Information
- Author Services

Initiatives
You are accessing a machine-readable page. In order to be human-readable, please install an RSS reader.
All articles published by MDPI are made immediately available worldwide under an open access license. No special permission is required to reuse all or part of the article published by MDPI, including figures and tables. For articles published under an open access Creative Common CC BY license, any part of the article may be reused without permission provided that the original article is clearly cited. For more information, please refer to https://www.mdpi.com/openaccess .
Feature papers represent the most advanced research with significant potential for high impact in the field. A Feature Paper should be a substantial original Article that involves several techniques or approaches, provides an outlook for future research directions and describes possible research applications.
Feature papers are submitted upon individual invitation or recommendation by the scientific editors and must receive positive feedback from the reviewers.
Editor’s Choice articles are based on recommendations by the scientific editors of MDPI journals from around the world. Editors select a small number of articles recently published in the journal that they believe will be particularly interesting to readers, or important in the respective research area. The aim is to provide a snapshot of some of the most exciting work published in the various research areas of the journal.
Original Submission Date Received: .
- Active Journals
- Find a Journal
- Proceedings Series
- For Authors
- For Reviewers
- For Editors
- For Librarians
- For Publishers
- For Societies
- For Conference Organizers
- Open Access Policy
- Institutional Open Access Program
- Special Issues Guidelines
- Editorial Process
- Research and Publication Ethics
- Article Processing Charges
- Testimonials
- Preprints.org
- SciProfiles
- Encyclopedia
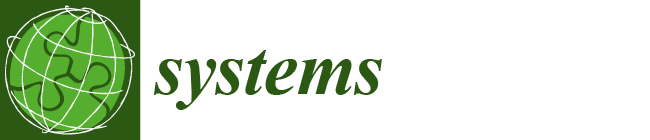
Article Menu

- Subscribe SciFeed
- Recommended Articles
- Google Scholar
- on Google Scholar
- Table of Contents
Find support for a specific problem in the support section of our website.
Please let us know what you think of our products and services.
Visit our dedicated information section to learn more about MDPI.
JSmol Viewer
A comprehensive review of recent research trends on unmanned aerial vehicles (uavs).
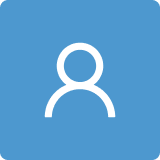
1. Introduction
- A comprehensive collection of relevant references related to the drone field, serving as a reliable and accessible source for researchers in this domain.
- Insights and predictions established through a rigorous scientific approach regarding the most active and rapidly expanding research directions in the UAV field over the past three years. The analysis is based on growth rate per year and acceleration, supported by robust evidence.
- Identification of potential UAV open development axes, offering valuable insights and ideas for future research directions. A systematic address of the consideration for the appropriate control algorithm of UAVs, providing an in-depth analysis of this critical aspect of UAV research.
- An overview of high-level UAV development software achieved through a systematic classification process, serving as an accessible guide to the available options in this area of UAV research.
- A rigorous extraction of the most prominent research directions in the UAV domain over the past three years, employing a scientifically sound methodology for a comprehensive understanding of the current state-of-the-art in UAV research.
- Presentation of a numerical analysis of the interrelationships among UAV research directions, offering clear insights into the current landscape of UAV research, facilitating the effective charting of future UAV research efforts.
2. Popular UAV Classification in Research
- Flying principle: This category includes fixed-wing, rotary-wing, hybrid, flapping-wing, and other types of UAVs that differ in their flying mechanism.
- Mission: UAVs can be classified based on their mission, such as reconnaissance, surveillance, attack, transport, search and rescue, and more.
- Weight: UAVs can be classified based on their weight, such as micro-UAVs, small UAVs, tactical UAVs, medium-altitude-long-endurance (MALE) UAVs, high-altitude-long-endurance (HALE) UAVs, and more.
- Propulsion: UAVs can be powered by electric, fuel, solar, or other sources.
- Control: UAVs can be remotely piloted, autonomous, semi-autonomous, or have other types of control.
- Altitude range: UAVs can be classified based on their altitude range, such as low-altitude UAVs, high-altitude UAVs, and stratospheric UAVs.
- Configuration: UAVs can have different configurations, such as mono-rotor, multi-rotor, tilt-rotor, tilt-wing, and others.
- Purpose: UAVs can have different purposes, such as military, civilian, commercial, industrial, scientific, and more.
- Launch method: UAVs can be launched from the ground, air, sea, or have other types of launch methods.
- Payload: UAVs can carry various payloads, such as sensors, cameras, communication systems, weapons, cargo, and others.
- Autonomy level: UAVs can have different levels of autonomy, such as fully autonomous, semi-autonomous, human-operated, and others.
- Size: UAVs can have different sizes, such as mini-UAVs, handheld UAVs, man-portable UAVs, vehicle-mounted UAVs, and more.
- Endurance: UAVs can have different endurance levels, such as short-endurance UAVs, long-endurance UAVs, ultra-long-endurance UAVs, and more.
- Range: UAVs can have different range levels, such as short-range UAVs, intermediate-range UAVs, long-range UAVs, and more.
3. Navigating the Latest UAV Research Challenges
3.1. communication and antennas, 3.3. aircraft detection, 3.4. control and autonomous flight, 3.5. perception and sensing, 3.6. energy-efficient flight, 3.7. human–uav interaction, 3.8. swarm behavior, 4. active and expanding uav research directions, 5. potentially open research directions for uavs, 5.1. integration of ai, generative ai and chatgpt for uavs, 5.2. environmental monitoring and conservation, 5.3. urban air mobility (uam), 5.4. miniaturization, 5.5. swarming and cooperative control, 5.6. beyond visual line-of-sight (bvlos) operations, 5.7. long-range and high-altitude flights, 5.8. flight safety, 5.9. uav suspension payload capabilities, 5.10. transformability or convertibility, 6. advancements in aircraft control: an overview of the development axes, 6.1. classical control, 6.2. modern control, 6.3. intelligent control, 6.4. adaptive control, 6.5. pushing the boundaries of uav control: exploring advanced techniques, 6.6. considerations for selecting an appropriate control algorithm.
- Stability and control: The algorithm should ensure the UAV’s stability and controllability, even under turbulent or challenging conditions.
- Performance: The algorithm should enable the UAV to achieve its performance objectives, such as speed, altitude, and maneuverability while minimizing power consumption and optimizing mission duration.
- Sensitivity to environment: The algorithm should consider environmental factors that can affect the UAV’s performance, such as wind, temperature, and humidity.
- Responsiveness: The algorithm should be capable of responding quickly to changes in the UAV’s mission objectives or unexpected events, such as obstacles or other aircraft.
- Computational requirements: The algorithm should be computationally efficient and feasible for the available onboard processing hardware and software.
- Robustness: The algorithm should be robust to uncertainties, such as sensor noise or errors in the UAV’s kinematic model.
- Safety: The algorithm should ensure that the UAV operates safely and avoids collisions with other objects, people, or animals.
- Regulatory compliance: The algorithm should comply with local regulations and guidelines for UAV operations, such as flight altitude restrictions and flight path limitations.
- Human interaction: The algorithm should enable human operators to interact with the UAV and provide inputs or commands, if necessary.
7. Fundamental Hardware/Software Architectures for UAVs: Applications and Issues
7.1. the hardware architecture of uavs, 7.1.1. flight computer and controller, 7.1.2. sensors, 7.1.3. actuators, 7.1.4. battery, 7.1.5. communication interfaces, 7.1.6. payload, 7.1.7. structural components, 7.2. the software architecture for uavs, 7.3. uav applications and main issues, 8. key trends: open source uav software and hardware projects, 8.1. px4 autopilot, 8.2. ardupilot, 8.3. tensorflow for uav, 8.4. paparazzi uav, 8.5. ground control, 8.6. airsim, 8.7. jderobot uavs, 8.8. dronekit and dronekit-python, 8.9. mavlink, 8.10. ros for uavs, 9. high-level uav development software and categorization, 9.1. simulation software, 9.2. flight control software, 9.3. ground control software, 9.4. computer vision software, 9.5. sensor integration software, 10. open issues and future research directions for uavs, 10.1. open issues, 10.2. future research directions, 11. conclusions, author contributions, institutional review board statement, data availability statement, conflicts of interest.
- Himeur, Y.; Al-Maadeed, S.; Varlamis, I.; Al-Maadeed, N.; Abualsaud, K.; Mohamed, A. Face mask detection in smart cities using deep and transfer learning: Lessons learned from the COVID-19 pandemic. Systems 2023 , 11 , 107. [ Google Scholar ]
- Kheddar, H.; Himeur, Y.; Awad, A.I. Deep Transfer Learning Applications in Intrusion Detection Systems: A Comprehensive Review. arXiv 2023 , arXiv:2304.10550. [ Google Scholar ]
- Atalla, S.; Daradkeh, M.; Gawanmeh, A.; Khalil, H.; Mansoor, W.; Miniaoui, S.; Himeur, Y. An Intelligent Recommendation System for Automating Academic Advising Based on Curriculum Analysis and Performance Modeling. Mathematics 2023 , 11 , 1098. [ Google Scholar ] [ CrossRef ]
- Copiaco, A.; Himeur, Y.; Amira, A.; Mansoor, W.; Fadli, F.; Atalla, S.; Sohail, S.S. An innovative deep anomaly detection of building energy consumption using energy time-series images. Eng. Appl. Artif. Intell. 2023 , 119 , 105775. [ Google Scholar ] [ CrossRef ]
- Himeur, Y.; Elnour, M.; Fadli, F.; Meskin, N.; Petri, I.; Rezgui, Y.; Bensaali, F.; Amira, A. Next-generation energy systems for sustainable smart cities: Roles of transfer learning. Sustain. Cities Soc. 2022 , 85 , 104059. [ Google Scholar ] [ CrossRef ]
- Elnour, M.; Fadli, F.; Himeur, Y.; Petri, I.; Rezgui, Y.; Meskin, N.; Ahmad, A.M. Performance and energy optimization of building automation and management systems: Towards smart sustainable carbon-neutral sports facilities. Renew. Sustain. Energy Rev. 2022 , 162 , 112401. [ Google Scholar ] [ CrossRef ]
