references
Acknowledgements
The authors are grateful to the Deanship of Scientific Research, King Saud University, for.
support through the Vice Deanship of Scientific Research Chairs.
Author information
Authors and affiliations.
Department of Psychiatry, College of Medicine, King Saud University, Riyadh, Saudi Arabia
Mohammed A. Aljaffer & Ahmad H. Almadani
College of Medicine, King Saud University, Riyadh, Saudi Arabia
Abdullah S. AlDughaither, Ali A. Basfar, Saad M. AlGhadir, Yahya A. AlGhamdi, Bassam N. AlHubaysh & Osamah A. AlMayouf
Department of Psychiatry, College of Medicine, Imam Mohammad Ibn Saud Islamic University, Riyadh, Saudi Arabia
Saleh A. AlGhamdi
Department of Medical Education, College of Medicine, King Saud University, P.O. Box: 230155, Riyadh, 11321, Saudi Arabia
Tauseef Ahmad
Department of Medical Education and Family Medicine, College of Medicine, King Saud University, Riyadh, Saudi Arabia
Hamza M. Abdulghani
You can also search for this author in PubMed Google Scholar
Contributions
Conception or design: AHA, MAA, and HMA. Acquisition, analysis, or interpretation of data: AAB, SMA, ASA, YAA, BNA, OAA and SAA. Drafting the work or revising: TA, AHA, ASA AAB. Final approval of the manuscript: MAA, HMA., AHA, and TA. The author(s) read and approved the final manuscript.
Corresponding author
Correspondence to Tauseef Ahmad .
Ethics declarations
Conflict of interest.
The Authors declare that they have no conflict of interest.
Consent for publication
Not applicable.
Competing interests
The authors declare no competing interests.
Human ethics and consent to participate declarations
Additional information, publisher’s note.
Springer Nature remains neutral with regard to jurisdictional claims in published maps and institutional affiliations.
Rights and permissions
Open Access This article is licensed under a Creative Commons Attribution-NonCommercial-NoDerivatives 4.0 International License, which permits any non-commercial use, sharing, distribution and reproduction in any medium or format, as long as you give appropriate credit to the original author(s) and the source, provide a link to the Creative Commons licence, and indicate if you modified the licensed material. You do not have permission under this licence to share adapted material derived from this article or parts of it. The images or other third party material in this article are included in the article’s Creative Commons licence, unless indicated otherwise in a credit line to the material. If material is not included in the article’s Creative Commons licence and your intended use is not permitted by statutory regulation or exceeds the permitted use, you will need to obtain permission directly from the copyright holder. To view a copy of this licence, visit http://creativecommons.org/licenses/by-nc-nd/4.0/ .
Reprints and permissions
About this article
Cite this article.
Aljaffer, M.A., Almadani, A.H., AlDughaither, A.S. et al. The impact of study habits and personal factors on the academic achievement performances of medical students. BMC Med Educ 24 , 888 (2024). https://doi.org/10.1186/s12909-024-05889-y
Download citation
Received : 26 September 2023
Accepted : 12 August 2024
Published : 19 August 2024
DOI : https://doi.org/10.1186/s12909-024-05889-y
Share this article
Anyone you share the following link with will be able to read this content:
Sorry, a shareable link is not currently available for this article.
Provided by the Springer Nature SharedIt content-sharing initiative
- Medical students
- Study habits
- Academic achievement
- Saudi Arabia
BMC Medical Education
ISSN: 1472-6920
- General enquiries: [email protected]
- Link to facebook
- Link to linkedin
- Link to twitter
- Link to youtube
- Writing Tips
The Four Types of Research Paradigms: A Comprehensive Guide
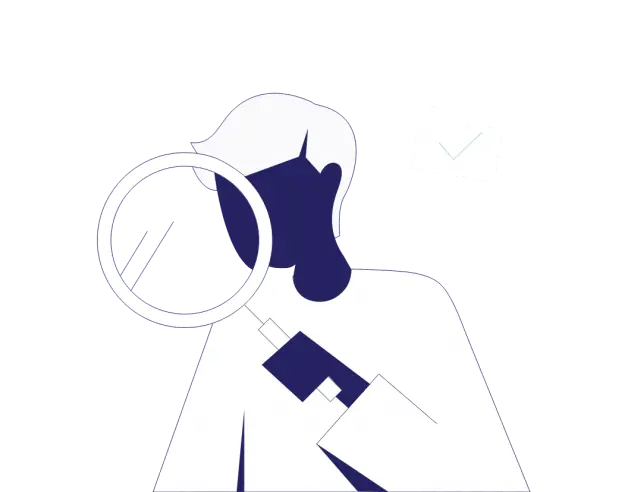
5-minute read
- 22nd January 2023
In this guide, you’ll learn all about the four research paradigms and how to choose the right one for your research.
Introduction to Research Paradigms
A paradigm is a system of beliefs, ideas, values, or habits that form the basis for a way of thinking about the world. Therefore, a research paradigm is an approach, model, or framework from which to conduct research. The research paradigm helps you to form a research philosophy, which in turn informs your research methodology.
Your research methodology is essentially the “how” of your research – how you design your study to not only accomplish your research’s aims and objectives but also to ensure your results are reliable and valid. Choosing the correct research paradigm is crucial because it provides a logical structure for conducting your research and improves the quality of your work, assuming it’s followed correctly.
Three Pillars: Ontology, Epistemology, and Methodology
Before we jump into the four types of research paradigms, we need to consider the three pillars of a research paradigm.
Ontology addresses the question, “What is reality?” It’s the study of being. This pillar is about finding out what you seek to research. What do you aim to examine?
Epistemology is the study of knowledge. It asks, “How is knowledge gathered and from what sources?”
Methodology involves the system in which you choose to investigate, measure, and analyze your research’s aims and objectives. It answers the “how” questions.
Let’s now take a look at the different research paradigms.
1. Positivist Research Paradigm
The positivist research paradigm assumes that there is one objective reality, and people can know this reality and accurately describe and explain it. Positivists rely on their observations through their senses to gain knowledge of their surroundings.
In this singular objective reality, researchers can compare their claims and ascertain the truth. This means researchers are limited to data collection and interpretations from an objective viewpoint. As a result, positivists usually use quantitative methodologies in their research (e.g., statistics, social surveys, and structured questionnaires).
This research paradigm is mostly used in natural sciences, physical sciences, or whenever large sample sizes are being used.
2. Interpretivist Research Paradigm
Interpretivists believe that different people in society experience and understand reality in different ways – while there may be only “one” reality, everyone interprets it according to their own view. They also believe that all research is influenced and shaped by researchers’ worldviews and theories.
As a result, interpretivists use qualitative methods and techniques to conduct their research. This includes interviews, focus groups, observations of a phenomenon, or collecting documentation on a phenomenon (e.g., newspaper articles, reports, or information from websites).
3. Critical Theory Research Paradigm
The critical theory paradigm asserts that social science can never be 100% objective or value-free. This paradigm is focused on enacting social change through scientific investigation. Critical theorists question knowledge and procedures and acknowledge how power is used (or abused) in the phenomena or systems they’re investigating.
Find this useful?
Subscribe to our newsletter and get writing tips from our editors straight to your inbox.
Researchers using this paradigm are more often than not aiming to create a more just, egalitarian society in which individual and collective freedoms are secure. Both quantitative and qualitative methods can be used with this paradigm.
4. Constructivist Research Paradigm
Constructivism asserts that reality is a construct of our minds ; therefore, reality is subjective. Constructivists believe that all knowledge comes from our experiences and reflections on those experiences and oppose the idea that there is a single methodology to generate knowledge.
This paradigm is mostly associated with qualitative research approaches due to its focus on experiences and subjectivity. The researcher focuses on participants’ experiences as well as their own.
Choosing the Right Research Paradigm for Your Study
Once you have a comprehensive understanding of each paradigm, you’re faced with a big question: which paradigm should you choose? The answer to this will set the course of your research and determine its success, findings, and results.
To start, you need to identify your research problem, research objectives , and hypothesis . This will help you to establish what you want to accomplish or understand from your research and the path you need to take to achieve this.
You can begin this process by asking yourself some questions:
- What is the nature of your research problem (i.e., quantitative or qualitative)?
- How can you acquire the knowledge you need and communicate it to others? For example, is this knowledge already available in other forms (e.g., documents) and do you need to gain it by gathering or observing other people’s experiences or by experiencing it personally?
- What is the nature of the reality that you want to study? Is it objective or subjective?
Depending on the problem and objective, other questions may arise during this process that lead you to a suitable paradigm. Ultimately, you must be able to state, explain, and justify the research paradigm you select for your research and be prepared to include this in your dissertation’s methodology and design section.
Using Two Paradigms
If the nature of your research problem and objectives involves both quantitative and qualitative aspects, then you might consider using two paradigms or a mixed methods approach . In this, one paradigm is used to frame the qualitative aspects of the study and another for the quantitative aspects. This is acceptable, although you will be tasked with explaining your rationale for using both of these paradigms in your research.
Choosing the right research paradigm for your research can seem like an insurmountable task. It requires you to:
● Have a comprehensive understanding of the paradigms,
● Identify your research problem, objectives, and hypothesis, and
● Be able to state, explain, and justify the paradigm you select in your methodology and design section.
Although conducting your research and putting your dissertation together is no easy task, proofreading it can be! Our experts are here to make your writing shine. Your first 500 words are free !
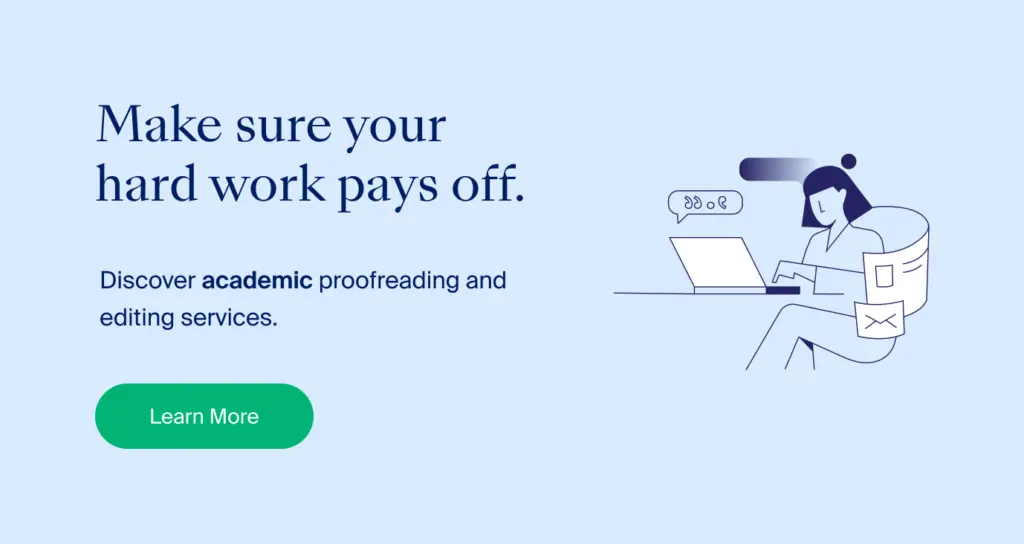
Share this article:
Post A New Comment
Got content that needs a quick turnaround? Let us polish your work. Explore our editorial business services.
Free email newsletter template (2024).
Promoting a brand means sharing valuable insights to connect more deeply with your audience, and...
6-minute read
How to Write a Nonprofit Grant Proposal
If you’re seeking funding to support your charitable endeavors as a nonprofit organization, you’ll need...
9-minute read
How to Use Infographics to Boost Your Presentation
Is your content getting noticed? Capturing and maintaining an audience’s attention is a challenge when...
8-minute read
Why Interactive PDFs Are Better for Engagement
Are you looking to enhance engagement and captivate your audience through your professional documents? Interactive...
7-minute read
Seven Key Strategies for Voice Search Optimization
Voice search optimization is rapidly shaping the digital landscape, requiring content professionals to adapt their...
4-minute read
Five Creative Ways to Showcase Your Digital Portfolio
Are you a creative freelancer looking to make a lasting impression on potential clients or...
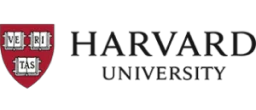
Make sure your writing is the best it can be with our expert English proofreading and editing.

An official website of the United States government
The .gov means it’s official. Federal government websites often end in .gov or .mil. Before sharing sensitive information, make sure you’re on a federal government site.
The site is secure. The https:// ensures that you are connecting to the official website and that any information you provide is encrypted and transmitted securely.
- Publications
- Account settings
- My Bibliography
- Collections
- Citation manager
Save citation to file
Email citation, add to collections.
- Create a new collection
- Add to an existing collection
Add to My Bibliography
Your saved search, create a file for external citation management software, your rss feed.
- Search in PubMed
- Search in NLM Catalog
- Add to Search
Experiences of habit formation: a qualitative study
Affiliation.
- 1 Health Behaviour Research Centre, Department of Epidemiology and Public Health, University College London, London, UK.
- PMID: 21749245
- DOI: 10.1080/13548506.2011.555774
Habit formation is an important goal for behaviour change interventions because habitual behaviours are elicited automatically and are therefore likely to be maintained. This study documented experiences of habit development in 10 participants enrolled on a weight loss intervention explicitly based on habit-formation principles. Thematic analysis revealed three themes: Strategies used to support initial engagement in a novel behaviour; development of behavioural automaticity; and selecting effective cues to support repeated behaviour. Results showed that behaviour change was initially experienced as cognitively effortful but as automaticity increased, enactment became easier. Habits were typically formed in work-based contexts. Weekends and vacations temporarily disrupted performance due to absence of associated cues, but habits were reinstated on return to work. Implications for theory and practice are discussed.
PubMed Disclaimer
Similar articles
- Habit formation following routine-based versus time-based cue planning: A randomized controlled trial. Keller J, Kwasnicka D, Klaiber P, Sichert L, Lally P, Fleig L. Keller J, et al. Br J Health Psychol. 2021 Sep;26(3):807-824. doi: 10.1111/bjhp.12504. Epub 2021 Jan 6. Br J Health Psychol. 2021. PMID: 33405284 Clinical Trial.
- Breaking habits or breaking habitual behaviours? Old habits as a neglected factor in weight loss maintenance. Gardner B, Richards R, Lally P, Rebar A, Thwaite T, Beeken RJ. Gardner B, et al. Appetite. 2021 Jul 1;162:105183. doi: 10.1016/j.appet.2021.105183. Epub 2021 Feb 27. Appetite. 2021. PMID: 33651994 Review.
- Participant experiences of two successful habit-based weight-loss interventions in Australia: a qualitative study. Cleo G, Hersch J, Thomas R. Cleo G, et al. BMJ Open. 2018 May 31;8(5):e020146. doi: 10.1136/bmjopen-2017-020146. BMJ Open. 2018. PMID: 29858412 Free PMC article. Clinical Trial.
- Opportunities and challenges of behavior change support systems for enhancing habit formation: A qualitative study. Karppinen P, Oinas-Kukkonen H, Alahäivälä T, Jokelainen T, Teeriniemi AM, Salonurmi T, Savolainen MJ. Karppinen P, et al. J Biomed Inform. 2018 Aug;84:82-92. doi: 10.1016/j.jbi.2018.06.012. Epub 2018 Jun 21. J Biomed Inform. 2018. PMID: 29936091 Clinical Trial.
- Could habits hold the key to weight loss maintenance? A narrative review. Cleo G, Isenring E, Thomas R, Glasziou P. Cleo G, et al. J Hum Nutr Diet. 2017 Oct;30(5):655-664. doi: 10.1111/jhn.12456. Epub 2017 Feb 2. J Hum Nutr Diet. 2017. PMID: 28150402 Review.
- Age Unplugged: A Brief Narrative Review on the Intersection of Digital Tools, Sedentary and Physical Activity Behaviors in Community-Dwelling Older Adults. Ramalho A, Paulo R, Duarte-Mendes P, Serrano J, Petrica J. Ramalho A, et al. Healthcare (Basel). 2024 May 1;12(9):935. doi: 10.3390/healthcare12090935. Healthcare (Basel). 2024. PMID: 38727492 Free PMC article. Review.
- Impact of an Oral Health Education Program on the Oral Health Literacy of Refugees. Muller R, Bilich L, Jones M. Muller R, et al. J Immigr Minor Health. 2024 Aug;26(4):699-710. doi: 10.1007/s10903-024-01594-6. Epub 2024 Apr 8. J Immigr Minor Health. 2024. PMID: 38589753 Free PMC article.
- Promoting evidence-based practice through peer teaching: Practical tips to run a departmental teaching program in a healthcare setting. Murinye Magwenzi M. Murinye Magwenzi M. MedEdPublish (2016). 2020 Oct 8;9:223. doi: 10.15694/mep.2020.000223.1. eCollection 2020. MedEdPublish (2016). 2020. PMID: 38073792 Free PMC article.
- The feasibility and acceptability of an online mind-body wellness program for patients with primary biliary cholangitis. Watt M, Hyde A, Spence JC, Wright GM, Vander Well S, Johnson E, Mason A, McLeod M, Tandon P. Watt M, et al. Can Liver J. 2023 Oct 30;6(3):314-331. doi: 10.3138/canlivj-2022-0045. eCollection 2023 Oct. Can Liver J. 2023. PMID: 38020194 Free PMC article.
- Codevelopment of a Text Messaging Intervention to Support Adherence to Adjuvant Endocrine Therapy in Women With Breast Cancer: Mixed Methods Approach. Green SMC, French DP, Hall LH, Bartlett YK, Rousseau N, Raine E, Parbutt C, Gardner B; ROSETA Investigators; Smith SG. Green SMC, et al. J Med Internet Res. 2023 May 24;25:e38073. doi: 10.2196/38073. J Med Internet Res. 2023. PMID: 37223964 Free PMC article.
- Search in MeSH
Related information
- Cited in Books
Grants and funding
- 14133/CRUK_/Cancer Research UK/United Kingdom
- G0802027/MRC_/Medical Research Council/United Kingdom
LinkOut - more resources
Full text sources.
- Taylor & Francis
- Citation Manager
NCBI Literature Resources
MeSH PMC Bookshelf Disclaimer
The PubMed wordmark and PubMed logo are registered trademarks of the U.S. Department of Health and Human Services (HHS). Unauthorized use of these marks is strictly prohibited.

An official website of the United States government
The .gov means it’s official. Federal government websites often end in .gov or .mil. Before sharing sensitive information, make sure you’re on a federal government site.
The site is secure. The https:// ensures that you are connecting to the official website and that any information you provide is encrypted and transmitted securely.
- Publications
- Account settings
Preview improvements coming to the PMC website in October 2024. Learn More or Try it out now .
- Advanced Search
- Journal List

What is Qualitative in Qualitative Research
Patrik aspers.
1 Department of Sociology, Uppsala University, Uppsala, Sweden
2 Seminar for Sociology, Universität St. Gallen, St. Gallen, Switzerland
3 Department of Media and Social Sciences, University of Stavanger, Stavanger, Norway
What is qualitative research? If we look for a precise definition of qualitative research, and specifically for one that addresses its distinctive feature of being “qualitative,” the literature is meager. In this article we systematically search, identify and analyze a sample of 89 sources using or attempting to define the term “qualitative.” Then, drawing on ideas we find scattered across existing work, and based on Becker’s classic study of marijuana consumption, we formulate and illustrate a definition that tries to capture its core elements. We define qualitative research as an iterative process in which improved understanding to the scientific community is achieved by making new significant distinctions resulting from getting closer to the phenomenon studied. This formulation is developed as a tool to help improve research designs while stressing that a qualitative dimension is present in quantitative work as well. Additionally, it can facilitate teaching, communication between researchers, diminish the gap between qualitative and quantitative researchers, help to address critiques of qualitative methods, and be used as a standard of evaluation of qualitative research.
If we assume that there is something called qualitative research, what exactly is this qualitative feature? And how could we evaluate qualitative research as good or not? Is it fundamentally different from quantitative research? In practice, most active qualitative researchers working with empirical material intuitively know what is involved in doing qualitative research, yet perhaps surprisingly, a clear definition addressing its key feature is still missing.
To address the question of what is qualitative we turn to the accounts of “qualitative research” in textbooks and also in empirical work. In his classic, explorative, interview study of deviance Howard Becker ( 1963 ) asks ‘How does one become a marijuana user?’ In contrast to pre-dispositional and psychological-individualistic theories of deviant behavior, Becker’s inherently social explanation contends that becoming a user of this substance is the result of a three-phase sequential learning process. First, potential users need to learn how to smoke it properly to produce the “correct” effects. If not, they are likely to stop experimenting with it. Second, they need to discover the effects associated with it; in other words, to get “high,” individuals not only have to experience what the drug does, but also to become aware that those sensations are related to using it. Third, they require learning to savor the feelings related to its consumption – to develop an acquired taste. Becker, who played music himself, gets close to the phenomenon by observing, taking part, and by talking to people consuming the drug: “half of the fifty interviews were conducted with musicians, the other half covered a wide range of people, including laborers, machinists, and people in the professions” (Becker 1963 :56).
Another central aspect derived through the common-to-all-research interplay between induction and deduction (Becker 2017 ), is that during the course of his research Becker adds scientifically meaningful new distinctions in the form of three phases—distinctions, or findings if you will, that strongly affect the course of his research: its focus, the material that he collects, and which eventually impact his findings. Each phase typically unfolds through social interaction, and often with input from experienced users in “a sequence of social experiences during which the person acquires a conception of the meaning of the behavior, and perceptions and judgments of objects and situations, all of which make the activity possible and desirable” (Becker 1963 :235). In this study the increased understanding of smoking dope is a result of a combination of the meaning of the actors, and the conceptual distinctions that Becker introduces based on the views expressed by his respondents. Understanding is the result of research and is due to an iterative process in which data, concepts and evidence are connected with one another (Becker 2017 ).
