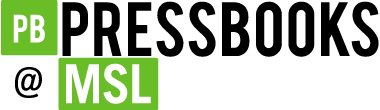
Chapter 8: Making Academic Arguments

8.2 Basic Structure and Content of Argument
Amanda Lloyd and Emilie Zickel
Basic Components of an Argumentative Essay
When you are tasked with crafting an argumentative essay, it is likely that you are to do so based on a number of sources–all of which should support your topic in some way. Your instructor might provide these sources for you, ask you to locate these sources, or provide you with some sources and ask you to find others. Whether or not you are asked to do additional research, an argumentative essay should be comprised of these basic components.
Claim: What do you want the reader to believe?
The thesis in an argument paper is often called a claim. This claim is a statement in which you take a stand on a debatable issue. A strong, debatable claim has at least one valid counterargument–an opposite or alternative point of view that is as sensible as the position that you take in your claim. In your thesis statement, you should clearly and specifically state the position you will convince your audience to adopt.
A closed thesis statement includes sub-claims or reasons why you choose to support your claim. For example:
- The city of Cleveland has displayed a commitment to attracting new residents by making improvements to its walkability, city centers, and green spaces.
In this instance, improvements to walkability, city centers, and green spaces are the sub-claims or reasons why you would make the claim that Cleveland is attracting new residents.
An open thesis statement does not include sub-claims and might be more appropriate when your argument is less easy to prove with two or three easily-defined sub-claims. The choice between an open or a closed thesis statement often depends upon the complexity of your argument. When in doubt about how to structure your thesis statement, you should seek the advice of your instructor.
Consult section 3.4 for help constructing a strong open or closed thesis statement.
Context: What background information about the topic does your audience need?
Before you get into defending your claim, you will need to place your topic (and argument) into context by including relevant background material. Remember, your audience is relying on you for vital information, such as definitions, historical placement, and controversial positions. This background material might appear in either your introductory paragraph/s or your body paragraphs. How and where to incorporate background material depends a lot upon your topic, assignment, evidence, and audience.
Evidence or Grounds: What makes your reasoning valid?
To validate the thinking that you put forward in your claim and sub-claims, you need to demonstrate that your reasoning is not only based on your personal opinion. Evidence, sometimes referred to as grounds, can take the form of research studies or scholarship, expert opinions, personal examples, observations made by yourself or others, or specific instances that make your reasoning seem sound and believable. Evidence only “works” if it directly supports your reasoning — and sometimes you must explain how the evidence supports your reasoning (do not assume that a reader can see the connection between evidence and reason that you see).
Section 4.4 provides a thorough overview of what evidence is and how evidence fits into body paragraphs. As you plan or draft your argument, use this chapter as a resource to help you organize ideas.
Warrants: Why should a reader accept your claim?
A warrant is the rationale the writer provides to show that the evidence properly supports the claim , with each element working towards a similar goal. Think of warrants as the glue that holds an argument together and ensures all pieces work together coherently.
An important way to ensure you are properly supplying warrants within your argument is to use “linking sentences” or a “link” that connects the particular claim directly back to the thesis. Ensuring that there are linking sentences in each paragraph will help to create consistency within your essay. Remember, the thesis statement is the driving force of organization in your essay, so each paragraph needs to have a specific purpose in proving or explaining your thesis; linking sentences complete this task. These linking sentences will often appear after your textual evidence in a paragraph. See Section 4.5 for help linking supporting evidence to your thesis.
Counterargument: But what about other perspectives?
In Section 10.4 , Steven Krause offers a thorough explanation of what counterargument is (and how to respond to it). In summary, a strong arguer should not be afraid to consider perspectives that either challenge or completely oppose his or her own claim. When you respectfully and thoroughly discuss perspectives or research that counters your own claim or even weaknesses in your own argument, you are showing yourself to be an ethical arguer. Here are some things that counterarguments may consist of:
- summarizing opposing views
- explaining how and where you actually agree with some opposing views
- acknowledging weaknesses or holes in your own argument
You have to be careful and clear that you are not conveying to a reader that you are rejecting your own claim; it is important to indicate that you are merely open to considering alternative viewpoints. Being open in this way shows that you are an ethical arguer – you are considering many viewpoints.
Response to Counterargument: I see that, but…
Just as it is important to include counterargument to show that you are fair-minded and balanced, you must respond to the counterargument so that a reader clearly sees that you are not agreeing with the counterargument and thus abandoning or somehow undermining your own claim. Failure to include the response to counterargument can confuse the reader. There are several ways to respond to a counterargument. You can:
- concede to a specific point or idea from the counterargument by explaining why that point or idea has validity. However, you must then be sure to return to your own claim, and explain why even that concession does not lead you to completely accept or support the counterargument
- reject the counterargument if you find it to be incorrect, fallacious, or otherwise invalid
- explain why the counterargument perspective does not invalidate your own claim
Again, Chapter 10.4 offers a much more developed discussion of how to respond to counterarguments.
A note about where to put the counterargument:
It is certainly possible to begin the argument section (after the background section) with your counterargument + response instead of placing it at the end of your essay. Some people prefer to have their counterargument first, where they can address it and then spend the rest of their essay building their own case and supporting their own claim. However, it is just as valid to have the counterargument + response appear at the end of the paper, after you have discussed all of your reasons.
What is important to remember is that wherever you place your counterargument, you
- Address the counterargument(s) fully. Explain what the counter perspectives are. Describe them thoroughly. Cite authors who have these counter perspectives. Quote them and summarize their thinking.
- Then, respond to these counterarguments. Make it clear to the reader of your argument why you concede to certain points of the counterargument or why you reject them. Make it clear that you do not accept the counterargument, even though you understand it. Be sure to use transition phrases that make this clear to your reader. This page contains material from “About Writing: A Guide” by Robin Jeffrey, OpenOregon Educational Resources , Higher Education Coordination Commission: Office of Community Colleges and Workforce Development is licensed under CC BY 4.0
1st Edition: A Guide to Rhetoric, Genre, and Success in First-Year Writing (No Longer Updated) by Amanda Lloyd and Emilie Zickel is licensed under a Creative Commons Attribution-NonCommercial-ShareAlike 4.0 International License , except where otherwise noted.
Feedback/Errata
Comments are closed.
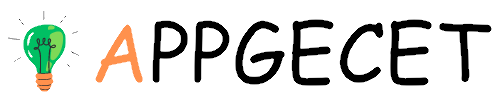
The Difference Between Open and Closed Theses
- by Adam Davis
- October 29, 2023
Are you struggling to understand the difference between an open and closed thesis? Don’t worry, you’re not alone! Many students grapple with this concept when writing academic papers. But fear not, because in this blog post, we’re going to break it down for you.
Before we dive in, let’s quickly clarify what a thesis is. A thesis is a statement or argument that you make in your essay or research paper, and it acts as the central point around which your entire piece revolves . It helps to guide your readers and gives them a clear understanding of what to expect.
Now, an open thesis is like an invitation to a party. It leaves some room for interpretation and allows for multiple possibilities and perspectives. On the other hand, a closed thesis is more decisive and limits the discussion to a specific point or argument. It leaves little room for interpretation and provides a clear stance.
So, with that basic understanding, let’s delve deeper into the world of open and closed theses and explore how they can shape your academic writing journey . Let’s get started!
What is the Difference Between an Open and Closed Thesis
So, you’re here because you’re knee-deep in the mysterious world of thesis statements. Fear not, my friend! Today, we will unravel the enigma that is the difference between an open and closed thesis. Prepare for enlightenment and a sprinkle of humor along the way!
Closed Thesis: Locked, Loaded, and Precise
Oh, the closed thesis! It’s like a perfectly folded origami crane – neat, precise, and delightfully structured. This type of thesis leaves no room for interpretation and nails its point home like a bullseye. It clearly states the main argument and provides a roadmap for the forthcoming paragraphs , leaving no space for ambiguity. Talk about confidence!
Open Thesis: The Wild, Untamed Maverick
Ah, the open thesis, the bohemian of the thesis world. Picture it as a brave intrepid explorer , venturing into the unknown. Unlike its closed counterpart, an open thesis is open-ended, allowing for exploration, expansion, and maybe even a surprising twist or two. It doesn’t lay everything on the table from the get-go, leaving readers intrigued and eager to uncover more.
Closed Thesis Vs. Open Thesis: A Showdown for the Ages
Now, let’s pit these contenders against each other in a friendly thought-provoking debate. Drumroll, please!
Precision: Closed 1, Open 0
In terms of precision, the closed thesis grabs the gold. It tells readers exactly what to expect. Like a master chef orchestrating a symphony of flavors, the closed thesis serves up a dish that leaves no room for guesswork. It’s straightforward, clear, and concise, making it perfect for shorter essays or when you want to hit that thesis nail right on the head!
Flexibility: Open 1, Closed 0
But here comes the wild child – the open thesis! While it may not be the king of precision, it reigns supreme in the realm of flexibility. The open thesis gives you the freedom to wander off the beaten path, explore side alleys, and embrace the serendipitous discoveries along the way. It allows for a more creative and dynamic approach, making it ideal for longer essays or research papers that demand a little extra spice.
Engagement: It’s a Tie!
Both closed and open theses have their unique superpowers when it comes to engagement. A well-crafted closed thesis grabs attention with its laser-focused argument, enticing readers to dive deeper into your writing. On the other hand, an open thesis sparks curiosity, leaving readers hungry for more. So, in the battle for engagement, we declare it a tie – both theses know how to work their charm!
So, Which One Wins
The beauty of the closed versus open thesis debate is that there’s no real winner. It all comes down to your writing goals, the type of essay, and your personal style. Sometimes, a closed thesis is like a finely tailored suit, while other times, an open thesis is the Bohemian artist’s smock you need. So, embrace both, my friend, and let the context guide you in choosing the best thesis for your writing adventures!
Ready to Forge Ahead
Now that you’re armed with the knowledge of the differences between an open and closed thesis, you can confidently choose which path to embark upon. Remember, the closed thesis is for those who desire precision, while the open thesis is for the daring souls seeking exploration. Whichever you choose, go forth and conquer the writing realm!
Happy writing, fellow wordsmiths!
This article is in markdown format and doesn’t contain any extra text or AI-generated disclosure.
FAQ: What is the difference between an open and closed thesis
What are open and closed questions.
In order to understand the difference between an open and closed thesis, it’s important to first grasp the concept of open and closed questions. Open questions are those that invite a detailed response, often requiring the respondent to provide their own perspective or insights. For example, “What is your favorite vacation destination and why?” is an open question. On the other hand, closed questions are those that can typically be answered with a simple “yes” or “no” or a brief response. An example of a closed question would be, “Have you ever been to Paris?”
What is the difference between an open and closed thesis
Now that we have a clear understanding of open and closed questions, it’s easier to comprehend the difference between an open and closed thesis. A thesis statement is the main argument or point that a writer aims to prove or support in an essay or research paper. An open thesis leaves room for interpretation and discussion, allowing the writer to explore various angles and perspectives. It presents a broader view of the topic and encourages critical thinking. Conversely, a closed thesis is more specific and directly states the writer’s position on the topic without leaving room for ambiguity. It leaves little room for interpretation and usually presents a clear and concise argument.
What does an open paragraph mean
An open paragraph, sometimes referred to as a topic paragraph or lead paragraph, is the opening section of a written piece. It sets the context, introduces the main topic, and engages the reader’s interest. Similar to an open thesis, an open paragraph leaves room for exploration and discussion. It may present a general overview or provide background information without immediately delving into a specific argument. The purpose of an open paragraph is to capture the reader’s attention and encourage them to continue reading.
What is the most challenging question to ask a boyfriend
Ah, the notorious challenge of coming up with the most difficult question to ask a boyfriend! While it may vary depending on the individuals involved, let’s explore a question that can really put their quick thinking and emotional intelligence to the test: “If you were stranded on a deserted island and could only save one item, what would it be and why?” This question not only prompts your boyfriend to consider his survival instincts but also gives you insight into his values and priorities. It’s always fascinating to see how one’s mind works when faced with such dilemmas!
So, now that you understand the difference between an open and closed thesis, you can confidently approach your writing with a clearer perspective. Consider the purpose and style of your piece, and choose the type of thesis that best aligns with your intentions. Whether you opt for an open or closed thesis, remember to engage your readers, provide solid arguments, and present your information with confidence. Happy writing!
Note: The content provided in this blog is for informational purposes only and does not constitute professional advice. Always consult a qualified expert for specific guidance.
- academic papers
- academic writing journey
- brave intrepid explorer
- clear understanding
- closed questions
- closed thesis
- forthcoming paragraphs
- interpretation
The Nebular Hypothesis: Exploring the Birth of our Solar System
Why you should consider becoming a surgical technologist, you may also like, how to get rid of the uncomfortable moodlet in sims 4.
- by Veronica Lopez
- October 27, 2023
My Eye Feels Like Something is Crawling: Causes and Solutions
- October 19, 2023
What Food Category is Mayonnaise?
- October 22, 2023
Cultism and Its Effects: Understanding the Dark Side
- by Sandra Vargas
- October 10, 2023
Do Synchros Go to the Graveyard? Exploring the Fate of Synchro Monsters
- by Matthew Morales
Can I Use an 8 Ohm Amp with 6 Ohm Speakers?
- by PatrickTurner
- October 18, 2023

What Is Open Thesis? 6 Tips to Make It More Understandable For Your Readers
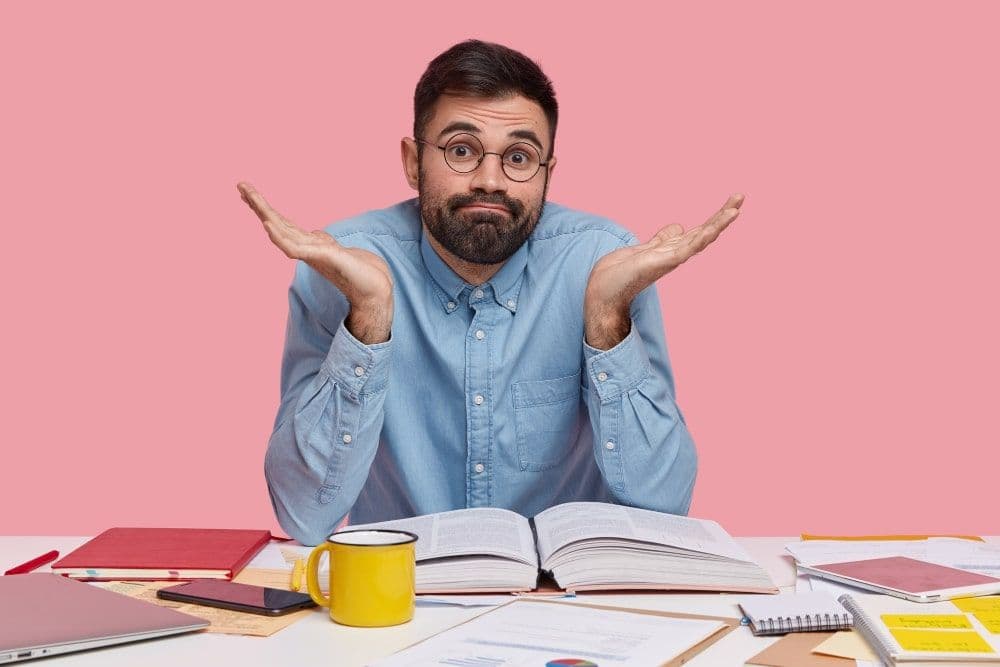
Often viewed as the elusive and enigmatic element of academic writing, open thesis statements send chills down the spines of even the most experienced and seasoned scholars. Reason? Because of its wider reach than a closed thesis, which commands attention and requires meticulous scrutiny.
Open thesis statements present a unique opportunity for creative exploration and expression. However, it is essential to ensure that these statements are lucid, succinct, and comprehensible to readers. How, then, can one demystify the complexity of open thesis statements and present them in a more accessible and discernible manner? Here are the 6 best tips to aid in crafting an open thesis statement that is approachable and lucid for your intended audience.
What Is an Open Thesis Statement?
An open thesis statement is a statement that is not fully formed or definitive in nature. It leaves room for interpretation and discussion, allowing the writer to explore various angles of a topic.
On the one hand, it allows for more creative expression and exploration. But, on the other hand, it can be confusing and difficult to understand for readers.
6 Tips to Write the Most Efficient Open Thesis Statement
1- understanding the open thesis statement.
An open thesis statement differs from a closed thesis statement because it is not a definitive claim. It's more of a starting point for the writer to explore a topic. For example, a closed thesis statement might be something like, "The death penalty is immoral and should be abolished." On the same topic, an open thesis statement may be, "The death penalty raises ethical concerns and merits further investigation."