- Kheddar, H.; Himeur, Y.; Al-Maadeed, S.; Amira, A.; Bensaali, F. Deep Transfer Learning for Automatic Speech Recognition: Towards Better Generalization. arXiv 2023 , arXiv:2304.14535. [ Google Scholar ] [ CrossRef ]
- Atalla, S.; Tarapiah, S.; Gawanmeh, A.; Daradkeh, M.; Mukhtar, H.; Himeur, Y.; Mansoor, W.; Hashim, K.F.B.; Daadoo, M. IoT-Enabled Precision Agriculture: Developing an Ecosystem for Optimized Crop Management. Information 2023 , 14 , 205. [ Google Scholar ] [ CrossRef ]
- Al-Hasan, T.M.; Shibeika, A.S.; Attique, U.; Bensaali, F.; Himeur, Y. Smart Speed Camera Based on Automatic Number Plate Recognition for Residential Compounds and Institutions Inside Qatar. In Proceedings of the 2022 5th International Conference on Signal Processing and Information Security (ICSPIS), Dubai, United Arab Emirates, 7–8 December 2022; pp. 42–45. [ Google Scholar ]
- Khalife, J.; Kassas, Z.M. On the achievability of submeter-accurate UAV navigation with cellular signals exploiting loose network synchronization. IEEE Trans. Aerosp. Electron. Syst. 2022 , 58 , 4261–4278. [ Google Scholar ] [ CrossRef ]
- Elharrouss, O.; Al-Maadeed, S.; Subramanian, N.; Ottakath, N.; Almaadeed, N.; Himeur, Y. Panoptic segmentation: A review. arXiv 2021 , arXiv:2111.10250. [ Google Scholar ]
- Liu, W.; Zhang, T.; Huang, S.; Li, K. A hybrid optimization framework for UAV reconnaissance mission planning. Comput. Ind. Eng. 2022 , 173 , 108653. [ Google Scholar ] [ CrossRef ]
- Ahmed, F.; Mohanta, J.; Keshari, A.; Yadav, P.S. Recent Advances in Unmanned Aerial Vehicles: A Review. Arab. J. Sci. Eng. 2022 , 47 , 7963–7984. [ Google Scholar ] [ CrossRef ]
- Ghazbi, S.N.; Aghli, Y.; Alimohammadi, M.; Akbari, A.A. Quadrotors unmanned aerial vehicles: A review. Int. J. Smart Sens. Intell. Syst. 2016 , 9 , 309–333. [ Google Scholar ] [ CrossRef ]
- Ismail, M.S.; Ahmad, A.; Ismail, S.; Yusop, N.M.M. A review on Unmanned Aerial Vehicle (UAV) threats assessments. In Proceedings of the AIP Conference, Kuala Lumpur, Malaysia, 23 March 2021; Volume 2617, p. 050007. [ Google Scholar ]
- Macrina, G.; Pugliese, L.D.P.; Guerriero, F.; Laporte, G. Drone-aided routing: A literature review. Transp. Res. Part C Emerg. Technol. 2020 , 120 , 102762. [ Google Scholar ] [ CrossRef ]
- Zhang, R.; Zhang, J.; Yu, H. Review of modeling and control in UAV autonomous maneuvering flight. In Proceedings of the 2018 IEEE International Conference on Mechatronics and Automation (ICMA), Changchun, China, 5–8 August 2018; pp. 1920–1925. [ Google Scholar ]
- Liang, H.; Lee, S.C.; Bae, W.; Kim, J.; Seo, S. Towards UAVs in Construction: Advancements, Challenges, and Future Directions for Monitoring and Inspection. Drones 2023 , 7 , 202. [ Google Scholar ] [ CrossRef ]
- Pina, P.; Vieira, G. UAVs for science in Antarctica. Remote Sens. 2022 , 14 , 1610. [ Google Scholar ] [ CrossRef ]
- Fan, B.; Li, Y.; Zhang, R.; Fu, Q. Review on the technological development and application of UAV systems. Chin. J. Electron. 2020 , 29 , 199–207. [ Google Scholar ] [ CrossRef ]
- Pasha, J.; Elmi, Z.; Purkayastha, S.; Fathollahi-Fard, A.M.; Ge, Y.E.; Lau, Y.Y.; Dulebenets, M.A. The drone scheduling problem: A systematic state-of-the-art review. IEEE Trans. Intell. Transp. Syst. 2022 , 23 , 14224–14247. [ Google Scholar ] [ CrossRef ]
- Mészarós, J. Aerial surveying UAV based on open-source hardware and software. Int. Arch. Photogramm. Remote Sens. Spat. Inf. Sci. 2012 , 38 , 155–159. [ Google Scholar ] [ CrossRef ] [ Green Version ]
- Ebeid, E.; Skriver, M.; Terkildsen, K.H.; Jensen, K.; Schultz, U.P. A survey of open-source UAV flight controllers and flight simulators. Microprocess. Microsyst. 2018 , 61 , 11–20. [ Google Scholar ] [ CrossRef ]
- Rojas-Perez, L.O.; Martínez-Carranza, J. On-board processing for autonomous drone racing: An overview. Integration 2021 , 80 , 46–59. [ Google Scholar ] [ CrossRef ]
- Aabid, A.; Parveez, B.; Parveen, N.; Khan, S.A.; Zayan, J.; Shabbir, O. Reviews on design and development of unmanned aerial vehicle (drone) for different applications. J. Mech. Eng. Res. Dev. 2022 , 45 , 53–69. [ Google Scholar ]
- Galimov, M.; Fedorenko, R.; Klimchik, A. Uav positioning mechanisms in landing stations: Classification and engineering design review. Sensors 2020 , 20 , 3648. [ Google Scholar ] [ CrossRef ] [ PubMed ]
- Amin, R.; Aijun, L.; Shamshirband, S. A review of quadrotor UAV: Control methodologies and performance evaluation. Int. J. Autom. Control 2016 , 10 , 87–103. [ Google Scholar ] [ CrossRef ]
- Campion, M.; Ranganathan, P.; Faruque, S. UAV swarm communication and control architectures: A review. J. Unmanned Veh. Syst. 2018 , 7 , 93–106. [ Google Scholar ] [ CrossRef ]
- Nawaz, H.; Ali, H.M.; Laghari, A.A. UAV communication networks issues: A review. Arch. Comput. Methods Eng. 2021 , 28 , 1349–1369. [ Google Scholar ] [ CrossRef ]
- Do, Q.T.; Lakew, D.S.; Tran, A.T.; Hua, D.T.; Cho, S. A Review on Recent Approaches in mmWave UAV-aided Communication Networks and Open Issues. In Proceedings of the 2023 International Conference on Information Networking (ICOIN), Bangkok, Thailand, 11–14 January 2023; pp. 728–731. [ Google Scholar ]
- Singh, R.; Ballal, K.D.; Berger, M.S.; Dittmann, L. Overview of Drone Communication Requirements in 5G. In Proceedings of the Internet of Things: 5th The Global IoT Summit, GIoTS 2022, Revised Selected Papers, Dublin, Ireland, 20–23 June 2022; pp. 3–16. [ Google Scholar ]
- Osco, L.P.; Junior, J.M.; Ramos, A.P.M.; de Castro Jorge, L.A.; Fatholahi, S.N.; de Andrade Silva, J.; Matsubara, E.T.; Pistori, H.; Gonçalves, W.N.; Li, J. A review on deep learning in UAV remote sensing. Int. J. Appl. Earth Obs. Geoinf. 2021 , 102 , 102456. [ Google Scholar ] [ CrossRef ]
- Puente-Castro, A.; Rivero, D.; Pazos, A.; Fernandez-Blanco, E. A review of artificial intelligence applied to path planning in UAV swarms. Neural Comput. Appl. 2022 , 34 , 153–170. [ Google Scholar ] [ CrossRef ]
- Chen, C.; Zheng, Z.; Xu, T.; Guo, S.; Feng, S.; Yao, W.; Lan, Y. YOLO-Based UAV Technology: A Review of the Research and Its Applications. Drones 2023 , 7 , 190. [ Google Scholar ] [ CrossRef ]
- Othman, N.A.; Aydin, I. Development of a Novel Lightweight CNN Model for Classification of Human Actions in UAV-Captured Videos. Drones 2023 , 7 , 148. [ Google Scholar ] [ CrossRef ]
- Hassanalian, M.; Abdelkefi, A. Classifications, applications, and design challenges of drones: A review. Prog. Aerosp. Sci. 2017 , 91 , 99–131. [ Google Scholar ] [ CrossRef ]
- Fudala, K.; Bialik, R.J. The use of drone-based aerial photogrammetry in population monitoring of Southern Giant Petrels in ASMA 1, King George Island, maritime Antarctica. Glob. Ecol. Conserv. 2022 , 33 , e01990. [ Google Scholar ] [ CrossRef ]
- Ventura, D.; Bonifazi, A.; Gravina, M.F.; Ardizzone, G.D. Unmanned aerial systems (UASs) for environmental monitoring: A review with applications in coastal habitats. In Aerial Robots-Aerodynamics, Control and Applications ; Intech: Rijeka, Croatia, 2017; pp. 165–184. [ Google Scholar ]
- Yang, Z.; Yu, X.; Dedman, S.; Rosso, M.; Zhu, J.; Yang, J.; Xia, Y.; Tian, Y.; Zhang, G.; Wang, J. UAV remote sensing applications in marine monitoring: Knowledge visualization and review. Sci. Total Environ. 2022 , 838 , 155939. [ Google Scholar ] [ CrossRef ]
- Kabir, R.H.; Lee, K. Wildlife monitoring using a multi-uav system with optimal transport theory. Appl. Sci. 2021 , 11 , 4070. [ Google Scholar ] [ CrossRef ]
- Mangewa, L.J.; Ndakidemi, P.A.; Munishi, L.K. Integrating UAV technology in an ecological monitoring system for community wildlife management areas in Tanzania. Sustainability 2019 , 11 , 6116. [ Google Scholar ] [ CrossRef ] [ Green Version ]
- Zmarz, A.; Rodzewicz, M.; Dąbski, M.; Karsznia, I.; Korczak-Abshire, M.; Chwedorzewska, K.J. Application of UAV BVLOS remote sensing data for multi-faceted analysis of Antarctic ecosystem. Remote Sens. Environ. 2018 , 217 , 375–388. [ Google Scholar ] [ CrossRef ]
- Ezequiel, C.A.F.; Cua, M.; Libatique, N.C.; Tangonan, G.L.; Alampay, R.; Labuguen, R.T.; Favila, C.M.; Honrado, J.L.E.; Canos, V.; Devaney, C.; et al. UAV aerial imaging applications for post-disaster assessment, environmental management and infrastructure development. In Proceedings of the 2014 International Conference on Unmanned Aircraft Systems (ICUAS), Orlando, FL, USA, 27–30 May 2014; pp. 274–283. [ Google Scholar ]
- Fumian, F.; Di Giovanni, D.; Martellucci, L.; Rossi, R.; Gaudio, P. Application of miniaturized sensors to unmanned aerial systems, a new pathway for the survey of polluted areas: Preliminary results. Atmosphere 2020 , 11 , 471. [ Google Scholar ] [ CrossRef ]
- Patnaik, K.; Zhang, W. Towards reconfigurable and flexible multirotors: A literature survey and discussion on potential challenges. Int. J. Intell. Robot. Appl. 2021 , 5 , 365–380. [ Google Scholar ] [ CrossRef ]
- Ta, D.A.; Fantoni, I.; Lozano, R. Modeling and control of a convertible mini-UAV. IFAC Proc. Vol. 2011 , 44 , 1492–1497. [ Google Scholar ] [ CrossRef ] [ Green Version ]
- da Silva Ferreira, M.A.; Begazo, M.F.T.; Lopes, G.C.; de Oliveira, A.F.; Colombini, E.L.; da Silva Simões, A. Drone reconfigurable architecture (dra): A multipurpose modular architecture for unmanned aerial vehicles (uavs). J. Intell. Robot. Syst. 2020 , 99 , 517–534. [ Google Scholar ] [ CrossRef ]
- Schiano, F.; Kornatowski, P.M.; Cencetti, L.; Floreano, D. Reconfigurable drone system for transportation of parcels with variable mass and size. IEEE Robot. Autom. Lett. 2022 , 7 , 12150–12157. [ Google Scholar ] [ CrossRef ]
- Singhal, G.; Bansod, B.; Mathew, L. Unmanned aerial vehicle classification, applications and challenges: A review. Preprints 2018 . [ Google Scholar ] [ CrossRef ] [ Green Version ]
- Mohsan, S.A.H.; Othman, N.Q.H.; Li, Y.; Alsharif, M.H.; Khan, M.A. Unmanned aerial vehicles (UAVs): Practical aspects, applications, open challenges, security issues, and future trends. Intell. Serv. Robot. 2023 , 16 , 109–137. [ Google Scholar ] [ CrossRef ]
- Arafat, M.Y.; Alam, M.M.; Moh, S. Vision-based navigation techniques for unmanned aerial vehicles: Review and challenges. Drones 2023 , 7 , 89. [ Google Scholar ] [ CrossRef ]
- Kapoor, R.; Shukla, A.; Goyal, V. Unmanned aerial vehicle (UAV) communications using multiple antennas. In Proceedings of the Advanced Computational Paradigms and Hybrid Intelligent Computing: Proceedings of ICACCP 2021 ; Springer: Singapore, 2022; pp. 261–272. [ Google Scholar ]
- Yan, C.; Fu, L.; Zhang, J.; Wang, J. A comprehensive survey on UAV communication channel modeling. IEEE Access 2019 , 7 , 107769–107792. [ Google Scholar ] [ CrossRef ]
- Song, Q.; Zeng, Y.; Xu, J.; Jin, S. A survey of prototype and experiment for UAV communications. Sci. China Inf. Sci. 2021 , 64 , 1–21. [ Google Scholar ] [ CrossRef ]
- Zhao, J.; Gao, F.; Ding, G.; Zhang, T.; Jia, W.; Nallanathan, A. Integrating communications and control for UAV systems: Opportunities and challenges. IEEE Access 2018 , 6 , 67519–67527. [ Google Scholar ] [ CrossRef ]
- Mozaffari, M.; Saad, W.; Bennis, M.; Debbah, M. Drone-based antenna array for service time minimization in wireless networks. In Proceedings of the 2018 IEEE International Conference on Communications (ICC), Kansas City, MO, USA, 20–24 May 2018; pp. 1–6. [ Google Scholar ]
- Khan, M.M.; Hossain, S.; Majumder, P.; Akter, S.; Ashique, R.H. A review on machine learning and deep learning for various antenna design applications. Heliyon 2022 , 8 , E09317. [ Google Scholar ] [ CrossRef ]
- Wu, C.; Lai, C.F. A survey on improving the wireless communication with adaptive antenna selection by intelligent method. Comput. Commun. 2022 , 181 , 374–403. [ Google Scholar ] [ CrossRef ]
- Kapoor, R.; Shukla, A.; Goyal, V. Analysis of multiple antenna techniques for unmanned aerial vehicle (UAV) communication. In Proceedings of the IOT with Smart Systems: Proceedings of ICTIS 2021 ; Springer: Singapore, 2022; Volume 2, pp. 347–357. [ Google Scholar ]
- Parvaresh, N.; Kulhandjian, M.; Kulhandjian, H.; D’Amours, C.; Kantarci, B. A tutorial on AI-powered 3D deployment of drone base stations: State of the art, applications and challenges. Veh. Commun. 2022 , 36 , 100474. [ Google Scholar ] [ CrossRef ]
- Shi, L.; Marcano, N.J.H.; Jacobsen, R.H. A review on communication protocols for autonomous unmanned aerial vehicles for inspection application. Microprocess. Microsyst. 2021 , 86 , 104340. [ Google Scholar ] [ CrossRef ]
- Khan, M.; Qureshi, I.; Khanzada, F. A hybrid communication scheme for efficient and low-cost deployment of future flying ad hoc network (FANET). Drones 2019 , 3 , 16. [ Google Scholar ] [ CrossRef ] [ Green Version ]
- Brown, T.; Argrow, B.; Dixon, C.; Doshi, S.; Thekkekunnel, R.G.; Henkel, D. Ad hoc UAV ground network (AUGNet). In Proceedings of the AIAA 3rd “ Unmanned Unlimited” Technical Conference, Workshop and Exhibit, Chicago, IL, USA, 20–23 September 2004; p. 6321. [ Google Scholar ]
- Yanmaz, E.; Hayat, S.; Scherer, J.; Bettstetter, C. Experimental performance analysis of two-hop aerial 802.11 networks. In Proceedings of the 2014 IEEE Wireless Communications and Networking Conference (WCNC), Istanbul, Turkey, 6–9 April 2014; pp. 3118–3123. [ Google Scholar ]
- Asadpour, M.; Giustiniano, D.; Hummel, K.A.; Heimlicher, S.; Egli, S. Now or later? delaying data transfer in time-critical aerial communication. In Proceedings of the Ninth ACM conference on Emerging Networking Experiments and Technologies, Paris, France, 5–8 December 2013; pp. 127–132. [ Google Scholar ]
- Morgenthaler, S.; Braun, T.; Zhao, Z.; Staub, T.; Anwander, M. UAVNet: A mobile wireless mesh network using unmanned aerial vehicles. In Proceedings of the 2012 IEEE Globecom Workshops, Anaheim, CA, USA, 3–7 December 2012; pp. 1603–1608. [ Google Scholar ]
- Lv, F.; Zhu, H.; Xue, H.; Zhu, Y.; Chang, S.; Dong, M.; Li, M. An empirical study on urban IEEE 802.11 p vehicle-to-vehicle communication. In Proceedings of the 2016 13th Annual IEEE International Conference on Sensing, Communication, and Networking (SECON), London, UK, 27–30 June 2016; pp. 1–9. [ Google Scholar ]
- Pereira, D.S.; De Morais, M.R.; Nascimento, L.B.; Alsina, P.J.; Santos, V.G.; Fernandes, D.H.; Silva, M.R. Zigbee protocol-based communication network for multi-unmanned aerial vehicle networks. IEEE Access 2020 , 8 , 57762–57771. [ Google Scholar ] [ CrossRef ]
- Ferreira, L.C.; Claudio Batista Da Silva, L.; Ferreira Rosa, P.F. Performance evaluation of LoRa and ZigBee technologies applied to FANETs. In Proceedings of the Latin American Robotics Symposium (LARS), 2021 Brazilian Symposium on Robotics (SBR), and 2021 Workshop on Robotics in Education (WRE), Natal, Brazil, 11–15 October 2021; pp. 48–53. [ Google Scholar ] [ CrossRef ]
- QasMarrogy, G.A.; Fadhil, A.J. A Comparative Study of Different FANET 802.11 Wireless Protocols with Different Data Loads. Polytech. J. 2022 , 12 , 61–66. [ Google Scholar ] [ CrossRef ]
- Paredes, W.D.; Kaushal, H.; Vakilinia, I.; Prodanoff, Z. LoRa Technology in Flying Ad Hoc Networks: A Survey of Challenges and Open Issues. Sensors 2023 , 23 , 2403. [ Google Scholar ] [ CrossRef ]
- Noor, F.; Khan, M.A.; Al-Zahrani, A.; Ullah, I.; Al-Dhlan, K.A. A review on communications perspective of flying ad hoc networks: Key enabling wireless technologies, applications, challenges and open research topics. Drones 2020 , 4 , 65. [ Google Scholar ] [ CrossRef ]
- Martinez-Caro, J.M.; Cano, M.D. IoT system integrating unmanned aerial vehicles and LoRa technology: A performance evaluation study. Wirel. Commun. Mob. Comput. 2019 , 2019 , 4307925. [ Google Scholar ] [ CrossRef ] [ Green Version ]
- Zhu, L.; Yin, D.; Yang, J.; Shen, L. Research of remote measurement and control technology of UAV based on mobile communication networks. In Proceedings of the IEEE International Conference on Information and Automation, Lijiang, China, 8–10 August 2015; pp. 2517–2522. [ Google Scholar ]
- Xu, Y.; Gui, G. Optimal resource allocation for wireless powered multi-carrier backscatter communication networks. IEEE Wirel. Commun. Lett. 2020 , 9 , 1191–1195. [ Google Scholar ] [ CrossRef ]
- Nguyen, H.C.; Amorim, R.; Wigard, J.; Kovács, I.Z.; Sørensen, T.B.; Mogensen, P.E. How to ensure reliable connectivity for aerial vehicles over cellular networks. IEEE Access 2018 , 6 , 12304–12317. [ Google Scholar ] [ CrossRef ]
- Hosseini, N.; Jamal, H.; Haque, J.; Magesacher, T.; Matolak, D.W. UAV command and control, navigation and surveillance: A review of potential 5G and satellite systems. In Proceedings of the 2019 IEEE Aerospace Conference, Big Sky, MT, USA, 2–9 March 2019; pp. 1–10. [ Google Scholar ]
- Colajanni, G.; Daniele, P.; Galluccio, L.; Grasso, C.; Schembra, G. Service Chain Placement Optimization in 5G FANET-Based Network Edge. IEEE Commun. Mag. 2022 , 60 , 60–65. [ Google Scholar ] [ CrossRef ]
- Amponis, G.; Lagkas, T.; Zevgara, M.; Katsikas, G.; Xirofotos, T.; Moscholios, I.; Sarigiannidis, P. Drones in B5G/6G networks as flying base stations. Drones 2022 , 6 , 39. [ Google Scholar ] [ CrossRef ]
- Grasso, C.; Raftopoulos, R.; Schembra, G. Tailoring fanet-based 6g network slices in remote areas for low-latency applications. Procedia Comput. Sci. 2022 , 203 , 69–78. [ Google Scholar ] [ CrossRef ]
- Oliveira, M.T.d.; Miranda, R.K.; da Costa, J.P.C.; de Almeida, A.L.; Sousa, R.T.d. Low cost antenna array based drone tracking device for outdoor environments. Wirel. Commun. Mob. Comput. 2019 , 2019 , 5437908. [ Google Scholar ] [ CrossRef ]
- Ganti, S.R.; Kim, Y. Design of low-cost on-board auto-tracking antenna for small UAS. In Proceedings of the 12th International Conference on Information Technology-New Generations, Las Vegas, NV, USA, 13–15 April 2015; pp. 273–279. [ Google Scholar ]
- Carneiro, A.F.A.; Torres, J.P.N.; Baptista, A.; Martins, M.J.M. Smart Antenna for Application in UAVs. Information 2018 , 9 , 328. [ Google Scholar ] [ CrossRef ] [ Green Version ]
- Abdelmaboud, A. The internet of drones: Requirements, taxonomy, recent advances, and challenges of research trends. Sensors 2021 , 21 , 5718. [ Google Scholar ] [ CrossRef ] [ PubMed ]
- Goyal, N.; Sharma, S.; Rana, A.K.; Tripathi, S.L. Internet of Things: Robotic and Drone Technology ; CRC Press: Boca Raton, FL, USA, 2022. [ Google Scholar ]
- Israr, A.; Abro, G.E.M.; Sadiq Ali Khan, M.; Farhan, M.; Bin Mohd Zulkifli, S.U.A. Internet of things (IoT)-Enabled unmanned aerial vehicles for the inspection of construction sites: A vision and future directions. Math. Probl. Eng. 2021 , 2021 , 9931112. [ Google Scholar ] [ CrossRef ]
- Aldeen, Y.; Abdulhadi, H.M. Data communication for drone-enabled internet of things. Indones. J. Electr. Eng. Comput. Sci. 2021 , 22 , 1216–1222. [ Google Scholar ] [ CrossRef ]
- Sun, Y.; Fesenko, H.; Kharchenko, V.; Zhong, L.; Kliushnikov, I.; Illiashenko, O.; Morozova, O.; Sachenko, A. UAV and IoT-Based Systems for the Monitoring of Industrial Facilities Using Digital Twins: Methodology, Reliability Models, and Application. Sensors 2022 , 22 , 6444. [ Google Scholar ] [ CrossRef ] [ PubMed ]
- Lakshman, S.A.; Ebenezer, D. Integration of internet of things and drones and its future applications. Mater. Today Proc. 2021 , 47 , 944–949. [ Google Scholar ] [ CrossRef ]
- Shen, S.; Mulgaonkar, Y.; Michael, N.; Kumar, V. Multi-sensor fusion for robust autonomous flight in indoor and outdoor environments with a rotorcraft MAV. In Proceedings of the IEEE International Conference on Robotics and Automation (ICRA), Hong Kong, China, 1 May–7 June 2014; pp. 4974–4981. [ Google Scholar ]
- Xin, L.; Tang, Z.; Gai, W.; Liu, H. Vision-Based Autonomous Landing for the UAV: A Review. Aerospace 2022 , 9 , 634. [ Google Scholar ] [ CrossRef ]
- Choi, S.Y.; Cha, D. Unmanned aerial vehicles using machine learning for autonomous flight; state-of-the-art. Adv. Robot. 2019 , 33 , 265–277. [ Google Scholar ] [ CrossRef ]
- Noor, M.Y.B.M.; Ismail, M.; b Khyasudeen, M.F.; Shariffuddin, A.; Kamel, N.; Azzuhri, S.R. Autonomous precision landing for commercial UAV: A review. Fuzzy Syst. Data Min. III Proc. Fsdm 2017 , 298 , 459–468. [ Google Scholar ]
- Zulu, A.; John, S. A review of control algorithms for autonomous quadrotors. arXiv 2016 , arXiv:1602.02622. [ Google Scholar ] [ CrossRef ] [ Green Version ]
- Li, Z.; Chen, W.H.; Liu, C. Review of UAV-Based Autonomous Search Algorithms for Hazardous Sources. Loughb. Univ. Sci. Sin. Inform. 2016 , 52 , 1579. [ Google Scholar ] [ CrossRef ]
- Crommelinck, S.; Bennett, R.; Gerke, M.; Nex, F.; Yang, M.Y.; Vosselman, G. Review of automatic feature extraction from high-resolution optical sensor data for UAV-based cadastral mapping. Remote Sens. 2016 , 8 , 689. [ Google Scholar ] [ CrossRef ] [ Green Version ]
- Corradi, F.; Fioranelli, F. Radar Perception for Autonomous Unmanned Aerial Vehicles: A Survey. Syst. Eng. Constrained Embed. Syst. 2022 , 14–20. [ Google Scholar ]
- Sun, Z.; Wang, X.; Wang, Z.; Yang, L.; Xie, Y.; Huang, Y. UAVs as remote sensing platforms in plant ecology: Review of applications and challenges. J. Plant Ecol. 2021 , 14 , 1003–1023. [ Google Scholar ] [ CrossRef ]
- Guan, S.; Zhu, Z.; Wang, G. A review on UAV-based remote sensing technologies for construction and civil applications. Drones 2022 , 6 , 117. [ Google Scholar ] [ CrossRef ]
- Ren, H.; Zhao, Y.; Xiao, W.; Hu, Z. A review of UAV monitoring in mining areas: Current status and future perspectives. Int. J. Coal Sci. Technol. 2019 , 6 , 320–333. [ Google Scholar ] [ CrossRef ] [ Green Version ]
- Bailon-Ruiz, R.; Lacroix, S. Wildfire remote sensing with UAVs: A review from the autonomy point of view. In Proceedings of the 2020 International Conference on Unmanned Aircraft Systems (ICUAS), Athens, Greece, 1–4 September 2020; pp. 412–420. [ Google Scholar ]
- Merino, L.; Caballero, F.; Ferruz, J.; Wiklund, J.; Forssén, P.E.; Ollero, A. Multi-UAV cooperative perception techniques. Mult. Heterog. Unmanned Aer. Veh. 2007 , 37 , 67–110. [ Google Scholar ]
- Chodnicki, M.; Siemiatkowska, B.; Stecz, W.; Stępień, S. Energy Efficient UAV Flight Control Method in an Environment with Obstacles and Gusts of Wind. Energies 2022 , 15 , 3730. [ Google Scholar ] [ CrossRef ]
- Boukoberine, M.N.; Zhou, Z.; Benbouzid, M. A critical review on unmanned aerial vehicles power supply and energy management: Solutions, strategies, and prospects. Appl. Energy 2019 , 255 , 113823. [ Google Scholar ] [ CrossRef ]
- Amici, C.; Ceresoli, F.; Pasetti, M.; Saponi, M.; Tiboni, M.; Zanoni, S. Review of propulsion system design strategies for unmanned aerial vehicles. Appl. Sci. 2021 , 11 , 5209. [ Google Scholar ] [ CrossRef ]
- Gur, O.; Rosen, A. Optimizing electric propulsion systems for unmanned aerial vehicles. J. Aircr. 2009 , 46 , 1340–1353. [ Google Scholar ] [ CrossRef ] [ Green Version ]
- Betancourth, N.J.P.; Villamarin, J.E.P.; Rios, J.J.V.; Bravo-Mosquera, P.D.; Cerón-Muñoz, H.D. Design and manufacture of a solar-powered unmanned aerial vehicle for civilian surveillance missions. J. Aerosp. Technol. Manag. 2016 , 8 , 385–396. [ Google Scholar ] [ CrossRef ] [ Green Version ]
- Rajendran, P.; Smith, H. Review of solar and battery power system development for solar-powered electric unmanned aerial vehicles. Adv. Mater. Res. 2015 , 1125 , 641–647. [ Google Scholar ] [ CrossRef ]
- Nemer, I.A.; Sheltami, T.R.; Belhaiza, S.; Mahmoud, A.S. Energy-efficient UAV movement control for fair communication coverage: A deep reinforcement learning approach. Sensors 2022 , 22 , 1919. [ Google Scholar ] [ CrossRef ]
- Xiao, T.; Wei, W.; Hongliang, H.; Zhang, R. Energy-efficient data collection for UAV-assisted IoT: Joint trajectory and resource optimization. Chin. J. Aeronaut. 2022 , 35 , 95–105. [ Google Scholar ]
- Do, H.T.; Truong, L.H.; Nguyen, M.T.; Chien, C.F.; Tran, H.T.; Hua, H.T.; Nguyen, C.V.; Nguyen, H.T.; Nguyen, N.T. Energy-efficient unmanned aerial vehicle (UAV) surveillance utilizing artificial intelligence (AI). Wirel. Commun. Mob. Comput. 2021 , 2021 , 8615367. [ Google Scholar ] [ CrossRef ]
- Jiao, R.; Wang, Z.; Chu, R.; Dong, M.; Rong, Y.; Chou, W. An intuitive end-to-end human-UAV interaction system for field exploration. Front. Neur. 2020 , 13 , 117. [ Google Scholar ] [ CrossRef ]
- Kassab, M.A.; Ahmed, M.; Maher, A.; Zhang, B. Real-time human-UAV interaction: New dataset and two novel gesture-based interacting systems. IEEE Access 2020 , 8 , 195030–195045. [ Google Scholar ] [ CrossRef ]
- Müezzinoğlu, T.; Karaköse, M. An intelligent human–unmanned aerial vehicle interaction approach in real time based on machine learning using wearable gloves. Sensors 2021 , 21 , 1766. [ Google Scholar ] [ CrossRef ]
- Chen, B.; Hua, C.; Li, D.; He, Y.; Han, J. Intelligent Human–UAV interaction system with joint cross-validation over Action–Gesture recognition and scene understanding. Appl. Sci. 2019 , 9 , 3277. [ Google Scholar ] [ CrossRef ] [ Green Version ]
- Maher, A.; Li, C.; Hu, H.; Zhang, B. Realtime human-UAV interaction using deep learning. In Proceedings of the Biometric Recognition: 12th Chinese Conference, CCBR 2017, Shenzhen, China, 28–29 October 2017; Proceedings 12. Springer: Singapore, 2017; pp. 511–519. [ Google Scholar ]
- Rajappa, S.; Bülthoff, H.; Stegagno, P. Design and implementation of a novel architecture for physical human-UAV interaction. Int. J. Robot. Res. 2017 , 36 , 800–819. [ Google Scholar ] [ CrossRef ]
- Rajappa, S. Towards Human-UAV Physical Interaction and Fully Actuated Aerial Vehicles. Ph.D. Thesis, University Tübingen, Wilhelmstrasse, Germany, 2017. [ Google Scholar ]
- Nisser, T.; Westin, C. Human Factors Challenges in Unmanned Aerial Vehicles (Uavs): A Literature Review ; School of Aviation of the Lund University: Ljungbyhed, Sweden, 2006. [ Google Scholar ]
- Hart, S.; Steane, V.; Bullock, S.; Noyes, J.M. Understanding Human Decision-Making when Controlling UAVs in a Search and Rescue Application. Hum. Interact. Emerg. Technol. 2022 , 68 . [ Google Scholar ] [ CrossRef ]
- Tezza, D.; Andujar, M. The state-of-the-art of human–drone interaction: A survey. IEEE Access 2019 , 7 , 167438–167454. [ Google Scholar ] [ CrossRef ]
- Zhou, Y.; Rao, B.; Wang, W. Uav swarm intelligence: Recent advances and future trends. IEEE Access 2020 , 8 , 183856–183878. [ Google Scholar ] [ CrossRef ]
- Khelifi, M.; Butun, I. Swarm Unmanned Aerial Vehicles (SUAVs): A Comprehensive Analysis of Localization, Recent Aspects, and Future Trends. J. Sens. 2022 , 2022 , 8600674. [ Google Scholar ] [ CrossRef ]
- Campion, M.; Ranganathan, P.; Faruque, S. A review and future directions of UAV swarm communication architectures. In Proceedings of the IEEE International Conference on Electro/Information Technology (EIT), Rochester, MI, USA, 3–5 May 2018; pp. 0903–0908. [ Google Scholar ]
- Paulsson, M. High-Level Control of UAV Swarms with RSSI Based Position Estimation. Master’s Thesis, Lund University, Lund, Sweden, 2017. [ Google Scholar ]
- KA, V.K.; Priyadarshini, R.; Kathik, P.; Madhan, E.; Sonya, A. Self-co-ordination algorithm (SCA) for multi-UAV systems using fair scheduling queue. Sens. Rev. 2022 . ahead-of-print . [ Google Scholar ] [ CrossRef ]
- Iqbal, M.M.; Ali, Z.A.; Khan, R.; Shafiq, M. Motion Planning of UAV Swarm: Recent Challenges and Approaches. In Aeronautics-New Advances ; IntechOpen: London, UK, 2022. [ Google Scholar ]
- Zhu, X.; Liu, Z.; Yang, J. Model of collaborative UAV swarm toward coordination and control mechanisms study. Procedia Comput. Sci. 2015 , 51 , 493–502. [ Google Scholar ] [ CrossRef ] [ Green Version ]
- Peng, Q.; Wu, H.; Xue, R. Review of dynamic task allocation methods for UAV swarms oriented to ground targets. Complex Syst. Model. Simul. 2021 , 1 , 163–175. [ Google Scholar ] [ CrossRef ]
- Qamar, S.; Khan, S.H.; Arshad, M.A.; Qamar, M.; Khan, A. Autonomous drone swarm navigation and multi-target tracking in 3D environments with dynamic obstacles. arXiv 2022 , arXiv:2202.06253. [ Google Scholar ]
- Taha, B.; Shoufan, A. Machine learning-based drone detection and classification: State-of-the-art in research. IEEE Access 2019 , 7 , 138669–138682. [ Google Scholar ] [ CrossRef ]
- Bhalara, R.; Shilu, R.J.; Nandi, D. A Review on Aircraft Detection Techniques and Feature Extraction Using Deep Learning. IJAEM 2020 , 7 , 305–307. [ Google Scholar ]
- Zhou, L.; Yan, H.; Shan, Y.; Zheng, C.; Liu, Y.; Zuo, X.; Qiao, B. Aircraft detection for remote sensing images based on deep convolutional neural networks. J. Electr. Comput. Eng. 2021 , 2021 , 4685644. [ Google Scholar ] [ CrossRef ]
- Kiyak, E.; Unal, G. Small aircraft detection using deep learning. Aircr. Eng. Aerosp. Technol. 2021 , 93 , 671–681. [ Google Scholar ] [ CrossRef ]
- Thoudoju, A.K. Detection of Aircraft, Vehicles and Ships in Aerial and Satellite Imagery Using Evolutionary Deep Learning. Master’s Thesis, Blekinge Institute of Technology, Karlskrona, Sweden, 2021. [ Google Scholar ]
- Ferraz, M.F.; Júnior, L.B.; Komori, A.S.; Rech, L.C.; Schneider, G.H.; Berger, G.S.; Cantieri, Á.R.; Lima, J.; Wehrmeister, M.A. Artificial Intelligence Architecture Based on Planar LiDAR Scan Data to Detect Energy Pylon Structures in a UAV Autonomous Detailed Inspection Process. In Proceedings of the Optimization, Learning Algorithms and Applications: First International Conference, OL2A 2021, Bragança, Portugal, 19–21 July 2021; Revised Selected Papers 1. Springer: Berlin/Heidelberg, Germany, 2021; pp. 430–443. [ Google Scholar ]
- Himeur, Y.; Sohail, S.S.; Bensaali, F.; Amira, A.; Alazab, M. Latest trends of security and privacy in recommender systems: A comprehensive review and future perspectives. Comput. Secur. 2022 , 118 , 102746. [ Google Scholar ] [ CrossRef ]
- Hendria, W.F.; Phan, Q.T.; Adzaka, F.; Jeong, C. Combining transformer and CNN for object detection in UAV imagery. ICT Express 2021 , 98 , 258–263. [ Google Scholar ] [ CrossRef ]
- Radovic, M.; Adarkwa, O.; Wang, Q. Object recognition in aerial images using convolutional neural networks. J. Imaging 2017 , 3 , 21. [ Google Scholar ] [ CrossRef ] [ Green Version ]
- Rahnemoonfar, M.; Murphy, R.; Miquel, M.V.; Dobbs, D.; Adams, A. Flooded area detection from UAV images based on densely connected recurrent neural networks. In Proceedings of the IGARSS 2018–2018 IEEE International Geoscience and Remote Sensing Symposium, Valencia, Spain, 22–27 July 2018; pp. 1788–1791. [ Google Scholar ]
- Bejiga, M.B.; Zeggada, A.; Melgani, F. Convolutional neural networks for near real-time object detection from UAV imagery in avalanche search and rescue operations. In Proceedings of the 2016 IEEE International Geoscience and Remote Sensing Symposium (IGARSS), Beijing, China, 10–15 July 2016; pp. 693–696. [ Google Scholar ]
- Himeur, Y.; Al-Maadeed, S.; Kheddar, H.; Al-Maadeed, N.; Abualsaud, K.; Mohamed, A.; Khattab, T. Video surveillance using deep transfer learning and deep domain adaptation: Towards better generalization. Eng. Appl. Artif. Intell. 2023 , 119 , 105698. [ Google Scholar ] [ CrossRef ]
- Li, S.; Jia, Y.; Yang, F.; Qin, Q.; Gao, H.; Zhou, Y. Collaborative Decision-Making Method for Multi-UAV Based on Multiagent Reinforcement Learning. IEEE Access 2022 , 10 , 91385–91396. [ Google Scholar ] [ CrossRef ]
- Rezwan, S.; Choi, W. Artificial intelligence approaches for UAV navigation: Recent advances and future challenges. IEEE Access 2022 , 10 , 26320–26339. [ Google Scholar ] [ CrossRef ]
- Yin, R.; Li, W.; Wang, Z.Q.; Xu, X.X. The application of artificial intelligence technology in UAV. In Proceedings of the 2020 5th International Conference on Information Science, Computer Technology and Transportation (ISCTT), Shenyang, China, 13–15 November 2020; pp. 238–241. [ Google Scholar ]
- OpenAI ChatGPT. Available online: https://chat.openai.com/ (accessed on 21 July 2023).
- Sohail, S.S.; Madsen, D.; Himeur, Y.; Ashraf, M. Using ChatGPT to Navigate Ambivalent and Contradictory Research Findings on Artificial Intelligence. Available at SSRN 4413913. 2023. Available online: https://papers.ssrn.com/sol3/papers.cfm?abstract_id=4413913 (accessed on 22 July 2023).
- Zhang, B.; Soh, H. Large Language Models as Zero-Shot Human Models for Human-Robot Interaction. arXiv 2023 , arXiv:2303.03548. [ Google Scholar ]
- Liu, J.X.; Yang, Z.; Idrees, I.; Liang, S.; Schornstein, B.; Tellex, S.; Shah, A. Lang2LTL: Translating Natural Language Commands to Temporal Robot Task Specification. arXiv 2023 , arXiv:2302.11649. [ Google Scholar ]
- Sohail, S.S.; Farhat, F.; Himeur, Y.; Nadeem, M.; Madsen, D.Ø.; Singh, Y.; Atalla, S.; Mansoor, W. The Future of GPT: A Taxonomy of Existing ChatGPT Research, Current Challenges, and Possible Future Directions. Curr. Challenges Possible Future Dir. 2023 . [ Google Scholar ] [ CrossRef ]
- Hong, Y.; Wu, Q.; Qi, Y.; Rodriguez-Opazo, C.; Gould, S. A recurrent vision-and-language bert for navigation. arXiv 2020 , arXiv:2011.13922. [ Google Scholar ]
- Bucker, A.; Figueredo, L.; Haddadinl, S.; Kapoor, A.; Ma, S.; Bonatti, R. Reshaping robot trajectories using natural language commands: A study of multi-modal data alignment using transformers. In Proceedings of the 2022 IEEE/RSJ International Conference on Intelligent Robots and Systems (IROS), Kyoto, Japan, 23–27 October 2022; pp. 978–984. [ Google Scholar ]
- Vemprala, S.; Bonatti, R.; Bucker, A.; Kapoor, A. Chatgpt for robotics: Design principles and model abilities. Microsoft Auton. Syst. Robot. Res. 2023 , 2 , 20. [ Google Scholar ]
- Microsoft AirSim ChatGPT. Available online: https://youtu.be/NYd0QcZcS6Q (accessed on 22 July 2023).
- AirSim-ChatGPT, PromptCraft Code. Available online: https://github.com/microsoft/PromptCraft-Robotics (accessed on 22 July 2023).
- Microsoft AirSim ChatGPT for Industrial Inspection. Available online: https://www.youtube.com/watch?v=38lA3U2J43w&feature=youtu.be (accessed on 22 July 2023).
- Tiwary, A.; Rimal, B.; Himeur, Y.; Amira, A. Monitoring Nature-Based Engineering Projects in Mountainous Region Incorporating Spatial Imaging: Case Study of a Hydroelectric Project in Nepal. In Proceedings of the CITIES 20.50–Creating Habitats for the 3rd Millennium: Smart–Sustainable–Climate Neutral. Proceedings of REAL CORP 2021, 26th International Conference on Urban Development, Regional Planning and Information Society. CORP–Competence Center of Urban and Regional Planning, Vienna, Austria, 7–10 September 2021; pp. 535–538. [ Google Scholar ]
- Himeur, Y.; Rimal, B.; Tiwary, A.; Amira, A. Using artificial intelligence and data fusion for environmental monitoring: A review and future perspectives. Inf. Fusion 2022 , 86–87 , 44–75. [ Google Scholar ]
- Serna, J.G.; Vanegas, F.; Gonzalez, F.; Flannery, D. A review of current approaches for UAV autonomous mission planning for Mars biosignatures detection. In Proceedings of the 2020 IEEE Aerospace Conference, Big Sky, MT, USA, 7–14 March 2020; pp. 1–15. [ Google Scholar ]
- Himeur, Y.; Elnour, M.; Fadli, F.; Meskin, N.; Petri, I.; Rezgui, Y.; Bensaali, F.; Amira, A. AI-big data analytics for building automation and management systems: A survey, actual challenges and future perspectives. Artif. Intell. Rev. 2022 , 56 , 4929–5021. [ Google Scholar ] [ CrossRef ] [ PubMed ]
- Wójcik, K.A.; Bialik, R.J.; Osińska, M.; Figielski, M. Investigation of Sediment-Rich glacial meltwater plumes using a high-resolution multispectral sensor mounted on an unmanned aerial vehicle. Water 2019 , 11 , 2405. [ Google Scholar ] [ CrossRef ] [ Green Version ]
- Bauranov, A.; Rakas, J. Designing airspace for urban air mobility: A review of concepts and approaches. Prog. Aerosp. Sci. 2021 , 125 , 100726. [ Google Scholar ]
- Garrow, L.A.; German, B.J.; Leonard, C.E. Urban air mobility: A comprehensive review and comparative analysis with autonomous and electric ground transportation for informing future research. Transp. Res. Part Emerg. Technol. 2021 , 132 , 103377. [ Google Scholar ]
- Rothfeld, R.; Straubinger, A.; Fu, M.; Al Haddad, C.; Antoniou, C. Urban air mobility. In Demand for Emerging Transportation Systems ; Elsevier: Amsterdam, The Netherlands, 2020; pp. 267–284. [ Google Scholar ]
- Shrestha, R.; Oh, I.; Kim, S. A survey on operation concept, advancements, and challenging issues of urban air traffic management. Front. Future Transp. 2021 , 2 , 1. [ Google Scholar ]
- Di Giovanni, D.; Fumian, F.; Chierici, A.; Bianchelli, M.; Martellucci, L.; Carminati, G.; Malizia, A.; d’Errico, F.; Gaudio, P. Design of Miniaturized Sensors for a Mission-Oriented UAV Application: A New Pathway for Early Warning. Int. J. Saf. Secur. Eng. 2021 , 11 , 435–444. [ Google Scholar ]
- Matalonga, S.; White, S.; Hartmann, J.; Riordan, J. A review of the legal, regulatory and practical aspects needed to unlock autonomous beyond visual line of sight unmanned aircraft systems operations. J. Intell. Robot. Syst. 2022 , 106 , 10. [ Google Scholar ]
- Skowron, M.; Chmielowiec, W.; Glowacka, K.; Krupa, M.; Srebro, A. Sense and avoid for small unmanned aircraft systems: Research on methods and best practices. Proc. Inst. Mech. Eng. Part G J. Aerosp. Eng. 2019 , 233 , 6044–6062. [ Google Scholar ]
- Hartley, R.J.A.L.; Henderson, I.L.; Jackson, C.L. BVLOS Unmanned Aircraft Operations in Forest Environments. Drones 2022 , 6 , 167. [ Google Scholar ]
- Gray, J. Design Optimization of a Boundary Layer Ingestion Propulsor Using a Coupled Aeropropulsive Model. Ph.D. Thesis, University of Michigan, Ann Arbor, MI, USA, 2018. [ Google Scholar ]
- Chen, Y. Overview of Solar UAV Power System. Acad. J. Sci. Technol. 2022 , 4 , 80–82. [ Google Scholar ] [ CrossRef ]
- Pal, S.; Mishra, A.; Singh, P. Recent Trends in Long Endurance Solar Powered UAVs: A Review. Int. J. Adv. Sci. Technol. 2021 , 19 , 6009–6018. [ Google Scholar ]
- Harun, M.; Abdullah, S.; Aras, M.; Bahar, M. Collision Avoidance Control for Unmanned Autonomous Vehicles (UAV): Recent Advancements and Future Prospects ; NIScPR-CSIR: New Delhi, India, 2021. [ Google Scholar ]
- Gan, X.; Wu, Y.; Liu, P.; Wang, Q. Dynamic collision avoidance zone modeling method based on UAV emergency collision avoidance trajectory. In Proceedings of the 2020 IEEE International Conference on Artificial Intelligence and Information Systems (ICAIIS), Dalian, China, 20–22 March 2020; pp. 693–696. [ Google Scholar ]
- Kakaletsis, E.; Symeonidis, C.; Tzelepi, M.; Mademlis, I.; Tefas, A.; Nikolaidis, N.; Pitas, I. Computer vision for autonomous UAV flight safety: An overview and a vision-based safe landing pipeline example. Acm Comput. Surv. 2021 , 54 , 1–37. [ Google Scholar ]
- Hadi, G.S.; Varianto, R.; Trilaksono, B.; Budiyono, A. Autonomous UAV system development for payload dropping mission. J. Instrum. Autom. Syst. 2014 , 1 , 72–77. [ Google Scholar ] [ CrossRef ]
- Cruz, P.J.; Fierro, R. Cable-suspended load lifting by a quadrotor UAV: Hybrid model, trajectory generation, and control. Auton. Robot. 2017 , 41 , 1629–1643. [ Google Scholar ]
- Santos, M.A.; Rego, B.; Raffo, G.V.; Ferramosca, A. Suspended load path tracking control strategy using a tilt-rotor UAV. J. Adv. Transp. 2017 , 1–22. [ Google Scholar ] [ CrossRef ]
- Mohammadi, K. Passivity-Based Control of Multiple Quad-Copters with a Cable-Suspended Payload. Ph.D. Thesis, McMaster University, Hamilton, ON, Canada, 2021. [ Google Scholar ]
- Derrouaoui, S.H.; Bouzid, Y.; Guiatni, M.; Dib, I. A comprehensive review on reconfigurable drones: Classification, characteristics, design and control technologies. Unmanned Syst. 2022 , 10 , 3–29. [ Google Scholar ]
- Moosavian, A.; Xi, F.; Hashemi, S.M. Design and motion control of fully variable morphing wings. J. Aircr. 2013 , 50 , 1189–1201. [ Google Scholar ] [ CrossRef ]
- Ajanic, E.; Feroskhan, M.; Mintchev, S.; Noca, F.; Floreano, D. Bioinspired wing and tail morphing extends drone flight capabilities. Sci. Robot. 2020 , 5 , eabc2897. [ Google Scholar ] [ CrossRef ]
- Jiakun, H.; Zhe, H.; Fangbao, T.; Gang, C. Review on bio-inspired flight systems and bionic aerodynamics. Chin. J. Aeronaut. 2021 , 34 , 170–186. [ Google Scholar ]
- Kilian, L.; Shahid, F.; Zhao, J.S.; Nayeri, C.N. Bioinspired morphing wings: Mechanical design and wind tunnel experiments. Bioinspirat. Biomimet. 2022 , 17 , 046019. [ Google Scholar ] [ CrossRef ] [ PubMed ]
- Miller, E.J.; Lokos, W.A.; Cruz, J.; Crampton, G.; Stephens, C.A.; Kota, S.; Ervin, G.; Flick, P. Approach for structurally clearing an adaptive compliant trailing edge flap for flight. In Proceedings of the Society of Flight Test Engineers International Annual Symposium, Lancaster, CA, USA, 14–17 September 2015. [ Google Scholar ]
- Frigioescu, T.F.; Condruz, M.R.; Badea, T.A.; Paraschiv, A. A Preliminary Study on the Development of a New UAV Concept and the Associated Flight Method. Drones 2023 , 7 , 166. [ Google Scholar ] [ CrossRef ]
- Gökbel, E.; Güllü, A.; Ersoy, S. Improvement of UAV: Design and implementation on launchability. Aircr. Eng. Aerosp. Technol. 2023 , 95 , 734–740. [ Google Scholar ] [ CrossRef ]
- DeFrangesco, R.; DeFrangesco, S. The Big Book of Drones ; CRC Press: Boca Raton, FL, USA, 2022. [ Google Scholar ]
- Falanga, D.; Kleber, K.; Mintchev, S.; Floreano, D.; Scaramuzza, D. The foldable drone: A morphing quadrotor that can squeeze and fly. IEEE Robot. Autom. Lett. 2018 , 4 , 209–216. [ Google Scholar ] [ CrossRef ] [ Green Version ]
- The Foldable Drone, Laboratory of Intelligent Systems at EPFL. Available online: https://actu.epfl.ch/news/new-foldable-drone-flies-through-narrow-holes-in-r (accessed on 22 July 2023).
- Podsędkowski, M.; Konopiński, R.; Obidowski, D.; Koter, K. Variable pitch propeller for UAV-experimental tests. Energies 2020 , 13 , 5264. [ Google Scholar ]
- Abhishek, A.; Duhoon, A.; Kothari, M.; Kadukar, S.; Rane, L.; Suryavanshi, G. Design, development, and closed-loop flight-testing of a single power plant variable pitch quadrotor unmanned air vehicle. In Proceedings of the 73rd American Helicopter Society Annual Forum, Fort Worth, TX, USA, 9–11 May 2017; pp. 205–218. [ Google Scholar ]
- Wu, X. Design and Development of Variable Pitch Quadcopter for Long Endurance Flight. Ph.D. Thesis, Oklahoma State University, Stillwater, OK, USA, 2018. [ Google Scholar ]
- Liu, Z.; He, Y.; Yang, L.; Han, J. Control techniques of tilt rotor unmanned aerial vehicle systems: A review. Chin. J. Aeronaut. 2017 , 30 , 135–148. [ Google Scholar ]
- Misra, A.; Jayachandran, S.; Kenche, S.; Katoch, A.; Suresh, A.; Gundabattini, E.; Selvaraj, S.K.; Legesse, A.A. A Review on Vertical Take-Off and Landing (VTOL) Tilt-Rotor and Tilt Wing Unmanned Aerial Vehicles (UAVs). J. Eng. 2022 , 2022 , 1803638. [ Google Scholar ]
- Kamel, M.; Verling, S.; Elkhatib, O.; Sprecher, C.; Wulkop, P.; Taylor, Z.; Siegwart, R.; Gilitschenski, I. The voliro omniorientational hexacopter: An agile and maneuverable tiltable-rotor aerial vehicle. IEEE Robot. Autom. Mag. 2018 , 25 , 34–44. [ Google Scholar ] [ CrossRef ] [ Green Version ]
- Voliro Hexcopter, ETH Zurich Team. Available online: https://voliro.com (accessed on 22 July 2023).
- GL-10, NASA Greased Lightning. Available online: https://ntrs.nasa.gov/citations/20180000765 (accessed on 22 July 2023).
- Kangunde, V.; Jamisola, R.S.; Theophilus, E.K. A review on drones controlled in real-time. Int. J. Dyn. Control 2021 , 9 , 1832–1846. [ Google Scholar ]
- Rubí, B.; Pérez, R.; Morcego, B. A survey of path following control strategies for UAVs focused on quadrotors. J. Intell. Robot. Syst. 2020 , 98 , 241–265. [ Google Scholar ]
- Sonugur, G. A Review of quadrotor UAV: Control and SLAM methodologies ranging from conventional to innovative approaches. Robot. Auton. Syst. 2022 , 161 , 104342. [ Google Scholar ]
- Hanif, A.S.; Han, X.; Yu, S.H. Independent Control Spraying System for UAV-Based Precise Variable Sprayer: A Review. Drones 2022 , 6 , 383. [ Google Scholar ]
- Idrissi, M.; Salami, M.; Annaz, F. A review of quadrotor unmanned aerial vehicles: Applications, architectural design and control algorithms. J. Intell. Robot. Syst. 2022 , 104 , 22. [ Google Scholar ]
- López, Y.Á.; Garcia-Fernandez, M.; Alvarez-Narciandi, G.; Andrés, F.L.H. Unmanned aerial vehicle-based ground-penetrating radar systems: A review. IEEE Geosci. Remote Sens. Mag. 2022 , 10 , 66–86. [ Google Scholar ] [ CrossRef ]
- Farid, G.; Hongwei, M.; Ali, S.M.; Liwei, Q. A review on linear and nonlinear control techniques for position and attitude control of a quadrotor. Control Intell. Syst. 2017 , 45 , 43–57. [ Google Scholar ]
- Derrouaoui, S.H.; Bouzid, Y.; Guiatni, M. Nonlinear robust control of a new reconfigurable unmanned aerial vehicle. Robotics 2021 , 10 , 76. [ Google Scholar ] [ CrossRef ]
- Priya, P.; Kamlu, S.S. Robust Control Algorithm for Drones. In Aeronautics-New Advances ; IntechOpen: London, UK, 2022. [ Google Scholar ]
- Cao, S.; Yu, H. An Adaptive Control Framework for the Autonomous Aerobatic Maneuvers of Fixed-Wing Unmanned Aerial Vehicle. Drones 2022 , 6 , 316. [ Google Scholar ]
- Nguen, V.; Putov, A.; Nguen, T. Adaptive control of an unmanned aerial vehicle. In AIP Conference Proceedings ; AIP Publishing LLC.: Melville, NY, USA, 2017; Volume 1798, p. 020124. [ Google Scholar ]
- Dief, T.N.; Yoshida, S. Modeling and classical controller of quad-rotor. arXiv 2017 , arXiv:1707.04173. [ Google Scholar ]
- Dolega, B.; Kopecki, G.; Kordos, D.; Rogalski, T. Review of Chosen Control Algorithms Used for Small UAV Control. In Solid State Phenomena ; Trans Tech Publications: Stafa-Zurich, Switzerland, 2017; Volume 260, pp. 175–183. [ Google Scholar ]
- Joukhadar, A.; Alchehabi, M.; Jejeh, A. Advanced UAVs nonlinear control systems and applications. In Unmanned Robotic Systems and Applications ; IntechOpen: London, UK, 2019; p. 79. [ Google Scholar ]
- Jafari, M.; Xu, H. Intelligent control for unmanned aerial systems with system uncertainties and disturbances using artificial neural network. Drones 2018 , 2 , 30. [ Google Scholar ] [ CrossRef ] [ Green Version ]
- Vachtsevanos, G.; Tang, L.; Drozeski, G.; Gutierrez, L. Intelligent control of unmanned aerial vehicles for improved autonomy and reliability. IFAC Proc. Vol. 2004 , 37 , 852–861. [ Google Scholar ] [ CrossRef ]
- Azar, A.T.; Koubaa, A.; Ali Mohamed, N.; Ibrahim, H.A.; Ibrahim, Z.F.; Kazim, M.; Ammar, A.; Benjdira, B.; Khamis, A.M.; Hameed, I.A.; et al. Drone deep reinforcement learning: A review. Electronics 2021 , 10 , 999. [ Google Scholar ]
- Goel, A.; Paredes, J.A.; Dadhaniya, H.; Islam, S.A.U.; Salim, A.M.; Ravela, S.; Bernstein, D. Experimental implementation of an adaptive digital autopilot. In Proceedings of the 2021 American Control Conference (ACC), New Orleans, LA, USA, 25–28 May 2021; pp. 3737–3742. [ Google Scholar ]
- Park, D.; Yu, H.; Xuan-Mung, N.; Lee, J.; Hong, S.K. Multicopter PID attitude controller gain auto-tuning through reinforcement learning neural networks. In Proceedings of the 2019 2nd International Conference on Control and Robot Technology, Jeju, Republic of Korea, 12–14 December 2019; pp. 80–84. [ Google Scholar ]
- Trilaksono, B.R.; Nasution, S.H.; Purwanto, E.B. Design and implementation of hardware-in-the-loop-simulation for uav using pid control method. In Proceedings of the 2013 3rd International Conference on Instrumentation, Communications, Information Technology and Biomedical Engineering (ICICI-BME), Bandung, Indonesia, 7–8 November 2013; pp. 124–130. [ Google Scholar ]
- Bangura, M.; Mahony, R. Real-time model predictive control for quadrotors. IFAC Proc. Vol. 2014 , 47 , 11773–11780. [ Google Scholar ] [ CrossRef ]
- Amadi, C.A. Design and Implementation of a Model Predictive Control on a Pixhawk Flight Controller. Ph.D. Thesis, Stellenbosch University, Stellenbosch, South Africa, 2018. [ Google Scholar ]
- Niit, E.A.; Smit, W.J. Integration of model reference adaptive control (MRAC) with PX4 firmware for quadcopters. In Proceedings of the 2017 24th International Conference on Mechatronics and Machine Vision in Practice (M2VIP), Auckland, New Zealand, 21–23 November 2017; pp. 1–6. [ Google Scholar ]
- Yadav, A.K.; Gaur, P. AI-based adaptive control and design of autopilot system for nonlinear UAV. Sadhana 2014 , 39 , 765–783. [ Google Scholar ]
- Zheng, E.H.; Xiong, J.J.; Luo, J.L. Second order sliding mode control for a quadrotor UAV. ISA Trans. 2014 , 53 , 1350–1356. [ Google Scholar ] [ CrossRef ]
- Benallegue, A.; Mokhtari, A.; Fridman, L. High-order sliding-mode observer for a quadrotor UAV. Int. J. Robust Nonlinear Control IFAC-Affil. J. 2008 , 18 , 427–440. [ Google Scholar ] [ CrossRef ]
- Chandler, P.R.; Pachter, M.; Swaroop, D.; Fowler, J.M.; Howlett, J.K.; Rasmussen, S.; Schumacher, C.; Nygard, K. Complexity in UAV cooperative control. In Proceedings of the 2002 American Control Conference (IEEE Cat. No. CH37301), Anchorage, AK, USA, 8–10 May 2002; Volume 3, pp. 1831–1836. [ Google Scholar ]
- Edwards, C.; Spurgeon, S. Sliding Mode Control: Theory and Applications ; CRC Press: Boca Raton, FL, USA, 1998. [ Google Scholar ]
- Lee, H.; Utkin, V.I. Chattering suppression methods in sliding mode control systems. Annu. Rev. Control 2007 , 31 , 179–188. [ Google Scholar ] [ CrossRef ]
- Young, K.D.; Utkin, V.I.; Ozguner, U. A control engineer’s guide to sliding mode control. IEEE Trans. Control Syst. Technol. 1999 , 7 , 328–342. [ Google Scholar ] [ CrossRef ] [ Green Version ]
- Tokat, S.; Fadali, M.S.; Eray, O. A classification and overview of sliding mode controller sliding surface design methods. Recent Adv. Sliding Modes Control Intell. Mech. 2015 , 24 , 417–439. [ Google Scholar ]
- Bartoszewicz, A.; Leśniewski, P. New switching and nonswitching type reaching laws for SMC of discrete time systems. IEEE Trans. Control Syst. Technol. 2015 , 24 , 670–677. [ Google Scholar ] [ CrossRef ]
- Latosiński, P.; Herkt, M. Non-switching Reaching Law Based DSMC Strategies in the Context of Robustness Comparison. In Proceedings of the 27th International Conference on Systems Engineering, ICSEng 2020 ; Springer: Berlin/Heidelberg, Germany, 2021; pp. 81–92. [ Google Scholar ]
- Khan, A.I.; Al-Mulla, Y. Unmanned aerial vehicle in the machine learning environment. Procedia Comput. Sci. 2019 , 160 , 46–53. [ Google Scholar ] [ CrossRef ]
- Ben Aissa, S.; Ben Letaifa, A. UAV Communications with machine learning: Challenges, applications and open issues. Arab. J. Sci. Eng. 2022 , 47 , 1559–1579. [ Google Scholar ]
- Gu, W.; Valavanis, K.P.; Rutherford, M.J.; Rizzo, A. UAV model-based flight control with artificial neural networks: A survey. J. Intell. Robot. Syst. 2020 , 100 , 1469–1491. [ Google Scholar ]
- Cordoba, G.M.A. Autonomous intelligent fuzzy logic guidance, and flight control system for the EFIGENIA EJ-1B MOZART unmanned aerial vehicle UAV. IFAC Proc. Vol. 2007 , 40 , 31–36. [ Google Scholar ] [ CrossRef ]
- Hajiyev, C.; Ersin Soken, H.; Yenal Vural, S.; Hajiyev, C.; Soken, H.E.; Vural, S.Y. Fuzzy Logic-Based Controller Design. In State Estimation and Control for Low-Cost Unmanned Aerial Vehicles ; Springer: Cham, Switzerland, 2015; pp. 201–221. [ Google Scholar ]
- Kurnaz, S.; Cetin, O.; Kaynak, O. Adaptive neuro-fuzzy inference system based autonomous flight control of unmanned air vehicles. Expert Syst. Appl. 2010 , 37 , 1229–1234. [ Google Scholar ] [ CrossRef ]
- Sargolzaei, A.; Abbaspour, A.; Crane, C.D. Control of cooperative unmanned aerial vehicles: Review of applications, challenges, and algorithms. Optim. Learn. Control Interdependent Complex Netw. 2020 , 1123 , 229–255. [ Google Scholar ]
- Ziquan, Y.; Zhang, Y.; Jiang, B.; Jun, F.; Ying, J. A review on fault-tolerant cooperative control of multiple unmanned aerial vehicles. Chin. J. Aeronaut. 2022 , 35 , 1–18. [ Google Scholar ]
- Ryan, A.; Zennaro, M.; Howell, A.; Sengupta, R.; Hedrick, J.K. An overview of emerging results in cooperative UAV control. In Proceedings of the 2004 43rd IEEE Conference on Decision and Control (CDC) (IEEE Cat. No. 04CH37601), Nassau, Bahamas, 14–17 December 2004; Volume 1, pp. 602–607. [ Google Scholar ]
- Kada, B.; Khalid, M.; Shaikh, M.S. Distributed cooperative control of autonomous multi-agent UAV systems using smooth control. J. Syst. Eng. Electron. 2020 , 31 , 1297–1307. [ Google Scholar ] [ CrossRef ]
- Sadeghzadeh, I.; Zhang, Y. A review on fault-tolerant control for unmanned aerial vehicles (UAVs). Infotech@ Aerosp. 2011 , 2011 , 1472. [ Google Scholar ]
- Bondyra, A.; Kołodziejczak, M.; Kulikowski, R.; Giernacki, W. An Acoustic Fault Detection and Isolation System for Multirotor UAV. Energies 2022 , 15 , 3955. [ Google Scholar ] [ CrossRef ]
- Puchalski, R.; Giernacki, W. UAV Fault Detection Methods, State-of-the-Art. Drones 2022 , 6 , 330. [ Google Scholar ] [ CrossRef ]
- Ducard, G. Actuator Fault Detection in UAVs, in Handbook of Unmanned Aircraft. In Handbook of Unmanned Aerial Vehicles ; Springer: Dordrecht, The Netherlands, 2014. [ Google Scholar ]
- Fourlas, G.K.; Karras, G.C. A survey on fault diagnosis and fault-tolerant control methods for unmanned aerial vehicles. Machines 2021 , 9 , 197. [ Google Scholar ] [ CrossRef ]
- Gao, B.; Liu, Y.J.; Liu, L. Adaptive neural fault-tolerant control of a quadrotor UAV via fast terminal sliding mode. Aerosp. Sci. Technol. 2022 , 129 , 107818. [ Google Scholar ] [ CrossRef ]
- Bu, X. Prescribed performance control approaches, applications and challenges: A comprehensive survey. Asian J. Control 2023 , 25 , 241–261. [ Google Scholar ] [ CrossRef ]
- Song, H.; Zhang, T.; Zhang, G.; Lu, C. Integrated interceptor guidance and control with prescribed performance. Int. J. Robust Nonlinear Control 2015 , 25 , 3179–3194. [ Google Scholar ] [ CrossRef ]
- Huang, H.; Luo, C.; Han, B. Prescribed performance fuzzy back-stepping control of a flexible air-breathing hypersonic vehicle subject to input constraints. J. Intell. Manuf. 2022 , 33 , 853–866. [ Google Scholar ] [ CrossRef ]
- Chen, B.W.; Tan, L.G. Adaptive anti-saturation tracking control with prescribed performance for hypersonic vehicle. Int. J. Control Autom. Syst. 2020 , 18 , 394–404. [ Google Scholar ] [ CrossRef ]
- Bu, X.; Xiao, Y. Prescribed performance-based low-computational cost fuzzy control of a hypersonic vehicle using non-affine models. Adv. Mech. Eng. 2018 , 10 , 1687814018757261. [ Google Scholar ] [ CrossRef ] [ Green Version ]
- Bu, X.; Xiao, Y.; Wang, K. A prescribed performance control approach guaranteeing small overshoot for air-breathing hypersonic vehicles via neural approximation. Aerosp. Sci. Technol. 2017 , 71 , 485–498. [ Google Scholar ] [ CrossRef ]
- Li, S.; Ma, T.; Luo, X.; Yang, Z. Adaptive fuzzy output regulation for unmanned surface vehicles with prescribed performance. Int. J. Control Autom. Syst. 2020 , 18 , 405–414. [ Google Scholar ] [ CrossRef ]
- Yu, Z.; Zhang, Y.; Liu, Z.; Qu, Y.; Su, C.Y.; Jiang, B. Decentralized finite-time adaptive fault-tolerant synchronization tracking control for multiple UAVs with prescribed performance. J. Frankl. Inst. 2020 , 357 , 11830–11862. [ Google Scholar ] [ CrossRef ]
- Koksal, N.; An, H.; Fidan, B. Backstepping-based adaptive control of a quadrotor UAV with guaranteed tracking performance. ISA Trans. 2020 , 105 , 98–110. [ Google Scholar ] [ CrossRef ]
- Han, B.; Zhou, Y.; Deveerasetty, K.K.; Hu, C. A review of control algorithms for quadrotor. In Proceedings of the 2018 IEEE International Conference on Information and Automation (ICIA), Wuyi Mountain, China, 11–13 August 2018; pp. 951–956. [ Google Scholar ]
- Roy, R.; Islam, M.; Sadman, N.; Mahmud, M.P.; Gupta, K.D.; Ahsan, M.M. A review on comparative remarks, performance evaluation and improvement strategies of quadrotor controllers. Technologies 2021 , 9 , 37. [ Google Scholar ] [ CrossRef ]
- Hasseni, S.E.I.; Abdou, L.; Glida, H.E. Parameters tuning of a quadrotor PID controllers by using nature-inspired algorithms. Evol. Intell. 2021 , 14 , 61–73. [ Google Scholar ] [ CrossRef ]
- Hasseni, S.E.I.; Abdou, L. Decentralized PID control by using GA optimization applied to a quadrotor. J. Autom. Mob. Robot. Intell. Syst. 2018 , 12 , 33–44. [ Google Scholar ] [ CrossRef ]
- Castillo-Zamora, J.J.; Camarillo-Gomez, K.A.; Perez-Soto, G.I.; Rodriguez-Resendiz, J. Comparison of PD, PID and sliding-mode position controllers for V–tail quadcopter stability. IEEE Access 2018 , 6 , 38086–38096. [ Google Scholar ] [ CrossRef ]
- Guo, X.; Hou, S.; Niu, P.; Zhao, D. A Review of Control Methods for Quadrotor UAVs. In Proceedings of the 2022 5th International Conference on Electronics and Electrical Engineering Technology (EEET), Beijing, China, 2–4 December 2022; pp. 132–138. [ Google Scholar ] [ CrossRef ]
- Okasha, M.; Kralev, J.; Islam, M. Design and Experimental Comparison of PID, LQR and MPC Stabilizing Controllers for Parrot Mambo Mini-Drone. Aerospace 2022 , 9 , 298. [ Google Scholar ] [ CrossRef ]
- Massé, C.; Gougeon, O.; Nguyen, D.T.; Saussié, D. Modeling and Control of a Quadcopter Flying in a Wind Field: A Comparison Between LQR and Structured ℋ ∞ Control Techniques. In Proceedings of the 2018 International Conference on Unmanned Aircraft Systems (ICUAS), Dallas, TX, USA, 12–15 June 2018; pp. 1408–1417. [ Google Scholar ] [ CrossRef ]
- Rugh, W.J.; Shamma, J.S. Research on gain scheduling. Automatica 2000 , 36 , 1401–1425. [ Google Scholar ] [ CrossRef ]
- Bouzid, Y.; Derrouaoui, S.H.; Guiatni, M. PID gain scheduling for 3D trajectory tracking of a quadrotor with rotating and extendable arms. In Proceedings of the 2021 International Conference on Recent Advances in Mathematics and Informatics (ICRAMI), Tebessa, Algeria, 21–22 September 2021; pp. 1–4. [ Google Scholar ]
- Glida, H.E.; Abdou, L.; Chelihi, A.; Sentouh, C.; Hasseni, S.E.I. Optimal model-free backstepping control for a quadrotor helicopter. Nonlinear Dyn. 2020 , 100 , 3449–3468. [ Google Scholar ] [ CrossRef ]
- Abdou, L. Integral backstepping/LFT-LPV ℋ ∞ control for the trajectory tracking of a quadcopter. In Proceedings of the 2018 7th International Conference on Systems and Control (ICSC), Valencia, Spain, 24–26 October 2018; pp. 348–353. [ Google Scholar ]
- Seif-El-Islam, H.; Abdou, L. Robust LPV control for attitude stabilization of a quadrotor helicopter under input saturations. Adv. Technol. Innov. 2020 , 5 , 98. [ Google Scholar ]
- Baldi, S.; Sun, D.; Xia, X.; Zhou, G.; Liu, D. ArduPilot-based adaptive autopilot: Architecture and software-in-the-loop experiments. IEEE Trans. Aerosp. Electron. Syst. 2022 , 58 , 4473–4485. [ Google Scholar ] [ CrossRef ]
- Nguyen, A.T.; Taniguchi, T.; Eciolaza, L.; Campos, V.; Palhares, R.; Sugeno, M. Fuzzy control systems: Past, present and future. IEEE Comput. Intell. Mag. 2019 , 14 , 56–68. [ Google Scholar ] [ CrossRef ]
- Ferdaus, M.M.; Anavatti, S.G.; Pratama, M.; Garratt, M.A. Towards the use of fuzzy logic systems in rotary wing unmanned aerial vehicle: A review. Artif. Intell. Rev. 2020 , 53 , 257–290. [ Google Scholar ] [ CrossRef ]
- Wai, R.J.; Prasetia, A.S. Adaptive neural network control and optimal path planning of UAV surveillance system with energy consumption prediction. IEEE Access 2019 , 7 , 126137–126153. [ Google Scholar ] [ CrossRef ]
- Fahlstrom, P.G.; Gleason, T.J.; Sadraey, M.H. Introduction to UAV Systems 5th Edition ; John Wiley & Sons: Hoboken, NJ, USA, 2022. [ Google Scholar ]
- Bigazzi, L.; Basso, M.; Boni, E.; Innocenti, G.; Pieraccini, M. A Multilevel Architecture for Autonomous UAVs. Drones 2021 , 5 , 55. [ Google Scholar ] [ CrossRef ]
- Ahmed, F.; Jenihhin, M. A Survey on UAV Computing Platforms: A Hardware Reliability Perspective. Sensors 2022 , 22 , 6286. [ Google Scholar ] [ CrossRef ] [ PubMed ]
- Changpradith, W. Application of Object Detection Using Hardware Acceleration for Autonomous UAV ; California State Polytechnic University: Pomona, CA, USA, 2022. [ Google Scholar ]
- Hristov, G.V.; Zahariev, P.Z.; Beloev, I.H. A review of the characteristics of modern unmanned aerial vehicles. Acta Technol. Agric. 2016 , 19 , 33–38. [ Google Scholar ] [ CrossRef ] [ Green Version ]
- Colorado Montaño, J. Towards MAV Autonomous Flight: A Modeling and Control Approach. Master’s Thesis, Industriales, University of Madrid, Madrid, Spain, 2010. [ Google Scholar ]
- Sharma, B.L.; Khatri, N.; Sharma, A. An analytical review on FPGA based autonomous flight control system for small UAVs. In Proceedings of the 2016 International Conference on Electrical, Electronics, and Optimization Techniques (ICEEOT), Chennai, India, 3–5 March 2016; pp. 1369–1372. [ Google Scholar ]
- Chao, H.; Cao, Y.; Chen, Y. Autopilots for small unmanned aerial vehicles: A survey. Int. J. Control Autom. Syst. 2010 , 8 , 36–44. [ Google Scholar ] [ CrossRef ]
- Monterrosa, N.; Montoya, J.; Jarquín, F.; Bran, C. Design, development and implementation of a UAV flight controller based on a state machine approach using a FPGA embedded system. In Proceedings of the 2016 IEEE/AIAA 35th Digital Avionics Systems Conference (DASC), Sacramento, CA, USA, 25–29 September 2016; pp. 1–8. [ Google Scholar ]
- Sohail, S.S.; Himeur, Y.; Amira, A.; Fadli, F.; Mansoor, W.; Atalla, S.; Copiaco, A. Deep Transfer Learning for 3d Point Cloud Understanding: A Comprehensive Survey. Available online: https://papers.ssrn.com/sol3/papers.cfm?abstract_id=4348272 (accessed on 22 July 2023).
- Pádua, L.; Vanko, J.; Hruška, J.; Adão, T.; Sousa, J.J.; Peres, E.; Morais, R. UAS, sensors, and data processing in agroforestry: A review towards practical applications. Int. J. Remote Sens. 2017 , 38 , 2349–2391. [ Google Scholar ] [ CrossRef ]
- Amarasingam, N.; Salgadoe, S.; Powell, K.; Gonzalez, L.F.; Natarajan, S. A review of UAV platforms, sensors, and applications for monitoring of sugarcane crops. Remote Sens. Appl. Soc. Environ. 2022 , 26 , 100712. [ Google Scholar ] [ CrossRef ]
- Olson, D.; Anderson, J. Review on unmanned aerial vehicles, remote sensors, imagery processing, and their applications in agriculture. Agron. J. 2021 , 113 , 971–992. [ Google Scholar ] [ CrossRef ]
- Kim, S.G.; Lee, E.; Hong, I.P.; Yook, J.G. Review of intentional electromagnetic interference on UAV sensor modules and experimental study. Sensors 2022 , 22 , 2384. [ Google Scholar ] [ CrossRef ] [ PubMed ]
- Samaras, S.; Diamantidou, E.; Ataloglou, D.; Sakellariou, N.; Vafeiadis, A.; Magoulianitis, V.; Lalas, A.; Dimou, A.; Zarpalas, D.; Votis, K.; et al. Deep learning on multi sensor data for counter UAV applications—A systematic review. Sensors 2019 , 19 , 4837. [ Google Scholar ] [ CrossRef ] [ Green Version ]
- Ecke, S.; Dempewolf, J.; Frey, J.; Schwaller, A.; Endres, E.; Klemmt, H.J.; Tiede, D.; Seifert, T. UAV-based forest health monitoring: A systematic review. Remote Sens. 2022 , 14 , 3205. [ Google Scholar ] [ CrossRef ]
- Joshi, D.; Deb, D.; Muyeen, S. Comprehensive Review on Electric Propulsion System of Unmanned Aerial Vehicles. Front. Energy Res. 2022 , 10 , 739. [ Google Scholar ] [ CrossRef ]
- Teubl, D.; Bitenc, T.; Hornung, M. Design and Development of an Actuator Control and Monitoring Unit for Small and Medium Size Research UAVs ; Deutsche Gesellschaft für Luft-und Raumfahrt-Lilienthal-Oberth eV: Bonn, Germany, 2021. [ Google Scholar ]
- Grundmann, S.; Frey, M.; Tropea, C. Unmanned aerial vehicle (UAV) with plasma actuators for separation control. In Proceedings of the 47th AIAA Aerospace Sciences Meeting including The New Horizons Forum and Aerospace Exposition, Orlando, FL, USA, 5–8 January 2009; p. 698. [ Google Scholar ]
- Boukoberine, M.N.; Zhou, Z.; Benbouzid, M. Power supply architectures for drones—A review. In Proceedings of the IECON 2019-45th Annual Conference of the IEEE Industrial Electronics Society, Lisbon, Portugal, 14–17 October 2019; Volume 1, pp. 5826–5831. [ Google Scholar ]
- Mohsan, S.A.H.; Othman, N.Q.H.; Khan, M.A.; Amjad, H.; Żywiołek, J. A comprehensive review of micro UAV charging techniques. Micromachines 2022 , 13 , 977. [ Google Scholar ] [ CrossRef ]
- Chittoor, P.K.; Chokkalingam, B.; Mihet-Popa, L. A review on UAV wireless charging: Fundamentals, applications, charging techniques and standards. IEEE Access 2021 , 9 , 69235–69266. [ Google Scholar ] [ CrossRef ]
- Xu, L.; Huangfu, Y.; Ma, R.; Xie, R.; Song, Z.; Zhao, D.; Yang, Y.; Wang, Y.; Xu, L. A Comprehensive Review on Fuel Cell UAV Key Technologies: Propulsion System, Management Strategy and Design Procedure. IEEE Trans. Transp. Electrif. 2022 , 8 , 4118–4139. [ Google Scholar ] [ CrossRef ]
- Zhang, C.; Qiu, Y.; Chen, J.; Li, Y.; Liu, Z.; Liu, Y.; Zhang, J.; Hwa, C.S. A comprehensive review of electrochemical hybrid power supply systems and intelligent energy managements for unmanned aerial vehicles in public services. Energy AI 2022 , 9 , 100175. [ Google Scholar ] [ CrossRef ]
- Townsend, A.; Jiya, I.N.; Martinson, C.; Bessarabov, D.; Gouws, R. A comprehensive review of energy sources for unmanned aerial vehicles, their shortfalls and opportunities for improvements. Heliyon 2020 , 6 , e05285. [ Google Scholar ] [ CrossRef ]
- Alsuhli, G.; Fahim, A.; Gadallah, Y. A survey on the role of UAVs in the communication process: A technological perspective. Comput. Commun. 2022 , 194 , 86–123. [ Google Scholar ] [ CrossRef ]
- Sharma, A.; Vanjani, P.; Paliwal, N.; Basnayaka, C.M.W.; Jayakody, D.N.K.; Wang, H.C.; Muthuchidambaranathan, P. Communication and networking technologies for UAVs: A survey. J. Netw. Comput. Appl. 2020 , 168 , 102739. [ Google Scholar ] [ CrossRef ]
- Chen, X.; Tang, J.; Lao, S. Review of unmanned aerial vehicle swarm communication architectures and routing protocols. Appl. Sci. 2020 , 10 , 3661. [ Google Scholar ] [ CrossRef ]
- Ghazali, M.H.M.; Teoh, K.; Rahiman, W. A systematic review of real-time deployments of UAV-based LoRa communication network. IEEE Access 2021 , 9 , 124817–124830. [ Google Scholar ] [ CrossRef ]
- Islam, N.; Rashid, M.M.; Pasandideh, F.; Ray, B.; Moore, S.; Kadel, R. A review of applications and communication technologies for internet of things (Iot) and unmanned aerial vehicle (uav) based sustainable smart farming. Sustainability 2021 , 13 , 1821. [ Google Scholar ] [ CrossRef ]
- Scott, J.; Scott, C. Drone Delivery Models for Healthcare. In Proceedings of the 50th Hawaii International Conference on System Sciences, Hilton Waikoloa Village, Hawaii; 2017; pp. 3297–3304, ISBN 978-0-9981331-0-2. Available online: https://scholarspace.manoa.hawaii.edu/items/947f05d9-71f2-467a-8291-084642e402ad (accessed on 22 July 2023).
- Brischetto, S.; Torre, R. Preliminary Finite Element Analysis and Flight Simulations of a Modular Drone Built through Fused Filament Fabrication. J. Compos. Sci. 2021 , 5 , 293. [ Google Scholar ] [ CrossRef ]
- Martinetti, A.; Margaryan, M.; van Dongen, L. Simulating mechanical stress on a micro Unmanned Aerial Vehicle (UAV) body frame for selecting maintenance actions. Procedia Manuf. 2018 , 16 , 61–66. [ Google Scholar ] [ CrossRef ]
- Mishra, A.; Pal, S.; Malhi, G.; Singh, P. Structural analysis of UAV airframe by using FEM techniques: A review. Int. J. Mech. Prod. ISSN 2020 , 10 , 2249–6890. [ Google Scholar ]
- Ferreira, J.F.M.A. Structural Analysis and Optimization of a UAV Wing. Master’s Thesis, Técnico Lisboa, Lisboa, Portugal, 2018. [ Google Scholar ]
- Landolfo, G.; Altman, A. Aerodynamic and structural design of a small nonplanar wing UAV. In Proceedings of the 47th AIAA Aerospace Sciences Meeting Including the New Horizons Forum and Aerospace Exposition, Orlando, FL, USA, 5–8 January 2009; p. 1068. [ Google Scholar ]
- Kim, M.; Joo, H.; Jang, B. Conceptual multicopter sizing and performance analysis via component database. In Proceedings of the 2017 Ninth International Conference on Ubiquitous and Future Networks (ICUFN), Milan, Italy, 4–7 July 2017; pp. 105–109. [ Google Scholar ]
- Souza, L.S.; Rocha, F.G.; Soares, M.S. A Review on Software/Systems Architecture Description for Autonomous Systems. Recent Adv. Comput. Sci. Commun. 2023 , 16 , 52–60. [ Google Scholar ]
- Spica, R.; Giordano, P.R.; Ryll, M.; Bülthoff, H.H.; Franchi, A. An open-source hardware/software architecture for quadrotor UAVs. IFAC Proc. Vol. 2013 , 46 , 198–205. [ Google Scholar ] [ CrossRef ]
- Madridano, Á.; Al-Kaff, A.; