Have a language expert improve your writing
Run a free plagiarism check in 10 minutes, generate accurate citations for free.
- Knowledge Base

Hypothesis Testing | A Step-by-Step Guide with Easy Examples
Published on November 8, 2019 by Rebecca Bevans . Revised on June 22, 2023.
Hypothesis testing is a formal procedure for investigating our ideas about the world using statistics . It is most often used by scientists to test specific predictions, called hypotheses, that arise from theories.
There are 5 main steps in hypothesis testing:
- State your research hypothesis as a null hypothesis and alternate hypothesis (H o ) and (H a or H 1 ).
- Collect data in a way designed to test the hypothesis.
- Perform an appropriate statistical test .
- Decide whether to reject or fail to reject your null hypothesis.
- Present the findings in your results and discussion section.
Though the specific details might vary, the procedure you will use when testing a hypothesis will always follow some version of these steps.
Table of contents
Step 1: state your null and alternate hypothesis, step 2: collect data, step 3: perform a statistical test, step 4: decide whether to reject or fail to reject your null hypothesis, step 5: present your findings, other interesting articles, frequently asked questions about hypothesis testing.
After developing your initial research hypothesis (the prediction that you want to investigate), it is important to restate it as a null (H o ) and alternate (H a ) hypothesis so that you can test it mathematically.
The alternate hypothesis is usually your initial hypothesis that predicts a relationship between variables. The null hypothesis is a prediction of no relationship between the variables you are interested in.
- H 0 : Men are, on average, not taller than women. H a : Men are, on average, taller than women.
Receive feedback on language, structure, and formatting
Professional editors proofread and edit your paper by focusing on:
- Academic style
- Vague sentences
- Style consistency
See an example
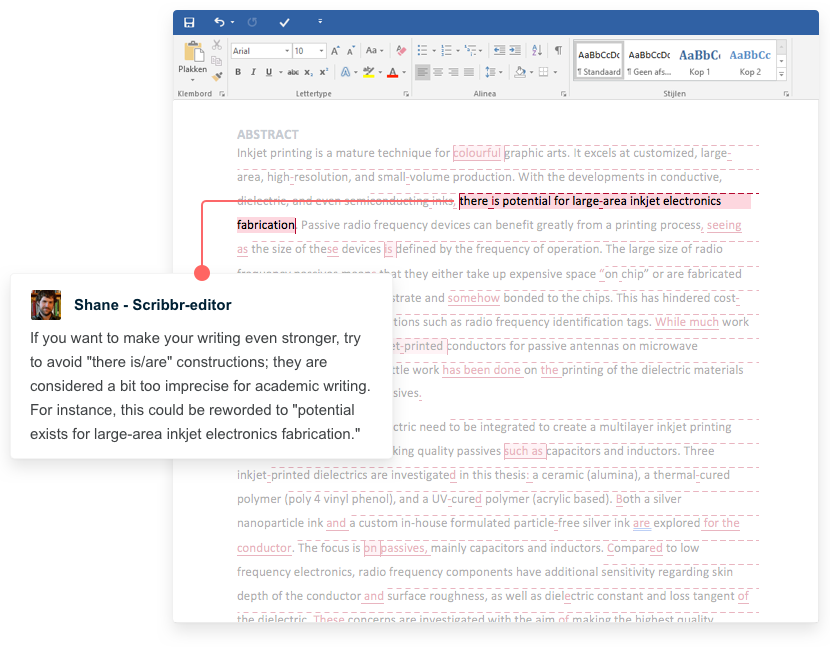
For a statistical test to be valid , it is important to perform sampling and collect data in a way that is designed to test your hypothesis. If your data are not representative, then you cannot make statistical inferences about the population you are interested in.
There are a variety of statistical tests available, but they are all based on the comparison of within-group variance (how spread out the data is within a category) versus between-group variance (how different the categories are from one another).
If the between-group variance is large enough that there is little or no overlap between groups, then your statistical test will reflect that by showing a low p -value . This means it is unlikely that the differences between these groups came about by chance.
Alternatively, if there is high within-group variance and low between-group variance, then your statistical test will reflect that with a high p -value. This means it is likely that any difference you measure between groups is due to chance.
Your choice of statistical test will be based on the type of variables and the level of measurement of your collected data .
- an estimate of the difference in average height between the two groups.
- a p -value showing how likely you are to see this difference if the null hypothesis of no difference is true.
Based on the outcome of your statistical test, you will have to decide whether to reject or fail to reject your null hypothesis.
In most cases you will use the p -value generated by your statistical test to guide your decision. And in most cases, your predetermined level of significance for rejecting the null hypothesis will be 0.05 – that is, when there is a less than 5% chance that you would see these results if the null hypothesis were true.
In some cases, researchers choose a more conservative level of significance, such as 0.01 (1%). This minimizes the risk of incorrectly rejecting the null hypothesis ( Type I error ).
Here's why students love Scribbr's proofreading services
Discover proofreading & editing
The results of hypothesis testing will be presented in the results and discussion sections of your research paper , dissertation or thesis .
In the results section you should give a brief summary of the data and a summary of the results of your statistical test (for example, the estimated difference between group means and associated p -value). In the discussion , you can discuss whether your initial hypothesis was supported by your results or not.
In the formal language of hypothesis testing, we talk about rejecting or failing to reject the null hypothesis. You will probably be asked to do this in your statistics assignments.
However, when presenting research results in academic papers we rarely talk this way. Instead, we go back to our alternate hypothesis (in this case, the hypothesis that men are on average taller than women) and state whether the result of our test did or did not support the alternate hypothesis.
If your null hypothesis was rejected, this result is interpreted as “supported the alternate hypothesis.”
These are superficial differences; you can see that they mean the same thing.
You might notice that we don’t say that we reject or fail to reject the alternate hypothesis . This is because hypothesis testing is not designed to prove or disprove anything. It is only designed to test whether a pattern we measure could have arisen spuriously, or by chance.
If we reject the null hypothesis based on our research (i.e., we find that it is unlikely that the pattern arose by chance), then we can say our test lends support to our hypothesis . But if the pattern does not pass our decision rule, meaning that it could have arisen by chance, then we say the test is inconsistent with our hypothesis .
If you want to know more about statistics , methodology , or research bias , make sure to check out some of our other articles with explanations and examples.
- Normal distribution
- Descriptive statistics
- Measures of central tendency
- Correlation coefficient
Methodology
- Cluster sampling
- Stratified sampling
- Types of interviews
- Cohort study
- Thematic analysis
Research bias
- Implicit bias
- Cognitive bias
- Survivorship bias
- Availability heuristic
- Nonresponse bias
- Regression to the mean
Hypothesis testing is a formal procedure for investigating our ideas about the world using statistics. It is used by scientists to test specific predictions, called hypotheses , by calculating how likely it is that a pattern or relationship between variables could have arisen by chance.
A hypothesis states your predictions about what your research will find. It is a tentative answer to your research question that has not yet been tested. For some research projects, you might have to write several hypotheses that address different aspects of your research question.
A hypothesis is not just a guess — it should be based on existing theories and knowledge. It also has to be testable, which means you can support or refute it through scientific research methods (such as experiments, observations and statistical analysis of data).
Null and alternative hypotheses are used in statistical hypothesis testing . The null hypothesis of a test always predicts no effect or no relationship between variables, while the alternative hypothesis states your research prediction of an effect or relationship.
Cite this Scribbr article
If you want to cite this source, you can copy and paste the citation or click the “Cite this Scribbr article” button to automatically add the citation to our free Citation Generator.
Bevans, R. (2023, June 22). Hypothesis Testing | A Step-by-Step Guide with Easy Examples. Scribbr. Retrieved September 2, 2024, from https://www.scribbr.com/statistics/hypothesis-testing/
Is this article helpful?
Rebecca Bevans
Other students also liked, choosing the right statistical test | types & examples, understanding p values | definition and examples, what is your plagiarism score.

Course Support. Past Paper Questions by Topic. For Free.
Hypothesis Testing
Previous Section: DRV’s & Binomial Distribution
Next Section: AS Statistics General Support
*Note. I like to teach both types of Hypothesis test before Critical Regions and have structured this chapter in that order (though of course you can go through it in the textbook order if you wish).
Whole Topic Notes
7a hypothesis testing, 7c one-tailed tests, 7d two-tailed tests, 7b critical regions, whole topic summary resources (including past paper questions), download full topic slides.
- Textbook Notes as PPTs (Courtesy of Owen134866)
Full Notes to Accompany Slides
- Dr Frost Hypothesis Tests Section
- Dr Frost Hypothesis Testing Slides
- Blank Notes to Follow Slides Word Doc or PDF (Courtesy of King’s Chester Maths Department)
Acute Learning Whole Chapter Problem Pairs
Textbook resources.
Courtesy of Owen134866 and Haberdashers’ Adams Maths Dept.
Download Blank Notes
Download slides.
7A Slides (Courtesy of Owen134866)
Dr Frost resources
Bicen maths live lesson.
Live Lesson Using Dr Frost Based Slides Courtesy of Bicen Maths
(Courtesy of King’s Chester Maths Department)
- Dr Frost Slides 1-12
Extra Questions
- Dr Frost Questions
- No Key Skills Yet
Other resources
Online videos.
Exam Solutions
Statistics joke pic.twitter.com/4XQ1Gm9GgW — Kyle D Evans (@kyledevans) March 20, 2021
Alternative Handouts
- Acute Learning Problem Pairs as PowerPoint or PDF
Online Platforms
- Khan Academy Basic Idea of Hypothesis Tests
7C Slides (Courtesy of Owen134866)
- Dr Frost Slides 21-26
Note all numbers are subject to change and will be updated once all key skills have been finished by Dr Frost
- 858 Conduct a One-Tailed Hypothesis Test on a Binomial Model.
Hypothesis Tests Key Skills Section (for selecting more than one)
Other Videos
Courtesy of Maths Genie (Up to 7:58)
Exam Solutions Examples for One-Tailed test
- Jethwa Maths Starter Activities (p1-2)
7D Slides (Courtesy of Owen134866)
- Dr Frost Slides 27-29
Topic Tests
- Standard Test
- Dr Frost Questions Very limited (most Q’s discuss critical regions)
- 859 Conduct a Two-Tailed Hypothesis Test on a Binomial Model.
Hypothesis Tests Key Skills Section (for selecting more than one)
Further Practice
- Practise Questions
Courtesy of Maths Genie (From 7:58)
- Jethwa Maths Starter Activities (p3-4)
7B Slides (Courtesy of Owen134866)
- Dr Frost Slides 13-20
- 856 Find the Critical Region For a One-Tailed Hypothesis Test Using a Binomial Model.
- 857 Find the Critical Region For a Two-Tailed Hypothesis Test Using a Binomial Model.
- Combination of All
Exam Solutions C-R For 1-Tail
Exam Solutions C-R For Two-Tail
- Jethwa Maths Starter Activities (p5-6)
- Jo Morgan Finding Critical Regions (Need to Download as Word Doc to View Qs)
- MEI Card Sort
Download PPQs as WW Powerpoint for AS Binomial Distribution or A2 Binomial Distribution
External Whole Topic Past Paper Style Questions
- Naiker Maths PPQs ( solutions )
- Jethwa Maths Starter Activities
- Maths Genie Worksheet and Solutions
- Dr Frost Topic Test (Teacher Log in Required)
- Edexcel Hypothesis tests – Tests on binomial
- OCR Hypothesis Tests for the Binomial Distribution
- OCR – MEI Hypothesis Testing for Binomial Probabilities
- Cheat Sheet
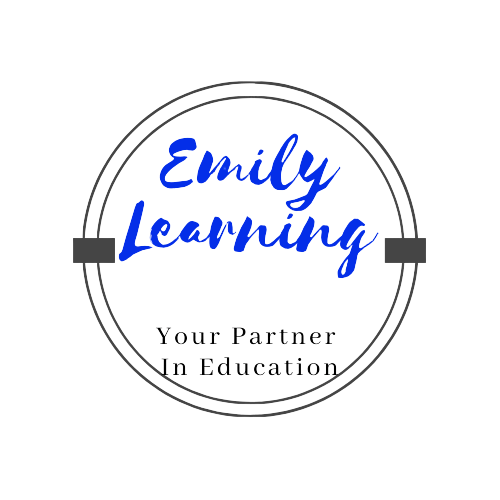
Hypothesis Testing Questions: A Level TYS Answers
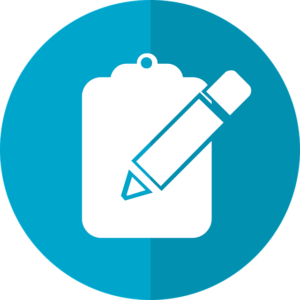
Hypothesis testing questions are found in paper 2, section B (Statistics section) of the H2 A Level Math exam. In this post, you will find the worked solutions for past A Level questions on hypothesis testing.
A list of past questions from each statistics topic can be found here .
A quick summary of formulae in each statistics topics can be found here .
Past A Level Hypothesis testing Questions
These are the past A Level hypothesis testing questions for the new syllabus (syllabus 9758) which started from 2017.
Click on the link to go straight to the worked solutions for each question.
2017 Paper 2 Question 7
2018 Paper 2 Question 5
2019 Paper 2 Question 9
2020 Paper 2 Question 10
2021 Paper 2 Question 8
Hypothesis Testing Question: 2017 Paper 2 Question 7
Hypothesis testing question: 2018 paper 2 question 5.
(i) A sample of at least 30 should be taken, so that sample size is large enough for the mean time to fail of the fans to be approximated to that of a normal distribution by Central Limit Theorem.
(ii) Test H o : µ = 65000 against H 1 : µ < 65000
µ = population mean time to fail of fan
H o = null hypothesis
H 1 = alternative hypothesis
Hypothesis Testing Question: 2019 Paper 2 Question 9
Hypothesis testing question: 2020 paper 2 question 10, hypothesis testing question: 2021 paper 2 question 8, a complete course on h2 a level math statistics.
Want a complete course on H2 A Level Math Statistics?
Check out our course here , where we cover all the topics tested in statistics.
The course includes concepts, types of questions, and how to apply them, so that you’ll score for your exam.
- Data Science
- Data Analysis
- Data Visualization
- Machine Learning
- Deep Learning
- Computer Vision
- Artificial Intelligence
- AI ML DS Interview Series
- AI ML DS Projects series
- Data Engineering
- Web Scrapping
Understanding Hypothesis Testing
Hypothesis testing involves formulating assumptions about population parameters based on sample statistics and rigorously evaluating these assumptions against empirical evidence. This article sheds light on the significance of hypothesis testing and the critical steps involved in the process.
What is Hypothesis Testing?
A hypothesis is an assumption or idea, specifically a statistical claim about an unknown population parameter. For example, a judge assumes a person is innocent and verifies this by reviewing evidence and hearing testimony before reaching a verdict.
Hypothesis testing is a statistical method that is used to make a statistical decision using experimental data. Hypothesis testing is basically an assumption that we make about a population parameter. It evaluates two mutually exclusive statements about a population to determine which statement is best supported by the sample data.
To test the validity of the claim or assumption about the population parameter:
- A sample is drawn from the population and analyzed.
- The results of the analysis are used to decide whether the claim is true or not.
Example: You say an average height in the class is 30 or a boy is taller than a girl. All of these is an assumption that we are assuming, and we need some statistical way to prove these. We need some mathematical conclusion whatever we are assuming is true.
Defining Hypotheses
- Null hypothesis (H 0 ): In statistics, the null hypothesis is a general statement or default position that there is no relationship between two measured cases or no relationship among groups. In other words, it is a basic assumption or made based on the problem knowledge. Example : A company’s mean production is 50 units/per da H 0 : [Tex]\mu [/Tex] = 50.
- Alternative hypothesis (H 1 ): The alternative hypothesis is the hypothesis used in hypothesis testing that is contrary to the null hypothesis. Example: A company’s production is not equal to 50 units/per day i.e. H 1 : [Tex]\mu [/Tex] [Tex]\ne [/Tex] 50.
Key Terms of Hypothesis Testing
- Level of significance : It refers to the degree of significance in which we accept or reject the null hypothesis. 100% accuracy is not possible for accepting a hypothesis, so we, therefore, select a level of significance that is usually 5%. This is normally denoted with [Tex]\alpha[/Tex] and generally, it is 0.05 or 5%, which means your output should be 95% confident to give a similar kind of result in each sample.
- P-value: The P value , or calculated probability, is the probability of finding the observed/extreme results when the null hypothesis(H0) of a study-given problem is true. If your P-value is less than the chosen significance level then you reject the null hypothesis i.e. accept that your sample claims to support the alternative hypothesis.
- Test Statistic: The test statistic is a numerical value calculated from sample data during a hypothesis test, used to determine whether to reject the null hypothesis. It is compared to a critical value or p-value to make decisions about the statistical significance of the observed results.
- Critical value : The critical value in statistics is a threshold or cutoff point used to determine whether to reject the null hypothesis in a hypothesis test.
- Degrees of freedom: Degrees of freedom are associated with the variability or freedom one has in estimating a parameter. The degrees of freedom are related to the sample size and determine the shape.
Why do we use Hypothesis Testing?
Hypothesis testing is an important procedure in statistics. Hypothesis testing evaluates two mutually exclusive population statements to determine which statement is most supported by sample data. When we say that the findings are statistically significant, thanks to hypothesis testing.
One-Tailed and Two-Tailed Test
One tailed test focuses on one direction, either greater than or less than a specified value. We use a one-tailed test when there is a clear directional expectation based on prior knowledge or theory. The critical region is located on only one side of the distribution curve. If the sample falls into this critical region, the null hypothesis is rejected in favor of the alternative hypothesis.
One-Tailed Test
There are two types of one-tailed test:
- Left-Tailed (Left-Sided) Test: The alternative hypothesis asserts that the true parameter value is less than the null hypothesis. Example: H 0 : [Tex]\mu \geq 50 [/Tex] and H 1 : [Tex]\mu < 50 [/Tex]
- Right-Tailed (Right-Sided) Test : The alternative hypothesis asserts that the true parameter value is greater than the null hypothesis. Example: H 0 : [Tex]\mu \leq50 [/Tex] and H 1 : [Tex]\mu > 50 [/Tex]
Two-Tailed Test
A two-tailed test considers both directions, greater than and less than a specified value.We use a two-tailed test when there is no specific directional expectation, and want to detect any significant difference.
Example: H 0 : [Tex]\mu = [/Tex] 50 and H 1 : [Tex]\mu \neq 50 [/Tex]
To delve deeper into differences into both types of test: Refer to link
What are Type 1 and Type 2 errors in Hypothesis Testing?
In hypothesis testing, Type I and Type II errors are two possible errors that researchers can make when drawing conclusions about a population based on a sample of data. These errors are associated with the decisions made regarding the null hypothesis and the alternative hypothesis.
- Type I error: When we reject the null hypothesis, although that hypothesis was true. Type I error is denoted by alpha( [Tex]\alpha [/Tex] ).
- Type II errors : When we accept the null hypothesis, but it is false. Type II errors are denoted by beta( [Tex]\beta [/Tex] ).
Null Hypothesis is True | Null Hypothesis is False | |
---|---|---|
Null Hypothesis is True (Accept) | Correct Decision | Type II Error (False Negative) |
Alternative Hypothesis is True (Reject) | Type I Error (False Positive) | Correct Decision |
How does Hypothesis Testing work?
Step 1: define null and alternative hypothesis.
State the null hypothesis ( [Tex]H_0 [/Tex] ), representing no effect, and the alternative hypothesis ( [Tex]H_1 [/Tex] ), suggesting an effect or difference.
We first identify the problem about which we want to make an assumption keeping in mind that our assumption should be contradictory to one another, assuming Normally distributed data.
Step 2 – Choose significance level
Select a significance level ( [Tex]\alpha [/Tex] ), typically 0.05, to determine the threshold for rejecting the null hypothesis. It provides validity to our hypothesis test, ensuring that we have sufficient data to back up our claims. Usually, we determine our significance level beforehand of the test. The p-value is the criterion used to calculate our significance value.

Step 3 – Collect and Analyze data.
Gather relevant data through observation or experimentation. Analyze the data using appropriate statistical methods to obtain a test statistic.
Step 4-Calculate Test Statistic
The data for the tests are evaluated in this step we look for various scores based on the characteristics of data. The choice of the test statistic depends on the type of hypothesis test being conducted.
There are various hypothesis tests, each appropriate for various goal to calculate our test. This could be a Z-test , Chi-square , T-test , and so on.
- Z-test : If population means and standard deviations are known. Z-statistic is commonly used.
- t-test : If population standard deviations are unknown. and sample size is small than t-test statistic is more appropriate.
- Chi-square test : Chi-square test is used for categorical data or for testing independence in contingency tables
- F-test : F-test is often used in analysis of variance (ANOVA) to compare variances or test the equality of means across multiple groups.
We have a smaller dataset, So, T-test is more appropriate to test our hypothesis.
T-statistic is a measure of the difference between the means of two groups relative to the variability within each group. It is calculated as the difference between the sample means divided by the standard error of the difference. It is also known as the t-value or t-score.
Step 5 – Comparing Test Statistic:
In this stage, we decide where we should accept the null hypothesis or reject the null hypothesis. There are two ways to decide where we should accept or reject the null hypothesis.
Method A: Using Crtical values
Comparing the test statistic and tabulated critical value we have,
- If Test Statistic>Critical Value: Reject the null hypothesis.
- If Test Statistic≤Critical Value: Fail to reject the null hypothesis.
Note: Critical values are predetermined threshold values that are used to make a decision in hypothesis testing. To determine critical values for hypothesis testing, we typically refer to a statistical distribution table , such as the normal distribution or t-distribution tables based on.
Method B: Using P-values
We can also come to an conclusion using the p-value,
- If the p-value is less than or equal to the significance level i.e. ( [Tex]p\leq\alpha [/Tex] ), you reject the null hypothesis. This indicates that the observed results are unlikely to have occurred by chance alone, providing evidence in favor of the alternative hypothesis.
- If the p-value is greater than the significance level i.e. ( [Tex]p\geq \alpha[/Tex] ), you fail to reject the null hypothesis. This suggests that the observed results are consistent with what would be expected under the null hypothesis.
Note : The p-value is the probability of obtaining a test statistic as extreme as, or more extreme than, the one observed in the sample, assuming the null hypothesis is true. To determine p-value for hypothesis testing, we typically refer to a statistical distribution table , such as the normal distribution or t-distribution tables based on.
Step 7- Interpret the Results
At last, we can conclude our experiment using method A or B.
Calculating test statistic
To validate our hypothesis about a population parameter we use statistical functions . We use the z-score, p-value, and level of significance(alpha) to make evidence for our hypothesis for normally distributed data .
1. Z-statistics:
When population means and standard deviations are known.
[Tex]z = \frac{\bar{x} – \mu}{\frac{\sigma}{\sqrt{n}}}[/Tex]
- [Tex]\bar{x} [/Tex] is the sample mean,
- μ represents the population mean,
- σ is the standard deviation
- and n is the size of the sample.
2. T-Statistics
T test is used when n<30,
t-statistic calculation is given by:
[Tex]t=\frac{x̄-μ}{s/\sqrt{n}} [/Tex]
- t = t-score,
- x̄ = sample mean
- μ = population mean,
- s = standard deviation of the sample,
- n = sample size
3. Chi-Square Test
Chi-Square Test for Independence categorical Data (Non-normally distributed) using:
[Tex]\chi^2 = \sum \frac{(O_{ij} – E_{ij})^2}{E_{ij}}[/Tex]
- [Tex]O_{ij}[/Tex] is the observed frequency in cell [Tex]{ij} [/Tex]
- i,j are the rows and columns index respectively.
- [Tex]E_{ij}[/Tex] is the expected frequency in cell [Tex]{ij}[/Tex] , calculated as : [Tex]\frac{{\text{{Row total}} \times \text{{Column total}}}}{{\text{{Total observations}}}}[/Tex]
Real life Examples of Hypothesis Testing
Let’s examine hypothesis testing using two real life situations,
Case A: D oes a New Drug Affect Blood Pressure?
Imagine a pharmaceutical company has developed a new drug that they believe can effectively lower blood pressure in patients with hypertension. Before bringing the drug to market, they need to conduct a study to assess its impact on blood pressure.
- Before Treatment: 120, 122, 118, 130, 125, 128, 115, 121, 123, 119
- After Treatment: 115, 120, 112, 128, 122, 125, 110, 117, 119, 114
Step 1 : Define the Hypothesis
- Null Hypothesis : (H 0 )The new drug has no effect on blood pressure.
- Alternate Hypothesis : (H 1 )The new drug has an effect on blood pressure.
Step 2: Define the Significance level
Let’s consider the Significance level at 0.05, indicating rejection of the null hypothesis.
If the evidence suggests less than a 5% chance of observing the results due to random variation.
Step 3 : Compute the test statistic
Using paired T-test analyze the data to obtain a test statistic and a p-value.
The test statistic (e.g., T-statistic) is calculated based on the differences between blood pressure measurements before and after treatment.
t = m/(s/√n)
- m = mean of the difference i.e X after, X before
- s = standard deviation of the difference (d) i.e d i = X after, i − X before,
- n = sample size,
then, m= -3.9, s= 1.8 and n= 10
we, calculate the , T-statistic = -9 based on the formula for paired t test
Step 4: Find the p-value
The calculated t-statistic is -9 and degrees of freedom df = 9, you can find the p-value using statistical software or a t-distribution table.
thus, p-value = 8.538051223166285e-06
Step 5: Result
- If the p-value is less than or equal to 0.05, the researchers reject the null hypothesis.
- If the p-value is greater than 0.05, they fail to reject the null hypothesis.
Conclusion: Since the p-value (8.538051223166285e-06) is less than the significance level (0.05), the researchers reject the null hypothesis. There is statistically significant evidence that the average blood pressure before and after treatment with the new drug is different.
Python Implementation of Case A
Let’s create hypothesis testing with python, where we are testing whether a new drug affects blood pressure. For this example, we will use a paired T-test. We’ll use the scipy.stats library for the T-test.
Scipy is a mathematical library in Python that is mostly used for mathematical equations and computations.
We will implement our first real life problem via python,
import numpy as np from scipy import stats # Data before_treatment = np . array ([ 120 , 122 , 118 , 130 , 125 , 128 , 115 , 121 , 123 , 119 ]) after_treatment = np . array ([ 115 , 120 , 112 , 128 , 122 , 125 , 110 , 117 , 119 , 114 ]) # Step 1: Null and Alternate Hypotheses # Null Hypothesis: The new drug has no effect on blood pressure. # Alternate Hypothesis: The new drug has an effect on blood pressure. null_hypothesis = "The new drug has no effect on blood pressure." alternate_hypothesis = "The new drug has an effect on blood pressure." # Step 2: Significance Level alpha = 0.05 # Step 3: Paired T-test t_statistic , p_value = stats . ttest_rel ( after_treatment , before_treatment ) # Step 4: Calculate T-statistic manually m = np . mean ( after_treatment - before_treatment ) s = np . std ( after_treatment - before_treatment , ddof = 1 ) # using ddof=1 for sample standard deviation n = len ( before_treatment ) t_statistic_manual = m / ( s / np . sqrt ( n )) # Step 5: Decision if p_value <= alpha : decision = "Reject" else : decision = "Fail to reject" # Conclusion if decision == "Reject" : conclusion = "There is statistically significant evidence that the average blood pressure before and after treatment with the new drug is different." else : conclusion = "There is insufficient evidence to claim a significant difference in average blood pressure before and after treatment with the new drug." # Display results print ( "T-statistic (from scipy):" , t_statistic ) print ( "P-value (from scipy):" , p_value ) print ( "T-statistic (calculated manually):" , t_statistic_manual ) print ( f "Decision: { decision } the null hypothesis at alpha= { alpha } ." ) print ( "Conclusion:" , conclusion )
T-statistic (from scipy): -9.0 P-value (from scipy): 8.538051223166285e-06 T-statistic (calculated manually): -9.0 Decision: Reject the null hypothesis at alpha=0.05. Conclusion: There is statistically significant evidence that the average blood pressure before and after treatment with the new drug is different.
In the above example, given the T-statistic of approximately -9 and an extremely small p-value, the results indicate a strong case to reject the null hypothesis at a significance level of 0.05.
- The results suggest that the new drug, treatment, or intervention has a significant effect on lowering blood pressure.
- The negative T-statistic indicates that the mean blood pressure after treatment is significantly lower than the assumed population mean before treatment.
Case B : Cholesterol level in a population
Data: A sample of 25 individuals is taken, and their cholesterol levels are measured.
Cholesterol Levels (mg/dL): 205, 198, 210, 190, 215, 205, 200, 192, 198, 205, 198, 202, 208, 200, 205, 198, 205, 210, 192, 205, 198, 205, 210, 192, 205.
Populations Mean = 200
Population Standard Deviation (σ): 5 mg/dL(given for this problem)
Step 1: Define the Hypothesis
- Null Hypothesis (H 0 ): The average cholesterol level in a population is 200 mg/dL.
- Alternate Hypothesis (H 1 ): The average cholesterol level in a population is different from 200 mg/dL.
As the direction of deviation is not given , we assume a two-tailed test, and based on a normal distribution table, the critical values for a significance level of 0.05 (two-tailed) can be calculated through the z-table and are approximately -1.96 and 1.96.
The test statistic is calculated by using the z formula Z = [Tex](203.8 – 200) / (5 \div \sqrt{25}) [/Tex] and we get accordingly , Z =2.039999999999992.
Step 4: Result
Since the absolute value of the test statistic (2.04) is greater than the critical value (1.96), we reject the null hypothesis. And conclude that, there is statistically significant evidence that the average cholesterol level in the population is different from 200 mg/dL
Python Implementation of Case B
import scipy.stats as stats import math import numpy as np # Given data sample_data = np . array ( [ 205 , 198 , 210 , 190 , 215 , 205 , 200 , 192 , 198 , 205 , 198 , 202 , 208 , 200 , 205 , 198 , 205 , 210 , 192 , 205 , 198 , 205 , 210 , 192 , 205 ]) population_std_dev = 5 population_mean = 200 sample_size = len ( sample_data ) # Step 1: Define the Hypotheses # Null Hypothesis (H0): The average cholesterol level in a population is 200 mg/dL. # Alternate Hypothesis (H1): The average cholesterol level in a population is different from 200 mg/dL. # Step 2: Define the Significance Level alpha = 0.05 # Two-tailed test # Critical values for a significance level of 0.05 (two-tailed) critical_value_left = stats . norm . ppf ( alpha / 2 ) critical_value_right = - critical_value_left # Step 3: Compute the test statistic sample_mean = sample_data . mean () z_score = ( sample_mean - population_mean ) / \ ( population_std_dev / math . sqrt ( sample_size )) # Step 4: Result # Check if the absolute value of the test statistic is greater than the critical values if abs ( z_score ) > max ( abs ( critical_value_left ), abs ( critical_value_right )): print ( "Reject the null hypothesis." ) print ( "There is statistically significant evidence that the average cholesterol level in the population is different from 200 mg/dL." ) else : print ( "Fail to reject the null hypothesis." ) print ( "There is not enough evidence to conclude that the average cholesterol level in the population is different from 200 mg/dL." )
Reject the null hypothesis. There is statistically significant evidence that the average cholesterol level in the population is different from 200 mg/dL.
Limitations of Hypothesis Testing
- Although a useful technique, hypothesis testing does not offer a comprehensive grasp of the topic being studied. Without fully reflecting the intricacy or whole context of the phenomena, it concentrates on certain hypotheses and statistical significance.
- The accuracy of hypothesis testing results is contingent on the quality of available data and the appropriateness of statistical methods used. Inaccurate data or poorly formulated hypotheses can lead to incorrect conclusions.
- Relying solely on hypothesis testing may cause analysts to overlook significant patterns or relationships in the data that are not captured by the specific hypotheses being tested. This limitation underscores the importance of complimenting hypothesis testing with other analytical approaches.
Hypothesis testing stands as a cornerstone in statistical analysis, enabling data scientists to navigate uncertainties and draw credible inferences from sample data. By systematically defining null and alternative hypotheses, choosing significance levels, and leveraging statistical tests, researchers can assess the validity of their assumptions. The article also elucidates the critical distinction between Type I and Type II errors, providing a comprehensive understanding of the nuanced decision-making process inherent in hypothesis testing. The real-life example of testing a new drug’s effect on blood pressure using a paired T-test showcases the practical application of these principles, underscoring the importance of statistical rigor in data-driven decision-making.
Frequently Asked Questions (FAQs)
1. what are the 3 types of hypothesis test.
There are three types of hypothesis tests: right-tailed, left-tailed, and two-tailed. Right-tailed tests assess if a parameter is greater, left-tailed if lesser. Two-tailed tests check for non-directional differences, greater or lesser.
2.What are the 4 components of hypothesis testing?
Null Hypothesis ( [Tex]H_o [/Tex] ): No effect or difference exists. Alternative Hypothesis ( [Tex]H_1 [/Tex] ): An effect or difference exists. Significance Level ( [Tex]\alpha [/Tex] ): Risk of rejecting null hypothesis when it’s true (Type I error). Test Statistic: Numerical value representing observed evidence against null hypothesis.
3.What is hypothesis testing in ML?
Statistical method to evaluate the performance and validity of machine learning models. Tests specific hypotheses about model behavior, like whether features influence predictions or if a model generalizes well to unseen data.
4.What is the difference between Pytest and hypothesis in Python?
Pytest purposes general testing framework for Python code while Hypothesis is a Property-based testing framework for Python, focusing on generating test cases based on specified properties of the code.
Please Login to comment...
Similar reads.
- data-science
- How to Delete Discord Servers: Step by Step Guide
- Google increases YouTube Premium price in India: Check our the latest plans
- California Lawmakers Pass Bill to Limit AI Replicas
- Best 10 IPTV Service Providers in Germany
- 15 Most Important Aptitude Topics For Placements [2024]
Improve your Coding Skills with Practice
What kind of Experience do you want to share?
Binomial Hypothesis Testing ( Edexcel A Level Maths: Statistics )
Revision note.
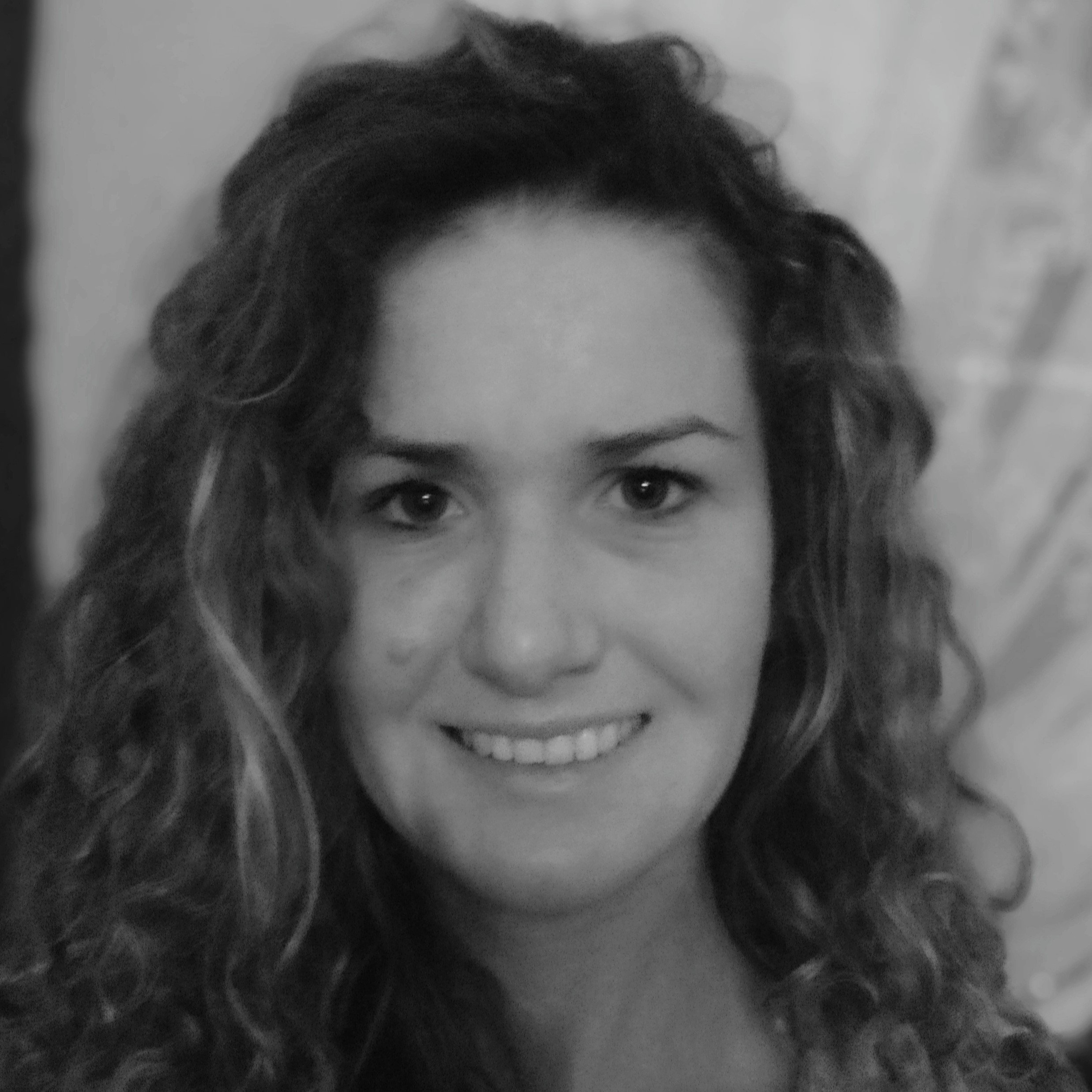
Binomial Hypothesis Testing
How is a hypothesis test carried out with the binomial distribution.
- The population parameter being tested will be the probability, p in a binomial distribution B(n , p)
- A hypothesis test is used when the assumed probability is questioned
- Make sure you clearly define p before writing the hypotheses
- The null hypothesis will always be H 0 : p = ...
- The alternative hypothesis will depend on if it is a one-tailed or two-tailed test
- The alternative hypothesis, H 1 will be H 1 : p > ... or H 1 : p < ...
- The alternative hypothesis, H 1 will be H 1 : p ≠ ...
- To carry out a hypothesis test with the binomial distribution, the test statistic will be the number of successes in a defined number of trials
- When defining the test statistic, remember that the value of p is being tested, so this should be written as p in the original definition, followed by the null hypothesis stating the assumed value of p
- The binomial distribution will be used to calculate the probability of the test statistic taking the observed value or a more extreme value
- either calculating the probability of the test statistic taking the observed or a more extreme value ( p – value ) and comparing this with the significance level
- Finding the critical region can be more useful for considering more than one observed value or for further testing
How is the critical value found in a hypothesis test with the binomial distribution?
- The binomial distribution is a discrete distribution so the probability of the observed value being within the critical region, given a true null hypothesis may be less than the significance level
- This is the actual significance level and is the probability of incorrectly rejecting the null hypothesis
- Use the tables of the binomial distribution or your calculator to find the first value for which the probability of that or a more extreme value is less than half of the given significance level in both the upper and lower tails
- Often one of the critical regions will be much bigger than the other
What steps should I follow when carrying out a hypothesis test with the binomial distribution?
- Step 1. Define the probability, p
- Step 2. Write the null and alternative hypotheses clearly using the form
H 0 : p = ...
H 1 : p = ...
- Step 4. Calculate either the critical value(s) or the p – value for the test
- Step 5. Compare the observed value of the test statistic with the critical value(s) or the p - value with the significance level
- Step 6. Decide whether there is enough evidence to reject H 0 or whether it has to be accepted
- Step 7. Write a conclusion in context
Worked example
Jacques, a breadmaker, claims that more than 80% of people that shop in a particular supermarket buy his brand of bread. Jacques takes a random sample of 100 customers that have purchased bread and asks them which brand of bread they have purchased. He records that 86 of them had purchased his brand of bread. Test, at the 10% level of significance, whether Jacques’ claim is justified.
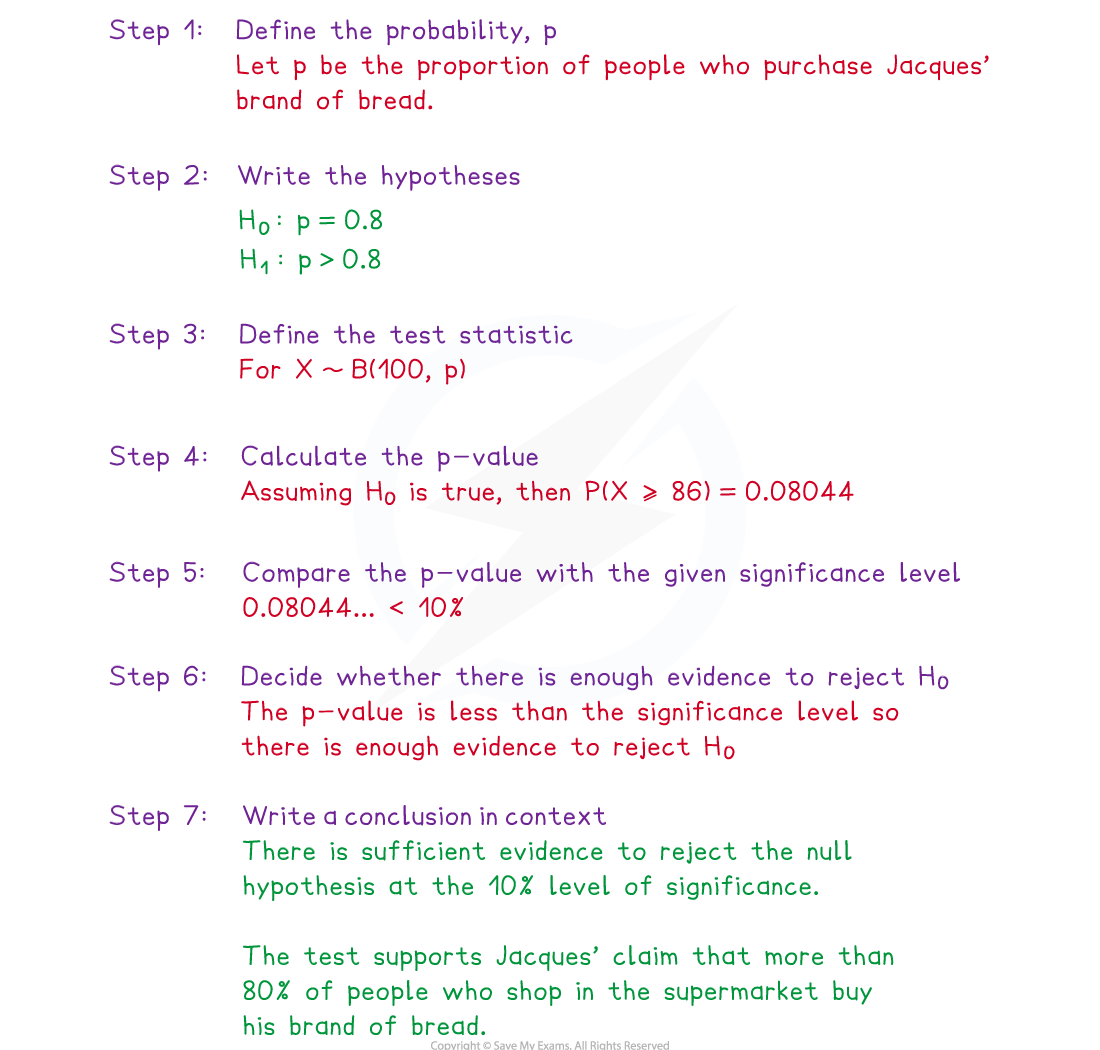
- Make sure you are careful with the inequalities when finding critical values from the binomial tables or from your calculator.
You've read 0 of your 10 free revision notes
Get unlimited access.
to absolutely everything:
- Downloadable PDFs
- Unlimited Revision Notes
- Topic Questions
- Past Papers
- Model Answers
- Videos (Maths and Science)
Join the 100,000 + Students that ❤️ Save My Exams
the (exam) results speak for themselves:
Did this page help you?
Author: Amber
Amber gained a first class degree in Mathematics & Meteorology from the University of Reading before training to become a teacher. She is passionate about teaching, having spent 8 years teaching GCSE and A Level Mathematics both in the UK and internationally. Amber loves creating bright and informative resources to help students reach their potential.