2- Importance of Clarity in Open Thesis Statements
The key to making open thesis statements more understandable is to ensure they are clear and concise. Avoid using vague or abstract language that can be interpreted in multiple ways. Instead, use specific language that leaves no room for confusion. Instead of expressing, "The economy is bad," say, "The unemployment rate has increased by 5% in the past year."
3- Use of Concrete Language
Open thesis statements can be made more understandable by using definite or concrete language. Concrete language is a language that refers to tangible, observable things. Abstract language, on the other hand, refers to intangible concepts or ideas. Rather than stating that "love is a powerful emotion," you may add, "When I'm with my partner, I feel happy and content."
4- Providing Context
Context helps the reader understand the writer's perspective and gives them a framework for understanding the argument. For example, instead of saying, "The education system needs to be reformed," you may say, "As a teacher, I've personally seen the negative impact of standardized testing on student learning."
5- Use of Supporting Evidence
Supporting evidence provides the reader with tangible examples illustrating the writer's argument. When selecting supporting evidence, choose relevant, reliable, and compelling examples. For example, instead of writing "Many people support gun control," state, "According to a recent poll conducted by McKinsey, 65% of Americans support stricter gun control laws."
6- Use of Examples
Examples provide the reader with concrete instances that illustrate the writer's point. When selecting examples, choose ones that are relevant, memorable, and thought-provoking. Instead of saying, "Fast food is bad for your health," for instance, say, "In 2019, a man ate nothing but McDonald's for 30 days and gained 17 pounds."
Hence, open thesis statements can seem daunting, but they don't have to be. Follow these six tips and make your open thesis statements more understandable and engaging for your readers. Being clear, concise, and specific is all you need to be here!
Editor’s pick
Get free updates.
Subscribe to our newsletter for regular insights from the research and publishing industry!

The Scholarly Tales
For and by researchers, librarians and other scholarly folk at KU Leuven's Faculty of Arts
Open or closed? Which availability to choose for your dissertation
In the blog series “Researcher questions” the Artes Research team shares some common and/or pertinent questions that we get from researchers at the Faculty of Arts. The goal of the series is to share the advice that we give more broadly, as it might be helpful to others as well or make you consider something you had not thought about before.
Sometimes, researchers ask us to close off access to a dissertation that had initially been made available in Open Access through our institutional repository Lirias . The main motivation for this is the fear that publishers won’t be willing to publish the material if it can already be freely consulted online. This fear stems from a general anxiety that characterizes the dissertation process: the idea that developing your dissertation is still something you mainly do alone, without publicly sharing too many details, driven by, on the one hand, a feeling of insecurity to already share your work before it’s “ready” and, on the other hand, the worry that your ideas might be “stolen”. [1] However, more collaboration can only help to improve the project. In this blogpost I will address some common concerns and show that sharing your dissertation in OA offers various advantages and does not contradict the ambition of publishing your work.
Am I obligated to make my dissertation available in Open Access?
At KU Leuven, OA for your dissertation is a choice, not an obligation. While you are always required to archive the electronic version of your PhD thesis in Lirias (practical guidelines about how to upload your dissertation can be found here ), opening it up publicly is not mandatory. We offer the following accessibility options:
- Public access: if you select this option your dissertation will be placed under a temporary embargo of 12 months starting from the date of your defense. This means that during those first 12 months, only internal KU Leuven users (staff and students) will be able to access your work. After the expiration of the embargo, the dissertation will become openly available so that everyone can find and read it. Of course, you can also choose to make your dissertation immediately available in Open Access. Just contact the Open Access Support Desk and they will gladly remove the embargo. The other way around is also possible: if you prefer a longer embargo period (e.g. 2 or 3 years) just ask them to make the change.
- Permanent embargo: this means that your dissertation is indefinitely only available for internal KU Leuven users.
- No access: while this option is available it is actually only recommended for material that has to remain strictly confidential (e.g. for economic or political reasons) as it means that nobody, except for a few repository administrators, can consult the file. Even you and your (co)supervisor(s) cannot access the dissertation anymore.
Most KU Leuven researchers go for the public access license so that they have 12 more months to either decide to close their dissertation off longer, or let it become publicly available.
I want to stress here that the choice you make when uploading your dissertation is not permanent. It might not be the best time to decide about the accessibility of your work when you are about to defend. I know from experience that this is a very stressful time and that you are not up to dealing with practicalities. Maybe you aren’t really familiar yet with how repositories and Green OA work and don’t have time to figure out the details. And you probably haven’t decided yet if, let alone where, you wish to publish your research, meaning that you can’t take publishers’ policies regarding the matter into account. Therefore, a solution might be to initially share your dissertation in OA, so that others can engage with your work while you are still exploring your options. Once you have signed a contract with a publisher and they prefer you to (temporarily) restrict access to your dissertation, you can always ask the Open Access Support Desk to change the availability.
Why would I publish my dissertation in Open Access?
Making your dissertation available in OA has various advantages. First and foremost, it cannot be denied that OA will increase readership and boost citations as your work will be easily findable and accessible to interested readers both inside and outside academia. This in turn promotes active debate about your research, and the feedback can be a great help when revising your manuscript. Moreover, your work can be picked up much faster on a global scale, which increases your chances of collaboration and puts you on the radar in the international job market.
While it might seem contradictory, the fact that your work is out in the open can also help to bring it to the attention of publishers. For example, back in 2013, Harvard University Press stated that thanks to OA the odds increase of them picking up the dissertation under the slogan “If you can’t find it, you can’t sign it” . [2] Open Access is also interesting for publishers from a marketing perspective. Thanks to the open availability the work can already attract readers and instigate discussions, the same audience will most likely also be interested in reading the reworked publication. [3]
What you might need to verify before publicly sharing your dissertation is if you are using material that is protected by copyright licenses (e.g. images, archival sources, etc.), or if you are working with personal data of living individuals. It’s best to already solve these kind of issues at the start of your project, but if you still have some uncertainties about this, just reach out to us or our colleagues from the Copyright Support Desk .
Will a publisher accept my manuscript if the dissertation is available in Open Access?
Unfortunately, a resource or up to date index that collects publisher policies concerning this issue does not exist. In any case, such a resource would be hard to maintain since policies are not set in stone and can change over time. Presses also do not provide much data about OA dissertations that they publish and it has been a while since surveys last asked publishers about the possible impact of OA on their willingness to publish dissertation-based books. Nevertheless, we can gain some valuable insights from older surveys about this topic.
Most notably is the study conducted in 2011 on the policies of arts, humanities, and social sciences journals and university presses. [4] They found that fewer than 5% of total respondents would never consider to publish a dissertation that is already available in OA. In other words, the great majority of the journal and university press editors surveyed were open to publish such a dissertation, on the condition that the original content was substantially adapted. Needless to say, their decisions to publish were made on a case-to-case basis (just as with any type of publication). The survey also revealed that publishers are much more concerned with the quality of the work than prior access to, what they qualify as, an unpublished dissertation. A similar, smaller-scale UK survey conducted in 2015-2016 surveyed 23 university and commercial presses, again in the field of arts, humanities, and social sciences. [5] Their findings revealed that none of the respondents would outright refuse to publish a monograph derived from a dissertation available in OA.
From these studies, and from our own experiences and anecdotal evidence we have gathered along the way, we can conclude that most publishers consider, without prejudice, submissions derived from openly available dissertations. Publishers most often expect you to significantly revise, rewrite, and reframe your dissertation when turning it into a monograph or article. As they consider major edits to be inevitable, they don’t particularly mind that the dissertation is openly available and won’t reject your manuscript on the grounds of prior publication. [6] However, publishers’ policies naturally differ; there are still publishers that may object to the open availability of your dissertation. More broadly speaking, there are also publishers that do not publish monographs that are too close to a PhD thesis in general, regardless of the dissertation’s OA status. [7] Don’t be afraid to just contact the publisher you are considering and, if need be, alter the availability of your dissertation with just one email to the Open Access Support Desk .
Does OA facilitate plagiarism?
Besides the fear that OA will prevent future publication, another common concern is that OA increases the chances of your work being plagiarized. This fear is unwarranted: OA can actually help to protect your work, as plagiarism is much easier to detect when the original work is freely available. Furthermore, if you are aiming for a book publication, it will take some time to rework your PhD into a monograph. If your work can be consulted online in the meantime, you create a transparent public record of your research. This can serve as proof that you formulated certain ideas first, protecting you from scooping rather than enabling it. This way, OA can deter plagiarism and idea theft. [8]
While I wanted to demonstrate in this post that OA is not something to fear, but to embrace, I do want to underline that you always have the final say in how you wish to share your work based on your own experiences, prospects, and what you feel comfortable with. The key takeaways I want to leave you with are:
- KU Leuven has an opt-in policy for OA when it comes to dissertations, and you are free to select your preferred availability.
- OA can help bring your work to the attention of interested scholars, possible future employers, and potentially even publishers.
- The chance that a publisher will reject a manuscript based on an openly available dissertation is minimal, but you can always just contact them to clarify the matter. If they do make objections, remember that the availability of your dissertation can always be altered.
- OA deters instead of enables plagiarism.
If you still have concerns or questions about the availability of your dissertation or any other digital scholarship-related matters, do not hesitate to contact the Artes Research team !
[1] Kathleen Fitzpatrick has described this unease with publicly sharing the dissertation process and puts forward digital scholarship as a way to eliminate such anxieties: Kathleen Fitzpatrick, “Dissertating in Public,” in Shaping the Digital Dissertation: Knowledge Production in the Arts and Humanities , ed. Virginia Kuhn and Anke Finger, 2021, 19–23, http://www.openbookpublishers.com/books/10.11647/obp.0239.
[2] “Can’t Find It, Can’t Sign It: On Dissertation Embargoes,” Harvard University Press Blog (blog), accessed August 19, 2022, https://harvardpress.typepad.com/hup_publicity/2013/07/cant-find-it-cant-sign-it-on-dissertation-embargoes.html; also cited in: Jill Cirasella and Polly Thistlethwaite, “Open Access and the Graduate Author: A Dissertation Anxiety Manual,” in Open Access and the Future of Scholarly Communication: Implementation , ed. Kevin L. Smith and Katherine A. Dickson (Lanham: Rowman & Littlefield Publishers, 2016), 206.
[3] Cirasella and Thistlethwaite, “Open Access and the Graduate Author: A Dissertation Anxiety Manual”, 210.
[4] Marisa L. Ramirez et al., “Do Open Access Electronic Theses and Dissertations Diminish Publishing Opportunities in the Social Sciences and Humanities? Findings from a 2011 Survey of Academic Publishers,” College & Research Libraries 74, no. 4 (2013): 368–80, https://doi.org/10.5860/crl-356.
[5] Christian Gilliam and Christine Daoutis, “Can Openly Accessible E- Theses Be Published as Monographs? A Short Survey of Academic Publishers,” The Serials Librarian 75, no. 1–4 (2019): 5–12, https://doi.org/10.1080/0361526X.2019.1589633.
[6] Cirasella and Thistlethwaite, “Open Access and the Graduate Author: A Dissertation Anxiety Manual”, 206; Fitzpatrick, “Dissertating in Public”, 22-23. A study conducted in 2018 alsoconcluded that onlya small percentage of dissertations are published as books with relatively few changes: Karen Rupp-Serrano and Jen Waller, “Dissertation-to-Book Publication Patterns Among a Sample of R1 Institutions,” Journal of Librarianship and Scholarly Communication 6, no. 1 (2018): 1–23, https://doi.org/10.7710/2162-3309.2187. If a publisher is right to see the dissertation and the monograph as two completely distinct species, or whether we should approach the dissertation more as an already finished product that could be published as such, is another discussion that I won’t address here.
[7] E.g. “Publishing Your Book with MUP,” Manchester University Press, accessed August 19, 2022, https://manchesteruniversitypress.co.uk/resources/publishing-your-book-with-mup/.
[8] Peter Suber, Open Access , MIT Press Essential Knowledge Series (Cambridge: MIT Press, 2012), 23–24; Cirasella and Thistlethwaite, “Open Access and the Graduate Author: A Dissertation Anxiety Manual”, 212.
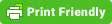

- Houston Community College
- Eagle Online

- Stacey Higdon
- English Composition I (ENGL 1301-76258)
Open vs. Closed Writing
To print or download this file, click the link below:

Skip navigation
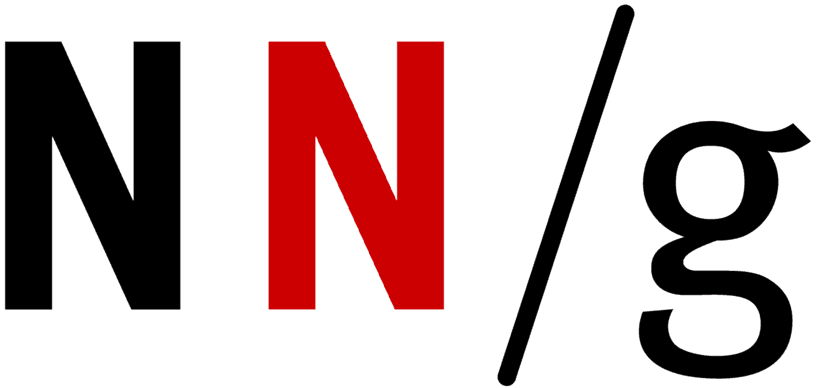
World Leaders in Research-Based User Experience
Open-ended vs. closed questions in user research.
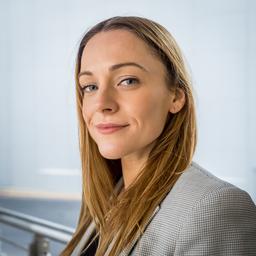
January 26, 2024 2024-01-26
- Email article
- Share on LinkedIn
- Share on Twitter
When conducting user research, asking questions helps you uncover insights. However, how you ask questions impacts what and how much you can discover .
In This Article:
Open-ended vs. closed questions, why asking open-ended questions is important, how to ask open-ended questions.
There are two types of questions we can use in research studies: open-ended and closed.
Open-ended questions allow participants to give a free-form text answer. Closed questions (or closed-ended questions) restrict participants to one of a limited set of possible answers.
Open-ended questions encourage exploration of a topic; a participant can choose what to share and in how much detail. Participants are encouraged to give a reasoned response rather than a one-word answer or a short phrase.
Examples of open-ended questions include:
- Walk me through a typical day.
- Tell me about the last time you used the website.
- What are you thinking?
- How did you feel about using the website to do this task?
Note that the first two open-ended questions are commands but act as questions. These are common questions asked in user interviews to get participants to share stories. Questions 3 and 4 are common questions that a usability-test facilitator may ask during and after a user attempts a task, respectively.
Closed questions have a short and limited response. Examples of closed questions include:
- What’s your job title?
- Have you used the website before?
- Approximately, how many times have you used the website?
- When was the last time you used the website?
Strictly speaking, questions 3 and 4 would only be considered “closed” if they were accompanied by answer options, such as (a) never, (b) once, (c) two times or more. This is because the number of times and days could be infinite. That being said, in UX, we treat questions like these as closed questions.
In the dialog between a facilitator and a user below, closed questions provide a short, clarifying response, while open-ended questions result in the user describing an experience.
T | |
Using Closed Questions in Surveys
Closed questions are heavily utilized in surveys because the responses can be analyzed statistically (and surveys are usually a quantitative exercise). When used in surveys, they often take the form of multiple-choice questions or rating-scale items , rather than open-text questions. This way, the respondent has the answer options provided, and researchers can easily quantify how popular certain responses are. That being said, some closed questions could be answered through an open-text field to provide a better experience for the respondent. Consider the following closed questions:
- In which industry do you work?
- What is your gender?
Both questions could be presented as multiple-choice questions in a survey. However, the respondent might find it more comfortable to share their industry and gender in a free-text field if they feel the survey does not provide an option that directly aligns with their situation or if there are too many options to review.
Another reason closed questions are used in surveys is that they are much easier to answer than open-ended ones. A survey with many open-ended questions will usually have a lower completion rate than one with more closed questions.
Using Closed Questions in Interviews and Usability Tests
Closed questions are used occasionally in interviews and usability tests to get clarification and extra details. They are often used when asking followup questions. For example, a facilitator might ask:
- Has this happened to you before?
- When was the last time this happened?
- Was this a different time than the time you mentioned previously?
Closed questions help facilitators gather important details. However, they should be used sparingly in qualitative research as they can limit what you can learn.
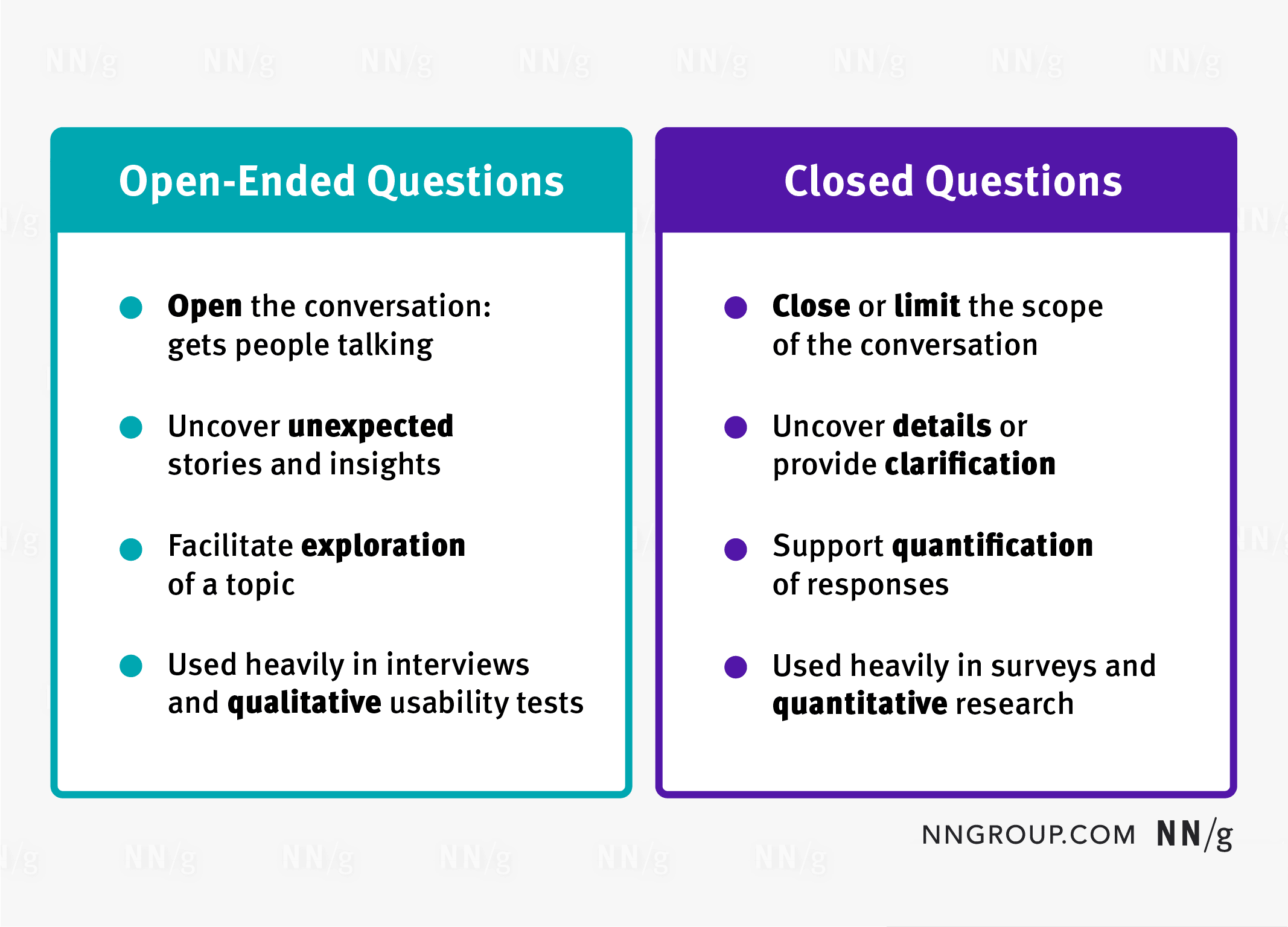
The greatest benefit of open-ended questions is that they allow you to find more than you anticipate. You don’t know what you don’t know. People may share motivations you didn’t expect and mention behaviors and concerns you knew nothing about. When you ask people to explain things, they often reveal surprising mental models , problem-solving strategies, hopes, and fears.
On the other hand, closed questions stop the conversation. If an interviewer or usability-test facilitator were to ask only closed questions, the conversation would be stilted and surface-level. The facilitator might not learn important things they didn’t think to ask because closed questions eliminate surprises: what you expect is what you get.
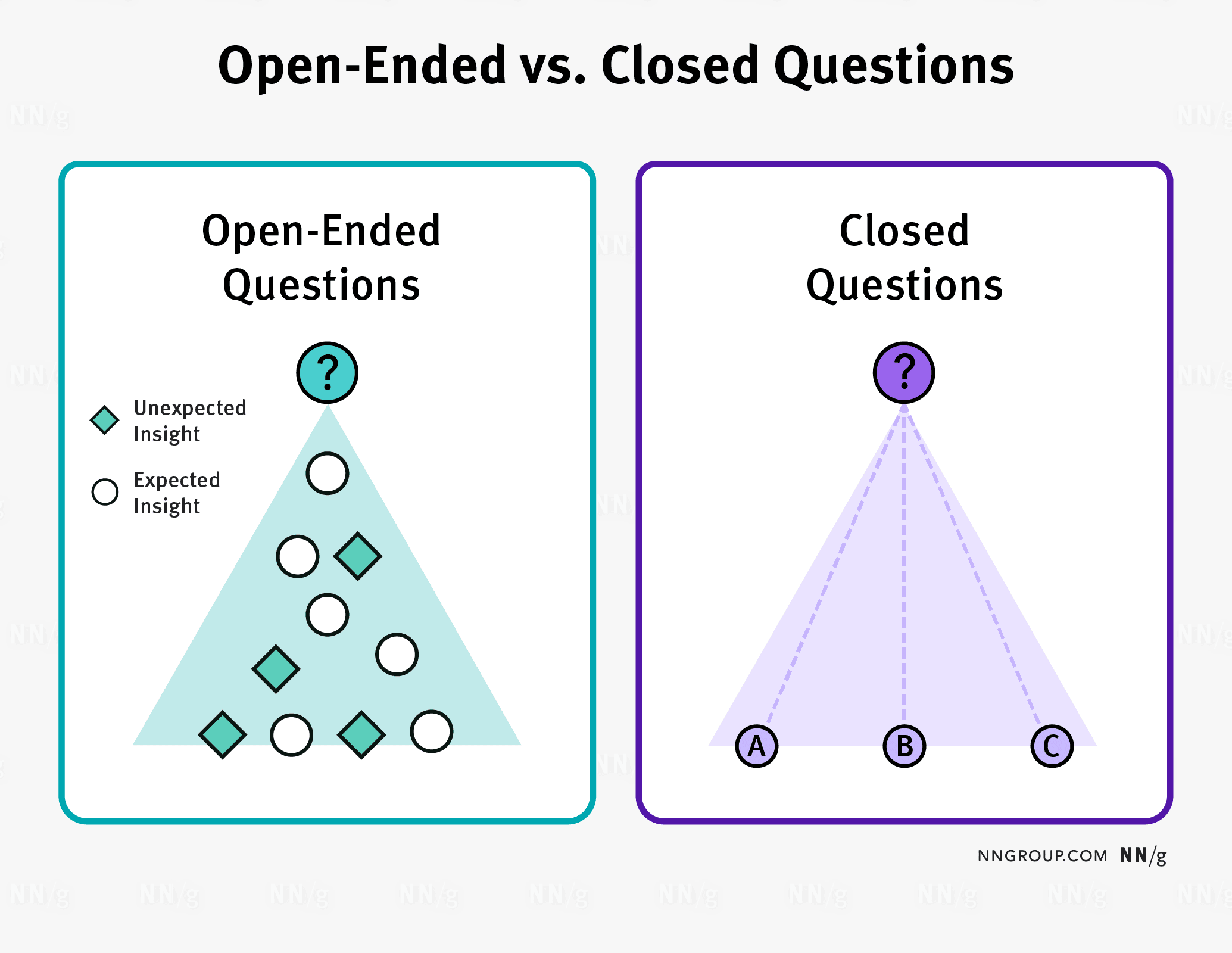

Closed Questions Can Sometimes Be Leading
When you ask closed questions, you may accidentally reveal what you’re interested in and prime participants to volunteer only specific information. This is why researchers use the funnel technique , where the session or followup questions begin with broad, open-ended questions before introducing specific, closed questions.
Not all closed questions are leading. That being said, it’s easy for a closed question to become leading if it suggests an answer.
The table below shows examples of leading closed questions . Reworking a question so it’s not leading often involves making it open-ended, as shown in column 2 of the table below.
One way to spot a leading, closed question is to look at how the question begins. Leading closed questions often start with the words “did,” “was,” or “is.” Open-ended questions often begin with “how” or “what.”
New interviewers and usability-test facilitators often struggle to ask enough open-ended questions. A new interviewer might be tempted to ask many factual, closed questions in quick succession, such as the following:
- Do you have children?
- Do you work?
- How old are you?
- Do you ever [insert behavior]?
However, these questions could be answered in response to a broad, open-ended question like Tell me a bit about yourself .
When constructing an interview guide for a user interview, try to think of a broad, open-ended version of a closed question that might get the participant talking about the question you want answered, like in the example above.
When asking questions in a usability test, try to favor questions that begin with “how,” or “what,” over “do,” or “did” like in the table below.
|
|
Another tip to help you ask open-ended questions is to use one of the following question stems :
- Walk me through [how/what]...
- Tell me a bit about…
- Tell me about a time where…
Finally, you can ask open-ended questions when probing. Probing questions are open-ended and are used in response to what a participant shares. They are designed to solicit more information. You can use the following probing questions in interviews and usability tests.
- Tell me more about that.
- What do you mean by that?
- Can you expand on that?
- What do you think about that?
- Why do you think that?
Ask open-ended questions in conversations with users to discover unanticipated answers and important insights. Use closed questions to gather additional small details, gain clarification, or when you want to analyze responses quantitatively.
Related Topics
- Research Methods Research Methods
Learn More:
Please accept marketing cookies to view the embedded video. https://www.youtube.com/watch?v=LpV3tMy_WZ0
Open vs. Closed Questions in User Research
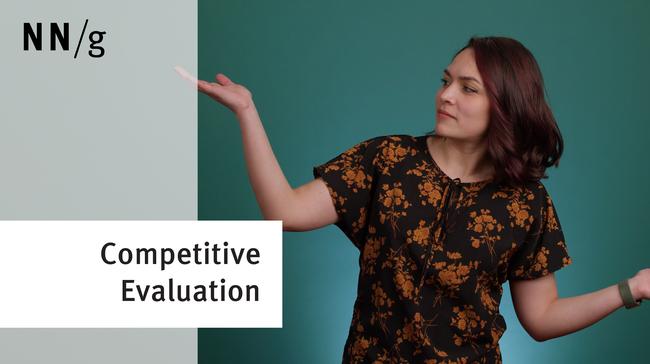
Competitive Reviews vs. Competitive Research
Therese Fessenden · 4 min
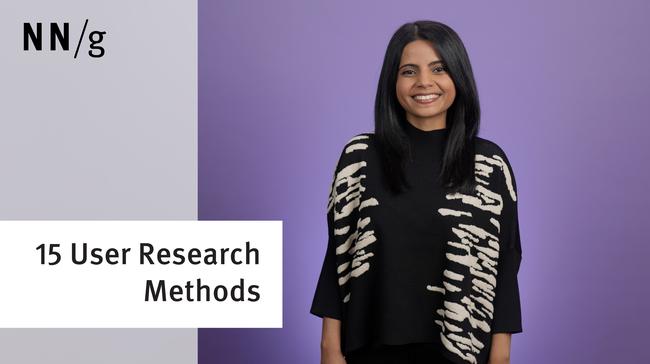
15 User Research Methods to Know Beyond Usability Testing
Samhita Tankala · 3 min
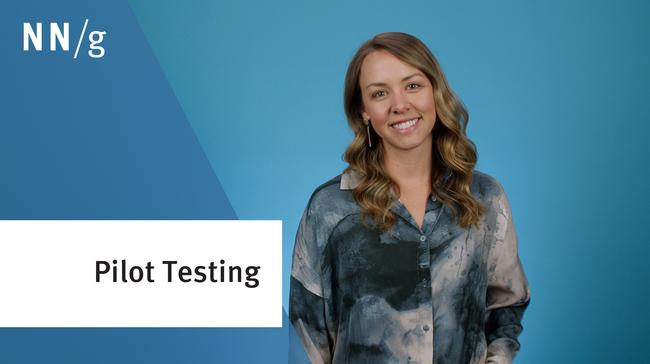
Always Pilot Test User Research Studies
Kim Salazar · 3 min
Related Articles:
Field Studies Done Right: Fast and Observational
Jakob Nielsen · 3 min
Should You Run a Survey?
Maddie Brown · 6 min
The Funnel Technique in Qualitative User Research
Maria Rosala and Kate Moran · 7 min
Card Sorting: Pushing Users Beyond Terminology Matches
Samhita Tankala and Jakob Nielsen · 5 min
Card Sorting: Uncover Users' Mental Models for Better Information Architecture
Samhita Tankala and Katie Sherwin · 11 min
The Diverge-and-Converge Technique for UX Workshops
Therese Fessenden · 6 min
Frequently asked questions
What’s the difference between closed-ended and open-ended questions.
Closed-ended, or restricted-choice, questions offer respondents a fixed set of choices to select from. These questions are easier to answer quickly.
Open-ended or long-form questions allow respondents to answer in their own words. Because there are no restrictions on their choices, respondents can answer in ways that researchers may not have otherwise considered.
Frequently asked questions: Methodology
Attrition refers to participants leaving a study. It always happens to some extent—for example, in randomized controlled trials for medical research.
Differential attrition occurs when attrition or dropout rates differ systematically between the intervention and the control group . As a result, the characteristics of the participants who drop out differ from the characteristics of those who stay in the study. Because of this, study results may be biased .
Action research is conducted in order to solve a particular issue immediately, while case studies are often conducted over a longer period of time and focus more on observing and analyzing a particular ongoing phenomenon.
Action research is focused on solving a problem or informing individual and community-based knowledge in a way that impacts teaching, learning, and other related processes. It is less focused on contributing theoretical input, instead producing actionable input.
Action research is particularly popular with educators as a form of systematic inquiry because it prioritizes reflection and bridges the gap between theory and practice. Educators are able to simultaneously investigate an issue as they solve it, and the method is very iterative and flexible.
A cycle of inquiry is another name for action research . It is usually visualized in a spiral shape following a series of steps, such as “planning → acting → observing → reflecting.”
To make quantitative observations , you need to use instruments that are capable of measuring the quantity you want to observe. For example, you might use a ruler to measure the length of an object or a thermometer to measure its temperature.
Criterion validity and construct validity are both types of measurement validity . In other words, they both show you how accurately a method measures something.
While construct validity is the degree to which a test or other measurement method measures what it claims to measure, criterion validity is the degree to which a test can predictively (in the future) or concurrently (in the present) measure something.
Construct validity is often considered the overarching type of measurement validity . You need to have face validity , content validity , and criterion validity in order to achieve construct validity.
Convergent validity and discriminant validity are both subtypes of construct validity . Together, they help you evaluate whether a test measures the concept it was designed to measure.
- Convergent validity indicates whether a test that is designed to measure a particular construct correlates with other tests that assess the same or similar construct.
- Discriminant validity indicates whether two tests that should not be highly related to each other are indeed not related. This type of validity is also called divergent validity .
You need to assess both in order to demonstrate construct validity. Neither one alone is sufficient for establishing construct validity.
- Discriminant validity indicates whether two tests that should not be highly related to each other are indeed not related
Content validity shows you how accurately a test or other measurement method taps into the various aspects of the specific construct you are researching.
In other words, it helps you answer the question: “does the test measure all aspects of the construct I want to measure?” If it does, then the test has high content validity.
The higher the content validity, the more accurate the measurement of the construct.
If the test fails to include parts of the construct, or irrelevant parts are included, the validity of the instrument is threatened, which brings your results into question.
Face validity and content validity are similar in that they both evaluate how suitable the content of a test is. The difference is that face validity is subjective, and assesses content at surface level.
When a test has strong face validity, anyone would agree that the test’s questions appear to measure what they are intended to measure.
For example, looking at a 4th grade math test consisting of problems in which students have to add and multiply, most people would agree that it has strong face validity (i.e., it looks like a math test).
On the other hand, content validity evaluates how well a test represents all the aspects of a topic. Assessing content validity is more systematic and relies on expert evaluation. of each question, analyzing whether each one covers the aspects that the test was designed to cover.
A 4th grade math test would have high content validity if it covered all the skills taught in that grade. Experts(in this case, math teachers), would have to evaluate the content validity by comparing the test to the learning objectives.
Snowball sampling is a non-probability sampling method . Unlike probability sampling (which involves some form of random selection ), the initial individuals selected to be studied are the ones who recruit new participants.
Because not every member of the target population has an equal chance of being recruited into the sample, selection in snowball sampling is non-random.
Snowball sampling is a non-probability sampling method , where there is not an equal chance for every member of the population to be included in the sample .
This means that you cannot use inferential statistics and make generalizations —often the goal of quantitative research . As such, a snowball sample is not representative of the target population and is usually a better fit for qualitative research .
Snowball sampling relies on the use of referrals. Here, the researcher recruits one or more initial participants, who then recruit the next ones.
Participants share similar characteristics and/or know each other. Because of this, not every member of the population has an equal chance of being included in the sample, giving rise to sampling bias .
Snowball sampling is best used in the following cases:
- If there is no sampling frame available (e.g., people with a rare disease)
- If the population of interest is hard to access or locate (e.g., people experiencing homelessness)
- If the research focuses on a sensitive topic (e.g., extramarital affairs)
The reproducibility and replicability of a study can be ensured by writing a transparent, detailed method section and using clear, unambiguous language.
Reproducibility and replicability are related terms.
- Reproducing research entails reanalyzing the existing data in the same manner.
- Replicating (or repeating ) the research entails reconducting the entire analysis, including the collection of new data .
- A successful reproduction shows that the data analyses were conducted in a fair and honest manner.
- A successful replication shows that the reliability of the results is high.
Stratified sampling and quota sampling both involve dividing the population into subgroups and selecting units from each subgroup. The purpose in both cases is to select a representative sample and/or to allow comparisons between subgroups.
The main difference is that in stratified sampling, you draw a random sample from each subgroup ( probability sampling ). In quota sampling you select a predetermined number or proportion of units, in a non-random manner ( non-probability sampling ).
Purposive and convenience sampling are both sampling methods that are typically used in qualitative data collection.
A convenience sample is drawn from a source that is conveniently accessible to the researcher. Convenience sampling does not distinguish characteristics among the participants. On the other hand, purposive sampling focuses on selecting participants possessing characteristics associated with the research study.
The findings of studies based on either convenience or purposive sampling can only be generalized to the (sub)population from which the sample is drawn, and not to the entire population.
Random sampling or probability sampling is based on random selection. This means that each unit has an equal chance (i.e., equal probability) of being included in the sample.
On the other hand, convenience sampling involves stopping people at random, which means that not everyone has an equal chance of being selected depending on the place, time, or day you are collecting your data.
Convenience sampling and quota sampling are both non-probability sampling methods. They both use non-random criteria like availability, geographical proximity, or expert knowledge to recruit study participants.
However, in convenience sampling, you continue to sample units or cases until you reach the required sample size.
In quota sampling, you first need to divide your population of interest into subgroups (strata) and estimate their proportions (quota) in the population. Then you can start your data collection, using convenience sampling to recruit participants, until the proportions in each subgroup coincide with the estimated proportions in the population.
A sampling frame is a list of every member in the entire population . It is important that the sampling frame is as complete as possible, so that your sample accurately reflects your population.
Stratified and cluster sampling may look similar, but bear in mind that groups created in cluster sampling are heterogeneous , so the individual characteristics in the cluster vary. In contrast, groups created in stratified sampling are homogeneous , as units share characteristics.
Relatedly, in cluster sampling you randomly select entire groups and include all units of each group in your sample. However, in stratified sampling, you select some units of all groups and include them in your sample. In this way, both methods can ensure that your sample is representative of the target population .
A systematic review is secondary research because it uses existing research. You don’t collect new data yourself.
The key difference between observational studies and experimental designs is that a well-done observational study does not influence the responses of participants, while experiments do have some sort of treatment condition applied to at least some participants by random assignment .
An observational study is a great choice for you if your research question is based purely on observations. If there are ethical, logistical, or practical concerns that prevent you from conducting a traditional experiment , an observational study may be a good choice. In an observational study, there is no interference or manipulation of the research subjects, as well as no control or treatment groups .
It’s often best to ask a variety of people to review your measurements. You can ask experts, such as other researchers, or laypeople, such as potential participants, to judge the face validity of tests.
While experts have a deep understanding of research methods , the people you’re studying can provide you with valuable insights you may have missed otherwise.
Face validity is important because it’s a simple first step to measuring the overall validity of a test or technique. It’s a relatively intuitive, quick, and easy way to start checking whether a new measure seems useful at first glance.
Good face validity means that anyone who reviews your measure says that it seems to be measuring what it’s supposed to. With poor face validity, someone reviewing your measure may be left confused about what you’re measuring and why you’re using this method.
Face validity is about whether a test appears to measure what it’s supposed to measure. This type of validity is concerned with whether a measure seems relevant and appropriate for what it’s assessing only on the surface.
Statistical analyses are often applied to test validity with data from your measures. You test convergent validity and discriminant validity with correlations to see if results from your test are positively or negatively related to those of other established tests.
You can also use regression analyses to assess whether your measure is actually predictive of outcomes that you expect it to predict theoretically. A regression analysis that supports your expectations strengthens your claim of construct validity .
When designing or evaluating a measure, construct validity helps you ensure you’re actually measuring the construct you’re interested in. If you don’t have construct validity, you may inadvertently measure unrelated or distinct constructs and lose precision in your research.
Construct validity is often considered the overarching type of measurement validity , because it covers all of the other types. You need to have face validity , content validity , and criterion validity to achieve construct validity.
Construct validity is about how well a test measures the concept it was designed to evaluate. It’s one of four types of measurement validity , which includes construct validity, face validity , and criterion validity.
There are two subtypes of construct validity.
- Convergent validity : The extent to which your measure corresponds to measures of related constructs
- Discriminant validity : The extent to which your measure is unrelated or negatively related to measures of distinct constructs
Naturalistic observation is a valuable tool because of its flexibility, external validity , and suitability for topics that can’t be studied in a lab setting.
The downsides of naturalistic observation include its lack of scientific control , ethical considerations , and potential for bias from observers and subjects.
Naturalistic observation is a qualitative research method where you record the behaviors of your research subjects in real world settings. You avoid interfering or influencing anything in a naturalistic observation.
You can think of naturalistic observation as “people watching” with a purpose.
A dependent variable is what changes as a result of the independent variable manipulation in experiments . It’s what you’re interested in measuring, and it “depends” on your independent variable.
In statistics, dependent variables are also called:
- Response variables (they respond to a change in another variable)
- Outcome variables (they represent the outcome you want to measure)
- Left-hand-side variables (they appear on the left-hand side of a regression equation)
An independent variable is the variable you manipulate, control, or vary in an experimental study to explore its effects. It’s called “independent” because it’s not influenced by any other variables in the study.
Independent variables are also called:
- Explanatory variables (they explain an event or outcome)
- Predictor variables (they can be used to predict the value of a dependent variable)
- Right-hand-side variables (they appear on the right-hand side of a regression equation).
As a rule of thumb, questions related to thoughts, beliefs, and feelings work well in focus groups. Take your time formulating strong questions, paying special attention to phrasing. Be careful to avoid leading questions , which can bias your responses.
Overall, your focus group questions should be:
- Open-ended and flexible
- Impossible to answer with “yes” or “no” (questions that start with “why” or “how” are often best)
- Unambiguous, getting straight to the point while still stimulating discussion
- Unbiased and neutral
A structured interview is a data collection method that relies on asking questions in a set order to collect data on a topic. They are often quantitative in nature. Structured interviews are best used when:
- You already have a very clear understanding of your topic. Perhaps significant research has already been conducted, or you have done some prior research yourself, but you already possess a baseline for designing strong structured questions.
- You are constrained in terms of time or resources and need to analyze your data quickly and efficiently.
- Your research question depends on strong parity between participants, with environmental conditions held constant.
More flexible interview options include semi-structured interviews , unstructured interviews , and focus groups .
Social desirability bias is the tendency for interview participants to give responses that will be viewed favorably by the interviewer or other participants. It occurs in all types of interviews and surveys , but is most common in semi-structured interviews , unstructured interviews , and focus groups .
Social desirability bias can be mitigated by ensuring participants feel at ease and comfortable sharing their views. Make sure to pay attention to your own body language and any physical or verbal cues, such as nodding or widening your eyes.
This type of bias can also occur in observations if the participants know they’re being observed. They might alter their behavior accordingly.
The interviewer effect is a type of bias that emerges when a characteristic of an interviewer (race, age, gender identity, etc.) influences the responses given by the interviewee.
There is a risk of an interviewer effect in all types of interviews , but it can be mitigated by writing really high-quality interview questions.
A semi-structured interview is a blend of structured and unstructured types of interviews. Semi-structured interviews are best used when:
- You have prior interview experience. Spontaneous questions are deceptively challenging, and it’s easy to accidentally ask a leading question or make a participant uncomfortable.
- Your research question is exploratory in nature. Participant answers can guide future research questions and help you develop a more robust knowledge base for future research.
An unstructured interview is the most flexible type of interview, but it is not always the best fit for your research topic.
Unstructured interviews are best used when:
- You are an experienced interviewer and have a very strong background in your research topic, since it is challenging to ask spontaneous, colloquial questions.
- Your research question is exploratory in nature. While you may have developed hypotheses, you are open to discovering new or shifting viewpoints through the interview process.
- You are seeking descriptive data, and are ready to ask questions that will deepen and contextualize your initial thoughts and hypotheses.
- Your research depends on forming connections with your participants and making them feel comfortable revealing deeper emotions, lived experiences, or thoughts.
The four most common types of interviews are:
- Structured interviews : The questions are predetermined in both topic and order.
- Semi-structured interviews : A few questions are predetermined, but other questions aren’t planned.
- Unstructured interviews : None of the questions are predetermined.
- Focus group interviews : The questions are presented to a group instead of one individual.
Deductive reasoning is commonly used in scientific research, and it’s especially associated with quantitative research .
In research, you might have come across something called the hypothetico-deductive method . It’s the scientific method of testing hypotheses to check whether your predictions are substantiated by real-world data.
Deductive reasoning is a logical approach where you progress from general ideas to specific conclusions. It’s often contrasted with inductive reasoning , where you start with specific observations and form general conclusions.
Deductive reasoning is also called deductive logic.
There are many different types of inductive reasoning that people use formally or informally.
Here are a few common types:
- Inductive generalization : You use observations about a sample to come to a conclusion about the population it came from.
- Statistical generalization: You use specific numbers about samples to make statements about populations.
- Causal reasoning: You make cause-and-effect links between different things.
- Sign reasoning: You make a conclusion about a correlational relationship between different things.
- Analogical reasoning: You make a conclusion about something based on its similarities to something else.
Inductive reasoning is a bottom-up approach, while deductive reasoning is top-down.
Inductive reasoning takes you from the specific to the general, while in deductive reasoning, you make inferences by going from general premises to specific conclusions.
In inductive research , you start by making observations or gathering data. Then, you take a broad scan of your data and search for patterns. Finally, you make general conclusions that you might incorporate into theories.
Inductive reasoning is a method of drawing conclusions by going from the specific to the general. It’s usually contrasted with deductive reasoning, where you proceed from general information to specific conclusions.
Inductive reasoning is also called inductive logic or bottom-up reasoning.
A hypothesis states your predictions about what your research will find. It is a tentative answer to your research question that has not yet been tested. For some research projects, you might have to write several hypotheses that address different aspects of your research question.
A hypothesis is not just a guess — it should be based on existing theories and knowledge. It also has to be testable, which means you can support or refute it through scientific research methods (such as experiments, observations and statistical analysis of data).
Triangulation can help:
- Reduce research bias that comes from using a single method, theory, or investigator
- Enhance validity by approaching the same topic with different tools
- Establish credibility by giving you a complete picture of the research problem
But triangulation can also pose problems:
- It’s time-consuming and labor-intensive, often involving an interdisciplinary team.
- Your results may be inconsistent or even contradictory.
There are four main types of triangulation :
- Data triangulation : Using data from different times, spaces, and people
- Investigator triangulation : Involving multiple researchers in collecting or analyzing data
- Theory triangulation : Using varying theoretical perspectives in your research
- Methodological triangulation : Using different methodologies to approach the same topic
Many academic fields use peer review , largely to determine whether a manuscript is suitable for publication. Peer review enhances the credibility of the published manuscript.
However, peer review is also common in non-academic settings. The United Nations, the European Union, and many individual nations use peer review to evaluate grant applications. It is also widely used in medical and health-related fields as a teaching or quality-of-care measure.
Peer assessment is often used in the classroom as a pedagogical tool. Both receiving feedback and providing it are thought to enhance the learning process, helping students think critically and collaboratively.
Peer review can stop obviously problematic, falsified, or otherwise untrustworthy research from being published. It also represents an excellent opportunity to get feedback from renowned experts in your field. It acts as a first defense, helping you ensure your argument is clear and that there are no gaps, vague terms, or unanswered questions for readers who weren’t involved in the research process.
Peer-reviewed articles are considered a highly credible source due to this stringent process they go through before publication.
In general, the peer review process follows the following steps:
- First, the author submits the manuscript to the editor.
- Reject the manuscript and send it back to author, or
- Send it onward to the selected peer reviewer(s)
- Next, the peer review process occurs. The reviewer provides feedback, addressing any major or minor issues with the manuscript, and gives their advice regarding what edits should be made.
- Lastly, the edited manuscript is sent back to the author. They input the edits, and resubmit it to the editor for publication.
Exploratory research is often used when the issue you’re studying is new or when the data collection process is challenging for some reason.
You can use exploratory research if you have a general idea or a specific question that you want to study but there is no preexisting knowledge or paradigm with which to study it.
Exploratory research is a methodology approach that explores research questions that have not previously been studied in depth. It is often used when the issue you’re studying is new, or the data collection process is challenging in some way.
Explanatory research is used to investigate how or why a phenomenon occurs. Therefore, this type of research is often one of the first stages in the research process , serving as a jumping-off point for future research.
Exploratory research aims to explore the main aspects of an under-researched problem, while explanatory research aims to explain the causes and consequences of a well-defined problem.
Explanatory research is a research method used to investigate how or why something occurs when only a small amount of information is available pertaining to that topic. It can help you increase your understanding of a given topic.
Clean data are valid, accurate, complete, consistent, unique, and uniform. Dirty data include inconsistencies and errors.
Dirty data can come from any part of the research process, including poor research design , inappropriate measurement materials, or flawed data entry.
Data cleaning takes place between data collection and data analyses. But you can use some methods even before collecting data.
For clean data, you should start by designing measures that collect valid data. Data validation at the time of data entry or collection helps you minimize the amount of data cleaning you’ll need to do.
After data collection, you can use data standardization and data transformation to clean your data. You’ll also deal with any missing values, outliers, and duplicate values.
Every dataset requires different techniques to clean dirty data , but you need to address these issues in a systematic way. You focus on finding and resolving data points that don’t agree or fit with the rest of your dataset.
These data might be missing values, outliers, duplicate values, incorrectly formatted, or irrelevant. You’ll start with screening and diagnosing your data. Then, you’ll often standardize and accept or remove data to make your dataset consistent and valid.
Data cleaning is necessary for valid and appropriate analyses. Dirty data contain inconsistencies or errors , but cleaning your data helps you minimize or resolve these.
Without data cleaning, you could end up with a Type I or II error in your conclusion. These types of erroneous conclusions can be practically significant with important consequences, because they lead to misplaced investments or missed opportunities.
Data cleaning involves spotting and resolving potential data inconsistencies or errors to improve your data quality. An error is any value (e.g., recorded weight) that doesn’t reflect the true value (e.g., actual weight) of something that’s being measured.
In this process, you review, analyze, detect, modify, or remove “dirty” data to make your dataset “clean.” Data cleaning is also called data cleansing or data scrubbing.
Research misconduct means making up or falsifying data, manipulating data analyses, or misrepresenting results in research reports. It’s a form of academic fraud.
These actions are committed intentionally and can have serious consequences; research misconduct is not a simple mistake or a point of disagreement but a serious ethical failure.
Anonymity means you don’t know who the participants are, while confidentiality means you know who they are but remove identifying information from your research report. Both are important ethical considerations .
You can only guarantee anonymity by not collecting any personally identifying information—for example, names, phone numbers, email addresses, IP addresses, physical characteristics, photos, or videos.
You can keep data confidential by using aggregate information in your research report, so that you only refer to groups of participants rather than individuals.
Research ethics matter for scientific integrity, human rights and dignity, and collaboration between science and society. These principles make sure that participation in studies is voluntary, informed, and safe.
Ethical considerations in research are a set of principles that guide your research designs and practices. These principles include voluntary participation, informed consent, anonymity, confidentiality, potential for harm, and results communication.
Scientists and researchers must always adhere to a certain code of conduct when collecting data from others .
These considerations protect the rights of research participants, enhance research validity , and maintain scientific integrity.
In multistage sampling , you can use probability or non-probability sampling methods .
For a probability sample, you have to conduct probability sampling at every stage.
You can mix it up by using simple random sampling , systematic sampling , or stratified sampling to select units at different stages, depending on what is applicable and relevant to your study.
Multistage sampling can simplify data collection when you have large, geographically spread samples, and you can obtain a probability sample without a complete sampling frame.
But multistage sampling may not lead to a representative sample, and larger samples are needed for multistage samples to achieve the statistical properties of simple random samples .
These are four of the most common mixed methods designs :
- Convergent parallel: Quantitative and qualitative data are collected at the same time and analyzed separately. After both analyses are complete, compare your results to draw overall conclusions.
- Embedded: Quantitative and qualitative data are collected at the same time, but within a larger quantitative or qualitative design. One type of data is secondary to the other.
- Explanatory sequential: Quantitative data is collected and analyzed first, followed by qualitative data. You can use this design if you think your qualitative data will explain and contextualize your quantitative findings.
- Exploratory sequential: Qualitative data is collected and analyzed first, followed by quantitative data. You can use this design if you think the quantitative data will confirm or validate your qualitative findings.
Triangulation in research means using multiple datasets, methods, theories and/or investigators to address a research question. It’s a research strategy that can help you enhance the validity and credibility of your findings.
Triangulation is mainly used in qualitative research , but it’s also commonly applied in quantitative research . Mixed methods research always uses triangulation.
In multistage sampling , or multistage cluster sampling, you draw a sample from a population using smaller and smaller groups at each stage.
This method is often used to collect data from a large, geographically spread group of people in national surveys, for example. You take advantage of hierarchical groupings (e.g., from state to city to neighborhood) to create a sample that’s less expensive and time-consuming to collect data from.
No, the steepness or slope of the line isn’t related to the correlation coefficient value. The correlation coefficient only tells you how closely your data fit on a line, so two datasets with the same correlation coefficient can have very different slopes.
To find the slope of the line, you’ll need to perform a regression analysis .
Correlation coefficients always range between -1 and 1.
The sign of the coefficient tells you the direction of the relationship: a positive value means the variables change together in the same direction, while a negative value means they change together in opposite directions.
The absolute value of a number is equal to the number without its sign. The absolute value of a correlation coefficient tells you the magnitude of the correlation: the greater the absolute value, the stronger the correlation.
These are the assumptions your data must meet if you want to use Pearson’s r :
- Both variables are on an interval or ratio level of measurement
- Data from both variables follow normal distributions
- Your data have no outliers
- Your data is from a random or representative sample
- You expect a linear relationship between the two variables
Quantitative research designs can be divided into two main categories:
- Correlational and descriptive designs are used to investigate characteristics, averages, trends, and associations between variables.
- Experimental and quasi-experimental designs are used to test causal relationships .
Qualitative research designs tend to be more flexible. Common types of qualitative design include case study , ethnography , and grounded theory designs.
A well-planned research design helps ensure that your methods match your research aims, that you collect high-quality data, and that you use the right kind of analysis to answer your questions, utilizing credible sources . This allows you to draw valid , trustworthy conclusions.
The priorities of a research design can vary depending on the field, but you usually have to specify:
- Your research questions and/or hypotheses
- Your overall approach (e.g., qualitative or quantitative )
- The type of design you’re using (e.g., a survey , experiment , or case study )
- Your sampling methods or criteria for selecting subjects
- Your data collection methods (e.g., questionnaires , observations)
- Your data collection procedures (e.g., operationalization , timing and data management)
- Your data analysis methods (e.g., statistical tests or thematic analysis )
A research design is a strategy for answering your research question . It defines your overall approach and determines how you will collect and analyze data.
Questionnaires can be self-administered or researcher-administered.
Self-administered questionnaires can be delivered online or in paper-and-pen formats, in person or through mail. All questions are standardized so that all respondents receive the same questions with identical wording.
Researcher-administered questionnaires are interviews that take place by phone, in-person, or online between researchers and respondents. You can gain deeper insights by clarifying questions for respondents or asking follow-up questions.
You can organize the questions logically, with a clear progression from simple to complex, or randomly between respondents. A logical flow helps respondents process the questionnaire easier and quicker, but it may lead to bias. Randomization can minimize the bias from order effects.
A questionnaire is a data collection tool or instrument, while a survey is an overarching research method that involves collecting and analyzing data from people using questionnaires.
The third variable and directionality problems are two main reasons why correlation isn’t causation .
The third variable problem means that a confounding variable affects both variables to make them seem causally related when they are not.
The directionality problem is when two variables correlate and might actually have a causal relationship, but it’s impossible to conclude which variable causes changes in the other.
Correlation describes an association between variables : when one variable changes, so does the other. A correlation is a statistical indicator of the relationship between variables.
Causation means that changes in one variable brings about changes in the other (i.e., there is a cause-and-effect relationship between variables). The two variables are correlated with each other, and there’s also a causal link between them.
While causation and correlation can exist simultaneously, correlation does not imply causation. In other words, correlation is simply a relationship where A relates to B—but A doesn’t necessarily cause B to happen (or vice versa). Mistaking correlation for causation is a common error and can lead to false cause fallacy .
Controlled experiments establish causality, whereas correlational studies only show associations between variables.
- In an experimental design , you manipulate an independent variable and measure its effect on a dependent variable. Other variables are controlled so they can’t impact the results.
- In a correlational design , you measure variables without manipulating any of them. You can test whether your variables change together, but you can’t be sure that one variable caused a change in another.
In general, correlational research is high in external validity while experimental research is high in internal validity .
A correlation is usually tested for two variables at a time, but you can test correlations between three or more variables.
A correlation coefficient is a single number that describes the strength and direction of the relationship between your variables.
Different types of correlation coefficients might be appropriate for your data based on their levels of measurement and distributions . The Pearson product-moment correlation coefficient (Pearson’s r ) is commonly used to assess a linear relationship between two quantitative variables.
A correlational research design investigates relationships between two variables (or more) without the researcher controlling or manipulating any of them. It’s a non-experimental type of quantitative research .
A correlation reflects the strength and/or direction of the association between two or more variables.
- A positive correlation means that both variables change in the same direction.
- A negative correlation means that the variables change in opposite directions.
- A zero correlation means there’s no relationship between the variables.
Random error is almost always present in scientific studies, even in highly controlled settings. While you can’t eradicate it completely, you can reduce random error by taking repeated measurements, using a large sample, and controlling extraneous variables .
You can avoid systematic error through careful design of your sampling , data collection , and analysis procedures. For example, use triangulation to measure your variables using multiple methods; regularly calibrate instruments or procedures; use random sampling and random assignment ; and apply masking (blinding) where possible.
Systematic error is generally a bigger problem in research.
With random error, multiple measurements will tend to cluster around the true value. When you’re collecting data from a large sample , the errors in different directions will cancel each other out.
Systematic errors are much more problematic because they can skew your data away from the true value. This can lead you to false conclusions ( Type I and II errors ) about the relationship between the variables you’re studying.
Random and systematic error are two types of measurement error.
Random error is a chance difference between the observed and true values of something (e.g., a researcher misreading a weighing scale records an incorrect measurement).
Systematic error is a consistent or proportional difference between the observed and true values of something (e.g., a miscalibrated scale consistently records weights as higher than they actually are).
On graphs, the explanatory variable is conventionally placed on the x-axis, while the response variable is placed on the y-axis.
- If you have quantitative variables , use a scatterplot or a line graph.
- If your response variable is categorical, use a scatterplot or a line graph.
- If your explanatory variable is categorical, use a bar graph.
The term “ explanatory variable ” is sometimes preferred over “ independent variable ” because, in real world contexts, independent variables are often influenced by other variables. This means they aren’t totally independent.
Multiple independent variables may also be correlated with each other, so “explanatory variables” is a more appropriate term.
The difference between explanatory and response variables is simple:
- An explanatory variable is the expected cause, and it explains the results.
- A response variable is the expected effect, and it responds to other variables.
In a controlled experiment , all extraneous variables are held constant so that they can’t influence the results. Controlled experiments require:
- A control group that receives a standard treatment, a fake treatment, or no treatment.
- Random assignment of participants to ensure the groups are equivalent.
Depending on your study topic, there are various other methods of controlling variables .
There are 4 main types of extraneous variables :
- Demand characteristics : environmental cues that encourage participants to conform to researchers’ expectations.
- Experimenter effects : unintentional actions by researchers that influence study outcomes.
- Situational variables : environmental variables that alter participants’ behaviors.
- Participant variables : any characteristic or aspect of a participant’s background that could affect study results.
An extraneous variable is any variable that you’re not investigating that can potentially affect the dependent variable of your research study.
A confounding variable is a type of extraneous variable that not only affects the dependent variable, but is also related to the independent variable.
In a factorial design, multiple independent variables are tested.
If you test two variables, each level of one independent variable is combined with each level of the other independent variable to create different conditions.
Within-subjects designs have many potential threats to internal validity , but they are also very statistically powerful .
Advantages:
- Only requires small samples
- Statistically powerful
- Removes the effects of individual differences on the outcomes
Disadvantages:
- Internal validity threats reduce the likelihood of establishing a direct relationship between variables
- Time-related effects, such as growth, can influence the outcomes
- Carryover effects mean that the specific order of different treatments affect the outcomes
While a between-subjects design has fewer threats to internal validity , it also requires more participants for high statistical power than a within-subjects design .
- Prevents carryover effects of learning and fatigue.
- Shorter study duration.
- Needs larger samples for high power.
- Uses more resources to recruit participants, administer sessions, cover costs, etc.
- Individual differences may be an alternative explanation for results.
Yes. Between-subjects and within-subjects designs can be combined in a single study when you have two or more independent variables (a factorial design). In a mixed factorial design, one variable is altered between subjects and another is altered within subjects.
In a between-subjects design , every participant experiences only one condition, and researchers assess group differences between participants in various conditions.
In a within-subjects design , each participant experiences all conditions, and researchers test the same participants repeatedly for differences between conditions.
The word “between” means that you’re comparing different conditions between groups, while the word “within” means you’re comparing different conditions within the same group.
Random assignment is used in experiments with a between-groups or independent measures design. In this research design, there’s usually a control group and one or more experimental groups. Random assignment helps ensure that the groups are comparable.
In general, you should always use random assignment in this type of experimental design when it is ethically possible and makes sense for your study topic.
To implement random assignment , assign a unique number to every member of your study’s sample .
Then, you can use a random number generator or a lottery method to randomly assign each number to a control or experimental group. You can also do so manually, by flipping a coin or rolling a dice to randomly assign participants to groups.
Random selection, or random sampling , is a way of selecting members of a population for your study’s sample.
In contrast, random assignment is a way of sorting the sample into control and experimental groups.
Random sampling enhances the external validity or generalizability of your results, while random assignment improves the internal validity of your study.
In experimental research, random assignment is a way of placing participants from your sample into different groups using randomization. With this method, every member of the sample has a known or equal chance of being placed in a control group or an experimental group.
“Controlling for a variable” means measuring extraneous variables and accounting for them statistically to remove their effects on other variables.
Researchers often model control variable data along with independent and dependent variable data in regression analyses and ANCOVAs . That way, you can isolate the control variable’s effects from the relationship between the variables of interest.
Control variables help you establish a correlational or causal relationship between variables by enhancing internal validity .
If you don’t control relevant extraneous variables , they may influence the outcomes of your study, and you may not be able to demonstrate that your results are really an effect of your independent variable .
A control variable is any variable that’s held constant in a research study. It’s not a variable of interest in the study, but it’s controlled because it could influence the outcomes.
Including mediators and moderators in your research helps you go beyond studying a simple relationship between two variables for a fuller picture of the real world. They are important to consider when studying complex correlational or causal relationships.
Mediators are part of the causal pathway of an effect, and they tell you how or why an effect takes place. Moderators usually help you judge the external validity of your study by identifying the limitations of when the relationship between variables holds.
If something is a mediating variable :
- It’s caused by the independent variable .
- It influences the dependent variable
- When it’s taken into account, the statistical correlation between the independent and dependent variables is higher than when it isn’t considered.
A confounder is a third variable that affects variables of interest and makes them seem related when they are not. In contrast, a mediator is the mechanism of a relationship between two variables: it explains the process by which they are related.
A mediator variable explains the process through which two variables are related, while a moderator variable affects the strength and direction of that relationship.
There are three key steps in systematic sampling :
- Define and list your population , ensuring that it is not ordered in a cyclical or periodic order.
- Decide on your sample size and calculate your interval, k , by dividing your population by your target sample size.
- Choose every k th member of the population as your sample.
Systematic sampling is a probability sampling method where researchers select members of the population at a regular interval – for example, by selecting every 15th person on a list of the population. If the population is in a random order, this can imitate the benefits of simple random sampling .
Yes, you can create a stratified sample using multiple characteristics, but you must ensure that every participant in your study belongs to one and only one subgroup. In this case, you multiply the numbers of subgroups for each characteristic to get the total number of groups.
For example, if you were stratifying by location with three subgroups (urban, rural, or suburban) and marital status with five subgroups (single, divorced, widowed, married, or partnered), you would have 3 x 5 = 15 subgroups.
You should use stratified sampling when your sample can be divided into mutually exclusive and exhaustive subgroups that you believe will take on different mean values for the variable that you’re studying.
Using stratified sampling will allow you to obtain more precise (with lower variance ) statistical estimates of whatever you are trying to measure.
For example, say you want to investigate how income differs based on educational attainment, but you know that this relationship can vary based on race. Using stratified sampling, you can ensure you obtain a large enough sample from each racial group, allowing you to draw more precise conclusions.
In stratified sampling , researchers divide subjects into subgroups called strata based on characteristics that they share (e.g., race, gender, educational attainment).
Once divided, each subgroup is randomly sampled using another probability sampling method.
Cluster sampling is more time- and cost-efficient than other probability sampling methods , particularly when it comes to large samples spread across a wide geographical area.
However, it provides less statistical certainty than other methods, such as simple random sampling , because it is difficult to ensure that your clusters properly represent the population as a whole.
There are three types of cluster sampling : single-stage, double-stage and multi-stage clustering. In all three types, you first divide the population into clusters, then randomly select clusters for use in your sample.
- In single-stage sampling , you collect data from every unit within the selected clusters.
- In double-stage sampling , you select a random sample of units from within the clusters.
- In multi-stage sampling , you repeat the procedure of randomly sampling elements from within the clusters until you have reached a manageable sample.
Cluster sampling is a probability sampling method in which you divide a population into clusters, such as districts or schools, and then randomly select some of these clusters as your sample.
The clusters should ideally each be mini-representations of the population as a whole.
If properly implemented, simple random sampling is usually the best sampling method for ensuring both internal and external validity . However, it can sometimes be impractical and expensive to implement, depending on the size of the population to be studied,
If you have a list of every member of the population and the ability to reach whichever members are selected, you can use simple random sampling.
The American Community Survey is an example of simple random sampling . In order to collect detailed data on the population of the US, the Census Bureau officials randomly select 3.5 million households per year and use a variety of methods to convince them to fill out the survey.
Simple random sampling is a type of probability sampling in which the researcher randomly selects a subset of participants from a population . Each member of the population has an equal chance of being selected. Data is then collected from as large a percentage as possible of this random subset.
Quasi-experimental design is most useful in situations where it would be unethical or impractical to run a true experiment .
Quasi-experiments have lower internal validity than true experiments, but they often have higher external validity as they can use real-world interventions instead of artificial laboratory settings.
A quasi-experiment is a type of research design that attempts to establish a cause-and-effect relationship. The main difference with a true experiment is that the groups are not randomly assigned.
Blinding is important to reduce research bias (e.g., observer bias , demand characteristics ) and ensure a study’s internal validity .
If participants know whether they are in a control or treatment group , they may adjust their behavior in ways that affect the outcome that researchers are trying to measure. If the people administering the treatment are aware of group assignment, they may treat participants differently and thus directly or indirectly influence the final results.
- In a single-blind study , only the participants are blinded.
- In a double-blind study , both participants and experimenters are blinded.
- In a triple-blind study , the assignment is hidden not only from participants and experimenters, but also from the researchers analyzing the data.
Blinding means hiding who is assigned to the treatment group and who is assigned to the control group in an experiment .
A true experiment (a.k.a. a controlled experiment) always includes at least one control group that doesn’t receive the experimental treatment.
However, some experiments use a within-subjects design to test treatments without a control group. In these designs, you usually compare one group’s outcomes before and after a treatment (instead of comparing outcomes between different groups).
For strong internal validity , it’s usually best to include a control group if possible. Without a control group, it’s harder to be certain that the outcome was caused by the experimental treatment and not by other variables.
An experimental group, also known as a treatment group, receives the treatment whose effect researchers wish to study, whereas a control group does not. They should be identical in all other ways.
Individual Likert-type questions are generally considered ordinal data , because the items have clear rank order, but don’t have an even distribution.
Overall Likert scale scores are sometimes treated as interval data. These scores are considered to have directionality and even spacing between them.
The type of data determines what statistical tests you should use to analyze your data.
A Likert scale is a rating scale that quantitatively assesses opinions, attitudes, or behaviors. It is made up of 4 or more questions that measure a single attitude or trait when response scores are combined.
To use a Likert scale in a survey , you present participants with Likert-type questions or statements, and a continuum of items, usually with 5 or 7 possible responses, to capture their degree of agreement.
In scientific research, concepts are the abstract ideas or phenomena that are being studied (e.g., educational achievement). Variables are properties or characteristics of the concept (e.g., performance at school), while indicators are ways of measuring or quantifying variables (e.g., yearly grade reports).
The process of turning abstract concepts into measurable variables and indicators is called operationalization .
There are various approaches to qualitative data analysis , but they all share five steps in common:
- Prepare and organize your data.
- Review and explore your data.
- Develop a data coding system.
- Assign codes to the data.
- Identify recurring themes.
The specifics of each step depend on the focus of the analysis. Some common approaches include textual analysis , thematic analysis , and discourse analysis .
There are five common approaches to qualitative research :
- Grounded theory involves collecting data in order to develop new theories.
- Ethnography involves immersing yourself in a group or organization to understand its culture.
- Narrative research involves interpreting stories to understand how people make sense of their experiences and perceptions.
- Phenomenological research involves investigating phenomena through people’s lived experiences.
- Action research links theory and practice in several cycles to drive innovative changes.
Hypothesis testing is a formal procedure for investigating our ideas about the world using statistics. It is used by scientists to test specific predictions, called hypotheses , by calculating how likely it is that a pattern or relationship between variables could have arisen by chance.
Operationalization means turning abstract conceptual ideas into measurable observations.
For example, the concept of social anxiety isn’t directly observable, but it can be operationally defined in terms of self-rating scores, behavioral avoidance of crowded places, or physical anxiety symptoms in social situations.
Before collecting data , it’s important to consider how you will operationalize the variables that you want to measure.
When conducting research, collecting original data has significant advantages:
- You can tailor data collection to your specific research aims (e.g. understanding the needs of your consumers or user testing your website)
- You can control and standardize the process for high reliability and validity (e.g. choosing appropriate measurements and sampling methods )
However, there are also some drawbacks: data collection can be time-consuming, labor-intensive and expensive. In some cases, it’s more efficient to use secondary data that has already been collected by someone else, but the data might be less reliable.
Data collection is the systematic process by which observations or measurements are gathered in research. It is used in many different contexts by academics, governments, businesses, and other organizations.
There are several methods you can use to decrease the impact of confounding variables on your research: restriction, matching, statistical control and randomization.
In restriction , you restrict your sample by only including certain subjects that have the same values of potential confounding variables.
In matching , you match each of the subjects in your treatment group with a counterpart in the comparison group. The matched subjects have the same values on any potential confounding variables, and only differ in the independent variable .
In statistical control , you include potential confounders as variables in your regression .
In randomization , you randomly assign the treatment (or independent variable) in your study to a sufficiently large number of subjects, which allows you to control for all potential confounding variables.
A confounding variable is closely related to both the independent and dependent variables in a study. An independent variable represents the supposed cause , while the dependent variable is the supposed effect . A confounding variable is a third variable that influences both the independent and dependent variables.
Failing to account for confounding variables can cause you to wrongly estimate the relationship between your independent and dependent variables.
To ensure the internal validity of your research, you must consider the impact of confounding variables. If you fail to account for them, you might over- or underestimate the causal relationship between your independent and dependent variables , or even find a causal relationship where none exists.
Yes, but including more than one of either type requires multiple research questions .
For example, if you are interested in the effect of a diet on health, you can use multiple measures of health: blood sugar, blood pressure, weight, pulse, and many more. Each of these is its own dependent variable with its own research question.
You could also choose to look at the effect of exercise levels as well as diet, or even the additional effect of the two combined. Each of these is a separate independent variable .
To ensure the internal validity of an experiment , you should only change one independent variable at a time.
No. The value of a dependent variable depends on an independent variable, so a variable cannot be both independent and dependent at the same time. It must be either the cause or the effect, not both!
You want to find out how blood sugar levels are affected by drinking diet soda and regular soda, so you conduct an experiment .
- The type of soda – diet or regular – is the independent variable .
- The level of blood sugar that you measure is the dependent variable – it changes depending on the type of soda.
Determining cause and effect is one of the most important parts of scientific research. It’s essential to know which is the cause – the independent variable – and which is the effect – the dependent variable.
In non-probability sampling , the sample is selected based on non-random criteria, and not every member of the population has a chance of being included.
Common non-probability sampling methods include convenience sampling , voluntary response sampling, purposive sampling , snowball sampling, and quota sampling .
Probability sampling means that every member of the target population has a known chance of being included in the sample.
Probability sampling methods include simple random sampling , systematic sampling , stratified sampling , and cluster sampling .
Using careful research design and sampling procedures can help you avoid sampling bias . Oversampling can be used to correct undercoverage bias .
Some common types of sampling bias include self-selection bias , nonresponse bias , undercoverage bias , survivorship bias , pre-screening or advertising bias, and healthy user bias.
Sampling bias is a threat to external validity – it limits the generalizability of your findings to a broader group of people.
A sampling error is the difference between a population parameter and a sample statistic .
A statistic refers to measures about the sample , while a parameter refers to measures about the population .
Populations are used when a research question requires data from every member of the population. This is usually only feasible when the population is small and easily accessible.
Samples are used to make inferences about populations . Samples are easier to collect data from because they are practical, cost-effective, convenient, and manageable.
There are seven threats to external validity : selection bias , history, experimenter effect, Hawthorne effect , testing effect, aptitude-treatment and situation effect.
The two types of external validity are population validity (whether you can generalize to other groups of people) and ecological validity (whether you can generalize to other situations and settings).
The external validity of a study is the extent to which you can generalize your findings to different groups of people, situations, and measures.
Cross-sectional studies cannot establish a cause-and-effect relationship or analyze behavior over a period of time. To investigate cause and effect, you need to do a longitudinal study or an experimental study .
Cross-sectional studies are less expensive and time-consuming than many other types of study. They can provide useful insights into a population’s characteristics and identify correlations for further research.
Sometimes only cross-sectional data is available for analysis; other times your research question may only require a cross-sectional study to answer it.
Longitudinal studies can last anywhere from weeks to decades, although they tend to be at least a year long.
The 1970 British Cohort Study , which has collected data on the lives of 17,000 Brits since their births in 1970, is one well-known example of a longitudinal study .
Longitudinal studies are better to establish the correct sequence of events, identify changes over time, and provide insight into cause-and-effect relationships, but they also tend to be more expensive and time-consuming than other types of studies.
Longitudinal studies and cross-sectional studies are two different types of research design . In a cross-sectional study you collect data from a population at a specific point in time; in a longitudinal study you repeatedly collect data from the same sample over an extended period of time.
Longitudinal study | Cross-sectional study |
---|---|
observations | Observations at a in time |
Observes the multiple times | Observes (a “cross-section”) in the population |
Follows in participants over time | Provides of society at a given point |
There are eight threats to internal validity : history, maturation, instrumentation, testing, selection bias , regression to the mean, social interaction and attrition .
Internal validity is the extent to which you can be confident that a cause-and-effect relationship established in a study cannot be explained by other factors.
In mixed methods research , you use both qualitative and quantitative data collection and analysis methods to answer your research question .
The research methods you use depend on the type of data you need to answer your research question .
- If you want to measure something or test a hypothesis , use quantitative methods . If you want to explore ideas, thoughts and meanings, use qualitative methods .
- If you want to analyze a large amount of readily-available data, use secondary data. If you want data specific to your purposes with control over how it is generated, collect primary data.
- If you want to establish cause-and-effect relationships between variables , use experimental methods. If you want to understand the characteristics of a research subject, use descriptive methods.
A confounding variable , also called a confounder or confounding factor, is a third variable in a study examining a potential cause-and-effect relationship.
A confounding variable is related to both the supposed cause and the supposed effect of the study. It can be difficult to separate the true effect of the independent variable from the effect of the confounding variable.
In your research design , it’s important to identify potential confounding variables and plan how you will reduce their impact.
Discrete and continuous variables are two types of quantitative variables :
- Discrete variables represent counts (e.g. the number of objects in a collection).
- Continuous variables represent measurable amounts (e.g. water volume or weight).
Quantitative variables are any variables where the data represent amounts (e.g. height, weight, or age).
Categorical variables are any variables where the data represent groups. This includes rankings (e.g. finishing places in a race), classifications (e.g. brands of cereal), and binary outcomes (e.g. coin flips).
You need to know what type of variables you are working with to choose the right statistical test for your data and interpret your results .
You can think of independent and dependent variables in terms of cause and effect: an independent variable is the variable you think is the cause , while a dependent variable is the effect .
In an experiment, you manipulate the independent variable and measure the outcome in the dependent variable. For example, in an experiment about the effect of nutrients on crop growth:
- The independent variable is the amount of nutrients added to the crop field.
- The dependent variable is the biomass of the crops at harvest time.
Defining your variables, and deciding how you will manipulate and measure them, is an important part of experimental design .
Experimental design means planning a set of procedures to investigate a relationship between variables . To design a controlled experiment, you need:
- A testable hypothesis
- At least one independent variable that can be precisely manipulated
- At least one dependent variable that can be precisely measured
When designing the experiment, you decide:
- How you will manipulate the variable(s)
- How you will control for any potential confounding variables
- How many subjects or samples will be included in the study
- How subjects will be assigned to treatment levels
Experimental design is essential to the internal and external validity of your experiment.
I nternal validity is the degree of confidence that the causal relationship you are testing is not influenced by other factors or variables .
External validity is the extent to which your results can be generalized to other contexts.
The validity of your experiment depends on your experimental design .
Reliability and validity are both about how well a method measures something:
- Reliability refers to the consistency of a measure (whether the results can be reproduced under the same conditions).
- Validity refers to the accuracy of a measure (whether the results really do represent what they are supposed to measure).
If you are doing experimental research, you also have to consider the internal and external validity of your experiment.
A sample is a subset of individuals from a larger population . Sampling means selecting the group that you will actually collect data from in your research. For example, if you are researching the opinions of students in your university, you could survey a sample of 100 students.
In statistics, sampling allows you to test a hypothesis about the characteristics of a population.
Quantitative research deals with numbers and statistics, while qualitative research deals with words and meanings.
Quantitative methods allow you to systematically measure variables and test hypotheses . Qualitative methods allow you to explore concepts and experiences in more detail.
Methodology refers to the overarching strategy and rationale of your research project . It involves studying the methods used in your field and the theories or principles behind them, in order to develop an approach that matches your objectives.
Methods are the specific tools and procedures you use to collect and analyze data (for example, experiments, surveys , and statistical tests ).
In shorter scientific papers, where the aim is to report the findings of a specific study, you might simply describe what you did in a methods section .
In a longer or more complex research project, such as a thesis or dissertation , you will probably include a methodology section , where you explain your approach to answering the research questions and cite relevant sources to support your choice of methods.
Ask our team
Want to contact us directly? No problem. We are always here for you.
- Email [email protected]
- Start live chat
- Call +1 (510) 822-8066
- WhatsApp +31 20 261 6040
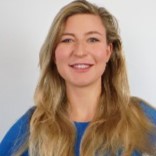
Our team helps students graduate by offering:
- A world-class citation generator
- Plagiarism Checker software powered by Turnitin
- Innovative Citation Checker software
- Professional proofreading services
- Over 300 helpful articles about academic writing, citing sources, plagiarism, and more
Scribbr specializes in editing study-related documents . We proofread:
- PhD dissertations
- Research proposals
- Personal statements
- Admission essays
- Motivation letters
- Reflection papers
- Journal articles
- Capstone projects
Scribbr’s Plagiarism Checker is powered by elements of Turnitin’s Similarity Checker , namely the plagiarism detection software and the Internet Archive and Premium Scholarly Publications content databases .
The add-on AI detector is powered by Scribbr’s proprietary software.
The Scribbr Citation Generator is developed using the open-source Citation Style Language (CSL) project and Frank Bennett’s citeproc-js . It’s the same technology used by dozens of other popular citation tools, including Mendeley and Zotero.
You can find all the citation styles and locales used in the Scribbr Citation Generator in our publicly accessible repository on Github .
Open-ended vs. closed-ended questions in survey
Discover the power of open-ended vs. closed-ended questions in research. Learn how each type influences insights, efficiency, and data collection
Open-Ended vs. Closed-Ended Questions
Do you ever feel like stakeholders undervalue your organization's impactful programs? Clearly communicating program success can be a challenge, leading to a disconnect and funding difficulties. This article tackles a critical tool for success: the art of crafting the right questions .
Well-designed questions, whether open-ended or closed-ended , are the foundation for effectively communicating your program's impact to stakeholders. Open-ended questions can capture the voices and experiences of stakeholders, while closed-ended questions provide valuable data for quantifying program effectiveness. By understanding the strengths and weaknesses of each approach, you can design surveys that:
- Capture stakeholder voices: Learn what matters most to your stakeholders and how your programs are affecting them.
- Demonstrate program effectiveness: Gather data that clearly shows the positive changes your programs are creating.
- Secure stakeholder support: Tell a compelling story about your program's impact that resonates with stakeholders and motivates them to lend their support.
This article will guide you through the power of question design, helping you choose the right approach to unlock stakeholder impact and secure the funding you deserve.
Diverse Closed-ended vs. Open-ended Questions for Actionable Insights
Closed-ended Questions | Open-ended Questions |
---|---|
On a scale of 0-10, how likely are you to recommend our program to a friend or colleague? | What specific aspects of our program would you highlight when recommending it to others? If you wouldn't recommend it, what improvements would make you more likely to do so? |
Rate your agreement with the statement: "The training provided by our organization has significantly improved my job skills." (1: Strongly Disagree, 2: Disagree, 3: Neutral, 4: Agree, 5: Strongly Agree) | Which specific skills from our training have you applied in your work? Can you provide an example of how these skills have impacted your job performance? |
How satisfied are you with the support provided by our organization? (Very Unsatisfied, Unsatisfied, Neutral, Satisfied, Very Satisfied) | What aspects of our support have been most valuable to you, and what areas could be improved to better meet your needs? |
Has your household income increased as a result of participating in our microfinance program? | How has our microfinance program impacted your financial situation? Please describe any changes in your income, savings, or overall financial stability. |
Which of the following best describes the primary benefit you've experienced from our health education program? (a) Improved personal hygiene practices (b) Better understanding of nutrition (c) Increased access to healthcare services (d) Enhanced mental health awareness | Can you elaborate on how the health education program has influenced your daily life and overall well-being? Please provide specific examples of changes you've made. |
On a scale of 1-7, how empowered do you feel to make positive changes in your community after participating in our leadership workshop? (1: Not at all empowered, 7: Extremely empowered) | In what ways has our leadership workshop empowered you to make changes in your community? Please describe any initiatives or actions you've taken as a result. |
How would you characterize the environmental impact of our sustainable agriculture project? Harmful 1 - 2 - 3 - 4 - 5 Beneficial | What specific changes have you observed in local agricultural practices and the environment since the implementation of our sustainable agriculture project? |
Defining Open-Ended and Closed-Ended Questions
Open-ended questions allow respondents to provide unrestricted, qualitative responses in their own words. These questions typically begin with phrases like "how," "what," or "why," encouraging respondents to express their thoughts, feelings, and opinions freely. Examples of open-ended questions include "What factors influenced your decision?" or "How do you feel about this product?"
On the other hand, closed-ended questions offer respondents a set of predetermined response options to choose from. These questions often require a simple "yes" or "no" answer or ask respondents to select from a list of predefined options. Closed-ended questions are designed to elicit specific, quantitative responses and are often used to gather structured data efficiently. Examples of closed-ended questions include "Did you purchase this product?" or "Which of the following options best describes your experience?"
Open Ended vs Closed Ended Survey
Step 1: survey design, quantitative question (nps):.
"On a scale of 0-10, how likely are you to recommend our scholarship program to a friend or colleague?"
Qualitative Question:
"Please explain how the scholarship has impacted your academic journey and future prospects."
Step 2: Data Collection
Collect responses from scholarship recipients through online surveys or interviews.
Step 3: Data Analysis and Pattern Recognition
Qualitative responses:, inductive analysis (automated pattern recognition):, deductive analysis (key insights):.
- Financial relief is the most prevalent theme, mentioned by all respondents and strongly correlated with high NPS scores (9.2 average).
- Supporting family financial struggles, though less frequent (33%), shows the highest correlation with NPS (9.8 average), indicating a significant impact on those facing family-related financial challenges.
- Avoidance of debt is a key factor, mentioned by 66% of respondents and associated with high NPS scores (9.5 average).
- Campus engagement, while mentioned less frequently, is associated with high NPS scores (9.3 average), suggesting that the scholarship enables a more fulfilling college experience beyond academics.
- Stress reduction and addressing commuting difficulties, though mentioned less often, still show a positive impact on NPS scores, indicating the scholarship's holistic effect on students' lives.
Step 4: Sopact Sense Generated Impact Story
"Our scholarship program is transforming lives with remarkable efficiency. With an NPS of 25, we're not just providing financial aid; we're catalyzing academic success and personal growth. The 100% mention rate of financial relief, coupled with an average NPS of 9.2 for this theme, underscores the program's core impact.
Notably, while only 33% of recipients mentioned support for family financial struggles, this aspect correlates with the highest average NPS of 9.8. This reveals the profound impact on students from challenging financial backgrounds. Additionally, the 66% who highlighted debt avoidance gave an average NPS of 9.5, demonstrating the long-term financial benefits of our program.
Beyond finances, we're fostering a more engaged student body. The 33% who mentioned increased campus engagement gave an average NPS of 9.3, indicating that our support extends to enriching the overall college experience. By addressing diverse needs from stress reduction to commuting difficulties, we're creating a comprehensive support system that resonates deeply with our recipients.
This data-driven analysis shows that our scholarship program isn't just meeting needs; it's exceeding expectations and creating loyal advocates. We're not only changing individual lives but potentially reshaping the landscape of higher education accessibility and student success."
Step 5: Actionable Insights
Based on the analysis, here are key actionable insights to improve the scholarship program:
1. Expand Family Financial Support Services
Insight: Supporting family financial struggles showed the highest NPS correlation (9.8).
Action: Develop a complementary program offering financial literacy workshops and resources for recipients' families. This could include budgeting tools, financial planning sessions, and information on additional support services.
2. Enhance Debt Avoidance Strategies
Insight: 66% of recipients mentioned debt avoidance, with a high NPS correlation (9.5).
Action: Partner with financial institutions to provide low-interest loan options or loan forgiveness programs for any remaining educational expenses. Offer targeted financial counseling to help students minimize overall debt burden.
3. Boost Campus Engagement Opportunities
Insight: Campus engagement, while mentioned by 33%, had a high NPS correlation (9.3).
Action: Allocate a portion of the scholarship funds specifically for campus activity fees or club memberships. Create a mentorship program pairing scholarship recipients with alumni or upperclassmen to encourage greater campus involvement.
4. Address Commuting Challenges
Insight: Commuting difficulties were mentioned by 33% of recipients, with a lower NPS correlation (8.5).
Action: Explore partnerships with local transportation services to offer discounted passes for scholarship recipients. Consider allocating additional funds for recipients with significant commuting challenges to support housing closer to campus.
5. Implement Stress Reduction Programs
Insight: Stress reduction was noted by 33% of recipients, with an NPS correlation of 8.7.
Advantages of Open-Ended Questions
One of the primary advantages of open-ended questions is their ability to capture rich, detailed responses. By allowing respondents to elaborate on their thoughts and experiences, open-ended questions provide researchers with a deeper understanding of complex issues. These responses can reveal unexpected insights, uncovering nuances that closed-ended questions may overlook.
Moreover, open-ended questions empower respondents to express themselves in their own words, fostering a sense of ownership and authenticity in their responses. This approach can lead to more honest and insightful feedback, as respondents feel valued and heard throughout the research process.
Additionally, open-ended questions are versatile and adaptable to various research contexts. They can be used to explore a wide range of topics and are particularly well-suited for exploratory research, hypothesis generation, and qualitative data analysis. Researchers can gain valuable insights into respondents' perspectives, attitudes, and behaviors, providing a holistic view of the subject under study.
Advantages of Closed-Ended Questions
Closed-ended questions offer several advantages, particularly in terms of data collection efficiency and analysis. By providing predefined response options, these questions enable researchers to gather standardized data quickly and easily. This structured approach streamlines the data collection process, allowing researchers to collect large volumes of data efficiently.
Moreover, closed-ended questions facilitate quantitative analysis, as the responses can be easily categorized and quantified. Researchers can use statistical techniques to analyze and interpret the data, identifying patterns, trends, and correlations with precision. This quantitative approach is particularly valuable for making data-driven decisions, evaluating hypotheses, and measuring the effectiveness of interventions or treatments.
Additionally, closed-ended questions minimize respondent burden by providing clear and concise response options. Respondents can answer these questions quickly, reducing survey fatigue and improving overall response rates. This efficiency is especially beneficial in large-scale research studies or surveys conducted in time-sensitive environments.
Choosing the Right Approach
While both open-ended and closed-ended questions offer distinct advantages, choosing the right approach depends on the research objectives, context, and target audience. In many cases, a combination of both types of questions may be most effective, allowing researchers to gather comprehensive data while balancing the need for depth and efficiency.
For exploratory research or when seeking in-depth insights into complex phenomena, open-ended questions are invaluable. They encourage respondents to share their perspectives openly, uncovering nuanced details and diverse viewpoints. Researchers can use qualitative analysis techniques, such as thematic coding or content analysis, to identify patterns and themes within the data, enriching their understanding of the subject matter.
In contrast, closed-ended questions are well-suited for research scenarios that require standardized data collection and quantitative analysis. They enable researchers to measure attitudes, behaviors, and preferences systematically, facilitating comparisons across groups or time periods. Statistical analysis techniques, such as descriptive statistics or inferential tests, can be applied to closed-ended data to draw meaningful conclusions and make evidence-based recommendations.
Difference between open and closed ended questions
Open-ended and closed-ended questions are two fundamental types of questions used in surveys, interviews, and research studies. Each type has its own strengths and weaknesses, and the choice between them depends on the goals of the data collection process.
Open-ended vs Closed-ended Questions
Comparison table.
Feature | Open-Ended Questions | Closed-Ended Questions |
---|---|---|
Response Type | Descriptive, detailed | Predefined options |
Data Type | Qualitative | Quantitative |
Analysis | Complex, time-consuming | Simple, quick |
Depth of Insight | High, rich detail | Low, limited detail |
Ease of Response | Requires more effort | Easy, quick |
Use Case | Exploring new topics, understanding experiences | Measuring specific variables, comparing responses |
Risk of Bias | Lower, as responses are freeform | Higher, depends on how options are framed |
Open-ended Questions
Open-ended questions allow respondents to answer in their own words, providing rich, detailed responses. They are excellent for exploring new topics or gathering in-depth information about experiences and opinions.
Closed-ended Questions
Closed-ended questions provide respondents with a set of predefined answer options to choose from. They are ideal for collecting quantitative data and making comparisons across respondents or over time.
Pros and Cons
Pros of open-ended questions.
- Provide rich, detailed insights
- Allow for unexpected responses
- Useful for exploring new topics
- Lower risk of bias in responses
Cons of Open-ended Questions
- Time-consuming to analyze
- May be difficult to quantify
- Require more effort from respondents
- Can lead to irrelevant responses
When to Use Each Type
Choose open-ended questions when you need detailed insights, are exploring new topics, or want to understand complex experiences. Opt for closed-ended questions when you need quantifiable data, want to make direct comparisons, or are dealing with a large sample size.
In practice, many surveys and research studies use a combination of both types to balance the need for detailed insights with the ability to quantify and compare responses efficiently.
Open-Ended Questions in Surveys
Open-ended questions are a cornerstone of qualitative research, providing a window into the deeper thoughts, feelings, and motivations of respondents. They are particularly effective when you need nuanced insights or varied perspectives that structured data cannot capture. To craft effective open-ended questions:
Focus on the Specific : Tailor questions to gather specific information that aligns with the research objectives. For instance, instead of asking "What do you think about our product?" refine it to "What specific features do you like most about our product, and why?"

invite detailed and descriptive answers. Consider using phrases such as "describe in detail," "explain how," or "what led you to," which guide respondents to delve deeper into their experiences and reasoning. This approach not only enriches the data but also brings forth the nuances of personal narratives and insights.
Equally crucial is the formulation of questions that remain neutral, avoiding any language that might steer respondents toward a specific answer. This practice is fundamental to preserving the objectivity of the data collected, ensuring that the insights gained are a genuine reflection of the respondent's thoughts and not influenced by the wording of the question.
Moreover, a well-designed survey question should accommodate a spectrum of perspectives. By framing questions broadly enough, researchers can capture a diverse array of responses, thereby reflecting the varied experiences and opinions of all participants. This inclusivity enriches the data set and provides a more comprehensive understanding of the surveyed group.
In gathering open-ended responses, the goal is to create a platform where each voice and experience can articulate itself freely and distinctly. This approach not only respects the diversity of the respondent pool but also enhances the depth and breadth of the insights gained from the research.

Before launching a survey broadly, it’s crucial to test the open-ended questions with a smaller, representative group. This preliminary phase aims to ensure that the questions effectively elicit the type of responses anticipated. Observing how these respondents interact with the questions provides invaluable insights, allowing for adjustments and refinements to the survey based on actual feedback. This iterative process helps fine-tune the questions to better capture the depth and variety of data needed.
To streamline and enhance this process, employing a modern qualitative data analytics platform like Sopact Sense can be transformative. Sopact Sense dramatically reduces the time and effort traditionally required for data analysis, condensing months of work into mere minutes. With its advanced capabilities, it offers 30 times better accuracy, facilitating both inductive and deductive analysis approaches. Researchers can utilize bottom-up pattern analysis to identify emerging themes without prior assumptions, or apply top-down strategies to test specific hypotheses or code responses post-collection.
Furthermore, Sopact Sense enables detailed demographic filtering, empowering researchers to dissect data layers and uncover genuine causality and correlations. This capability is particularly valuable in understanding how different groups perceive and respond to various issues, enhancing the overall quality and applicability of the research outcomes. By integrating such advanced tools into the survey design and testing phase, researchers can achieve a more dynamic, responsive, and precise exploration of the data they collect.

To ensure that analytics efforts align closely with key organizational goals and foster actionable outcomes at an individual stakeholder level, it’s essential to craft a narrative that integrates both qualitative and quantitative data. This approach allows for a comprehensive understanding of how initiatives are impacting stakeholders, highlighting necessary adjustments and future strategies.
Creating a Comprehensive Narrative:
- Identify and Prioritize Goals: Start by clearly identifying the most crucial goals for the analytics project. Determine what success looks like for each goal and how it aligns with broader organizational objectives.
- Collect Qualitative and Quantitative Data: Use a mixed-methods approach to data collection. Qualitative data can be gathered through open-ended survey questions, interviews, and focus groups that explore stakeholders' feelings, experiences, and suggestions for improvement. Quantitative data should be collected through structured surveys, performance metrics, and other measurable indicators.
- Pre and Post Analysis: If applicable, conduct a pre-intervention analysis to establish a baseline, followed by a post-intervention analysis to measure changes. This comparative analysis can highlight the direct impacts of specific changes and help in communicating these changes effectively.
- Narrate the Story of Each Goal: For each key goal, create a narrative that weaves together the qualitative insights with the quantitative results. This story should outline what was initially found, what changes were implemented, and how these changes influenced the outcomes. Emphasize both the successes and the areas needing improvement.
- Detail Actionable Insights: Based on the narrative, extract actionable insights specific to each stakeholder group. Detail what steps will be taken to address the issues uncovered in the analysis. This might include strategic adjustments, resource reallocations, or new initiatives.
- Communicate Changes and Impact: Use the narratives to communicate with stakeholders about the changes made and their impacts. This communication should be clear and tailored to the audience, ensuring that each stakeholder understands how the findings relate to them and what future actions are planned.
- Plan for Continuous Improvement: Establish a plan for ongoing monitoring and evaluation based on the narrative outcomes. This plan should include regular check-ins and updates to the data collection and analysis processes to ensure they remain aligned with the organization's evolving needs and goals.
By meticulously linking each story to the most important analytics goals and utilizing a narrative that blends qualitative depth with quantitative rigor, organizations can not only achieve a more thorough understanding of their impact but also engage stakeholders in a meaningful way that promotes sustained improvement and strategic decision-making.
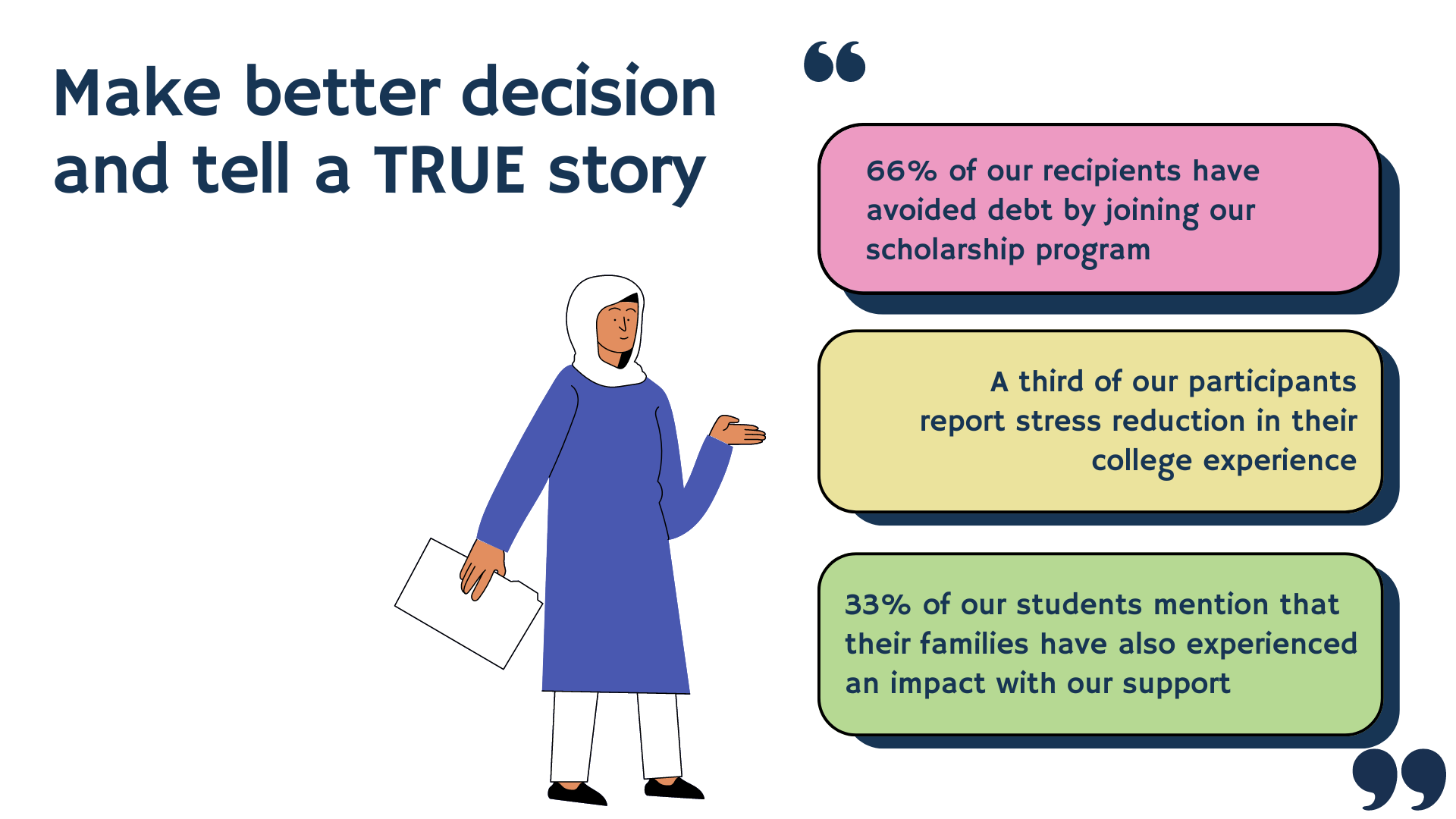
Closed-Ended Questions in Surveys
Creating closed-ended questions for surveys involves a meticulous design process to ensure that the quantitative data collected is accurate, clear, and meaningful. These types of questions are pivotal for confirming hypotheses, measuring trends, and obtaining data that are straightforward to analyze statistically. Here’s how to enhance the effectiveness of closed-ended questions in your surveys:
1. Define Clear Options
To begin, it’s crucial that each closed-ended question provides specific, mutually exclusive categories. This step is essential to cover all possible responses, thus eliminating any potential ambiguity or overlap. For example, if you're asking about frequency of service usage, your options should range clearly from 'Never' to 'Daily' without any vague terms in between. This clarity ensures that the data you collect can be analyzed straightforwardly, leading to more reliable insights.
2. Balance the Scales
Using balanced rating scales, such as Likert scales, can significantly enhance the quality of the data gathered. These scales should offer an equal number of options on either side of a neutral option (if one is included) to ensure an unbiased distribution of responses. For instance, a satisfaction survey might use a scale from 'Very Dissatisfied' to 'Very Satisfied' with 'Neither Satisfied nor Dissatisfied' as a midpoint. This balance helps in minimizing response biases, providing a more accurate picture of respondent sentiments.
3. Keep It Simple
Each question should be formulated to be as clear and straightforward as possible. The language used needs to be simple enough that respondents do not require additional information or context to give an answer. This approach reduces the risk of misinterpretation and ensures that responses are based on the respondents’ true opinions and experiences. An example might be using "Do you agree that the customer service was helpful?" instead of a more complex phrasing that could confuse the respondent.
4. Include an 'Other' Option
Sometimes, even well-designed questions might not capture all possible respondent experiences. In such cases, including an 'Other' option with a space for respondents to specify their answer can be invaluable. This option acts as a safety net, capturing data that might otherwise be missed and offering insights that could lead to new discoveries or considerations in your analysis.
5. Pre-test Your Questions
Before deploying the survey to a larger audience, conduct a pre-test with a small, representative group. This testing helps ensure that the questions are understood as intended and that all potential responses are adequately covered. Gather feedback on the clarity of the questions and the adequacy of the response options. Use this feedback to make necessary adjustments, refining your survey to better meet its objectives.
Putting It All Together
When these elements are carefully integrated into the design of closed-ended questions, the resulting data becomes a powerful tool for statistical analysis and decision-making. These questions not only streamline the data collection process but also enhance the precision and applicability of the insights gained. By rigorously crafting and testing your closed-ended questions, you ensure that the survey effectively measures the intended variables and yields high-quality data that can support robust conclusions and strategic actions.
Open and Closed-Ended Questions in Survey Design
Incorporating both open and closed-ended questions in surveys can significantly enhance the data collection process by melding the depth of qualitative feedback with the quantitative data's scope. This dual approach proves invaluable in multifaceted research areas, where understanding the underlying reasons behind behaviors, decisions, or preferences is key.
Sequential Integration : Begin with closed-ended questions to collect broad data, then follow up with open-ended questions to delve into specific areas of interest more deeply. This technique helps provide a contextual backdrop for the quantitative findings through rich qualitative insights.
Parallel Integration : Simultaneously employ open and closed-ended questions regarding the same subject within a survey. This strategy captures a wide array of data, offering both statistical comprehensiveness and insightful qualitative depth.
Iterative Design : Utilize initial survey responses to refine or introduce new questions that probe deeper into significant themes that arise. This responsive design allows the survey to evolve based on real-time insights, making it highly adaptive to the research needs.
By strategically combining these approaches, researchers can leverage the strengths of both qualitative and quantitative methodologies, resulting in more rounded and actionable data.
For more detailed strategies on mixed-method surveys, refer to the guide provided by Sopact. Learn more about mixed-method surveys at Sopact .

In conclusion, the choice between open-ended and closed-ended questions is a critical consideration in research design and data collection. Each type of question offers unique advantages and limitations, influencing the depth, efficiency, and quality of insights obtained. By understanding the strengths and weaknesses of both approaches and selecting the most appropriate method for each research context, researchers can maximize the value of their findings and generate meaningful contributions to their field.
How helpful was this?
We're sorry to hear that. How can we improve?
Thanks for your feedback! Let us know how this article helped:
Looking for something else?
Still need help.

Research Writing Process (Book)
Find the following links to be useful in learning about the research writing process., writing a preliminary outline, another sample outline, sample final outline.
Johnny Johnson
31 March, 2010
Education: Why does it seem everyone is against it?
Thesis Statement: Poverty, segregation of minorities, and changes in federal funding policies negatively affect education.
I. The schools from low-income communities lack the revenues to provide students with equal opportunities.
A. Schools in low-income areas usually have limited resources, which makes it difficult to provide students with quality education.
1. Those schools usually have under-qualified teachers.
2. Those low-income communities supporting the school do not bring in enough revenue to provide the schools with proper curriculum, supplies, and extra-curricular programs.
B. Students from schools in low-income communities have less of an opportunity to get a decent job later in life.
1. When students are not equally educated, the economy suffers due to a lack of competition in the job market.
2. Those students from under-funded schools are usually born into the bottom of the economy and are not given the proper opportunities to better themselves.
II. The majority of the population of poverty stricken schools consists of minorities.
A. In most urban centers, minorities are the majority
1. Urban school serving minorities contain the greatest number of teachers teaching outside of their training.
2. Many of the minority students attending these schools believe society does not care to give them the resources they need to succeed.
B. The achievement gap between white students and minorities is large.
III. The quality of education suffers due to changes in policies, such as the addition of the “No Child Left Behind Act.”
A. The “No Child Left Behind Act” usually forces schools to make choices to lower standards in order to avoid sanctions and retain funding.
1. Many times teachers are forced to abandon the normal curriculum in order to focus on teaching the students what the government decides is most important.
2. NCLB has caused much controversy among educational systems.
B. Standards established by the “No Child Left Behind Act” are hard to develop and enforce because curriculum differs all over the country.
1. The government should not be responsible for developing the standards because they are not the trained educators.
2. Standardized tests are not a fair or accurate way of measuring how much children are learning due to the variation of curriculum throughout the country.
C. The “No Child Left Behind Act” does not work properly because it is not adequately funded by the federal government.
1. The government provides only two-thirds of the money originally promised making states responsible for the rest.
2. The federal government often cuts money for education funding.
Watch the ERP systems compliance and validation webinar!
Navigating 21 CFR Part 11: Open vs. Closed Systems
Tuesday august 20, 2024.
Compliance with 21 CFR Part 11 is essential for ensuring the integrity and security of electronic records and signatures. One crucial aspect of this regulation is understanding the difference between open and closed systems and their respective compliance requirements. This blog delves into these distinctions, explores the specific compliance challenges associated with each, and illustrates how Arbour Group can help life sciences companies navigate these complexities effectively.
What is 21 CFR Part 11?
21 CFR Part 11 is a regulation established by the U.S. Food and Drug Administration (FDA) that sets the criteria for electronic records and electronic signatures to be considered trustworthy, reliable, and equivalent to paper records. This regulation is vital for life sciences companies, ensuring electronic data meets the same rigorous standards as traditional paper documentation.
Understanding Open and Closed Systems
Open Systems
According to 21 CFR 11.3(b)(9), an open system is an environment where system access is not controlled by the persons responsible for the content of electronic records. This means that users can create accounts and access the system without needing approval from an administrator, making it more accessible and more vulnerable to security risks.
Security Risks: Open systems can pose significant security risks due to the lack of controlled access. Unauthorized individuals could potentially access sensitive data, leading to breaches and data integrity issues.
Data Integrity: Ensuring the accuracy and reliability of electronic records can be challenging in open systems. Companies must implement robust controls to protect data from unauthorized access and manipulation.
Compliance Requirements
Encryption: Documents must be encrypted to protect sensitive data from unauthorized access. Encryption ensures that only authorized personnel can read the data, maintaining its confidentiality.
Digital Signatures: Using digital signature standards, such as public key infrastructure (PKI) and multi-factor authentication (MFA), helps verify the signer's identity and maintain the integrity of electronic records.
Examples of Open Systems
Email Systems: Email platforms are examples of open systems where users can create accounts freely. Companies must ensure strong password policies and encryption to maintain the security of email communications.
Cloud Storage Services: Services like Google Drive and Dropbox allow users to store and share files. Companies must implement access controls and encryption to protect sensitive data stored in these open systems.
Closed Systems
As defined by 21 CFR 11.3(b)(4), a closed system is an environment where system access is controlled by the persons responsible for the content of electronic records. Only authorized personnel can access the system, and their actions are monitored and recorded, providing a higher level of security and control.
Access Control: It is crucial to maintain strict access control to ensure that only authorized individuals can use the system. This includes implementing user authentication and authorization processes.
Audit Trails : Closed systems must have audit trails that record all user activities and changes to electronic records, ensuring accountability and traceability.
Validation: Systems must be validated to ensure they operate correctly and meet regulatory requirements. This includes testing for accuracy, reliability, and performance.
Audit Trails: Secure, computer-generated, and time-stamped audit trails must be used to record operator entries and actions on electronic records.
User Authentication: Only authorized personnel should be allowed to use the system and electronic signatures, ensuring data integrity and security.
Examples of Closed Systems
Document Management Systems (DMS): These systems manage electronic documents like standard operating procedures and batch records, with access restricted to authorized users.
Audit Trails: QMS software solutions manage quality-related activities within a controlled environment, such as audits and employee training.
Differences Between Open and Closed Systems
The primary difference between open and closed systems lies in access control. Open systems allow users to create their own accounts, which can introduce security risks and compliance challenges. On the other hand, closed systems require administrative approval for access, providing a higher level of control over data integrity and security. While both systems must comply with 21 CFR Part 11 requirements, open systems have additional requirements, such as encryption and digital signature standards.
How Arbour Group Can Help
Arbour Group offers a range of services to ensure your systems comply with 21 CFR Part 11, whether open or closed. Our comprehensive validation services ensure your systems meet regulatory standards and operate reliably, while our in-depth security assessments identify vulnerabilities and implement robust security measures to protect your data. We assist in preparing for FDA audits and inspections, ensuring all compliance requirements are met. Understanding that each organization has unique needs and challenges, Arbour Group provides tailored solutions to address your specific compliance requirements, ensuring that your open and closed systems are secure and compliant. Our team of experts is dedicated to providing ongoing support and guidance, helping you navigate the complexities of 21 CFR Part 11 compliance from initial assessment to continuous monitoring.
Arbour Group has provided us with competent validation project leadership that has enabled us to complete projects in a timely and cost effective manner. The use of Arbour’s validation product greatly facilitated the process.
The regulatory assistance provided by Arbour Group has enabled us to enhance our compliance profile with life sciences customers. Their Managed Services for software development and quality assurance play a key role in controlling business risk and reducing costs.
Arbour Group provided effective validation services to us and were a valuable part of the overall success of our company-wide ERP implementation. Their integration into our multi-phase ERP roll out was seamless and assured us of comprehensive regulatory compliance.

IMAGES
COMMENTS
The thesis is a declarative sentence. It is a clear, specific statement, which states the main point of a the paper, thereby limiting the topic and indicating the researcher's approach to the topic. For this research paper we will be discussing the difference between the open (implicit) thesis approach, and the closed (explicit) thesis. Open ...
The choice between an open or a closed thesis statement often depends upon the complexity of your argument. When in doubt about how to structure your thesis statement, you should seek the advice of your instructor. Consult section 3.4 for help constructing a strong open or closed thesis statement.
Types of Theses Open Thesis: A thesis that tells the reader only your position on a topic. Boston is a better city than New York. Advantages: You can hook the reader's attention and get him/her to read on. Closed Thesis: A thesis that tells the reader both your position and the reasons you feel that way.
A thesis statement usually evolves only after considerable reading, writing, and thinking has been done on your topic. You can begin the writing process of an essay with a preliminary or open thesis, move on to a tentative thesis or hypothesis, and finally arrive at your closed or final thesis.
An open thesis, also known as an open dissertation, is a thesis that is freely available for members of the public to access upon publication, and often also during the planning and writing process. The decision to write an open thesis is made by the author, who will usually explain their rationale for creating an open thesis as part of the ...
Now that we have a clear understanding of open and closed questions, it's easier to comprehend the difference between an open and closed thesis. A thesis statement is the main argument or point that a writer aims to prove or support in an essay or research paper.
Open Form: -when you want to delay your thesis rather than announce it in the introduction to create suspense; a delayed thesis structure is less combative and more friendly; it conveys an unfolding "let's think this through together" feeling. -when your aim is expressive, exploratory, or literary. These aims tend to be served better ...
What Is Open Thesis? 6 Tips to Make It More Understandable For Your Readers Often viewed as the elusive and enigmatic element of academic writing, open thesis statements send chills down the spines of even the most experienced and seasoned scholars. Reason? Because of its wider reach than a closed thesis, which commands attention and requires meticulous scrutiny.
After the expiration of the embargo, the dissertation will become openly available so that everyone can find and read it. Of course, you can also choose to make your dissertation immediately available in Open Access. Just contact the Open Access Support Desk and they will gladly remove the embargo. The other way around is also possible: if you ...
The thesis is a declarative sentence. It is a clear, specific statement, which states the main point of a the paper, thereby limiting the topic and indicating the researcher's approach to the topic. For this research paper we will be discussing the difference between the open (implicit) thesis approach, and the closed (explicit) thesis.
Essay Structure Options. Despite what you may have been told, a thesis can appear anywhere in an essay. If it appears near the beginning — typically at the end of the first paragraph, though longer essays can sometimes require introductions longer than a single paragraph — the essay is closed form. Each paragraph then offers evidence to ...
Learn how to write an open and closed claim (thesis) for an argument.(Recorded with https://screencast-o-matic.com)
Are you writing your academic project but don't know the difference between open vs close thesis? Read this blog to learn it in detail with writing tips and examples.
To print or download this file, click the link below: OPEN FORM TO CLOSED FORM WRITING STYLES.pdf — PDF document, 82 KB (84144 bytes)
Open-Ended vs. Closed Questions in User Research. Summary: Open-ended questions result in deeper insights. Closed questions provide clarification and detail, but no unexpected insights. When conducting user research, asking questions helps you uncover insights. However, how you ask questions impacts what and how much you can discover.
What's the difference between closed-ended and open-ended questions? Closed-ended, or restricted-choice, questions offer respondents a fixed set of choices to select from. These questions are easier to answer quickly.
Open Thesis. one that does not list all the points the writer intends to cover in an essay. Counter argument Thesis. a summary of a counter argument usually qualified by although or but precedes the writer's opinion. Study with Quizlet and memorize flashcards containing terms like Closed Thesis, limits, Open Thesis and more.
SAMPLE PRELIMINARY OUTLINE. Education: Why does it seem everyone is against it? Thesis Statement: Poverty, segregation of minorities, and changes in. federal funding policies negatively affect education. I. The schools from low-income communities lack revenues. A. Limited resources. 1.
Open-Ended vs. Closed-Ended Questions Do you ever feel like stakeholders undervalue your organization's impactful programs? Clearly communicating program success can be a challenge, leading to a disconnect and funding difficulties. This article tackles a critical tool for success: the art of crafting the right questions.
Open vs. Closed Thesis Statements - Free download as PDF File (.pdf), Text File (.txt) or read online for free.
individual practice Write three thesis statements for your topic. You should have an OPEN, CLOSED, and COUNTERARGUMENT THESIS STATEMENT.
Open vs. Closed Dissertation Defenses - pros and cons? My department is considering switching from a closed to open format and is seeking feedback from students and faculty. I'm not sure what to think - on one hand, if open, your family and friends can be there, but they can also witness you getting grilled and possibly humiliated.
English 12 31 March, 2010 Education: Why does it seem everyone is against it? Thesis Statement: Poverty, segregation of minorities, and changes in federal funding policies negatively affect education. I. The schools from low-income communities lack the revenues to provide students with equal opportunities.
The primary difference between open and closed systems lies in access control. Open systems allow users to create their own accounts, which can introduce security risks and compliance challenges. On the other hand, closed systems require administrative approval for access, providing a higher level of control over data integrity and security.