Indeed, there are many definitions of qualitative research, but if we look for a definition that addresses its distinctive feature of being “qualitative,” the literature across the broad field of social science is meager. The main reason behind this article lies in the paradox, which, to put it bluntly, is that researchers act as if they know what it is, but they cannot formulate a coherent definition. Sociologists and others will of course continue to conduct good studies that show the relevance and value of qualitative research addressing scientific and practical problems in society. However, our paper is grounded in the idea that providing a clear definition will help us improve the work that we do. Among researchers who practice qualitative research there is clearly much knowledge. We suggest that a definition makes this knowledge more explicit. If the first rationale for writing this paper refers to the “internal” aim of improving qualitative research, the second refers to the increased “external” pressure that especially many qualitative researchers feel; pressure that comes both from society as well as from other scientific approaches. There is a strong core in qualitative research, and leading researchers tend to agree on what it is and how it is done. Our critique is not directed at the practice of qualitative research, but we do claim that the type of systematic work we do has not yet been done, and that it is useful to improve the field and its status in relation to quantitative research.
The literature on the “internal” aim of improving, or at least clarifying qualitative research is large, and we do not claim to be the first to notice the vagueness of the term “qualitative” (Strauss and Corbin 1998 ). Also, others have noted that there is no single definition of it (Long and Godfrey 2004 :182), that there are many different views on qualitative research (Denzin and Lincoln 2003 :11; Jovanović 2011 :3), and that more generally, we need to define its meaning (Best 2004 :54). Strauss and Corbin ( 1998 ), for example, as well as Nelson et al. (1992:2 cited in Denzin and Lincoln 2003 :11), and Flick ( 2007 :ix–x), have recognized that the term is problematic: “Actually, the term ‘qualitative research’ is confusing because it can mean different things to different people” (Strauss and Corbin 1998 :10–11). Hammersley has discussed the possibility of addressing the problem, but states that “the task of providing an account of the distinctive features of qualitative research is far from straightforward” ( 2013 :2). This confusion, as he has recently further argued (Hammersley 2018 ), is also salient in relation to ethnography where different philosophical and methodological approaches lead to a lack of agreement about what it means.
Others (e.g. Hammersley 2018 ; Fine and Hancock 2017 ) have also identified the treat to qualitative research that comes from external forces, seen from the point of view of “qualitative research.” This threat can be further divided into that which comes from inside academia, such as the critique voiced by “quantitative research” and outside of academia, including, for example, New Public Management. Hammersley ( 2018 ), zooming in on one type of qualitative research, ethnography, has argued that it is under treat. Similarly to Fine ( 2003 ), and before him Gans ( 1999 ), he writes that ethnography’ has acquired a range of meanings, and comes in many different versions, these often reflecting sharply divergent epistemological orientations. And already more than twenty years ago while reviewing Denzin and Lincoln’ s Handbook of Qualitative Methods Fine argued:
While this increasing centrality [of qualitative research] might lead one to believe that consensual standards have developed, this belief would be misleading. As the methodology becomes more widely accepted, querulous challengers have raised fundamental questions that collectively have undercut the traditional models of how qualitative research is to be fashioned and presented (1995:417).
According to Hammersley, there are today “serious treats to the practice of ethnographic work, on almost any definition” ( 2018 :1). He lists five external treats: (1) that social research must be accountable and able to show its impact on society; (2) the current emphasis on “big data” and the emphasis on quantitative data and evidence; (3) the labor market pressure in academia that leaves less time for fieldwork (see also Fine and Hancock 2017 ); (4) problems of access to fields; and (5) the increased ethical scrutiny of projects, to which ethnography is particularly exposed. Hammersley discusses some more or less insufficient existing definitions of ethnography.
The current situation, as Hammersley and others note—and in relation not only to ethnography but also qualitative research in general, and as our empirical study shows—is not just unsatisfactory, it may even be harmful for the entire field of qualitative research, and does not help social science at large. We suggest that the lack of clarity of qualitative research is a real problem that must be addressed.
Towards a Definition of Qualitative Research
Seen in an historical light, what is today called qualitative, or sometimes ethnographic, interpretative research – or a number of other terms – has more or less always existed. At the time the founders of sociology – Simmel, Weber, Durkheim and, before them, Marx – were writing, and during the era of the Methodenstreit (“dispute about methods”) in which the German historical school emphasized scientific methods (cf. Swedberg 1990 ), we can at least speak of qualitative forerunners.
Perhaps the most extended discussion of what later became known as qualitative methods in a classic work is Bronisław Malinowski’s ( 1922 ) Argonauts in the Western Pacific , although even this study does not explicitly address the meaning of “qualitative.” In Weber’s ([1921–-22] 1978) work we find a tension between scientific explanations that are based on observation and quantification and interpretative research (see also Lazarsfeld and Barton 1982 ).
If we look through major sociology journals like the American Sociological Review , American Journal of Sociology , or Social Forces we will not find the term qualitative sociology before the 1970s. And certainly before then much of what we consider qualitative classics in sociology, like Becker’ study ( 1963 ), had already been produced. Indeed, the Chicago School often combined qualitative and quantitative data within the same study (Fine 1995 ). Our point being that before a disciplinary self-awareness the term quantitative preceded qualitative, and the articulation of the former was a political move to claim scientific status (Denzin and Lincoln 2005 ). In the US the World War II seem to have sparked a critique of sociological work, including “qualitative work,” that did not follow the scientific canon (Rawls 2018 ), which was underpinned by a scientifically oriented and value free philosophy of science. As a result the attempts and practice of integrating qualitative and quantitative sociology at Chicago lost ground to sociology that was more oriented to surveys and quantitative work at Columbia under Merton-Lazarsfeld. The quantitative tradition was also able to present textbooks (Lundberg 1951 ) that facilitated the use this approach and its “methods.” The practices of the qualitative tradition, by and large, remained tacit or was part of the mentoring transferred from the renowned masters to their students.
This glimpse into history leads us back to the lack of a coherent account condensed in a definition of qualitative research. Many of the attempts to define the term do not meet the requirements of a proper definition: A definition should be clear, avoid tautology, demarcate its domain in relation to the environment, and ideally only use words in its definiens that themselves are not in need of definition (Hempel 1966 ). A definition can enhance precision and thus clarity by identifying the core of the phenomenon. Preferably, a definition should be short. The typical definition we have found, however, is an ostensive definition, which indicates what qualitative research is about without informing us about what it actually is :
Qualitative research is multimethod in focus, involving an interpretative, naturalistic approach to its subject matter. This means that qualitative researchers study things in their natural settings, attempting to make sense of, or interpret, phenomena in terms of the meanings people bring to them. Qualitative research involves the studied use and collection of a variety of empirical materials – case study, personal experience, introspective, life story, interview, observational, historical, interactional, and visual texts – that describe routine and problematic moments and meanings in individuals’ lives. (Denzin and Lincoln 2005 :2)
Flick claims that the label “qualitative research” is indeed used as an umbrella for a number of approaches ( 2007 :2–4; 2002 :6), and it is not difficult to identify research fitting this designation. Moreover, whatever it is, it has grown dramatically over the past five decades. In addition, courses have been developed, methods have flourished, arguments about its future have been advanced (for example, Denzin and Lincoln 1994) and criticized (for example, Snow and Morrill 1995 ), and dedicated journals and books have mushroomed. Most social scientists have a clear idea of research and how it differs from journalism, politics and other activities. But the question of what is qualitative in qualitative research is either eluded or eschewed.
We maintain that this lacuna hinders systematic knowledge production based on qualitative research. Paul Lazarsfeld noted the lack of “codification” as early as 1955 when he reviewed 100 qualitative studies in order to offer a codification of the practices (Lazarsfeld and Barton 1982 :239). Since then many texts on “qualitative research” and its methods have been published, including recent attempts (Goertz and Mahoney 2012 ) similar to Lazarsfeld’s. These studies have tried to extract what is qualitative by looking at the large number of empirical “qualitative” studies. Our novel strategy complements these endeavors by taking another approach and looking at the attempts to codify these practices in the form of a definition, as well as to a minor extent take Becker’s study as an exemplar of what qualitative researchers actually do, and what the characteristic of being ‘qualitative’ denotes and implies. We claim that qualitative researchers, if there is such a thing as “qualitative research,” should be able to codify their practices in a condensed, yet general way expressed in language.
Lingering problems of “generalizability” and “how many cases do I need” (Small 2009 ) are blocking advancement – in this line of work qualitative approaches are said to differ considerably from quantitative ones, while some of the former unsuccessfully mimic principles related to the latter (Small 2009 ). Additionally, quantitative researchers sometimes unfairly criticize the first based on their own quality criteria. Scholars like Goertz and Mahoney ( 2012 ) have successfully focused on the different norms and practices beyond what they argue are essentially two different cultures: those working with either qualitative or quantitative methods. Instead, similarly to Becker ( 2017 ) who has recently questioned the usefulness of the distinction between qualitative and quantitative research, we focus on similarities.
The current situation also impedes both students and researchers in focusing their studies and understanding each other’s work (Lazarsfeld and Barton 1982 :239). A third consequence is providing an opening for critiques by scholars operating within different traditions (Valsiner 2000 :101). A fourth issue is that the “implicit use of methods in qualitative research makes the field far less standardized than the quantitative paradigm” (Goertz and Mahoney 2012 :9). Relatedly, the National Science Foundation in the US organized two workshops in 2004 and 2005 to address the scientific foundations of qualitative research involving strategies to improve it and to develop standards of evaluation in qualitative research. However, a specific focus on its distinguishing feature of being “qualitative” while being implicitly acknowledged, was discussed only briefly (for example, Best 2004 ).
In 2014 a theme issue was published in this journal on “Methods, Materials, and Meanings: Designing Cultural Analysis,” discussing central issues in (cultural) qualitative research (Berezin 2014 ; Biernacki 2014 ; Glaeser 2014 ; Lamont and Swidler 2014 ; Spillman 2014). We agree with many of the arguments put forward, such as the risk of methodological tribalism, and that we should not waste energy on debating methods separated from research questions. Nonetheless, a clarification of the relation to what is called “quantitative research” is of outmost importance to avoid misunderstandings and misguided debates between “qualitative” and “quantitative” researchers. Our strategy means that researchers, “qualitative” or “quantitative” they may be, in their actual practice may combine qualitative work and quantitative work.
In this article we accomplish three tasks. First, we systematically survey the literature for meanings of qualitative research by looking at how researchers have defined it. Drawing upon existing knowledge we find that the different meanings and ideas of qualitative research are not yet coherently integrated into one satisfactory definition. Next, we advance our contribution by offering a definition of qualitative research and illustrate its meaning and use partially by expanding on the brief example introduced earlier related to Becker’s work ( 1963 ). We offer a systematic analysis of central themes of what researchers consider to be the core of “qualitative,” regardless of style of work. These themes – which we summarize in terms of four keywords: distinction, process, closeness, improved understanding – constitute part of our literature review, in which each one appears, sometimes with others, but never all in the same definition. They serve as the foundation of our contribution. Our categories are overlapping. Their use is primarily to organize the large amount of definitions we have identified and analyzed, and not necessarily to draw a clear distinction between them. Finally, we continue the elaboration discussed above on the advantages of a clear definition of qualitative research.
In a hermeneutic fashion we propose that there is something meaningful that deserves to be labelled “qualitative research” (Gadamer 1990 ). To approach the question “What is qualitative in qualitative research?” we have surveyed the literature. In conducting our survey we first traced the word’s etymology in dictionaries, encyclopedias, handbooks of the social sciences and of methods and textbooks, mainly in English, which is common to methodology courses. It should be noted that we have zoomed in on sociology and its literature. This discipline has been the site of the largest debate and development of methods that can be called “qualitative,” which suggests that this field should be examined in great detail.
In an ideal situation we should expect that one good definition, or at least some common ideas, would have emerged over the years. This common core of qualitative research should be so accepted that it would appear in at least some textbooks. Since this is not what we found, we decided to pursue an inductive approach to capture maximal variation in the field of qualitative research; we searched in a selection of handbooks, textbooks, book chapters, and books, to which we added the analysis of journal articles. Our sample comprises a total of 89 references.
In practice we focused on the discipline that has had a clear discussion of methods, namely sociology. We also conducted a broad search in the JSTOR database to identify scholarly sociology articles published between 1998 and 2017 in English with a focus on defining or explaining qualitative research. We specifically zoom in on this time frame because we would have expect that this more mature period would have produced clear discussions on the meaning of qualitative research. To find these articles we combined a number of keywords to search the content and/or the title: qualitative (which was always included), definition, empirical, research, methodology, studies, fieldwork, interview and observation .
As a second phase of our research we searched within nine major sociological journals ( American Journal of Sociology , Sociological Theory , American Sociological Review , Contemporary Sociology , Sociological Forum , Sociological Theory , Qualitative Research , Qualitative Sociology and Qualitative Sociology Review ) for articles also published during the past 19 years (1998–2017) that had the term “qualitative” in the title and attempted to define qualitative research.
Lastly we picked two additional journals, Qualitative Research and Qualitative Sociology , in which we could expect to find texts addressing the notion of “qualitative.” From Qualitative Research we chose Volume 14, Issue 6, December 2014, and from Qualitative Sociology we chose Volume 36, Issue 2, June 2017. Within each of these we selected the first article; then we picked the second article of three prior issues. Again we went back another three issues and investigated article number three. Finally we went back another three issues and perused article number four. This selection criteria was used to get a manageable sample for the analysis.
The coding process of the 89 references we gathered in our selected review began soon after the first round of material was gathered, and we reduced the complexity created by our maximum variation sampling (Snow and Anderson 1993 :22) to four different categories within which questions on the nature and properties of qualitative research were discussed. We call them: Qualitative and Quantitative Research, Qualitative Research, Fieldwork, and Grounded Theory. This – which may appear as an illogical grouping – merely reflects the “context” in which the matter of “qualitative” is discussed. If the selection process of the material – books and articles – was informed by pre-knowledge, we used an inductive strategy to code the material. When studying our material, we identified four central notions related to “qualitative” that appear in various combinations in the literature which indicate what is the core of qualitative research. We have labeled them: “distinctions”, “process,” “closeness,” and “improved understanding.” During the research process the categories and notions were improved, refined, changed, and reordered. The coding ended when a sense of saturation in the material arose. In the presentation below all quotations and references come from our empirical material of texts on qualitative research.
Analysis – What is Qualitative Research?
In this section we describe the four categories we identified in the coding, how they differently discuss qualitative research, as well as their overall content. Some salient quotations are selected to represent the type of text sorted under each of the four categories. What we present are examples from the literature.
Qualitative and Quantitative
This analytic category comprises quotations comparing qualitative and quantitative research, a distinction that is frequently used (Brown 2010 :231); in effect this is a conceptual pair that structures the discussion and that may be associated with opposing interests. While the general goal of quantitative and qualitative research is the same – to understand the world better – their methodologies and focus in certain respects differ substantially (Becker 1966 :55). Quantity refers to that property of something that can be determined by measurement. In a dictionary of Statistics and Methodology we find that “(a) When referring to *variables, ‘qualitative’ is another term for *categorical or *nominal. (b) When speaking of kinds of research, ‘qualitative’ refers to studies of subjects that are hard to quantify, such as art history. Qualitative research tends to be a residual category for almost any kind of non-quantitative research” (Stiles 1998:183). But it should be obvious that one could employ a quantitative approach when studying, for example, art history.
The same dictionary states that quantitative is “said of variables or research that can be handled numerically, usually (too sharply) contrasted with *qualitative variables and research” (Stiles 1998:184). From a qualitative perspective “quantitative research” is about numbers and counting, and from a quantitative perspective qualitative research is everything that is not about numbers. But this does not say much about what is “qualitative.” If we turn to encyclopedias we find that in the 1932 edition of the Encyclopedia of the Social Sciences there is no mention of “qualitative.” In the Encyclopedia from 1968 we can read:
Qualitative Analysis. For methods of obtaining, analyzing, and describing data, see [the various entries:] CONTENT ANALYSIS; COUNTED DATA; EVALUATION RESEARCH, FIELD WORK; GRAPHIC PRESENTATION; HISTORIOGRAPHY, especially the article on THE RHETORIC OF HISTORY; INTERVIEWING; OBSERVATION; PERSONALITY MEASUREMENT; PROJECTIVE METHODS; PSYCHOANALYSIS, article on EXPERIMENTAL METHODS; SURVEY ANALYSIS, TABULAR PRESENTATION; TYPOLOGIES. (Vol. 13:225)
Some, like Alford, divide researchers into methodologists or, in his words, “quantitative and qualitative specialists” (Alford 1998 :12). Qualitative research uses a variety of methods, such as intensive interviews or in-depth analysis of historical materials, and it is concerned with a comprehensive account of some event or unit (King et al. 1994 :4). Like quantitative research it can be utilized to study a variety of issues, but it tends to focus on meanings and motivations that underlie cultural symbols, personal experiences, phenomena and detailed understanding of processes in the social world. In short, qualitative research centers on understanding processes, experiences, and the meanings people assign to things (Kalof et al. 2008 :79).
Others simply say that qualitative methods are inherently unscientific (Jovanović 2011 :19). Hood, for instance, argues that words are intrinsically less precise than numbers, and that they are therefore more prone to subjective analysis, leading to biased results (Hood 2006 :219). Qualitative methodologies have raised concerns over the limitations of quantitative templates (Brady et al. 2004 :4). Scholars such as King et al. ( 1994 ), for instance, argue that non-statistical research can produce more reliable results if researchers pay attention to the rules of scientific inference commonly stated in quantitative research. Also, researchers such as Becker ( 1966 :59; 1970 :42–43) have asserted that, if conducted properly, qualitative research and in particular ethnographic field methods, can lead to more accurate results than quantitative studies, in particular, survey research and laboratory experiments.
Some researchers, such as Kalof, Dan, and Dietz ( 2008 :79) claim that the boundaries between the two approaches are becoming blurred, and Small ( 2009 ) argues that currently much qualitative research (especially in North America) tries unsuccessfully and unnecessarily to emulate quantitative standards. For others, qualitative research tends to be more humanistic and discursive (King et al. 1994 :4). Ragin ( 1994 ), and similarly also Becker, ( 1996 :53), Marchel and Owens ( 2007 :303) think that the main distinction between the two styles is overstated and does not rest on the simple dichotomy of “numbers versus words” (Ragin 1994 :xii). Some claim that quantitative data can be utilized to discover associations, but in order to unveil cause and effect a complex research design involving the use of qualitative approaches needs to be devised (Gilbert 2009 :35). Consequently, qualitative data are useful for understanding the nuances lying beyond those processes as they unfold (Gilbert 2009 :35). Others contend that qualitative research is particularly well suited both to identify causality and to uncover fine descriptive distinctions (Fine and Hallett 2014 ; Lichterman and Isaac Reed 2014 ; Katz 2015 ).
There are other ways to separate these two traditions, including normative statements about what qualitative research should be (that is, better or worse than quantitative approaches, concerned with scientific approaches to societal change or vice versa; Snow and Morrill 1995 ; Denzin and Lincoln 2005 ), or whether it should develop falsifiable statements; Best 2004 ).
We propose that quantitative research is largely concerned with pre-determined variables (Small 2008 ); the analysis concerns the relations between variables. These categories are primarily not questioned in the study, only their frequency or degree, or the correlations between them (cf. Franzosi 2016 ). If a researcher studies wage differences between women and men, he or she works with given categories: x number of men are compared with y number of women, with a certain wage attributed to each person. The idea is not to move beyond the given categories of wage, men and women; they are the starting point as well as the end point, and undergo no “qualitative change.” Qualitative research, in contrast, investigates relations between categories that are themselves subject to change in the research process. Returning to Becker’s study ( 1963 ), we see that he questioned pre-dispositional theories of deviant behavior working with pre-determined variables such as an individual’s combination of personal qualities or emotional problems. His take, in contrast, was to understand marijuana consumption by developing “variables” as part of the investigation. Thereby he presented new variables, or as we would say today, theoretical concepts, but which are grounded in the empirical material.
Qualitative Research
This category contains quotations that refer to descriptions of qualitative research without making comparisons with quantitative research. Researchers such as Denzin and Lincoln, who have written a series of influential handbooks on qualitative methods (1994; Denzin and Lincoln 2003 ; 2005 ), citing Nelson et al. (1992:4), argue that because qualitative research is “interdisciplinary, transdisciplinary, and sometimes counterdisciplinary” it is difficult to derive one single definition of it (Jovanović 2011 :3). According to them, in fact, “the field” is “many things at the same time,” involving contradictions, tensions over its focus, methods, and how to derive interpretations and findings ( 2003 : 11). Similarly, others, such as Flick ( 2007 :ix–x) contend that agreeing on an accepted definition has increasingly become problematic, and that qualitative research has possibly matured different identities. However, Best holds that “the proliferation of many sorts of activities under the label of qualitative sociology threatens to confuse our discussions” ( 2004 :54). Atkinson’s position is more definite: “the current state of qualitative research and research methods is confused” ( 2005 :3–4).
Qualitative research is about interpretation (Blumer 1969 ; Strauss and Corbin 1998 ; Denzin and Lincoln 2003 ), or Verstehen [understanding] (Frankfort-Nachmias and Nachmias 1996 ). It is “multi-method,” involving the collection and use of a variety of empirical materials (Denzin and Lincoln 1998; Silverman 2013 ) and approaches (Silverman 2005 ; Flick 2007 ). It focuses not only on the objective nature of behavior but also on its subjective meanings: individuals’ own accounts of their attitudes, motivations, behavior (McIntyre 2005 :127; Creswell 2009 ), events and situations (Bryman 1989) – what people say and do in specific places and institutions (Goodwin and Horowitz 2002 :35–36) in social and temporal contexts (Morrill and Fine 1997). For this reason, following Weber ([1921-22] 1978), it can be described as an interpretative science (McIntyre 2005 :127). But could quantitative research also be concerned with these questions? Also, as pointed out below, does all qualitative research focus on subjective meaning, as some scholars suggest?
Others also distinguish qualitative research by claiming that it collects data using a naturalistic approach (Denzin and Lincoln 2005 :2; Creswell 2009 ), focusing on the meaning actors ascribe to their actions. But again, does all qualitative research need to be collected in situ? And does qualitative research have to be inherently concerned with meaning? Flick ( 2007 ), referring to Denzin and Lincoln ( 2005 ), mentions conversation analysis as an example of qualitative research that is not concerned with the meanings people bring to a situation, but rather with the formal organization of talk. Still others, such as Ragin ( 1994 :85), note that qualitative research is often (especially early on in the project, we would add) less structured than other kinds of social research – a characteristic connected to its flexibility and that can lead both to potentially better, but also worse results. But is this not a feature of this type of research, rather than a defining description of its essence? Wouldn’t this comment also apply, albeit to varying degrees, to quantitative research?
In addition, Strauss ( 2003 ), along with others, such as Alvesson and Kärreman ( 2011 :10–76), argue that qualitative researchers struggle to capture and represent complex phenomena partially because they tend to collect a large amount of data. While his analysis is correct at some points – “It is necessary to do detailed, intensive, microscopic examination of the data in order to bring out the amazing complexity of what lies in, behind, and beyond those data” (Strauss 2003 :10) – much of his analysis concerns the supposed focus of qualitative research and its challenges, rather than exactly what it is about. But even in this instance we would make a weak case arguing that these are strictly the defining features of qualitative research. Some researchers seem to focus on the approach or the methods used, or even on the way material is analyzed. Several researchers stress the naturalistic assumption of investigating the world, suggesting that meaning and interpretation appear to be a core matter of qualitative research.
We can also see that in this category there is no consensus about specific qualitative methods nor about qualitative data. Many emphasize interpretation, but quantitative research, too, involves interpretation; the results of a regression analysis, for example, certainly have to be interpreted, and the form of meta-analysis that factor analysis provides indeed requires interpretation However, there is no interpretation of quantitative raw data, i.e., numbers in tables. One common thread is that qualitative researchers have to get to grips with their data in order to understand what is being studied in great detail, irrespective of the type of empirical material that is being analyzed. This observation is connected to the fact that qualitative researchers routinely make several adjustments of focus and research design as their studies progress, in many cases until the very end of the project (Kalof et al. 2008 ). If you, like Becker, do not start out with a detailed theory, adjustments such as the emergence and refinement of research questions will occur during the research process. We have thus found a number of useful reflections about qualitative research scattered across different sources, but none of them effectively describe the defining characteristics of this approach.
Although qualitative research does not appear to be defined in terms of a specific method, it is certainly common that fieldwork, i.e., research that entails that the researcher spends considerable time in the field that is studied and use the knowledge gained as data, is seen as emblematic of or even identical to qualitative research. But because we understand that fieldwork tends to focus primarily on the collection and analysis of qualitative data, we expected to find within it discussions on the meaning of “qualitative.” But, again, this was not the case.
Instead, we found material on the history of this approach (for example, Frankfort-Nachmias and Nachmias 1996 ; Atkinson et al. 2001), including how it has changed; for example, by adopting a more self-reflexive practice (Heyl 2001), as well as the different nomenclature that has been adopted, such as fieldwork, ethnography, qualitative research, naturalistic research, participant observation and so on (for example, Lofland et al. 2006 ; Gans 1999 ).
We retrieved definitions of ethnography, such as “the study of people acting in the natural courses of their daily lives,” involving a “resocialization of the researcher” (Emerson 1988 :1) through intense immersion in others’ social worlds (see also examples in Hammersley 2018 ). This may be accomplished by direct observation and also participation (Neuman 2007 :276), although others, such as Denzin ( 1970 :185), have long recognized other types of observation, including non-participant (“fly on the wall”). In this category we have also isolated claims and opposing views, arguing that this type of research is distinguished primarily by where it is conducted (natural settings) (Hughes 1971:496), and how it is carried out (a variety of methods are applied) or, for some most importantly, by involving an active, empathetic immersion in those being studied (Emerson 1988 :2). We also retrieved descriptions of the goals it attends in relation to how it is taught (understanding subjective meanings of the people studied, primarily develop theory, or contribute to social change) (see for example, Corte and Irwin 2017 ; Frankfort-Nachmias and Nachmias 1996 :281; Trier-Bieniek 2012 :639) by collecting the richest possible data (Lofland et al. 2006 ) to derive “thick descriptions” (Geertz 1973 ), and/or to aim at theoretical statements of general scope and applicability (for example, Emerson 1988 ; Fine 2003 ). We have identified guidelines on how to evaluate it (for example Becker 1996 ; Lamont 2004 ) and have retrieved instructions on how it should be conducted (for example, Lofland et al. 2006 ). For instance, analysis should take place while the data gathering unfolds (Emerson 1988 ; Hammersley and Atkinson 2007 ; Lofland et al. 2006 ), observations should be of long duration (Becker 1970 :54; Goffman 1989 ), and data should be of high quantity (Becker 1970 :52–53), as well as other questionable distinctions between fieldwork and other methods:
Field studies differ from other methods of research in that the researcher performs the task of selecting topics, decides what questions to ask, and forges interest in the course of the research itself . This is in sharp contrast to many ‘theory-driven’ and ‘hypothesis-testing’ methods. (Lofland and Lofland 1995 :5)
But could not, for example, a strictly interview-based study be carried out with the same amount of flexibility, such as sequential interviewing (for example, Small 2009 )? Once again, are quantitative approaches really as inflexible as some qualitative researchers think? Moreover, this category stresses the role of the actors’ meaning, which requires knowledge and close interaction with people, their practices and their lifeworld.
It is clear that field studies – which are seen by some as the “gold standard” of qualitative research – are nonetheless only one way of doing qualitative research. There are other methods, but it is not clear why some are more qualitative than others, or why they are better or worse. Fieldwork is characterized by interaction with the field (the material) and understanding of the phenomenon that is being studied. In Becker’s case, he had general experience from fields in which marihuana was used, based on which he did interviews with actual users in several fields.
Grounded Theory
Another major category we identified in our sample is Grounded Theory. We found descriptions of it most clearly in Glaser and Strauss’ ([1967] 2010 ) original articulation, Strauss and Corbin ( 1998 ) and Charmaz ( 2006 ), as well as many other accounts of what it is for: generating and testing theory (Strauss 2003 :xi). We identified explanations of how this task can be accomplished – such as through two main procedures: constant comparison and theoretical sampling (Emerson 1998:96), and how using it has helped researchers to “think differently” (for example, Strauss and Corbin 1998 :1). We also read descriptions of its main traits, what it entails and fosters – for instance, an exceptional flexibility, an inductive approach (Strauss and Corbin 1998 :31–33; 1990; Esterberg 2002 :7), an ability to step back and critically analyze situations, recognize tendencies towards bias, think abstractly and be open to criticism, enhance sensitivity towards the words and actions of respondents, and develop a sense of absorption and devotion to the research process (Strauss and Corbin 1998 :5–6). Accordingly, we identified discussions of the value of triangulating different methods (both using and not using grounded theory), including quantitative ones, and theories to achieve theoretical development (most comprehensively in Denzin 1970 ; Strauss and Corbin 1998 ; Timmermans and Tavory 2012 ). We have also located arguments about how its practice helps to systematize data collection, analysis and presentation of results (Glaser and Strauss [1967] 2010 :16).
Grounded theory offers a systematic approach which requires researchers to get close to the field; closeness is a requirement of identifying questions and developing new concepts or making further distinctions with regard to old concepts. In contrast to other qualitative approaches, grounded theory emphasizes the detailed coding process, and the numerous fine-tuned distinctions that the researcher makes during the process. Within this category, too, we could not find a satisfying discussion of the meaning of qualitative research.
Defining Qualitative Research
In sum, our analysis shows that some notions reappear in the discussion of qualitative research, such as understanding, interpretation, “getting close” and making distinctions. These notions capture aspects of what we think is “qualitative.” However, a comprehensive definition that is useful and that can further develop the field is lacking, and not even a clear picture of its essential elements appears. In other words no definition emerges from our data, and in our research process we have moved back and forth between our empirical data and the attempt to present a definition. Our concrete strategy, as stated above, is to relate qualitative and quantitative research, or more specifically, qualitative and quantitative work. We use an ideal-typical notion of quantitative research which relies on taken for granted and numbered variables. This means that the data consists of variables on different scales, such as ordinal, but frequently ratio and absolute scales, and the representation of the numbers to the variables, i.e. the justification of the assignment of numbers to object or phenomenon, are not questioned, though the validity may be questioned. In this section we return to the notion of quality and try to clarify it while presenting our contribution.
Broadly, research refers to the activity performed by people trained to obtain knowledge through systematic procedures. Notions such as “objectivity” and “reflexivity,” “systematic,” “theory,” “evidence” and “openness” are here taken for granted in any type of research. Next, building on our empirical analysis we explain the four notions that we have identified as central to qualitative work: distinctions, process, closeness, and improved understanding. In discussing them, ultimately in relation to one another, we make their meaning even more precise. Our idea, in short, is that only when these ideas that we present separately for analytic purposes are brought together can we speak of qualitative research.
Distinctions
We believe that the possibility of making new distinctions is one the defining characteristics of qualitative research. It clearly sets it apart from quantitative analysis which works with taken-for-granted variables, albeit as mentioned, meta-analyses, for example, factor analysis may result in new variables. “Quality” refers essentially to distinctions, as already pointed out by Aristotle. He discusses the term “qualitative” commenting: “By a quality I mean that in virtue of which things are said to be qualified somehow” (Aristotle 1984:14). Quality is about what something is or has, which means that the distinction from its environment is crucial. We see qualitative research as a process in which significant new distinctions are made to the scholarly community; to make distinctions is a key aspect of obtaining new knowledge; a point, as we will see, that also has implications for “quantitative research.” The notion of being “significant” is paramount. New distinctions by themselves are not enough; just adding concepts only increases complexity without furthering our knowledge. The significance of new distinctions is judged against the communal knowledge of the research community. To enable this discussion and judgements central elements of rational discussion are required (cf. Habermas [1981] 1987 ; Davidsson [ 1988 ] 2001) to identify what is new and relevant scientific knowledge. Relatedly, Ragin alludes to the idea of new and useful knowledge at a more concrete level: “Qualitative methods are appropriate for in-depth examination of cases because they aid the identification of key features of cases. Most qualitative methods enhance data” (1994:79). When Becker ( 1963 ) studied deviant behavior and investigated how people became marihuana smokers, he made distinctions between the ways in which people learned how to smoke. This is a classic example of how the strategy of “getting close” to the material, for example the text, people or pictures that are subject to analysis, may enable researchers to obtain deeper insight and new knowledge by making distinctions – in this instance on the initial notion of learning how to smoke. Others have stressed the making of distinctions in relation to coding or theorizing. Emerson et al. ( 1995 ), for example, hold that “qualitative coding is a way of opening up avenues of inquiry,” meaning that the researcher identifies and develops concepts and analytic insights through close examination of and reflection on data (Emerson et al. 1995 :151). Goodwin and Horowitz highlight making distinctions in relation to theory-building writing: “Close engagement with their cases typically requires qualitative researchers to adapt existing theories or to make new conceptual distinctions or theoretical arguments to accommodate new data” ( 2002 : 37). In the ideal-typical quantitative research only existing and so to speak, given, variables would be used. If this is the case no new distinction are made. But, would not also many “quantitative” researchers make new distinctions?
Process does not merely suggest that research takes time. It mainly implies that qualitative new knowledge results from a process that involves several phases, and above all iteration. Qualitative research is about oscillation between theory and evidence, analysis and generating material, between first- and second -order constructs (Schütz 1962 :59), between getting in contact with something, finding sources, becoming deeply familiar with a topic, and then distilling and communicating some of its essential features. The main point is that the categories that the researcher uses, and perhaps takes for granted at the beginning of the research process, usually undergo qualitative changes resulting from what is found. Becker describes how he tested hypotheses and let the jargon of the users develop into theoretical concepts. This happens over time while the study is being conducted, exemplifying what we mean by process.
In the research process, a pilot-study may be used to get a first glance of, for example, the field, how to approach it, and what methods can be used, after which the method and theory are chosen or refined before the main study begins. Thus, the empirical material is often central from the start of the project and frequently leads to adjustments by the researcher. Likewise, during the main study categories are not fixed; the empirical material is seen in light of the theory used, but it is also given the opportunity to kick back, thereby resisting attempts to apply theoretical straightjackets (Becker 1970 :43). In this process, coding and analysis are interwoven, and thus are often important steps for getting closer to the phenomenon and deciding what to focus on next. Becker began his research by interviewing musicians close to him, then asking them to refer him to other musicians, and later on doubling his original sample of about 25 to include individuals in other professions (Becker 1973:46). Additionally, he made use of some participant observation, documents, and interviews with opiate users made available to him by colleagues. As his inductive theory of deviance evolved, Becker expanded his sample in order to fine tune it, and test the accuracy and generality of his hypotheses. In addition, he introduced a negative case and discussed the null hypothesis ( 1963 :44). His phasic career model is thus based on a research design that embraces processual work. Typically, process means to move between “theory” and “material” but also to deal with negative cases, and Becker ( 1998 ) describes how discovering these negative cases impacted his research design and ultimately its findings.
Obviously, all research is process-oriented to some degree. The point is that the ideal-typical quantitative process does not imply change of the data, and iteration between data, evidence, hypotheses, empirical work, and theory. The data, quantified variables, are, in most cases fixed. Merging of data, which of course can be done in a quantitative research process, does not mean new data. New hypotheses are frequently tested, but the “raw data is often the “the same.” Obviously, over time new datasets are made available and put into use.
Another characteristic that is emphasized in our sample is that qualitative researchers – and in particular ethnographers – can, or as Goffman put it, ought to ( 1989 ), get closer to the phenomenon being studied and their data than quantitative researchers (for example, Silverman 2009 :85). Put differently, essentially because of their methods qualitative researchers get into direct close contact with those being investigated and/or the material, such as texts, being analyzed. Becker started out his interview study, as we noted, by talking to those he knew in the field of music to get closer to the phenomenon he was studying. By conducting interviews he got even closer. Had he done more observations, he would undoubtedly have got even closer to the field.
Additionally, ethnographers’ design enables researchers to follow the field over time, and the research they do is almost by definition longitudinal, though the time in the field is studied obviously differs between studies. The general characteristic of closeness over time maximizes the chances of unexpected events, new data (related, for example, to archival research as additional sources, and for ethnography for situations not necessarily previously thought of as instrumental – what Mannay and Morgan ( 2015 ) term the “waiting field”), serendipity (Merton and Barber 2004 ; Åkerström 2013 ), and possibly reactivity, as well as the opportunity to observe disrupted patterns that translate into exemplars of negative cases. Two classic examples of this are Becker’s finding of what medical students call “crocks” (Becker et al. 1961 :317), and Geertz’s ( 1973 ) study of “deep play” in Balinese society.
By getting and staying so close to their data – be it pictures, text or humans interacting (Becker was himself a musician) – for a long time, as the research progressively focuses, qualitative researchers are prompted to continually test their hunches, presuppositions and hypotheses. They test them against a reality that often (but certainly not always), and practically, as well as metaphorically, talks back, whether by validating them, or disqualifying their premises – correctly, as well as incorrectly (Fine 2003 ; Becker 1970 ). This testing nonetheless often leads to new directions for the research. Becker, for example, says that he was initially reading psychological theories, but when facing the data he develops a theory that looks at, you may say, everything but psychological dispositions to explain the use of marihuana. Especially researchers involved with ethnographic methods have a fairly unique opportunity to dig up and then test (in a circular, continuous and temporal way) new research questions and findings as the research progresses, and thereby to derive previously unimagined and uncharted distinctions by getting closer to the phenomenon under study.
Let us stress that getting close is by no means restricted to ethnography. The notion of hermeneutic circle and hermeneutics as a general way of understanding implies that we must get close to the details in order to get the big picture. This also means that qualitative researchers can literally also make use of details of pictures as evidence (cf. Harper 2002). Thus, researchers may get closer both when generating the material or when analyzing it.
Quantitative research, we maintain, in the ideal-typical representation cannot get closer to the data. The data is essentially numbers in tables making up the variables (Franzosi 2016 :138). The data may originally have been “qualitative,” but once reduced to numbers there can only be a type of “hermeneutics” about what the number may stand for. The numbers themselves, however, are non-ambiguous. Thus, in quantitative research, interpretation, if done, is not about the data itself—the numbers—but what the numbers stand for. It follows that the interpretation is essentially done in a more “speculative” mode without direct empirical evidence (cf. Becker 2017 ).
Improved Understanding
While distinction, process and getting closer refer to the qualitative work of the researcher, improved understanding refers to its conditions and outcome of this work. Understanding cuts deeper than explanation, which to some may mean a causally verified correlation between variables. The notion of explanation presupposes the notion of understanding since explanation does not include an idea of how knowledge is gained (Manicas 2006 : 15). Understanding, we argue, is the core concept of what we call the outcome of the process when research has made use of all the other elements that were integrated in the research. Understanding, then, has a special status in qualitative research since it refers both to the conditions of knowledge and the outcome of the process. Understanding can to some extent be seen as the condition of explanation and occurs in a process of interpretation, which naturally refers to meaning (Gadamer 1990 ). It is fundamentally connected to knowing, and to the knowing of how to do things (Heidegger [1927] 2001 ). Conceptually the term hermeneutics is used to account for this process. Heidegger ties hermeneutics to human being and not possible to separate from the understanding of being ( 1988 ). Here we use it in a broader sense, and more connected to method in general (cf. Seiffert 1992 ). The abovementioned aspects – for example, “objectivity” and “reflexivity” – of the approach are conditions of scientific understanding. Understanding is the result of a circular process and means that the parts are understood in light of the whole, and vice versa. Understanding presupposes pre-understanding, or in other words, some knowledge of the phenomenon studied. The pre-understanding, even in the form of prejudices, are in qualitative research process, which we see as iterative, questioned, which gradually or suddenly change due to the iteration of data, evidence and concepts. However, qualitative research generates understanding in the iterative process when the researcher gets closer to the data, e.g., by going back and forth between field and analysis in a process that generates new data that changes the evidence, and, ultimately, the findings. Questioning, to ask questions, and put what one assumes—prejudices and presumption—in question, is central to understand something (Heidegger [1927] 2001 ; Gadamer 1990 :368–384). We propose that this iterative process in which the process of understanding occurs is characteristic of qualitative research.
Improved understanding means that we obtain scientific knowledge of something that we as a scholarly community did not know before, or that we get to know something better. It means that we understand more about how parts are related to one another, and to other things we already understand (see also Fine and Hallett 2014 ). Understanding is an important condition for qualitative research. It is not enough to identify correlations, make distinctions, and work in a process in which one gets close to the field or phenomena. Understanding is accomplished when the elements are integrated in an iterative process.
It is, moreover, possible to understand many things, and researchers, just like children, may come to understand new things every day as they engage with the world. This subjective condition of understanding – namely, that a person gains a better understanding of something –is easily met. To be qualified as “scientific,” the understanding must be general and useful to many; it must be public. But even this generally accessible understanding is not enough in order to speak of “scientific understanding.” Though we as a collective can increase understanding of everything in virtually all potential directions as a result also of qualitative work, we refrain from this “objective” way of understanding, which has no means of discriminating between what we gain in understanding. Scientific understanding means that it is deemed relevant from the scientific horizon (compare Schütz 1962 : 35–38, 46, 63), and that it rests on the pre-understanding that the scientists have and must have in order to understand. In other words, the understanding gained must be deemed useful by other researchers, so that they can build on it. We thus see understanding from a pragmatic, rather than a subjective or objective perspective. Improved understanding is related to the question(s) at hand. Understanding, in order to represent an improvement, must be an improvement in relation to the existing body of knowledge of the scientific community (James [ 1907 ] 1955). Scientific understanding is, by definition, collective, as expressed in Weber’s famous note on objectivity, namely that scientific work aims at truths “which … can claim, even for a Chinese, the validity appropriate to an empirical analysis” ([1904] 1949 :59). By qualifying “improved understanding” we argue that it is a general defining characteristic of qualitative research. Becker‘s ( 1966 ) study and other research of deviant behavior increased our understanding of the social learning processes of how individuals start a behavior. And it also added new knowledge about the labeling of deviant behavior as a social process. Few studies, of course, make the same large contribution as Becker’s, but are nonetheless qualitative research.
Understanding in the phenomenological sense, which is a hallmark of qualitative research, we argue, requires meaning and this meaning is derived from the context, and above all the data being analyzed. The ideal-typical quantitative research operates with given variables with different numbers. This type of material is not enough to establish meaning at the level that truly justifies understanding. In other words, many social science explanations offer ideas about correlations or even causal relations, but this does not mean that the meaning at the level of the data analyzed, is understood. This leads us to say that there are indeed many explanations that meet the criteria of understanding, for example the explanation of how one becomes a marihuana smoker presented by Becker. However, we may also understand a phenomenon without explaining it, and we may have potential explanations, or better correlations, that are not really understood.
We may speak more generally of quantitative research and its data to clarify what we see as an important distinction. The “raw data” that quantitative research—as an idealtypical activity, refers to is not available for further analysis; the numbers, once created, are not to be questioned (Franzosi 2016 : 138). If the researcher is to do “more” or “change” something, this will be done by conjectures based on theoretical knowledge or based on the researcher’s lifeworld. Both qualitative and quantitative research is based on the lifeworld, and all researchers use prejudices and pre-understanding in the research process. This idea is present in the works of Heidegger ( 2001 ) and Heisenberg (cited in Franzosi 2010 :619). Qualitative research, as we argued, involves the interaction and questioning of concepts (theory), data, and evidence.
Ragin ( 2004 :22) points out that “a good definition of qualitative research should be inclusive and should emphasize its key strengths and features, not what it lacks (for example, the use of sophisticated quantitative techniques).” We define qualitative research as an iterative process in which improved understanding to the scientific community is achieved by making new significant distinctions resulting from getting closer to the phenomenon studied. Qualitative research, as defined here, is consequently a combination of two criteria: (i) how to do things –namely, generating and analyzing empirical material, in an iterative process in which one gets closer by making distinctions, and (ii) the outcome –improved understanding novel to the scholarly community. Is our definition applicable to our own study? In this study we have closely read the empirical material that we generated, and the novel distinction of the notion “qualitative research” is the outcome of an iterative process in which both deduction and induction were involved, in which we identified the categories that we analyzed. We thus claim to meet the first criteria, “how to do things.” The second criteria cannot be judged but in a partial way by us, namely that the “outcome” —in concrete form the definition-improves our understanding to others in the scientific community.
We have defined qualitative research, or qualitative scientific work, in relation to quantitative scientific work. Given this definition, qualitative research is about questioning the pre-given (taken for granted) variables, but it is thus also about making new distinctions of any type of phenomenon, for example, by coining new concepts, including the identification of new variables. This process, as we have discussed, is carried out in relation to empirical material, previous research, and thus in relation to theory. Theory and previous research cannot be escaped or bracketed. According to hermeneutic principles all scientific work is grounded in the lifeworld, and as social scientists we can thus never fully bracket our pre-understanding.
We have proposed that quantitative research, as an idealtype, is concerned with pre-determined variables (Small 2008 ). Variables are epistemically fixed, but can vary in terms of dimensions, such as frequency or number. Age is an example; as a variable it can take on different numbers. In relation to quantitative research, qualitative research does not reduce its material to number and variables. If this is done the process of comes to a halt, the researcher gets more distanced from her data, and it makes it no longer possible to make new distinctions that increase our understanding. We have above discussed the components of our definition in relation to quantitative research. Our conclusion is that in the research that is called quantitative there are frequent and necessary qualitative elements.
Further, comparative empirical research on researchers primarily working with ”quantitative” approaches and those working with ”qualitative” approaches, we propose, would perhaps show that there are many similarities in practices of these two approaches. This is not to deny dissimilarities, or the different epistemic and ontic presuppositions that may be more or less strongly associated with the two different strands (see Goertz and Mahoney 2012 ). Our point is nonetheless that prejudices and preconceptions about researchers are unproductive, and that as other researchers have argued, differences may be exaggerated (e.g., Becker 1996 : 53, 2017 ; Marchel and Owens 2007 :303; Ragin 1994 ), and that a qualitative dimension is present in both kinds of work.
Several things follow from our findings. The most important result is the relation to quantitative research. In our analysis we have separated qualitative research from quantitative research. The point is not to label individual researchers, methods, projects, or works as either “quantitative” or “qualitative.” By analyzing, i.e., taking apart, the notions of quantitative and qualitative, we hope to have shown the elements of qualitative research. Our definition captures the elements, and how they, when combined in practice, generate understanding. As many of the quotations we have used suggest, one conclusion of our study holds that qualitative approaches are not inherently connected with a specific method. Put differently, none of the methods that are frequently labelled “qualitative,” such as interviews or participant observation, are inherently “qualitative.” What matters, given our definition, is whether one works qualitatively or quantitatively in the research process, until the results are produced. Consequently, our analysis also suggests that those researchers working with what in the literature and in jargon is often called “quantitative research” are almost bound to make use of what we have identified as qualitative elements in any research project. Our findings also suggest that many” quantitative” researchers, at least to some extent, are engaged with qualitative work, such as when research questions are developed, variables are constructed and combined, and hypotheses are formulated. Furthermore, a research project may hover between “qualitative” and “quantitative” or start out as “qualitative” and later move into a “quantitative” (a distinct strategy that is not similar to “mixed methods” or just simply combining induction and deduction). More generally speaking, the categories of “qualitative” and “quantitative,” unfortunately, often cover up practices, and it may lead to “camps” of researchers opposing one another. For example, regardless of the researcher is primarily oriented to “quantitative” or “qualitative” research, the role of theory is neglected (cf. Swedberg 2017 ). Our results open up for an interaction not characterized by differences, but by different emphasis, and similarities.
Let us take two examples to briefly indicate how qualitative elements can fruitfully be combined with quantitative. Franzosi ( 2010 ) has discussed the relations between quantitative and qualitative approaches, and more specifically the relation between words and numbers. He analyzes texts and argues that scientific meaning cannot be reduced to numbers. Put differently, the meaning of the numbers is to be understood by what is taken for granted, and what is part of the lifeworld (Schütz 1962 ). Franzosi shows how one can go about using qualitative and quantitative methods and data to address scientific questions analyzing violence in Italy at the time when fascism was rising (1919–1922). Aspers ( 2006 ) studied the meaning of fashion photographers. He uses an empirical phenomenological approach, and establishes meaning at the level of actors. In a second step this meaning, and the different ideal-typical photographers constructed as a result of participant observation and interviews, are tested using quantitative data from a database; in the first phase to verify the different ideal-types, in the second phase to use these types to establish new knowledge about the types. In both of these cases—and more examples can be found—authors move from qualitative data and try to keep the meaning established when using the quantitative data.
A second main result of our study is that a definition, and we provided one, offers a way for research to clarify, and even evaluate, what is done. Hence, our definition can guide researchers and students, informing them on how to think about concrete research problems they face, and to show what it means to get closer in a process in which new distinctions are made. The definition can also be used to evaluate the results, given that it is a standard of evaluation (cf. Hammersley 2007 ), to see whether new distinctions are made and whether this improves our understanding of what is researched, in addition to the evaluation of how the research was conducted. By making what is qualitative research explicit it becomes easier to communicate findings, and it is thereby much harder to fly under the radar with substandard research since there are standards of evaluation which make it easier to separate “good” from “not so good” qualitative research.
To conclude, our analysis, which ends with a definition of qualitative research can thus both address the “internal” issues of what is qualitative research, and the “external” critiques that make it harder to do qualitative research, to which both pressure from quantitative methods and general changes in society contribute.
Acknowledgements
Financial Support for this research is given by the European Research Council, CEV (263699). The authors are grateful to Susann Krieglsteiner for assistance in collecting the data. The paper has benefitted from the many useful comments by the three reviewers and the editor, comments by members of the Uppsala Laboratory of Economic Sociology, as well as Jukka Gronow, Sebastian Kohl, Marcin Serafin, Richard Swedberg, Anders Vassenden and Turid Rødne.
Biographies
is professor of sociology at the Department of Sociology, Uppsala University and Universität St. Gallen. His main focus is economic sociology, and in particular, markets. He has published numerous articles and books, including Orderly Fashion (Princeton University Press 2010), Markets (Polity Press 2011) and Re-Imagining Economic Sociology (edited with N. Dodd, Oxford University Press 2015). His book Ethnographic Methods (in Swedish) has already gone through several editions.
is associate professor of sociology at the Department of Media and Social Sciences, University of Stavanger. His research has been published in journals such as Social Psychology Quarterly, Sociological Theory, Teaching Sociology, and Music and Arts in Action. As an ethnographer he is working on a book on he social world of big-wave surfing.
Publisher’s Note
Springer Nature remains neutral with regard to jurisdictional claims in published maps and institutional affiliations.
Contributor Information
Patrik Aspers, Email: [email protected] .
Ugo Corte, Email: [email protected] .
- Åkerström M. Curiosity and serendipity in qualitative research. Qualitative Sociology Review. 2013; 9 (2):10–18. [ Google Scholar ]
- Alford, Robert R. 1998. The craft of inquiry. Theories, methods, evidence . Oxford: Oxford University Press.
- Alvesson M, Kärreman D. Qualitative research and theory development . Mystery as method . London: SAGE Publications; 2011. [ Google Scholar ]
- Aspers, Patrik. 2006. Markets in Fashion, A Phenomenological Approach. London Routledge.
- Atkinson P. Qualitative research. Unity and diversity. Forum: Qualitative Social Research. 2005; 6 (3):1–15. [ Google Scholar ]
- Becker HS. Outsiders. Studies in the sociology of deviance . New York: The Free Press; 1963. [ Google Scholar ]
- Becker HS. Whose side are we on? Social Problems. 1966; 14 (3):239–247. [ Google Scholar ]
- Becker HS. Sociological work. Method and substance. New Brunswick: Transaction Books; 1970. [ Google Scholar ]
- Becker HS. The epistemology of qualitative research. In: Richard J, Anne C, Shweder RA, editors. Ethnography and human development. Context and meaning in social inquiry. Chicago: University of Chicago Press; 1996. pp. 53–71. [ Google Scholar ]
- Becker HS. Tricks of the trade. How to think about your research while you're doing it. Chicago: University of Chicago Press; 1998. [ Google Scholar ]
- Becker, Howard S. 2017. Evidence . Chigaco: University of Chicago Press.
- Becker H, Geer B, Hughes E, Strauss A. Boys in White, student culture in medical school. New Brunswick: Transaction Publishers; 1961. [ Google Scholar ]
- Berezin M. How do we know what we mean? Epistemological dilemmas in cultural sociology. Qualitative Sociology. 2014; 37 (2):141–151. [ Google Scholar ]
- Best, Joel. 2004. Defining qualitative research. In Workshop on Scientific Foundations of Qualitative Research , eds . Charles, Ragin, Joanne, Nagel, and Patricia White, 53-54. http://www.nsf.gov/pubs/2004/nsf04219/nsf04219.pdf .
- Biernacki R. Humanist interpretation versus coding text samples. Qualitative Sociology. 2014; 37 (2):173–188. [ Google Scholar ]
- Blumer H. Symbolic interactionism: Perspective and method. Berkeley: University of California Press; 1969. [ Google Scholar ]
- Brady H, Collier D, Seawright J. Refocusing the discussion of methodology. In: Henry B, David C, editors. Rethinking social inquiry. Diverse tools, shared standards. Lanham: Rowman and Littlefield; 2004. pp. 3–22. [ Google Scholar ]
- Brown AP. Qualitative method and compromise in applied social research. Qualitative Research. 2010; 10 (2):229–248. [ Google Scholar ]
- Charmaz K. Constructing grounded theory. London: Sage; 2006. [ Google Scholar ]
- Corte, Ugo, and Katherine Irwin. 2017. “The Form and Flow of Teaching Ethnographic Knowledge: Hands-on Approaches for Learning Epistemology” Teaching Sociology 45(3): 209-219.
- Creswell JW. Research design. Qualitative, quantitative, and mixed method approaches. 3. Thousand Oaks: SAGE Publications; 2009. [ Google Scholar ]
- Davidsson D. The myth of the subjective. In: Davidsson D, editor. Subjective, intersubjective, objective. Oxford: Oxford University Press; 1988. pp. 39–52. [ Google Scholar ]
- Denzin NK. The research act: A theoretical introduction to Ssociological methods. Chicago: Aldine Publishing Company Publishers; 1970. [ Google Scholar ]
- Denzin NK, Lincoln YS. Introduction. The discipline and practice of qualitative research. In: Denzin NK, Lincoln YS, editors. Collecting and interpreting qualitative materials. Thousand Oaks: SAGE Publications; 2003. pp. 1–45. [ Google Scholar ]
- Denzin NK, Lincoln YS. Introduction. The discipline and practice of qualitative research. In: Denzin NK, Lincoln YS, editors. The Sage handbook of qualitative research. Thousand Oaks: SAGE Publications; 2005. pp. 1–32. [ Google Scholar ]
- Emerson RM, editor. Contemporary field research. A collection of readings. Prospect Heights: Waveland Press; 1988. [ Google Scholar ]
- Emerson RM, Fretz RI, Shaw LL. Writing ethnographic fieldnotes. Chicago: University of Chicago Press; 1995. [ Google Scholar ]
- Esterberg KG. Qualitative methods in social research. Boston: McGraw-Hill; 2002. [ Google Scholar ]
- Fine, Gary Alan. 1995. Review of “handbook of qualitative research.” Contemporary Sociology 24 (3): 416–418.
- Fine, Gary Alan. 2003. “ Toward a Peopled Ethnography: Developing Theory from Group Life.” Ethnography . 4(1):41-60.
- Fine GA, Hancock BH. The new ethnographer at work. Qualitative Research. 2017; 17 (2):260–268. [ Google Scholar ]
- Fine GA, Hallett T. Stranger and stranger: Creating theory through ethnographic distance and authority. Journal of Organizational Ethnography. 2014; 3 (2):188–203. [ Google Scholar ]
- Flick U. Qualitative research. State of the art. Social Science Information. 2002; 41 (1):5–24. [ Google Scholar ]
- Flick U. Designing qualitative research. London: SAGE Publications; 2007. [ Google Scholar ]
- Frankfort-Nachmias C, Nachmias D. Research methods in the social sciences. 5. London: Edward Arnold; 1996. [ Google Scholar ]
- Franzosi R. Sociology, narrative, and the quality versus quantity debate (Goethe versus Newton): Can computer-assisted story grammars help us understand the rise of Italian fascism (1919- 1922)? Theory and Society. 2010; 39 (6):593–629. [ Google Scholar ]
- Franzosi R. From method and measurement to narrative and number. International journal of social research methodology. 2016; 19 (1):137–141. [ Google Scholar ]
- Gadamer, Hans-Georg. 1990. Wahrheit und Methode, Grundzüge einer philosophischen Hermeneutik . Band 1, Hermeneutik. Tübingen: J.C.B. Mohr.
- Gans H. Participant Observation in an Age of “Ethnography” Journal of Contemporary Ethnography. 1999; 28 (5):540–548. [ Google Scholar ]
- Geertz C. The interpretation of cultures. New York: Basic Books; 1973. [ Google Scholar ]
- Gilbert N. Researching social life. 3. London: SAGE Publications; 2009. [ Google Scholar ]
- Glaeser A. Hermeneutic institutionalism: Towards a new synthesis. Qualitative Sociology. 2014; 37 :207–241. [ Google Scholar ]
- Glaser, Barney G., and Anselm L. Strauss. [1967] 2010. The discovery of grounded theory. Strategies for qualitative research. Hawthorne: Aldine.
- Goertz G, Mahoney J. A tale of two cultures: Qualitative and quantitative research in the social sciences. Princeton: Princeton University Press; 2012. [ Google Scholar ]
- Goffman E. On fieldwork. Journal of Contemporary Ethnography. 1989; 18 (2):123–132. [ Google Scholar ]
- Goodwin J, Horowitz R. Introduction. The methodological strengths and dilemmas of qualitative sociology. Qualitative Sociology. 2002; 25 (1):33–47. [ Google Scholar ]
- Habermas, Jürgen. [1981] 1987. The theory of communicative action . Oxford: Polity Press.
- Hammersley M. The issue of quality in qualitative research. International Journal of Research & Method in Education. 2007; 30 (3):287–305. [ Google Scholar ]
- Hammersley, Martyn. 2013. What is qualitative research? Bloomsbury Publishing.
- Hammersley M. What is ethnography? Can it survive should it? Ethnography and Education. 2018; 13 (1):1–17. [ Google Scholar ]
- Hammersley M, Atkinson P. Ethnography . Principles in practice . London: Tavistock Publications; 2007. [ Google Scholar ]
- Heidegger M. Sein und Zeit. Tübingen: Max Niemeyer Verlag; 2001. [ Google Scholar ]
- Heidegger, Martin. 1988. 1923. Ontologie. Hermeneutik der Faktizität, Gesamtausgabe II. Abteilung: Vorlesungen 1919-1944, Band 63, Frankfurt am Main: Vittorio Klostermann.
- Hempel CG. Philosophy of the natural sciences. Upper Saddle River: Prentice Hall; 1966. [ Google Scholar ]
- Hood JC. Teaching against the text. The case of qualitative methods. Teaching Sociology. 2006; 34 (3):207–223. [ Google Scholar ]
- James W. Pragmatism. New York: Meredian Books; 1907. [ Google Scholar ]
- Jovanović G. Toward a social history of qualitative research. History of the Human Sciences. 2011; 24 (2):1–27. [ Google Scholar ]
- Kalof L, Dan A, Dietz T. Essentials of social research. London: Open University Press; 2008. [ Google Scholar ]
- Katz J. Situational evidence: Strategies for causal reasoning from observational field notes. Sociological Methods & Research. 2015; 44 (1):108–144. [ Google Scholar ]
- King G, Keohane RO, Sidney S, Verba S. Scientific inference in qualitative research. Princeton: Princeton University Press; 1994. Designing social inquiry. [ Google Scholar ]
- Lamont M. Evaluating qualitative research: Some empirical findings and an agenda. In: Lamont M, White P, editors. Report from workshop on interdisciplinary standards for systematic qualitative research. Washington, DC: National Science Foundation; 2004. pp. 91–95. [ Google Scholar ]
- Lamont M, Swidler A. Methodological pluralism and the possibilities and limits of interviewing. Qualitative Sociology. 2014; 37 (2):153–171. [ Google Scholar ]
- Lazarsfeld P, Barton A. Some functions of qualitative analysis in social research. In: Kendall P, editor. The varied sociology of Paul Lazarsfeld. New York: Columbia University Press; 1982. pp. 239–285. [ Google Scholar ]
- Lichterman, Paul, and Isaac Reed I (2014), Theory and Contrastive Explanation in Ethnography. Sociological methods and research. Prepublished 27 October 2014; 10.1177/0049124114554458.
- Lofland J, Lofland L. Analyzing social settings. A guide to qualitative observation and analysis. 3. Belmont: Wadsworth; 1995. [ Google Scholar ]
- Lofland J, Snow DA, Anderson L, Lofland LH. Analyzing social settings. A guide to qualitative observation and analysis. 4. Belmont: Wadsworth/Thomson Learning; 2006. [ Google Scholar ]
- Long AF, Godfrey M. An evaluation tool to assess the quality of qualitative research studies. International Journal of Social Research Methodology. 2004; 7 (2):181–196. [ Google Scholar ]
- Lundberg G. Social research: A study in methods of gathering data. New York: Longmans, Green and Co.; 1951. [ Google Scholar ]
- Malinowski B. Argonauts of the Western Pacific: An account of native Enterprise and adventure in the archipelagoes of Melanesian New Guinea. London: Routledge; 1922. [ Google Scholar ]
- Manicas P. A realist philosophy of science: Explanation and understanding. Cambridge: Cambridge University Press; 2006. [ Google Scholar ]
- Marchel C, Owens S. Qualitative research in psychology. Could William James get a job? History of Psychology. 2007; 10 (4):301–324. [ PubMed ] [ Google Scholar ]
- McIntyre LJ. Need to know. Social science research methods. Boston: McGraw-Hill; 2005. [ Google Scholar ]
- Merton RK, Barber E. The travels and adventures of serendipity . A Study in Sociological Semantics and the Sociology of Science. Princeton: Princeton University Press; 2004. [ Google Scholar ]
- Mannay D, Morgan M. Doing ethnography or applying a qualitative technique? Reflections from the ‘waiting field‘ Qualitative Research. 2015; 15 (2):166–182. [ Google Scholar ]
- Neuman LW. Basics of social research. Qualitative and quantitative approaches. 2. Boston: Pearson Education; 2007. [ Google Scholar ]
- Ragin CC. Constructing social research. The unity and diversity of method. Thousand Oaks: Pine Forge Press; 1994. [ Google Scholar ]
- Ragin, Charles C. 2004. Introduction to session 1: Defining qualitative research. In Workshop on Scientific Foundations of Qualitative Research , 22, ed. Charles C. Ragin, Joane Nagel, Patricia White. http://www.nsf.gov/pubs/2004/nsf04219/nsf04219.pdf
- Rawls, Anne. 2018. The Wartime narrative in US sociology, 1940–7: Stigmatizing qualitative sociology in the name of ‘science,’ European Journal of Social Theory (Online first).
- Schütz A. Collected papers I: The problem of social reality. The Hague: Nijhoff; 1962. [ Google Scholar ]
- Seiffert H. Einführung in die Hermeneutik. Tübingen: Franke; 1992. [ Google Scholar ]
- Silverman D. Doing qualitative research. A practical handbook. 2. London: SAGE Publications; 2005. [ Google Scholar ]
- Silverman D. A very short, fairly interesting and reasonably cheap book about qualitative research. London: SAGE Publications; 2009. [ Google Scholar ]
- Silverman D. What counts as qualitative research? Some cautionary comments. Qualitative Sociology Review. 2013; 9 (2):48–55. [ Google Scholar ]
- Small ML. “How many cases do I need?” on science and the logic of case selection in field-based research. Ethnography. 2009; 10 (1):5–38. [ Google Scholar ]
- Small, Mario L 2008. Lost in translation: How not to make qualitative research more scientific. In Workshop on interdisciplinary standards for systematic qualitative research, ed in Michelle Lamont, and Patricia White, 165–171. Washington, DC: National Science Foundation.
- Snow DA, Anderson L. Down on their luck: A study of homeless street people. Berkeley: University of California Press; 1993. [ Google Scholar ]
- Snow DA, Morrill C. New ethnographies: Review symposium: A revolutionary handbook or a handbook for revolution? Journal of Contemporary Ethnography. 1995; 24 (3):341–349. [ Google Scholar ]
- Strauss AL. Qualitative analysis for social scientists. 14. Chicago: Cambridge University Press; 2003. [ Google Scholar ]
- Strauss AL, Corbin JM. Basics of qualitative research. Techniques and procedures for developing grounded theory. 2. Thousand Oaks: Sage Publications; 1998. [ Google Scholar ]
- Swedberg, Richard. 2017. Theorizing in sociological research: A new perspective, a new departure? Annual Review of Sociology 43: 189–206.
- Swedberg R. The new 'Battle of Methods'. Challenge January–February. 1990; 3 (1):33–38. [ Google Scholar ]
- Timmermans S, Tavory I. Theory construction in qualitative research: From grounded theory to abductive analysis. Sociological Theory. 2012; 30 (3):167–186. [ Google Scholar ]
- Trier-Bieniek A. Framing the telephone interview as a participant-centred tool for qualitative research. A methodological discussion. Qualitative Research. 2012; 12 (6):630–644. [ Google Scholar ]
- Valsiner J. Data as representations. Contextualizing qualitative and quantitative research strategies. Social Science Information. 2000; 39 (1):99–113. [ Google Scholar ]
- Weber, Max. 1904. 1949. Objectivity’ in social Science and social policy. Ed. Edward A. Shils and Henry A. Finch, 49–112. New York: The Free Press.
- Open access
- Published: 19 August 2024
Physical activity policies in Saudi Arabia and Oman: a qualitative study using stakeholder interviews
- Ali Ahmed Alzahrani 1 ,
- Peter Gelius 2 ,
- Adrian E. Bauman 3 &
- Klaus Gebel ORCID: orcid.org/0000-0003-0330-7756 1
Health Research Policy and Systems volume 22 , Article number: 111 ( 2024 ) Cite this article
15 Accesses
1 Altmetric
Metrics details
Countries in the Middle East have some of the lowest rates of physical activity and some of the highest rates of obesity in the world. Policies can influence population levels of physical activity. However, there is a dearth of research on physical activity policies in the Gulf region. This qualitative study analyses cross-sectoral barriers and facilitators for the development, implementation and evaluation of physical activity policies in Saudi Arabia and Oman, two of the largest countries in the region.
Semi-structured interviews were conducted with 19 senior policymakers from the Ministries of Health, Education, and Sport in Saudi Arabia and Oman, and were examined using thematic analysis.
We identified seven themes related to physical activity policies in Saudi Arabia and Oman: leadership; existing policies; physical activity programs related to policies; private sector policies; challenges; data/monitoring; and future opportunities. Both countries have a central document that guides policy-makers in promoting physical activity, and the available policies in both countries are implemented via multiple programs and initiatives to increase physical activity. Compared with Oman, in Saudi Arabia, programs from the non-profit sector, represented by community groups, play a more significant role in promoting physical activity outside the government framework. The private sector has contributed to promoting physical activity in both countries, but interviewees stated that more financial support is required. Policy limitations differ between Saudi Arabia and Oman: intersectoral collaboration in Oman is limited and mainly based on individuals’ own initiative, while the health transformation in Saudi Arabia tends to slow down policy implementation in relevant areas. Physical education in Saudi Arabia and Oman is similar; however, increased support and collaboration between government agencies and the private sector for out-of-school sports academies are needed.
Conclusions
This study addresses key gaps in analysing physical activity policies in Gulf Cooperation Council countries. Our study highlights the importance of increasing financial support, improving collaboration between governmental agencies and between them and the private sector and consolidating efforts to back physical activity policies and dismantle cross-sectoral barriers in Saudi Arabia and Oman. Educational institutions in Saudi Arabia and Oman play a crucial role in promoting physical activity from early childhood to young adults. Our insights assist policy-makers, public health officials and stakeholders in shaping effective physical activity-promoting policies, programs and interventions to prevent non-communicable diseases. Challenges identified in Saudi Arabia and Oman's policies will inform their future development.
Peer Review reports
Despite the health benefits of physical activity [ 1 , 2 , 3 ], in most countries, large proportions of the population remain insufficiently active [ 4 , 5 ]. In line with social–ecological models of health [ 6 ], the need for policies to increase population levels of physical activity has been highlighted [ 7 , 8 ]. Physical activity policy is defined as any formal or informal legislative or regulatory action or organized guidance provided by governments and organizations [ 9 , 10 , 11 , 12 ]. Policies can be cross-sectoral and encompass access to various forms of physical activity, including walking and cycling, regulate and promote programs and initiatives, and provide a comprehensive framework for the design, funding and implementation of diverse physical activity interventions [ 9 , 13 , 14 ].
Countries in the Middle East have amongst the lowest levels of physical activity and some of the highest rates of overweight and obesity in the world [ 4 , 15 ]. In Saudi Arabia and Oman, only 29.7% and 25.6% of the adult population, respectively, meet the physical activity recommendations. For the Middle East and North Africa (MENA) region the rate is 38.5% and the global average is 31.3% [ 4 ]. Moreover, in 2020 in Oman, Qatar, Bahrain, Kuwait and the United Arab Emirates, more than 80% of teenagers and 55% of adults (45% of men and 65% of women) were not sufficiently active [ 16 ]. In Saudi Arabia and Oman, dietary patterns are shifting due to increased consumption of processed foods, leading to higher rates of obesity, diabetes and cardiovascular diseases. Traditional diets rich in fruits, vegetables and lean meats are being replaced by fast food and sugary drinks. Moreover, reduced physical activity and increasingly sedentary lifestyles are major determinants of the obesity epidemic [ 17 , 18 ]. Therefore, there is an urgent need in this region for action on physical activity, including policy development, implementation and evaluation.
The 2014 report from the WHO Regional Office for the Eastern Mediterranean on promoting physical activity in the region included a policy mapping exercise on national policy and action on physical activity in all 22 member states [ 19 ]. National physical activity policies were reported for most countries, including all members of the Gulf Cooperation Council (GCC), a political and economic alliance of six Middle Eastern countries [Saudi Arabia, Kuwait, the United Arab Emirates (UAE), Qatar, Bahrain and Oman] that share a similar cultural, social and economic background. According to WHO’s recent Global Status Report on Physical Activity [ 16 ], only 11 of the 22 countries in the Eastern Mediterranean Region had a national physical activity strategy, while all GCC countries had one. The report also identified some partnerships to promote physical activity between various ministries in the Gulf states, particularly across the health, sports and education sectors.
In a systematic scoping review, Klepac Pogrmilovic et al. examined the academic literature on physical activity policy and identified 163 papers, covering 168 countries, including all 6 GCC members [ 20 ]. Few papers on physical activity policy across the Eastern Mediterranean region were identified, thus, the findings for the region were largely based on the 2014 report of the WHO Regional Office [ 20 ]. In another study by Klepac Pogrmilovic and colleagues, a survey on national physical activity policies was completed by representatives of 76 countries. However, this only included three of the 22 countries from the Eastern Mediterranean Region. The authors also emphasized that although national policies and strategies exist in all GCC countries, implementation is lacking. The authors recommended that further research be undertaken into physical activity and sedentary behaviour policy in the region [ 8 ]. It is essential to contextualize these findings with regard to physical activity policies in the Gulf region, including disparities and challenges in their implementation.
Recently, Albujulaya et al. analysed physical activity policy initiatives in Saudi Arabia by conducting semi-structured interviews with three policy-makers from the Ministries of Education and Sports and with six Saudi academics working in this field [ 21 ]. Surprisingly, they did not interview anyone from the Ministry of Health. They concluded that while physical activity amongst Saudis overall has increased, levels among women are still low. While Albujulaya et al. analysed aspects of physical activity policy development, implementation and evaluation in Saudi Arabia, they did not address potential cross-sectoral barriers and facilitators for these processes.
The primary objective of the present study was to analyse cross-sectoral barriers and facilitators for physical activity policy development, implementation and evaluation in GCC countries. The best research paradigm for this study is qualitative research. We chose interpretivist research to describe complex social realities, explore new or under-researched subjects and generate hypotheses for future quantitative research. This approach allows for a detailed examination of phenomena, which is difficult with quantitative methods. Practically, we also lacked a large, easily accessible sample to survey quantitatively. Therefore, interpretivism is particularly effective for understanding stakeholder perspectives on physical activity policies in unique cultural settings, such as those in Saudi Arabia and Oman, providing deep insights into subjective experiences and nuanced views. To achieve this, we interviewed policy-makers from the Ministries of Health, Sport and Education in Saudi Arabia and Oman, the two largest countries in the GCC. We picked these three ministries as previous research highlights that they are chiefly responsible for promoting physical activity and implementing related policies in the Gulf region [ 22 , 23 , 24 ].
Study design
This is a qualitative study using the interpretivist research paradigm [ 25 ], comprising semi-structured interviews with key stakeholders in physical activity policy-making in Saudi Arabia and Oman. We utilized the COREQ checklist to ensure the rigour and transparency of our qualitative methods (Appendix 1).
Sampling and recruitment
We defined stakeholders as those that are directly involved in the development, implementation and evaluation of physical activity policies. We initially sought to conduct interviews with stakeholders in all six GCC countries. We attempted to reach a contact in the Ministry of Health (MoH) in each GCC country by writing to the official email address of the ministries to introduce the study and request the contact details of relevant stakeholders. Despite following up with non-responders, we did not receive any replies from Qatar, Bahrain, Kuwait and the United Arab Emirates. Therefore, we narrowed the scope to Saudi Arabia and Oman, the two largest countries of the six, with 36.4 million and 4.6 million inhabitants, respectively, accounting for 70% of the GCC’s population [ 26 ]. After obtaining contact details of key stakeholders from the ministries, the participants were contacted directly via email or telephone. To recruit additional participants, we used snowball sampling by asking interviewees to provide contact details of other relevant stakeholders in their organization. Because of the documented previous involvement of the sports and education sectors in promoting physical activity in the Gulf States [ 19 ], we also asked participants to identify relevant stakeholders in the Ministries of Sports and Education in Saudi Arabia and Oman. Our study focussed on the Ministries of Sports, Health and Education as they are significantly involved in promoting physical activity within Gulf Cooperation Council countries [ 22 , 23 , 24 ]. Attempts to contact the ministers directly were unsuccessful. However, we managed to reach senior staff in the ministries and in university sports federations which belong to the Ministries of Education. Additionally, we employed different strategies to engage participants from the non-governmental sector in both countries, including networking through professional contacts of the first author, utilizing online platforms such as Twitter and Facebook and forums and capitalizing on established partnerships with both non-governmental organizations (NGOs) and governmental sectors.
Participants were provided with information on the study, and all gave written consent to participate. Additionally, participants were informed at the beginning of the interview that they could withdraw from the study at any time if they chose not to continue participating. An interview guide, based on a review of previous literature [ 22 , 24 ] and collaborative input from the authors, was developed (Appendix 2). In the interviews, we explored stakeholders’ perspectives and opinions regarding existing physical activity policy documents from the Ministries of Health, Education and Sport in their country, as well as facilitators and barriers to physical activity policy development, implementation and evaluation, with field notes made during the interviews. A.A. conducted the interviews via Zoom due to the coronavirus disease 2019 (COVID-19) pandemic between December 2021 and February 2022. Interviews were conducted in Arabic, were audio-recorded with permission obtained from the participants and transcribed verbatim, and lasted between 10 min and 45 min. Repeat interviews were not carried out. The following personal information was collected from each participant: name, organization, position, age, gender and previous work experience. The participants were provided with an opportunity to review the Arabic transcripts for accuracy, and the final transcripts were translated to English. Monitoring of data saturation, an ongoing process based on the notion of informational redundancy [ 27 ], was conducted to ensure that comprehensive insights were obtained. The determination of the number of samples needed to reach data saturation was done separately in each country.
Data analysis
Authors A.A., A.B. and P.G. reviewed the transcripts to familiarize themselves with the content, after which thematic analysis was jointly undertaken by A.A., A.B. and P.G. to code each transcript. Themes were subsequently developed through a partially deductive approach: Main categories in Table 1 were derived from existing frameworks of the policy process, such as the HARDWIRED framework [ 28 ] (covering aspects such as development process, partnerships, resources, communication, evaluation and evidence-base) and CAPPA criteria [ 29 ] (including sectors/institutions involved, implementation, legal status, target groups, goals and targets, timeframe, budget and evaluation/surveillance). Subsequently, sub-categories were added via an inductive process conducted by A.A. during the thematic exploration stage, involving a comprehensive review of all codes within the combined dataset encompassing both Saudi Arabia and Oman. A.A., A.B. and P.G. discussed the coding and the preliminary interpretations to cross-validate the findings. These themes were informed by a comprehensive analysis of the extant literature and relevant findings from previous studies [ 22 , 24 ]. As a medical doctor at King Faisal Medical City in the southern region of Saudi Arabia, the lead author’s professional background informed his approach to data analysis in this qualitative study.
Ethical approval
The study was approved by the Human Research Ethics Committee of the University of Technology Sydney (UTS HREC ref. no. ETH21-6428).
Interviews were conducted with 19 high-level stakeholders in physical activity policy; 12 from Saudi Arabia and 7 from Oman. A total of four of the participants (two from each country) were women. In Saudi Arabia, four participants were from the Ministry of Health (MoH, including one from an NGO that is supervised by the MoH), five were from the Ministry of Education (MoE) and three represented the Ministry of Sport (MoS). In Oman, one participant represented the Ministry of Health, three were from the Ministry of Education and three were from the Ministry of Sport.
We generated six themes regarding physical activity policies in Saudi Arabia and Oman: leadership; existing policy documents; implementation of physical activity policies; challenges; data/monitoring for physical activity policies; and future opportunities. The theme non-profit sector/community groups was generated only for Saudi Arabia. Table 1 provides an overview of the derived main themes and findings.
- Saudi Arabia
Participants from the Saudi MoH and MoS stated that the leadership in the legislation, regulation, and evaluation of physical activity policies is divided between the two ministries. They also collaborate with other government agencies, including the MoE and the Ministry of Municipal and Rural Affairs, to increase physical activity opportunities in schools, workplaces and communities. Two participants from the MoE mentioned that the University Affairs Council and the Supreme Economic Council also work in the promotion of physical activity by regulating the implementation of programs in their sectors according to policy documents from the MoH and MoE.
According to the participant from the NGO supervised by the MoH, the non-profit sector is instrumental in promoting physical activity through walking groups. These have been established throughout the country to provide opportunities for people to engage in physical activity and to socialize, and which are co-organized by various stakeholders, including local businesses, schools, healthcare providers and government agencies: “I firmly believe that the non-profit sector plays a pivotal role in advocating for physical activity in Saudi Arabia” (NGO participant).
Participants from the Saudi MoH underlined that Health in All Policies is one of the main objectives in the new health care transformation in the kingdom. Most participants from the three ministries identified a certain level of cooperation between different stakeholders, particularly between the Ministries of Health, Sport and Education.
The Omani MoH leads the policies and programs to promote physical activity. Participant 1 from the MoH said that their non-communicable disease (NCD) and Health Committees are crucial for leading policy-making and promoting physical activity related to the National Policy for Prevention and Control of NCDs document [ 30 ].
Regarding Health in All Policies, all participants from Oman stated that there is cooperation between the different ministries, but that it is limited and based on individual agencies’ own initiatives rather than combined efforts or a policy imprimatur: “Partnerships exist, but they are limited and based on individual initiatives” (participant 2 from the MoS).
Relevant existing policies
Most of the participants from all three ministries in Saudi Arabia referred to the Quality of Life document [ 31 ], an economic and social reform blueprint that is part of the government’s overarching Saudi Vision 2030 development program [ 32 ]. The Quality of Life document includes the most important physical activity policies implemented by the three ministries: “There is no doubt that the 2030 Vision is our basic guidance” (participant 3 from the MoH). “Before 2017 there were no clear policies. Everyone works on vision files, and everyone has to achieve the [Vision 2030] target to increase the quality of life of the Saudi community” (participant 2 from the MoE). The Quality of Life document emphasizes the need to enhance public health and healthcare services, promote healthy behaviours, and provide opportunities for physical activity and sports participation.
Interviewees from the MoE highlighted that the University Sports Federation strategy promotes physical activity and sport in tertiary education [ 33 ]. Some important miscellaneous policy documents were identified by staff of the three ministries, such as the National Strategy for Healthy Food and Physical Activity 2015–2025 [ 34 ], the Physical Activity Guidelines for Health Practitioners [ 35 ], the 24-Hour Movement Guidelines [ 36 ] (all by the MoH) and the annual report of the Sport for All Federation by the MoS [ 37 ].
Almost all participants across all three ministries stated that the government of Oman’s overarching Vision 2040 [ 38 ] is currently the most important policy document. Participants from the MoE reported that the Vision 2040 guides the promotion of student physical activity, with support from related documents such as the student learning calendar, education document, standards document and the school sports curriculum. These policy documents aim to promote physical activity among students by better integrating physical education (PE) classes into the overall curriculum to encourage regular physical activity and healthy habits. According to participant 1 from the MoH, the Education Document is a comprehensive strategy, including PE and promotion of physical activity in schools and universities. The document outlines various initiatives, policies and guidelines to ensure that education includes a focus on physical health and fitness [ 39 ]. Three participants from the three different Omani ministries referred to the National Policy for Prevention and Control of NCDs [ 30 ], published by the MoH, as the central policy document for physical activity promotion in Oman. Three study participants from the MoS suggested that the Omani Sports Strategy [ 40 ] is important for guiding the promotion of physical activity for different segments of society.
Implementation of physical activity policies
(a) physical activity programs.
The MoH and MoE collaborate on a range of health-related issues (obesity, diabetes, mental health and visual acuity) to improve the overall health of school students and to detect problems at an early stage [ 41 ]. This led to the development of the Rashaka initiative, a large-scale multi-component school-based obesity prevention program, which started in 2016 and involved nearly 1000 schools across the country. In 2020, Rashaka was replaced by an annual program composed of screening of students for early signs of chronic diseases and lectures highlighting the significance of physical activity and a healthy diet.
Participants 3 and 4 of the Saudi MoH mentioned the Walk 30 Minutes initiative, which was implemented in 2021 and intends to increase physical activity through mass media and social media, and forms part of the National Strategy for Healthy Food and Physical Activity 2015–2025 of the MoH. An initiative by the University Sports Federation [ 33 ] (related to policies from the MoS), aiming to support sports facilities and clubs for girls and women, was mentioned by three participants from the MoS, and one participant highlighted the MoS’s Talent Support Program to identify and develop talented athletes in different sports.
Participant 3 of the Saudi MoH and participant 5 of the MoE mentioned the Healthy Mall Campaign and the Healthy Campus Project to promote physical activity in air-conditioned malls and universities. These initiatives are related to policies of the National Strategy for Healthy Food and Physical Activity (MoH and MoE). Despite challenges, the study participants considered the Saudi physical activity programs to be effective.
Participants 1 and 2 of the MoE identified programs related to PE policies from the Education Document, which include increasing the number of PE classes per week and organizing tournaments in different sports. According to participants from the three different ministries, a range of physical activity programs took place during the COVID-19 pandemic, including campaigns calling for physical activity at home using apps with exercise videos. These initiatives, as reported by the participants, align with and are supported by the physical activity policy documents from the Ministries of Health, Sport and Education, that is, the National Policy for Prevention and Control of NCDs [ 30 ] and the Oman Sport Strategy [ 40 ].
According to multiple participants, several community-based initiatives were underway in Oman, such as Active Community, Healthy Cities and Healthy Villages and The Green Playgrounds Project. These initiatives had been set up in all Omani cities to make the built environment more activity friendly, for example, by improving neighbourhood walkability, which is also based on the National Policy for Prevention and Control of NCDs [ 30 ].
(B) Private sector
According to most of the participants, the private sector makes important contributions to physical activity promotion in Saudi Arabia by being directly involved in the policy development process. The Tatweer Educational Company, a private holding dedicated to implementing the government's education reform initiative, has developed programs to promote physical activity in schools, aligning with its commitment to a holistic education system. In line with the conceptualization of active travel as physical activity, the Red Sea Company drives the development of Saudi Arabia’s west coast and aims to improve neighbourhood walkability. Participants from the MoE were unanimous in the view that private universities make significant contributions to the promotion of physical activity among staff and students on the basis of financial support from their funders. International and local investors in the education sector are urging private universities to promote physical activity to enhance the universities’ reputation and to be more attractive for prospective students.
According to participant 1 from the Ministry of Health, walking and hiking groups supported by the Health Promotion Center [ 42 ] (a non-profit charitable organization under the umbrella of the Saudi Ministry of Labor and Social Affairs) play an important role in promoting physical activity in Saudi Arabia. This is despite a lack of governmental support, with influencers and celebrities utilizing social media platforms for the promotion of physical activity. This organization has internal policies for the promotion of physical activity through different programs: “The fact is that community groups working to spread this culture of walking have no ceilings, no limits, and no bureaucracy” (participant 1 from the MoH).
In contrast to Saudi Arabia, participants in Oman stated that more support from the private sector is needed to promote physical activity in the country. However, participant 3 from the MoE mentioned an agreement between the MoE and Muscat Pharmacy & Stores limited liability company (LLC) to hold a football tournament for elementary school students. Nevertheless, it is important to highlight that there is a prevailing perception that physical activity cannot be easily monetized. This contributes to the private sector’s limited interest in supporting such initiatives in the Gulf countries, as it is not perceived to yield immediate profits. Overall, the participants from both Saudi Arabia and Oman stated that, unlike in government-supported programs, in the private sector there are no performance indicators to track the progress of physical activity programs.
Data/monitoring
Two participants from the Saudi MoH and MoE mentioned challenges in relation to physical activity monitoring, as each of the three involved ministries independently conducts surveys on physical activity with different methodologies and tools, which may yield conflicting results in monitoring the effects or end-product of physical activity policies. Furthermore, participants from all three ministries confirmed the existence of national surveys for physical activity and emphasized their importance in assessing activity levels. They also shared insights about how they monitor the implementation of physical activity policies and evaluate the effectiveness of various initiatives in their respective sectors: “We follow and evaluate health through periodic national surveys, but each ministry has its own survey with different results. It is not clear how widely these data are utilized in different sectors, such as education, and sports, to inform policies and interventions” (participant 2 from the MoH).
Participants from the MoH and MoS stated that participation rates, policy compliance, budget allocation and health outcomes are being tracked to assess the effectiveness and progress of physical activity policies, with a specific focus on regular reports and data analysis. Furthermore, one participant from the MoE said that there is no monitoring happening for their education-specific policies.
Participant 4 from the MoH suggested that limited policy implementation is likely until the health transformation process is completed by 2030. The health transformation in Saudi Arabia is a recent, prominent project that aims to restructure the health sector to make it more comprehensive, effective and integrated. The transformation is mainly focussed on the prevention of chronic diseases and the promotion of a healthy lifestyle through physical activity and a nutritious diet. On the ground, this transformation entails significant changes in healthcare infrastructure, delivery and accessibility, as well as increased awareness campaigns and the implementation of various programs aimed at improving public health and wellbeing [ 43 ]. To expedite the health transformation in Saudi Arabia, the respondent considered it essential to implement the policies from the Quality of Life document, which is part of the Kingdom’s Vision 2030. This particularly involves promoting physical activity and healthy lifestyles. By fostering these habits, the country could achieve significant improvements in public health and wellbeing. Participant 3 suggested that bureaucracy is a limitation, as new policies and regulations take a long time to implement under different administrations. Moreover, participants from the MoH and MoE declared that a lack of sustainability of physical activity programs and funding were major limitations due to changes in administrations over short periods. Most participants representing the three Saudi ministries stated that a lack of collaboration and cooperation between government departments was a major challenge. For instance, some policies from the MoH need to be implemented by the MoE, such as the screening program of school students [ 41 ]. The hot climate and the desert environment in the Gulf region also present challenges when it comes to implementing physical activity policies.
According to most participants, a lack of funding for the promotion of physical activity was a common challenge in Saudi Arabia because health budgets are mostly allocated to hospitals and curative services rather than to NCD prevention, including physical activity promotion. A lack of facilities (e.g. sports fields, recreation centres, indoor and outdoor courts and multi-use sporting hubs) is a further challenge according to most Saudi respondents. Moreover, negative societal attitudes towards physical activity were highlighted by one participant, citing challenges such as the prevalence of social norms that prioritize sedentary pastimes and leisure activities, thereby reinforcing a culture that tends to discourage physical activity [ 44 ].
According to most participants, insufficient collaboration between different stakeholders, as well as a lack of government funding and support from the private sector, were the most important barriers to physical activity promotion. Like in Saudi Arabia, participants from Oman said that the hot climate and desert environment in the Gulf region, a lack of facilities and/or a lack of access to them and negative attitudes in the population towards physical activity (social norms that prioritize sedentary pastimes and leisure activities) all pose obstacles to physical activity. Some participants from the MoE stated that a lack of support for PE facilities in schools has been a major obstacle to increasing students’ physical activity. Like in Saudi Arabia, respondents from Oman felt that the country should rapidly implement the provisions of the Vision 2040, specifically those related to the prevention of chronic diseases through the promotion of a healthy lifestyle and physical activity. Furthermore, an increase in government funding dedicated to promoting physical activity would be desirable.
Future opportunities
Participant 3 from the Saudi MoH stated that future policies might include investment in technology, such as developing smartphone apps for the promotion of physical activity during pandemics, and these could contribute to future physical activity programs. However, this requires direct support from the government, both financially and technically, by providing technical expertise, developing and implementing technology-based solutions and ensuring privacy and security. Furthermore, participant 1 from the MoS and participant 3 of the MoE claimed that programs, such as out-of- school academies for talented young athletes, could further encourage physical activity and foster athletic talent. Three participants from the MoE pointed out that unifying efforts between different stakeholders would be important. Moreover, more support in terms of funding, infrastructure, a skilled workforce, and research are considered promising opportunities by most participants from the three ministries.
Participants from the three ministries stated that community participation, such as activating schools as centres for physical activity promotion in the community, would be a great opportunity in the future if cooperation between different ministries is enhanced. Three participants from the Omani MoS and MoE said that improvements to facilities and the skills of the workforce (e.g. PE teachers, coaches, gym instructors, etc.) are required to increase activity levels. Despite the existence of the national plan for physical activity promotion in Oman [ 45 ], all participants emphasized that the lack of partnerships between the government and other relevant parties, such as schools, sports clubs and community organizations, is a common and significant obstacle. Furthermore, participant 1 from the MoS and participant 3 from the MoE said that more support for out-of-school sport academies, including improved collaboration between governmental agencies and the private sector, and supporting physical education as a part of the Omani education strategy, would be great opportunities.
Participants from the Ministries of Education of Saudi Arabia and Oman highlighted that physical education is crucial for the future in both countries, fostering healthy habits essential for a productive workforce. Integrating physical education into the curriculum promotes wellness and prevents lifestyle-related diseases, contributing to various sectors [ 39 ]. As both nations progress, emphasizing physical education will enhance individual health and serve as a strategic investment in a robust, dynamic workforce.
This study examined cross-sectoral barriers and facilitators for physical activity policy development, implementation and evaluation in Saudi Arabia and Oman by interviewing high-level stakeholders from their Ministries of Health, Sports and Education. Oman established a national policy framework for physical activity in 2014 [ 18 ] under the umbrella of the Oman Vision 2040 [ 38 ], 3 years before Saudi Arabia introduced its Quality of Life Program [ 46 ] under the umbrella of the Saudi Vision 2030 [ 31 ]. Many programs and initiatives to enhance physical activity in both countries were identified, particularly sports initiatives. However, it is worth noting that these efforts often lack comprehensiveness, encompassing a broad range of activities and demographics, and face challenges in effective implementation – observations that are in line with findings from a survey on national physical activity policies by Klepac Pogrmilovic et al. [ 8 ]. The programs and initiatives are predominantly focussed on urban areas and may neglect rural communities [ 44 , 47 ]. Interest in promoting gender equity in physical activity in Oman was prominent, with several programs for female participants [ 24 ]; there was less focus on gender equity in Saudi Arabia, although a positive development was the introduction of PE classes for female students in primary and secondary education in 2018. Policies for promoting physical activity should also support individuals with special needs, patients and the elderly by implementing community programs targeted at these groups, such as walking groups and fitness classes for older adults, and programs targeting people with chronic conditions. Additionally, it is important to focus on policies and programs that support gender equality in physical activity and health.
Our finding regarding insufficient backing of policy interventions to create environments supportive of physical activity in both countries aligns with a study by Allender et al., who interviewed stakeholders in local government in Victoria, Australia, to analyse physical activity policies and initiatives. Similar to our findings from Saudi Arabia and Oman, they identified a lack of relevance and competing priorities (i.e. promoting healthy eating environments was not considered a priority above food safety) as reasons for the lack of support towards creating supportive environments for physical activity and healthy eating [ 48 ].
Health in All Policies has been embraced in Saudi Arabia by integrating the promotion of physical activity into policy development across various areas such as education, sports and the private sector. However, while this integration is mentioned in the respective documents, challenges in implementation may have arisen due to limited cooperation between sectors. Many partnerships have been established with multiple parties in the Kingdom (MoH, MoS, MoE, and the Ministry of Municipal and Rural Affairs) to further develop physical activity policies in each sector and to remove obstacles to the implementation of physical activity programs. According to a WHO report from 2017, promoting Health in All Policies in Saudi Arabia has been identified as a national priority, monitored by the Ministry of Health [ 49 ]. Intersectoral collaboration in Oman is apparently less developed than in Saudi Arabia, hindering the integration of physical activity policies across sectors. Unlike Saudi Arabia’s comprehensive approach of Health in All Policies, Oman relies more on individual agency initiatives. However, there is potential for improvement in Oman to strengthen intersectoral collaboration and enhance the integration of physical activity policies by establishing a comprehensive policy framework and promoting coordinated efforts among sectors. For instance, the Omani government could create an intersectoral task force or committee dedicated to fostering collaboration.
In Saudi Arabia, the non-profit sector, represented by community groups, plays a significant role in promoting physical activity outside of the government framework, aided by the promotion of physical activity by influencers and celebrities through social media platforms [ 42 ]. By contrast, our study participants did not mention any significant involvement of the non-profit sector in physical activity promotion in Oman, either because these types of organizations do not play a role or because their role is not valued by the government stakeholders who we interviewed. The private sector contributes to promoting physical activity in both countries, with particularly strong partnerships with the governmental sector in Saudi Arabia. This may be because tax benefits exist for companies that encourage and promote physical activity in Saudi Arabia [ 31 ]. There are previous examples of these types of partnerships in Oman, such as a collaboration between the education and private sector aiming to create a healthier environment and lifestyle in schools. These partnerships have been achieved through the provision of financial support and sponsorships, specifically targeting sports equipment, facilities and physical activity programs in schools [ 50 ]. However, insufficient budget allocation in this area was considered a challenge, which is consistent with our study, with most participants from the sultanate expressing the need for greater financial support from the private sector.
Policy limitations differ between both countries, mainly due to the health transformation in Saudi Arabia, specifically with the Kingdom’s Vision 2030 [ 51 ]. The slow pace of transformation in various Saudi public health, infrastructure, urban planning, sports and recreation policies may impact their likelihood of being fully implemented by 2030, potentially falling short of WHO’s target for increased physical activity by that year set in their Global Action Plan on Physical Activity [ 52 ]. One of the objectives of the health sector transformation and the Quality of Life Program is to decrease the proportion of the Saudi population who are not sufficiently physically active below (67%) by 2030 [ 51 ]. The government has taken proactive measures to promote physical activity, investing in initiatives that raise awareness about its importance and the associated health benefits. This includes campaigns, public awareness programs and the establishment of recreational facilities to facilitate physical activity. In Oman, more collaboration between political parties is essential to improve the implementation of physical activity policies. According to participant 1 from the Omani MoH, several meetings were recently held with all parties to create plans to better implement physical activity policies. These meetings have led to increased monitoring and stricter enforcement by the Omani government regarding the implementation of physical activity policies in all relevant ministries. Effective health system policies significantly depend on inter-institutional collaboration. While primary health institutions play a central role, the impact of related entities is equally crucial. Educational bodies, sports organizations and community health centres contribute significantly to decision-making processes. Their closer involvement would ensure a more comprehensive approach to promoting physical activity, enhancing wellness and preventing lifestyle-related diseases [ 53 ].
According to previous studies, insufficient funding for promoting physical activity, and more broadly for NCD prevention, is a challenge in both Saudi Arabia and Oman [ 23 , 24 ]. Similarly, in a US study, state public health practitioners were interviewed about the National Physical Activity Plan, who also identified implementation costs and the complexity of physical activity policies as significant challenges [ 54 ]. Financial incentives and private sector involvement, while valuable, do not ensure adequate funding for all aspects of public health initiatives. The private sector’s contribution is often focussed on areas aligned with their business interests or corporate social responsibility. Nevertheless, in Saudi Arabia, certain private sector companies run national programs, such as the Tatweer Educational Company, a private holding dedicated to implementing the government's education reform initiative [ 55 , 56 ]. Enhancing funding for the implementation of physical activity policies in the Gulf region holds great promise in advancing infrastructure, cultivating a proficient workforce, and fostering research initiatives. On the basis of the Saudi Quality of Life document 2030 [ 46 ], the budget of the Ministries of Health, Sport and Education for promoting physical activity will likely increase until 2030.
The monitoring and evaluation of policy implementation across ministries in both countries is challenging due to the absence of comprehensive and precise data on physical activity prevalence in key sectors such as health, sport and education. Therefore, there is an urgent need to address data quality issues, such as inconsistent measurement methods employed across different sectors, which make it difficult to compare and consolidate data, and the lack of standardized protocols which undermines the accuracy and reliability of prevalence estimates. To efficiently monitor policy implementation, the responsibility could, for instance, be entrusted to the MoH for coordination with all pertinent sectors, while the General Statistics Authority should be designated to collect data on physical activity.
Responsibility for ensuring data quality rests with various stakeholders involved in the data collection, including researchers, survey administrators, data analysts and policy-makers. These stakeholders must work collectively to implement robust data collection methodologies, appropriate sampling techniques, rigorous quality control measures and transparent reporting practices. Monitoring of the impact of policies on population levels of physical activity in Saudi Arabia and Oman has improved, but more work is needed, particularly in the education sector in Oman, which requires evaluation of the progress and impact of policies.
To create and implement comprehensive policies, programs and supporting environments, a variety of sectors must collaborate in both countries. This may include transport, urban planning, media, social work, religious and cultural affairs [ 23 ]. In Saudi Arabia, one of the most effective health-promoting practices is physician-recommended physical activity [ 57 ], which is recognized as one of the eight best investments for physical activity by the International Society for Physical Activity and Health [ 58 ]. Therefore, the primary healthcare system in the Gulf region has a critical role in the promotion of physical activity, and further policy development in this area would be promising [ 57 ]. In addition, physical education policies in schools play an integral part in the Gulf states [ 59 ]. Educational institutions in Saudi Arabia and Oman are crucial in promoting physical activity among various age groups. From childhood through adolescence to young adulthood, schools offer structured physical education programs, health education and gender-inclusive activities. Universities support these efforts by providing sports facilities and activities as well as by conducting research on active lifestyles. Coordinated national policies amplify the effectiveness of these initiatives [ 22 , 39 , 60 ]. Furthermore, active transport and urban design policies have not yet been developed sufficiently to become potential contributors to population levels of physical activity in these countries due to cultural, environmental and climatic differences [ 23 ].
Strengths and limitations
Strengths of this study include a sample of high-level stakeholders who are directly involved in the formulation of physical activity policies from three ministries in Saudi Arabia and Oman, the two largest countries in the GCC. Additionally, we recruited one prominent participant from an NGO that is supervised by the Saudi Ministry of Health. We were not able to reach participants from the non-governmental sector in Oman. The original research plan was targeted at all six member countries of the Gulf Cooperation Council, but we were not able to recruit participants from the other GCC states due to political sensitivities surrounding the subject and a lack of responsiveness from relevant authorities. This may limit the generalizability of our findings beyond these specific contexts. However, we managed to recruit senior participants from the two largest GCC countries, which account for 70% of the GCC population. As in many countries, governmental representatives may have been constrained in what they reported in these interviews. Regarding the limitation of interview responses, some participants spoke about the existence of physical activity policies but did not want to provide further information on how they are being implemented, and this kind of information is not easy to obtain from other sources either. Thus, these gaps constitute a need for future research. To ensure research quality based on Lincoln and Guba’s criteria [ 61 ], we implemented several strategies. For credibility, we used investigator triangulation (involving multiple researchers) and theoretical triangulation (utilizing multiple policy frameworks). We did not use methodological triangulation, as we only conducted interviews, nor data triangulation, relying solely on audio transcripts. To ensure dependability, we kept detailed records of data collection and assessed coding accuracy and reliability among our team. For transferability, we addressed inherent challenges by collecting data from two countries, Saudi Arabia and Oman, enhancing the applicability of our findings to similar contexts. Although achieving confirmability was challenging due to our focus on individual perceptions, we aimed for transparency and objectivity in documenting our procedures and decisions.
This study fills important gaps in the analysis of physical activity policies in the Gulf region. Understanding the unique challenges, barriers and successes in promoting physical activity in the GCC countries is essential for developing relevant policies and strategies in the future. Our study highlights the importance of increasing financial support, improving collaboration between governmental agencies and between them and the private sector, and consolidating efforts to back physical activity policies and dismantle cross-sectoral barriers in Saudi Arabia and Oman. Educational institutions in Saudi Arabia and Oman play a crucial role in promoting physical activity from early childhood to young adults. Schools offer structured physical education, health education and gender-inclusive activities, while universities provide sport facilities and conduct research on active lifestyles. Coordinated national policies enhance the effectiveness of these efforts.
Specifically, we recommend allocating dedicated funds, establishing a centralized task force for coordinated policy implementation, creating incentives for private sector investment, developing a national strategy with measurable targets and conducting comprehensive policy reviews to remove bureaucratic obstacles. These steps will facilitate sustained progress and broader engagement in physical activity initiatives.
Our findings provide valuable insights and evidence for policy-makers, public health officials and other stakeholders in the region to develop targeted policies, programs and interventions that promote physical activity and prevent non-communicable diseases. The identified challenges and limitations of physical activity policies in Saudi Arabia and Oman will guide their future development.
Availability of data and materials
The datasets generated and/or analysed during the current study are not publicly available to maintain participants’ confidentiality. However, they can be obtained from the corresponding author upon reasonable request.
Abbreviations
Coronavirus disease 2019
Gulf Cooperation Council
Health in all policies
Limited liability company
Ministry of Education
Ministry of Health
Ministry of Sport
Non-communicable disease
Non-governmental organization
- Physical activity
Physical education
United Arab Emirates
United States
World Health Organization
Physical Activity Guidelines Advisory Committee. 2018 physical activity guidelines advisory committee scientific report. Washington DC: US Department of Health and Human Services; 2018.
Google Scholar
World Health Organization. WHO guidelines on physical activity and sedentary behaviour. Geneva: World Health Organization; 2020.
Dishman RK, Heath G, Schmidt MD, Lee I-M. Physical activity epidemiology. Champaign: Human Kinetics; 2022.
Strain T, Flaxman S, Guthold R, Semenova E, Cowan M, Riley LM, Bull FC, Stevens GA. National, regional, and global trends in insufficient physical activity among adults from 2000 to 2022: a pooled analysis of 507 population-based surveys with 5·7 million participants. Lancet Glob Health. 2024;12(8):e1232–43.
Article PubMed PubMed Central CAS Google Scholar
Guthold R, Stevens GA, Riley LM, Bull FC. Global trends in insufficient physical activity among adolescents: a pooled analysis of 298 population-based surveys with 1·6 million participants. Lancet Child Adolesc Health. 2020;4(1):23–35.
Article PubMed PubMed Central Google Scholar
Sallis JF, Owen N, Fisher E. Ecological models of health behavior. In: Glanz K, Rimer BK, Viswanath K, editors. Health behavior: theory, research, and practice. 5th ed. Hoboken: John Wiley and Sons; 2015.
Calvert HG, Turner L, Leider J, Piekarz-Porter E, Chriqui JF. Comprehensive policies to support comprehensive practices: physical activity in elementary schools. J Phys Act Health. 2020;17(3):313–22.
Article PubMed Google Scholar
Klepac Pogrmilovic B, Ramirez Varela A, Pratt M, Milton K, Bauman A, Biddle SJ, Pedisic Z. National physical activity and sedentary behaviour policies in 76 countries: availability, comprehensiveness, implementation, and effectiveness. Int J Behav Nutr Phys Act. 2020;17(1):1–13.
Article Google Scholar
Gelius P, Messing S, Goodwin L, Schow D, Abu-Omar K. What are effective policies for promoting physical activity? A systematic review of reviews. Prev Med Rep. 2020;18:101095.
Sallis J, Bauman A, Pratt M. Environmental and policy interventions to promote physical activity. Am J Prev Med. 1998;15(4):379–97.
Article PubMed CAS Google Scholar
Schmid TL, Pratt M, Witmer L. A framework for physical activity policy research. J Phys Act Health. 2006;3(s1):S20–9.
Bull F, Milton K, Kahlmeier S, Arlotti A, Juričan AB, Belander O, Martin B, Martin-Diener E, Marques A, Mota J. Turning the tide: national policy approaches to increasing physical activity in seven European countries. Br J Sports Med. 2015;49(11):749–56.
Volf K, Kelly L, García Bengoechea E, Casey B, Gobis A, Lakerveld J, Zukowska J, Gelius P, Messing S, Forberger S. Policy Evaluation Network (PEN): protocol for systematic literature reviews examining the evidence for impact of policies on physical activity across seven different policy domains. HRB Open Res. 2022;3:62.
Volf K, Kelly L, García Bengoechea E, Casey B, Gelius P, Messing S, Forberger S, Lakerveld J, Den Braver NR, Zukowska J. Evidence of the impact of sport policies on physical activity and sport participation: a systematic mixed studies review. Int J Sport Pol Polit. 2022;14(4):697–712.
Abarca-Gómez L, Abdeen ZA, Hamid ZA, Abu-Rmeileh NM, Acosta-Cazares B, Acuin C, Adams RJ, Aekplakorn W, Afsana K, Aguilar-Salinas CA. Worldwide trends in body-mass index, underweight, overweight, and obesity from 1975 to 2016: a pooled analysis of 2416 population-based measurement studies in 128·9 million children, adolescents, and adults. Lancet. 2017;390(10113):2627–42.
World Health Organization. Global status report on physical activity. Geneva: World Health Organization; 2022.
Al-Hazzaa HM. Physical inactivity in Saudi Arabia revisited: a systematic review of inactivity prevalence and perceived barriers to active living. Int J Health Sci. 2018;12(6):50.
Al Siyabi H, Mabry RM, Al Siyabi A, Al Subhi M, Milton K. The development of a national policy framework for physical activity in Oman. J Phys Act Health. 2021;18(12):1471–2.
World Health Organization. Promoting physical activity in the Eastern Mediterranean Region through a life-course approach. Geneva: World Health Organization; 2014.
Klepac Pogrmilovic B, O’Sullivan G, Milton K, Biddle SJ, Bauman A, Bull F, Kahlmeier S, Pratt M, Pedisic Z. A global systematic scoping review of studies analysing indicators, development, and content of national-level physical activity and sedentary behaviour policies. Int J Behav Nutr Phys Act. 2018;15(1):1–17.
Albujulaya N, Stevinson C, Piggin J. Physical activity policy in Saudi Arabia: analysis of progress and challenges. Int J Sport Pol Polit. 2023. https://doi.org/10.1080/19406940.2023.2228812 .
Al-Hazzaa HM, AlMarzooqi MA. Descriptive analysis of physical activity initiatives for health promotion in Saudi Arabia. Front Public Health. 2018;6:329.
Al-Hazzaa HM. Physical activity research in the Gulf Cooperation Council Countries: progress made but work still to do. J Phys Act Health. 2022;19(11):769–70.
Al Siyabi H, Mabry RM, Al Siyabi A, Milton K. A critique of national physical activity policy in Oman using 3 established policy frameworks. J Phys Act Health. 2021;18(12):1473–8.
Denzin NK, Lincoln YS. The Sage handbook of qualitative research. 5th ed. Los Angeles: Sage; 2018.
The World Bank: Data for Saudi Arabia and Oman. https://data.worldbank.org/?locations=SA-OM . Accessed 5 Jan 2024.
Saunders B, Sim J, Kingstone T, Baker S, Waterfield J, Bartlam B, Burroughs H, Jinks C. Saturation in qualitative research: exploring its conceptualization and operationalization. Qual Quant. 2018;52(4):1893–907.
Bellew B, Bauman A, Bull FC, Schoeppe S. The rise and fall of Australian physical activity policy 1996–2006: a national review framed in an international context. Aust N Z Health Policy. 2008. https://doi.org/10.1186/1743-8462-5-18 .
Klepac Pogrmilovic B, O’Sullivan G, Milton K, Biddle SJH, Bauman A, Bellew W, Cavill N, Kahlmeier S, Kelly MP, Mutrie N, et al. The development of the Comprehensive Analysis of Policy on Physical Activity (CAPPA) framework. Int J Behav Nutr Phys Act. 2019;16(1):60.
Omani Ministry of Health: The national policy for prevention and control of NCDs. https://extranet.who.int/nutrition/gina/sites/default/filesstore/OMN_2016_%20%D8%A7%D9%84%D8%AE%D8%B7%D8%A9%20%D8%A7%D9%84%D9%88%D8%B7%D9%86%D9%8A%D8%A9%20%D9%84%D9%84%D9%88%D9%82%D8%A7%D9%8A%D8%A9%20%D9%85%D9%86%20%D8%A7%D9%84%D8%A7%D9%85%D8%B1%D8%A7%D8%B6%20%D8%A7%D9%84%D9%85%D8%B2%D9%85%D9%86%D8%A9%20%D8%BA%D9%8A%D8%B1%20%D8%A7%D9%84%D9%85%D8%B9%D8%AF%D9%8A%D8%A9%20%D9%88%D9%85%D9%83%D8%A7%D9%81%D8%AD%D8%AA%D9%87%D8%A7_1.pdf . Accessed 12 Dec 2023.
Saudi Vision 2030: Quality of life program. https://www.vision2030.gov.sa/v2030/vrps/qol/ . Accessed 25 Dec 2023.
Kingdom of Saudi Arabia: National transformation program. https://vision2030.gov.sa/sites/default/files/NTP_En.pdf . Accessed 2 Jan 2024.
University Sports Federation: University sports federation strategy. https://m.susfweb.com/publication/f-a4f22d74-b999-465c-9cd0-2a89895555a8.pdf . Accessed 17 Nov 2023.
Ministry of Health 2015: The National Diet and Physical Activity Strategy (DPAS) 2015–2025. https://extranet.who.int/nutrition/gina/sites/default/filesstore/SAU_2015_%D8%A7%D9%84%D8%A7%D8%B3%D8%AA%D8%B1%D8%A7%D8%AA%D9%8A%D8%AC%D9%8A%D8%A9%20%D8%A7%D9%84%D9%88%D8%B7%D9%86%D9%8A%D8%A9%20%D9%84%D9%84%D8%BA%D8%B0%D8%A7%D8%A1%20%D8%A7%D9%84%D8%B5%D8%AD%D9%8A%20%D8%A9%D8%A7%D9%84%D9%86%D8%B4%D8%A7%D8%B7%20%D8%A7%D9%84%D8%A8%D8%AF%D9%86%D9%8A.pdf . Accessed 23 Dec 2023.
Saudi Ministry of Health: Physical activity guidelines for health practitioners. https://www.moh.gov.sa/Ministry/About/Health%20Policies/037.pdf . Accessed 2 Nov 2023.
Public Health Authority: 24-hour movement guidance Saudi Arabia. https://covid19.cdc.gov.sa/wp-content/uploads/2021/10/24-hrs-ar.pdf . Accessed 4 Jan 2024.
Saudi Sports for All Federation: Annual report. https://sportsforall.com.sa/wp-content/uploads/2021/03/SFA-Annual-Report-2020-EN-V2-4.pdf . Accessed 24 Oct 2023.
Oman Vision 2040: Vision Document. https://www.oman2040.om/assets/books/oman2040-en/index.html#p=51 . Accessed 10 Sept 2023.
Omani Ministry of Education: The physical education standards document. https://ict.moe.gov.om/omcust/PDF/Sport1_9_Oman.pdf . Accessed 26 Dec 2023.
Oman Olympic Committee: Regulations. https://ooc.om/wp-content/uploads/2021/08/RulesAndRegulationsArabic.pdf . Accessed 7 Jan 2024.
Saudi Ministry of Health: Exploratory examination for students. https://www.my.gov.sa/downloads/manuals/87d1f31c-1c7a-41a2-8a89-650a718a4a9d.pdf . Accessed 2 Dec 2023.
Saudi Health Promotion Centre 2021: HPC. https://saudihpc.com/ . Accessed 21 Sept 2023.
Chowdhury S, Mok D, Leenen L. Transformation of health care and the new model of care in Saudi Arabia: Kingdom’s Vision 2030. J Med Life. 2021;14(3):347–54.
Alqahtani BA, Alenazi AM, Alhowimel AS, Elnaggar RK. The descriptive pattern of physical activity in Saudi Arabia: analysis of national survey data. Int Health. 2021;13(3):232–9.
Omani Ministry of Health: National nutrition strategy. https://extranet.who.int/nutrition/gina/sites/default/filesstore/OMN%202014%20National%20Nutrition%20Startegy.pdf . Accessed 8 Jan 2024.
Quality of Life Program 2020: Vision 2030. https://planipolis.iiep.unesco.org/sites/default/files/ressources/saudi_arabia_quality_of_life.pdf . Accessed 27 Nov 2023.
Evenson KR, Alhusseini N, Moore CC, Hamza MM, Al-Qunaibet A, Rakic S, Alsukait RF, Herbst CH, AlAhmed R, Al-Hazzaa HM. Scoping review of population-based physical activity and sedentary behavior in Saudi Arabia. J Phys Act Health. 2023;20(6):471–86.
Allender S, Gleeson E, Crammond B, Sacks G, Lawrence M, Peeters A, Loff B, Swinburn B. Policy change to create supportive environments for physical activity and healthy eating: which options are the most realistic for local government? Health Promot Int. 2012;27(2):261–74.
World Health Organization: Country cooperation strategy at glance. https://apps.who.int/iris/bitstream/handle/10665/136842/ccsbrief_sau_en.pdf?sequence=1 . Accessed 18 Oct 2023.
World Health Organization. Health-promoting schools initiative in Oman: a WHO case study in intersectoral action. Cairo: Regional Office for the Eastern Mediterranean; 2013.
Government of Saudi Arabia: Vision 2030. https://www.vision2030.gov.sa/media/rc0b5oy1/saudi_vision203.pdf . Accessed 9 Jan 2024.
World Health Organization: Global action plan on physical activity 2018–2030. https://apps.who.int/iris/bitstream/handle/10665/272722/9789241514187-eng.pdf . Accessed 11 Nov 2023.
Aunger JA, Millar R, Greenhalgh J, Mannion R, Rafferty A-M, McLeod H. Why do some inter-organisational collaborations in healthcare work when others do not? A realist review. Syst Rev. 2021;10(1):82.
Evenson KR, Satinsky SB, Valko C, Gustat J, Healy I, Litt JS, Hooker SP, Reed HL, Tompkins NOH. In-depth interviews with state public health practitioners on the United States National Physical Activity Plan. Int J Behav Nutr Phys Act. 2013;10(1):1–9.
Al-Otaibi HH. Measuring stages of change, perceived barriers and self efficacy for physical activity in Saudi Arabia. Asian Pac J Cancer Prev. 2013;14(2):1009–16.
Abdel-Salam D, Abdel-Khalek E. Pattern and barriers of physical activity among medical students of Al-Jouf University, Saudi Arabia. J High Inst Public Health. 2016;46(2):41–8.
Alahmed Z, Lobelo F. Physical activity promotion in Saudi Arabia: a critical role for clinicians and the health care system. J Epidemiol Global Health. 2018;7:S7–15.
International Society for Physical Activity and Health (ISPAH): ISPAH’s eight investments that work for physical activity. www.ISPAH.org/Resources . Accessed 2 Oct 2023.
Alhumaid MM, Khoo S, Bastos T. The effect of an adapted physical activity intervention program on pre-service physical education teachers’ self-efficacy towards inclusion in Saudi Arabia. Sustainability. 2021;13(6):3459.
Alghannam AF, Malkin JD, Al-Hazzaa HM, AlAhmed R, Evenson KR, Rakic S, Alsukait R, Herbst CH, Alqahtani SA, Finkelstein EA. Public policies to increase physical activity and reduce sedentary behavior: a narrative synthesis of “reviews of reviews.” Glob Health Action. 2023;16(1):2194715.
Lincoln YS, Guba EG. But is it rigorous? Trustworthiness and authenticity in naturalistic evaluation. New Dir Eval. 1986;1986(30):73–84.
Download references
Acknowledgements
We would like to express our sincere thanks to the policy-makers in the Kingdom of Saudi Arabia and Oman for their participation in this research.
This project is kindly supported through a PhD scholarship from King Faisal Medical City in Saudi Arabia and PhD funding from the Faculty of Health of the University of Technology Sydney.
Author information
Authors and affiliations.
School of Public Health, Faculty of Health, University of Technology Sydney, City Campus, Ultimo, NSW, 2007, Australia
Ali Ahmed Alzahrani & Klaus Gebel
Faculty of Social and Political Sciences, Institute of Sport Sciences, Quartier UNIL-Centre, Bâtiment Synathlon, CH-1015, Lausanne, Switzerland
Peter Gelius
Prevention Research Collaboration, Sydney School of Public Health, University of Sydney, Sydney, NSW, Australia
Adrian E. Bauman
You can also search for this author in PubMed Google Scholar
Contributions
A.A. recruited participants and conducted interviews with policy-makers. A.A., A.B. and P.G. collectively analysed the transcripts. K.G., A.B. and P.G. made significant contributions to the thorough review and editing of the manuscript.
Corresponding author
Correspondence to Klaus Gebel .
Ethics declarations
Ethics approval and consent to participate, competing interests.
The authors declare that they have no competing interests.
Additional information
Publisher’s note.
Springer Nature remains neutral with regard to jurisdictional claims in published maps and institutional affiliations.
Supplementary Information
Supplementary material 1., supplementary material 2., rights and permissions.
Open Access This article is licensed under a Creative Commons Attribution-NonCommercial-NoDerivatives 4.0 International License, which permits any non-commercial use, sharing, distribution and reproduction in any medium or format, as long as you give appropriate credit to the original author(s) and the source, provide a link to the Creative Commons licence, and indicate if you modified the licensed material. You do not have permission under this licence to share adapted material derived from this article or parts of it. The images or other third party material in this article are included in the article’s Creative Commons licence, unless indicated otherwise in a credit line to the material. If material is not included in the article’s Creative Commons licence and your intended use is not permitted by statutory regulation or exceeds the permitted use, you will need to obtain permission directly from the copyright holder. To view a copy of this licence, visit http://creativecommons.org/licenses/by-nc-nd/4.0/ .
Reprints and permissions
About this article
Cite this article.
Alzahrani, A.A., Gelius, P., Bauman, A.E. et al. Physical activity policies in Saudi Arabia and Oman: a qualitative study using stakeholder interviews. Health Res Policy Sys 22 , 111 (2024). https://doi.org/10.1186/s12961-024-01192-w
Download citation
Received : 17 January 2024
Accepted : 20 July 2024
Published : 19 August 2024
DOI : https://doi.org/10.1186/s12961-024-01192-w
Share this article
Anyone you share the following link with will be able to read this content:
Sorry, a shareable link is not currently available for this article.
Provided by the Springer Nature SharedIt content-sharing initiative
- Health policy
- Gulf region
Health Research Policy and Systems
ISSN: 1478-4505
- Submission enquiries: Access here and click Contact Us
- General enquiries: [email protected]
Log in using your username and password
- Search More Search for this keyword Advanced search
- Latest content
- BMJ NPH Collections
- BMJ Journals
You are here
- Online First
- COVID-19’s impact on food environment in the Indian states of Telangana, Maharashtra, West Bengal, Tamil Nadu and Punjab: a descriptive qualitative study to build further research in India’s food environment resilience building
- Article Text
- Article info
- Citation Tools
- Rapid Responses
- Article metrics

- http://orcid.org/0000-0001-8041-9968 Jørgen Torgerstuen Johnsen 1 , 2 ,
- Marjorie Rafaela Lima do Vale 1 ,
- Rekha Bhangaonkar 3 ,
- http://orcid.org/0009-0005-4014-2518 Wanja Nyaga 1 ,
- Sally Ayyad 1 and
- http://orcid.org/0000-0003-3295-168X Sumantra Ray 1 , 2 , 4
- 1 NNEdPro Global Institute for Food Nutrition and Health , Cambridge , UK
- 2 School of Biomedical Sciences , Ulster University , Coleraine , UK
- 3 Department of Land Economy , University of Cambridge , Cambridge , UK
- 4 School of the Humanities and Social Sciences, University of Cambridge , Cambridge , UK
- Correspondence to Jørgen Torgerstuen Johnsen; j.johnsen{at}nnedpro.org.uk ; Professor Sumantra Ray; s.ray{at}nnedpro.org.uk
Background and aim Globally, COVID-19 has had a profound impact on food and nutrition security. This paper aims to gather the perspective from Transforming India’s Green Revolution by Research and Empowerment for Sustainable food Supplies (TIGR2ESS) Flagship Project 6 (FP-6) team on the impact of COVID-19 on the food systems in India. The responses collected will be used for further research projects after TIGR2ESS ends in March 2022.
Method Members of the TIGR2ESS FP-6 team in India were invited to complete an online open-ended questionnaire with 21 questions exploring the impact of the COVID-19 pandemic on food systems and environments in India. The questionnaire and data analysis were guided by the food environment framework developed by Turner et al and the adaptations proposed by the United Nations System Standing Committee on Nutrition. Discussions and organisation of codes under the respective themes and subthemes were held online using the virtual platform Miro. 35 individual codes and 65 subcodes were agreed on. Responses were collated and analysed using the template with support from NVivo software and synthesised the relevant themes under Turner et al ’s framework.
Results The organisation representatives from TIGR2ESS FP-6 (n=16) captured the perceived impact of the COVID-19 on food systems and the environment from the Indian states of Maharashtra, Punjab, Tamil Nadu, Telangana and West Bengal. Negative disruptions were caused by the COVID-19 restrictions across all the themes affecting food actors and consumers. Myths and misconception on dietary intake were reported across the state affecting especially the consumption of poultry. Positive aspects such as home cooking and awareness around healthy food emerged.
Conclusion Potential research areas were identified and involve the effects of supply chain resilience buidling, farmers selling their produce directly to consumer and the revival of local and traditional food’s impact on diets, understanding the harm for consumers by implementing restrictions, how indigenous and local food may impact peoples’ diets, how to build on the encouragement of healthy home cooking during the pandemic, investigate the negative and positive effects of digital environments during the pandemic and dispelling myths and misconception while advocating for healthy diets.
- Malnutrition
Data availability statement
Data are available on reasonable request. Please contact the main author to request the data material.
This is an open access article distributed in accordance with the Creative Commons Attribution Non Commercial (CC BY-NC 4.0) license, which permits others to distribute, remix, adapt, build upon this work non-commercially, and license their derivative works on different terms, provided the original work is properly cited, appropriate credit is given, any changes made indicated, and the use is non-commercial. See: http://creativecommons.org/licenses/by-nc/4.0/ .
https://doi.org/10.1136/bmjnph-2023-000844
Statistics from Altmetric.com
Request permissions.
If you wish to reuse any or all of this article please use the link below which will take you to the Copyright Clearance Center’s RightsLink service. You will be able to get a quick price and instant permission to reuse the content in many different ways.
WHAT IS ALREADY KNOWN ON THIS TOPIC
COVID-19 and its measures to contain its spread have profoundly impacted food and nutrition security worldwide.
WHAT THIS STUDY ADDS
Key insights and break down of COVID-19 impact on food system mechanisms in the Indian states of Telangana, Maharashtra, West Begal, Tamil Nadu and Punjab.
HOW THIS STUDY MIGHT AFFECT RESEARCH, PRACTICE OR POLICY
Future potential action-research areas were identified within supply chain resilience, interstate collaboration for emergency response, price stabilisation strategies, market dynamics, and consumer engagement for future food systems resilience building.
This study provides key insights into the similarities and differences in the impact of major shocks to food systems across five diverse Indian states spanning different ago-ecological zones.
The global spread of COVID-19 since early 2020 has profoundly impacted food and nutrition security. 1 Measures to contain its spread led to disruptions in food systems worldwide, affecting various stages from production to distribution. This affected the agricultural workforce, packers, farmers and vendors, with many having to close or limit their operations. Additionally, global trade disruptions, physical distancing and restrictions in grocery stores resulted in consequences like empty shelves, panic buying and long queues. 2–5 The COVID-19 preventive policies on suspending important social protection programmes were profound and disproportionately affected the poor and marginalised regions and communities. 1 6
In India, COVID-19 restrictions disrupted agricultural production and supply. 7 Strict mobility limits led to labour shortages, impacting production and food distribution. 8 Many agricultural workers were compelled to return to their home villages in some states, intensifying strains on local food systems. 9 The discontinuity in transportation led to dumping of food produce with an estimated 10%–20% drop in fruit and vegetable availability. 7 Reduced market hours have reportedly further exacerbated food waste. Misinformation, largely from social media, claiming that poultry carried COVID-19 was reported to be widespread by the media. 10 The restrictions also impacted the economic activity of consumers 2 considering a widespread loss of jobs and incomes, 11 especially for informal workers, the poor, 2 12 women 13 and smallholder farmers, which constitute around 86% of India’s agricultural sector. 14 Changes in food prices further restricted food affordability for consumers. Changes in food prices hindered affordability, with declines for some crops but increases for items like lentils, vegetables, fish and meat. 2 7 There were reports of consumers prioritising essential products followed by a focus on healthy products. 11 The Indian government responded to the COVID-19 pandemic with relief packages, including food and cash transfers. 2
It is essential for countries to monitor the situation closely, gather lessons learnt and data to avert the worst outcomes with respect to food security and malnutrition and protect the human right to food. The former United Nations System Standing Committee on Nutrition (UNSCN), now United Nations (UN) Nutrition, argued that there was a need to understand better the impact of the COVID-19 pandemic on both external and personal domains of food environments. The external domain includes aspects related to food availability, prices and vendors—while the personal dimension includes geographical access, affordability, convenience and desirability. 15
Using the UN Nutrition framework, this paper aims to gather the perspective from Transforming India’s Green Revolution by Research and Empowerment for Sustainable food Supplies (TIGR2ESS) Flagship Project 6 (FP-6) team on the impact of the COVID-19 on the food systems in India. The responses collected will be used for further research projects after TIGR2ESS ends in March 2022.
An open-ended structured online survey was conducted to gather information on the perception of relevant stakeholders on the impact of the COVID-19 pandemic on food systems and environments in their respective Indian states. The data were collected once by the respective organisations between May and September 2020 using Qualtrics, an online survey software hosted by the University of Cambridge. The survey was designed to capture the current situation in the respective states.
Sample of key stakeholders
Relevant information on the potential impact of COVID-19 on food systems and environments was collected through individuals engaged in the TIGR2ESS project. TIGR2ESS is organised around six FPs. The FP-6: Impacting Well-being in Rural and Urban Communities consists of a multidisciplinary group of social scientists, land economists, basic scientists and a nutrition think tank, 16 thus hosting vast knowledge of Indian food systems and environments. Table 1 presents more detailed characteristics of TIGR2ESS and its FP-6 study participant organisations.
- View inline
Description of participant organisations from TIGR2ESS FP-6
Food environments in the COVID-19 pandemic framework
The research team designed a 21-question open-ended questionnaire to explore the potential disruption in food systems and environments in the TIGR2ESS FP-6 operated states and regions (see online supplemental material 1 for more details). The open-ended structured questionnaire was informed by the food environment framework developed by Turner et al 17 and the adaptations proposed by the UNSCN to describe potential COVID-19’s impact on food systems and environments. 15 This framework divides the food environment into external and personal domains. The external domain considers aspects such as availability, food prices, vendors, markets and regulation while the personal domain considers affordability, accessibility, convenience and desirability. The questions enclosed the potential impact of COVID-19 on food production, availability, access and waste in the respective organisation’s region/state. The responses could be informed by existing published materials or personal experiences of the respective team. The questions enclosed the background information about the members of the respective team and COVID-19 impact on food production, food availability, food access and consumer behaviours. The questionnaire was reviewed by volunteers from the NNEdPro virtual core, consisting of over 70 individuals globally with scientific experiences across the nutrition field.
Supplemental material
Data collection.
Initial discussions to participate were held with all FP-6 stakeholders. Five organisations agreed to partake in the study and formed respective teams to answer the questionnaire. The following organisations participated: the International Crops Research Institute for the Semi-Arid Tropics (ICRISAT) (Telangana) (n=5), Indian Institute of Technology (IIT-Bombay) (Maharashtra) (n=3), MS Swaminathan Research Foundation (Tamil Nadu) (n=3), NNEdPro-Kolkata office (West Bengal) (n=3) and Panjab University (Punjab) (n=2) (see table 1 ). Team leaders within the respective organisations were asked to compile responses from their respective teams, which could be achieved by holding a workshop, interviews or capturing the perceptions of all its members. While IIT-Bombay, MMSRF, NNEdPro-Kolkata office and Panjab University relied on published materials and personal experiences of their respective teams, the ICRISAT team chose to undertake a telephonic survey to understand the different perspectives of the COVID-19 crisis. They used a translated version of the questionnaire and phoned 40 randomly selected households between July and August 2020 in the urban, periurban, tribal and rural locations of Telangana. The households were recruited as respondents from previous surveys and 10 households from urban, periurban, tribal and rural location, respectively, within Telangana were randomly selected. Two women and 38 men responded to the telephone survey. ICRISAT then synthesised the responses among the team and summarised the respective answers for the authors to analyse.
Data analysis
Data were analysed using template analysis. 18 All five transcripts provided by the team leaders of the respective organisations were initially coded by one researcher (RB) using a deductive approach with predefined codes based on the framework developed by Turner et al . 17 Another researcher (MRLdV) used an inductive approach introducing further codes. The two (RB and MRLdV) researchers met with the lead author (JTJ) to compare the codes and agree on the codes that should be applied to subsequent transcripts. Discussions and organisation of codes under the respective themes (availability and consumer accessibility; food prices and consumer affordability; vendors, markets, regulations and consumer convenience; and consumer desirability) were held online using the virtual platform Miro. 19 35 individual codes and 64 subcodes were agreed on. Various examples of the codes were product shelf-life, food waste, food ration, online shopping/delivery services and gardening behaviour while examples of subcodes were fast food, waiting time, production and trade restrictions (see online supplemental material 2 for more details). The agreed template was then applied to all transcripts by three researchers (MRLdV, WN and SA) using NVivo and further verified by the lead author (JTJ). The template created was used to descriptively summarise the information provided by participants. The senior researcher (SR) discussed the summaries and way forward with the lead author (JTJ). The present the results, the researchers decided to synthesise the relevant themes under the Turner et al 17 framework.
The perceived impact of COVID-19 on food systems and environments in India was captured and presented by the following categories under Turner et al 17 framework: availability and consumer accessibility; food prices and consumer affordability; vendors, markets, regulations and consumer convenience and consumer desirability. Negative disruptions were caused by the COVID-19 restrictions across all the themes affecting food actors and consumers. Myths and misconceptions on dietary intake were reported across the states, affecting especially the consumption of poultry. However, some positive aspects emerged, such as home cooking and awareness of healthy food. The representation from TIGR2ESS FP-6 participants (n=16) captured perspectives from the states of Maharashtra, Punjab, Tamil Nadu, Telangana and West Bengal. The respective organisation quotations were given individual numbers at random to keep the anonymity of the participants.
Availability and consumer accessibility
During the COVID-19 lockdown, the organisations noted a marked shortage of fresh produce, especially vegetables, poultry and fish. Despite this, local vendors managed to maintain limited supplies in certain areas. The agricultural sector faced labour shortages, leading to crops standing unharvested. Transportation breakdowns inflated costs and hindered market access. Early on, panic buying led to shortages of staple foods at the beginning of the COVID-19 implemented restrictions.
For consumers, fish and fruits were hardest hit, with limited access to vegetables, animal products and pulses. While high-income groups leaned towards fresh produce, the less affluent turned to cereal-based diets. One organisation also reported consumers had limited access to markets and an increased consumption of local and indigenous products. Efforts to address food scarcity included local kitchens and government-supported meal schemes, but manpower constraints due to movement restrictions were a major bottleneck in implementation and posed challenges.
Social protection programmes (such as the mid-day meal programme and community kitchens) initially struggled but quickly adapted, providing dry rations and doorstep delivery of mid-day meals. Financial aid, fuel support and free food kits were established. Youth club volunteers and institutions were crucial in providing for vulnerable populations. Essential items were distributed through the public distribution system. These combined efforts, though facing initial challenges, played a vital role in alleviating food scarcity during the pandemic.
Free food kit (rice, oil, sugar and pulses) was distributed to the BPL (below the poverty line) household through ration card along with Rs.1000 for other necessities. In many places, local formal and informal institutions including panchayat, youth clubs and other volunteers join together to meet the food needs of older people as well as the people who reside at the roadside. Organisation 4
The organisations described curfew affecting individuals’ accessibility to markets by restricting mobility to access diverse foods. These restrictions were reported to influence diets across socioeconomic categories differently, yet one of the organisations reported no apparent changes in individuals’ dietary intake.
Digital food ordering increased among high-income groups, and the pandemic encouraged more people to shop online. Reportedly, only a small section of society procured food through mobile-based applications.
… the pandemic is causing more people to shop for groceries online. The demand for local products, digital commerce and omnichannel services such a home delivery, chat features and virtual consultations is surging, and is likely to endure beyond this crisis. Organisation 2
Food prices and consumers’ affordability
Participants universally reported increased food prices nationwide, particularly for vegetables, fruits, greens, meat and seafood. In some states, there was an initial surge in vegetable and fruit prices at the start of the pandemic, followed by a return to prepandemic levels. Interestingly, despite farmers selling their products at lower rates, consumers often paid higher prices at the point of purchase. Price disparities were also observed based on the place of purchase, with wholesalers offering lower prices than retailers.
The uptick in food prices coupled with reduced income and earning opportunities had varying impacts on different socioeconomic groups. Those reliant on the informal sector were hit hardest, experiencing job loss and significant income reduction, pushing them into debt and food insecurity. Consumers generally bought smaller quantities and shifted towards predominantly consuming cereals and coarse grains. Notably, the rise in food prices, along with limited food accessibility, prompted a resurgence in locally produced and traditional foods.
Vendors, markets, regulations and consumer convenience
The organisations detailed how shortages of fruits and vegetables, stemming from harvest and supply chain restrictions, significantly impacted vendors and markets. With road and rail closures due to movement restrictions, producers faced challenges in reaching markets. Interstate transportation disruptions particularly affected vendors reliant on supplies from other states, affecting a range of products, including vegetables, fruits, poultry and marine items, leading to losses for poultry and milk farmers.
As it was the harvesting period, there were delays in harvesting due to shortage of labour and transportation to market. Also, the traders who were involved in the value chain were missed out during the crisis. Farmers fetched low prices for their grain crops. In case of perishables (fruits and vegetables) they incurred heavy losses as they couldn't find proper markets to sell their produce. There were serious implications on the export market as the fresh products such as fruits and sea food could not timely have marketed due to COVID restrictions. Organisation 1.
Food shortages and high prices, alongside movement restrictions, resulted in reduced customer turnout, prompting vendors and markets to shorten business hours or close altogether. Some organisations reported the emergence of small farmer groups directly trading with consumers. Grocers and supermarkets implemented purchasing limits on select products.
All organisations noted a surge in food loss and waste as closures left vendors unable to sell perishable items, resulting in significant losses of crops, poultry, meat and seafood. Two of the organisations reported losses of poultry, meat and seafood, which had to be thrown.
Vegetables, fruits, and greens diversity as well as animal and seafood had decreased hugely, which mainly due to lack of financial capability among middle/low-income/BPL populations, thereby reducing the demand for these produces overall. Along with this, the factor of availability and price also matters a lot here. Organisation 3.
Consumer experiences were marked by long queues, especially at local supermarkets, necessitating multiple visits for a single item. However, those shopping at local groceries or neighbourhood shops encountered shorter queues.
Most organisations reported an uptick in home cooking due to food outlet closures. While there was a positive shift towards healthier options like nuts, salads and fruits, some states observed an increased burden on women for household cooking responsibilities. A positive trend emerged with family members sharing cooking duties. One organisation noted an emphasis on more hygienic food preparation methods, including vegetable washing in salt or turmeric solution before cooking and storing.
Consumer desirability
The organisations noted a surge in demand for local produce, seasonal items and hot meals in their respective states. Two organisations mentioned a shift away from ready-made and fast foods, with a reluctance towards roadside and mobile kitchens. One organisation highlighted increased efforts to bolster local food systems, leading to a rise in terrace and home gardening. Another organisation reported increased consumption of fruits, vegetables, poultry, eggs and spices among urban consumers, as they perceived them as protective foods. The consumers preferred locally produced produce in these cases.
More of poultry and meat were consumed as protein-rich foods were advocated through the social media, and newspapers by the local government. There was an increase in the consumption of fruits and vegetables and also spices. Outside food was completely avoided. Organisation 1
Consumers were observed to prefer locally produced fruits, vegetables, poultry, eggs and spices, perceiving them as protective foods. However, myths and misconceptions play a role in purchasing decisions. For instance, misinformation about COVID-19 spreading through poultry significantly impacted consumption in one state, while in another, consumers believed poultry, meat and eggs to boost immunity.
Further misconceptions included a belief that non-vegetarian foods lowered immunity and increased susceptibility to COVID-19. Some consumers viewed ginger, garlic, turmeric and fruits as immunity boosters. Additionally, there was an increase in the consumption of warm water, lemon, spices, herbal teas and citrus fruits. Boiling and cooling portable water was considered necessary to ensure sterilisation from the COVID-19 virus.
In this study, five organisations across five state in India reported profound COVID-19 disruption in the food systems in their respective states in four ways: (1) disruption in market-open hours and access to vendors and markets, (2) reduction in consumer affordability due to increased food prices, (3) the constraints on availability and consumer accessibility of food and (4) change in consumer desirability. In this section, we will discuss the effects of each theme and consider further research which can be done by the respective organisations post-TIGR2ESS FP-6. A clear cascade effect from food actors influencing consumers and vice versa could be seen in the results as many statements overlap the respective themes.
The observations in this study reinforce previous assumptions that vendors and markets were heavily affected by regulations, resulting in disruption in food supply chains. Movement restrictions between states particularly affected vendors relying on produce from other states. This dependency on interstate trade and centralised production poses challenges for future food system resilience. 20–23 Exploring strategies to mitigate such dependencies is crucial for ensuring access to essential and nutritious produce during emergencies. Further research in this area is imperative for building robust systems resilient to future disruptions.
Access restrictions due to COVID-19 resulted in long queues for the consumers, a phenomenon observed globally. 1 Interestingly, participants noted instances where consumers turned to local shops, experiencing shorter wait times. A study from Italy also highlighted a similar shift towards local and local agri-food. 24 A seeming natural shift due to the restrictions in market access was described as smallholder farmers self-organised to trade directly with consumers. This shift prompted smallholder farmers to trade independently with consumers. Future investigations on the factors behind varying customer traffic in different shops could provide insights for supporting short-chain suppliers and promoting decentralised food access.
While studies have shown a shift during COVID-19 in consumer waste patterns leading to a reduction of food waste at the household level, 25 one concern raised in our study was the waste generated by marketplaces that were unable to sell their perishable products. This points to the lack of measures in place to effectively use perishable products and should be brought to future attention in future resilience building. Further research and mitigations can be built on existing studies and guidance like the six priority areas for action report by the Global Panel on Agriculture and Food Systems for Nutrition. 26
Understanding the health implications, particularly among socioeconomic groups, requires further exploration. The reported shift towards less diverse, cereal-dominant diets, rather than nutritionally balanced options is of concern. A study from Gujarat and Maharashtra, India, also observed a similar trend towards more unhealthy food choices. 27 While many factors are in play for this change, one organisation notably reported an initial increase in fruit and vegetable prices at the onset of the pandemic, which later returned to prepandemic levels. Another study from the early stages of the pandemic noted rising vegetable prices but highlighted a reduction in poultry and egg prices due to misinformation surrounding COVID-19 and these food groups, 28 of which could serve as important food groups against malnutrition.
Our study acknowledges the increased reliance on food aid yet does not delve into its operational details or impact on dietary patterns. This indicates a need for further research into the role of food banks and distribution centres in promoting and sustaining a healthy diet within the Indian context. One avenue to explore is the development of evidence-based nutrition standards for food distribution, 29 applicable at both national and local levels. The approach outlined in the study by Macaninch et al 30 could serve as a valuable guide for conducting such assessments. Additionally, a crucial preventative measure lies in school/mid-day meal programmes. Social programmes like school/mid-day meals play a vital role for vulnerable populations, particularly during a pandemic. Another study clearly demonstrates the negative impact of these disruptions on decades of efforts to combat malnutrition. 31
Consumers reportedly shifted to online shopping, and a small section of the society used mobile-based applications to order food. However, this was mainly observed in high-income groups in our findings. This is not unique to India as multiple observations have been reported elsewhere, 32 especially for food delivery from restaurants. 33 There is a need for a better understanding of this shift in diets for Indians and to prevent unhealthy food marketing. For example, the shift to digital environments promoted unhealthy food consumption in Brazil. 34 Digital food environment can positively and negatively impact peoples’ diets, 35 especially for children and adolescents. 36 Research on the diet impact of increased utilisation of the digital food environment is needed in an Indian context.
Interestingly, the organisations in this study described some positive trends in consumer desirability that occurred and should be further explored. The increase in home cooking, with proper knowledge and ingredients, can positively impact peoples’ diets. 37 38 However, as pointed out in this study, there is a risk of shifting these types of household responsibilities to women, burdening them with more responsibilities. Proper education and encouragement to share these responsibilities are important to lessen further burdens on women during emergencies.
The findings underscore how myths and misconceptions can significantly impact perceptions of certain food products. Items believed to be linked to diseases, like poultry, tend to suffer from negative consumer sentiment. This not only affects the income of vendors and farmers but also leads to a reduction in the consumption of nutritious foods, especially among groups that rely on them. The sudden exclusion of certain food groups can result in a drop in essential nutrients in people’s diets. Interestingly, there was an uptick in vegetable consumption as they were believed to boost the immune system. However, health-promoting products like herbal teas and ginger can be wrongly marketed as preventive or curative foods for diseases. Myth-busting is an essential preventative measure. The Indian Government, media and the WHO promptly took action to debunk such myths, which either affected the sales of nutritious produce or led to unnecessary product sales. 10 39 However, a more in-depth understanding of how to swiftly identify and counter instances of myths and misconceptions would be invaluable for prompt action in the face of disruptive misinformation.
Strengths, limitations and assumptions
This analysis is limited in scope. It draws from key stakeholders within the TIGR2ESS FP-6 group, with additional input from ICRSIAT providing household-level data summaries. The findings reflect a specific group’s experiences and perspectives. While the strengths lie in participants’ expertise in nutrition and food systems, their insights are often secondhand, which can lead to potential misinterpretation. Additionally, recall bias may influence their reflections. The study’s primary objective is not to discuss the nutritional impact of COVID-19-related food system shocks but to gather insights for future research on food environment disruption and resilience-building projects.
The COVID-19 pandemic has illustrated the vulnerabilities of food systems and environments in India. This descriptive analysis highlights several critical themes for future exploration and research by FP-6 stakeholders after the conclusion of the TIGR2ESS project.
For actors in food and nutrition security, potential action-research areas include supply chain resilience, interstate collaboration for emergency response, price stabilisation strategies and a more nuanced understanding of market and vendor policies, particularly for indigenous communities. Examining direct farmer-to-consumer sales and the resurgence of local and traditional foods on dietary patterns, as well as understanding how local decentralised shops can enhance consumer access, are key topics for further investigation.
From the consumer perspective, potential research areas encompass understanding the impact of restrictions on consumers, safeguarding and operating social security programmes during emergencies, assessing the influence of indigenous and local foods on dietary habits, leveraging the promotion of healthy home cooking during the pandemic, exploring both positive and negative effects of digital environments and addressing myths and misconceptions while advocating for nutritious diets.
These insights extend beyond the COVID-19 context, contributing to the ongoing pursuit of building robust food systems capable of withstanding future disruption.
Ethics statements
Patient consent for publication.
Not applicable.
- United Nations
- Swinnen J ,
- McDermott J
- Laborde D ,
- International Labour Organization
- Global Panel on Agriculture and Food Systems for Nutrition
- World Food Programme
- Mahajan K ,
- Narayanan S ,
- Kim EJ , et al
- Times of India
- O’Meara L ,
- Coitinho DC , et al
- Deshpande T
- Aggarwal A ,
- Walls H , et al
- Food and Agriculture Organization of The United Nations
- Ceballos F ,
- Cariappa AA ,
- Acharya KK ,
- Adhav CA , et al
- Iranmanesh M ,
- Ghobakhloo M ,
- Nilashi M , et al
- TCI (Tata–Cornell Institute)
- Schwartz M ,
- Campbell E , et al
- Macaninch E ,
- Lima do Vale M
- Borkowski A ,
- Santiago J , et al
- Matos J de P ,
- World Health Organization Regional Office for Europe
- Wolfson JA ,
- Wrieden W , et al
- World Health Organization
Supplementary materials
Supplementary data.
This web only file has been produced by the BMJ Publishing Group from an electronic file supplied by the author(s) and has not been edited for content.
- Data supplement 1
- Data supplement 2
Contributors JTJ is the guarantor. Significant inputs, draft and comments was provided by all authors. Additional data analysis and coding were provided by MRLdV, RB, WN and SA.
Funding This research was supported by TIGR2ESS through the Global Challenges Reserach Fund, UK
Competing interests None declared.
Provenance and peer review Not commissioned; externally peer reviewed.
Supplemental material This content has been supplied by the author(s). It has not been vetted by BMJ Publishing Group Limited (BMJ) and may not have been peer-reviewed. Any opinions or recommendations discussed are solely those of the author(s) and are not endorsed by BMJ. BMJ disclaims all liability and responsibility arising from any reliance placed on the content. Where the content includes any translated material, BMJ does not warrant the accuracy and reliability of the translations (including but not limited to local regulations, clinical guidelines, terminology, drug names and drug dosages), and is not responsible for any error and/or omissions arising from translation and adaptation or otherwise.
Read the full text or download the PDF:

IMAGES
COMMENTS
Study habits are the most important predictor of academic performance and global research has revealed that study habits affect academic performance. 8 In this regard, ... The t-test was used to compare the mean of study habits in terms of dual-mode qualitative variables (gender and marital status) and ANOVA was used to compare the mean of ...
Exploratory research is intended to examine poorly understood phenomena and generate new insights and hypotheses that can guide future research on the topic (Slavin 2002; Stebbins 2001).In this exploratory study, we examine the study habits of a group of STEM students, with a focus on describing the lived experiences and subjective interpretations of individuals and groups or what cultural ...
The conceptual model, shown in. Figure 1. , assumes that the influence of Study Skill Habits on academic procrastination is mediated by Study Self-Efficacy. The SSH construct is specified as a formative latent construct, whereas SSE and procrastination are specified as reflective latent constructs.
conducting study groups that students perform. frequently, and regularly accomplishing the. learning goals. It can be defined as effective or. counterproductive based onwhetherit serves the ...
Using a qualitative approach, we add to the current field of research by targeting an in-depth exploration of students' study habits, which is currently lacking. Insight into these are essential to tailor educational strategies and training to better align with students' behaviors and needs.
Students' study sessions outside class are important learning opportunities in college courses. However, we often depend on students to study effectively without explicit instruction. In this study, we described students' self-reported study habits and related those habits to their performance on exams. Notably, in these analyses, we controlled for potential confounds, such as academic ...
Study habits are defined as techniques and methods used to manage the learning process (Bayır, 2015). Study ... In the qualitative part of the research, it was designed according to the case study. Descriptive case study design is used. This pattern answers why and how questions about a situation (Karasar, 2012).
The autonomy associated with a large degree of freedom in the study situation makes the student particularly vulnerable if skills are low (→Low focus on study skills training) and if the student fails to develop good habits and routines. Habits help people accomplish more and procrastinate less (e.g., Steel et al., 2018). Of note, study ...
Our recent small-scale qualitative study investigated behavioral diferences between higher- and lower- performing students by conducting multiple interviews with a few students as the CS1 course progressed [18]. We found noticeable diferences in the study habits of higher- and lower- performing students. As the findings resulted from interviews ...
Study habits are the well-planned intended methods of study, the chain of approaches in the process of memorising, systematizing, regulating, retaining novel facts and ideas related to the learning materials, which has gained the shape of consistent endeavours on the part of students, towards comprehending academic subjects and qualifying examinations. The constant practices a person utilizes ...
In this article the author examines the role of habits in and on the practice of qualitative research. To illustrate this topic, he examines how researcher habits can influence the pacing of an inquiry. Qualitative research requires the learned practice of active waiting: striking a balance throughout a research project between moving forward ...
The research team collected study data using an electronic questionnaire containing three sections: socio-demographic data, personal characteristics, and study habits. The study results indicated a statistically significant association between self-fulfillment as a motivation toward studying and academic achievement (p = 0.04).
In this study, the association between study habits and academic performance of students is examined. Sample of 270 students were taken from two colleges Govt. Allama Iqbal College for Women ...
Abstract. This paper aims to provide an overview of the use and assessment of qualitative research methods in the health sciences. Qualitative research can be defined as the study of the nature of phenomena and is especially appropriate for answering questions of why something is (not) observed, assessing complex multi-component interventions ...
International Journal of Quantitative and Qualitative Research Methods Vol.6, No.3, pp.10-23, August 2018 ... District could be attributed to their poor study habits. However, research into the effect between study habits and students' academic performance in the Ekumfi District is rare. It is against
The study explored the priorities and intentions of working students who manage the challenging roles of being a student and a worker. This qualitative study used a descriptive phenomenological ...
A case study is one of the most commonly used methodologies of social research. This article attempts to look into the various dimensions of a case study research strategy, the different epistemological strands which determine the particular case study type and approach adopted in the field, discusses the factors which can enhance the effectiveness of a case study research, and the debate ...
Writing Research. Andrea Bingham. Reporting the findings from a qualitative study in a way that is interesting, meaningful, and trustworthy can be a struggle. Those new to qualitative research often find themselves trying to quantify everything to make it seem more "rigorous," or asking themselves, "Do I really need this much data to ...
In this, one paradigm is used to frame the qualitative aspects of the study and another for the quantitative aspects. This is acceptable, although you will be tasked with explaining your rationale for using both of these paradigms in your research. Takeaway. Choosing the right research paradigm for your research can seem like an insurmountable ...
Qualitative study about study habits of shs students - Free download as PDF File (.pdf), Text File (.txt) or read online for free. This document discusses a research study on the relationship between study habits and academic performance in Statistics and Probability among Grade 11 students. It provides background on the importance of study habits for student success and reviews previous ...
Qualitative research is a methodological approach that involves gathering and analyzing non-numerical data to understand and interpret social phenomena. Unlike quantitative research, which emphasizes the collection of numerical data through surveys and experiments, qualitative research is concerned with exploring the subjective experiences ...
Abstract. Habit formation is an important goal for behaviour change interventions because habitual behaviours are elicited automatically and are therefore likely to be maintained. This study documented experiences of habit development in 10 participants enrolled on a weight loss intervention explicitly based on habit-formation principles.
Qualitative research involves the studied use and collection of a variety of empirical materials - case study, personal experience, introspective, life story, interview, observational, historical, interactional, and visual texts - that describe routine and problematic moments and meanings in individuals' lives.
A nearly 4,000 word, first-person essay about a man's experiences masturbating to Japanese comics of underage boys was published to the peer-reviewed journal Qualitative Research—and is now ...
In this article the author examines the role of habits in and on the practice of qualitative research. To illustrate this topic, he examines how researcher habits can influence the pacing of an inquiry. Qualitative research requires the learned practice of active waiting: striking a balance throughout a research project between moving forward ...
Countries in the Middle East have some of the lowest rates of physical activity and some of the highest rates of obesity in the world. Policies can influence population levels of physical activity. However, there is a dearth of research on physical activity policies in the Gulf region. This qualitative study analyses cross-sectoral barriers and facilitators for the development, implementation ...
Background and aim Globally, COVID-19 has had a profound impact on food and nutrition security. This paper aims to gather the perspective from Transforming India's Green Revolution by Research and Empowerment for Sustainable food Supplies (TIGR2ESS) Flagship Project 6 (FP-6) team on the impact of COVID-19 on the food systems in India. The responses collected will be used for further research ...
To evaluate the app's effectiveness, a sequential mixed-design method combining a pre-and-post-design experiment and focus groups was conducted. Quantitative and qualitative assessments indicated the effectiveness of this mobile app in fostering the development of eco-habits.