A-Level OCR Stats Maths Past Paper Questions by Topic
Finish sign up, years 1 & 2, other resources, data presentation and interpretation, probability, statistical distributions, hypothesis testing.

IMAGES
VIDEO
COMMENTS
Hypothesis Testing www.naikermaths.com 10. (a) Define the critical region of a test statistic.(2) A discrete random variable X has a Binomial distribution B(30, p).A single observation is used to test H 0: p = 0.3 against H 1: p ≠ 0.3 (b) Using a 1% level of significance find the critical region of this test.You should state the probability of rejection in each tail which should be as close ...
Hypothesis Testing - Edexcel Past Exam Questions MARK SCHEME Question 1: June 05 Q7 Question 2: Jan 06 Q7 . Hypothesis Testing www.naikermaths.com Question 3: June 06 Q7 ... 2nd Bl for "reject the null hypothesis" o.e or the test is significant Ml for using 1st Al 2nd Al 0.0064 3rd Al for (X) < 2 or (X) < 3 They get AO ifthey write 2/ X < 3) ...
State your hypotheses clearly. The data and scatter diagram (Figure 1) show the weight of chickens, x kilograms, and the average weight, y grams, of eggs laid by a random sample of 10 chickens. The product moment correlation coefficient for the average weight of eggs and weight of chickens is −0.136. Test for evidence of a negative population ...
Watch more tutorials in my Edexcel S2 playlist: http://goo.gl/gt1upThis is the fifth in a sequence of tutorials about hypothesis testing. I look at some ques...
Learn how to perform hypothesis tests using binomial distribution with detailed solutions and examples. ExamSolutions - your guide to maths success.
Step 5: Present your findings. The results of hypothesis testing will be presented in the results and discussion sections of your research paper, dissertation or thesis.. In the results section you should give a brief summary of the data and a summary of the results of your statistical test (for example, the estimated difference between group means and associated p-value).
2 - (9709-S 2012-Paper 7/2-Q3) - Hypothesis Testing Using Binomial Distribution, Hypothesis Testing Using Normal Distribution. 3 - (9709-S 2012-Paper 7/3-Q5) - Hypothesis Testing Using Binomial Distribution. 4 - (9709-W 2012-Paper 7/3-Q3) - Hypothesis Testing Using Binomial Distribution, Hypothesis Testing Using Normal Distribution.
All answers. 1 6 marks. A hypothesis test uses a sample of data in an experiment to test a statement made about the value of a population parameter ( ). Explain, in the context of hypothesis testing, what is meant by: (i) 'sample of data', (ii) 'population parameter'. (iii)
Past paper questions for the Hypothesis Testing for Binomial Distributions topic of A-Level Edexcel Maths. Home. ... knowledge. Instead of just taking notes, transform your textbooks into interactive quizzes. Engage with your material, test your understanding, and retain information in a smarter, more effective way. Information Home Help Center ...
Fifteen randomly chosen teenagers were asked how many hours per week they spend on the phone. The sample mean was 4.75 hours with a sample standard deviation of 2.0. Conduct a hypothesis test. The null and alternative hypotheses are: H0: ˉx = 4.5, Ha: ˉx> 4.5 H 0: x ¯ = 4.5, H a: x ¯> 4.5.
A hypothesis test uses a sample of data in an experiment to test a statement made about the population. The statement is either about a population parameter or the distribution of the population. The hypothesis test will look at the probability of observed outcomes happening under set conditions. The probability found will be compared against a ...
HYPOTHESIS TESTING ©MathsDIY.com Page 1 of 4 HYPOTHESIS TESTING AS Unit 2: Applied Mathematics A Section A: Statistics Past paper questions and sample questions: 2017/8 Total marks available 60 (approximately 1 hour 15 minutes) ... In the past, he has found that 70% of all seeds successfully germinate and grow into
Hypothesis tests are based on two hypotheses. The null hypothesis, H_ {0}, is a statement about the value of a population parameter (a parameter of the distribution of a random variable) which our data will tell us whether or not to reject. The alternate hypothesis, H_ {1}, is what we believe the parameter is if we reject the null hypothesis.
Note all numbers are subject to change and will be updated once all key skills have been finished by Dr Frost. 856 Find the Critical Region For a One-Tailed Hypothesis Test Using a Binomial Model. 857 Find the Critical Region For a Two-Tailed Hypothesis Test Using a Binomial Model. Combination of All. Hypothesis Tests Key Skills Section (for ...
A hypothesis test is carried out at the 5% level of significance to test if a normal coin is fair or not. (i) Describe what the population parameter could be for the hypothesis test. (ii) State whether the hypothesis test should be a one-tailed test or a two-tailed test, give a reason for your answer. (iii)
These are the past A Level hypothesis testing questions for the new syllabus (syllabus 9758) which started from 2017. ... 2021 Paper 2 Question 8. Hypothesis Testing Question: 2017 Paper 2 Question 7 Hypothesis Testing Question: 2018 Paper 2 Question 5 (i) A sample of at least 30 should be taken, so that sample size is large enough for the mean ...
Past paper questions for the Hypothesis Testing for Normal Distributions topic of A-Level Edexcel Maths. Home. ... knowledge. Instead of just taking notes, transform your textbooks into interactive quizzes. Engage with your material, test your understanding, and retain information in a smarter, more effective way. Information Home Help Center ...
Here you'll find statistics past paper questions by topic that are applicable to the latest Edexcel specification. As with your exams, these questions cover every difficulty level from E to A* - we recommend not skipping the easier questions as they often trip students in exams. If you're stuggling, we recommend viewing our collection of A ...
Hypothesis testing involves formulating assumptions about population parameters based on sample statistics and rigorously evaluating these assumptions against empirical evidence. ... This paper has been awarded the Best Paper Award in ICLR 2019. ... Machine learning involves conducting experiments based on past experiences, and these hypotheses ...
How is a hypothesis test carried out with the binomial distribution? The population parameter being tested will be the probability, p in a binomial distribution B(n , p); A hypothesis test is used when the assumed probability is questioned ; The null hypothesis, H 0 and alternative hypothesis, H 1 will always be given in terms of p. Make sure you clearly define p before writing the hypotheses
A-Level Pure Maths Paper 2 past paper questions organised by topic for OCR. Home. Revision. ... Hypothesis Tests for the Binomial Distribution. Year 2. Hypothesis Testing for The Normal Distribution. Hypothesis Testing using Pearson's Correlation Coefficient. Other Resources. Video Tutorials.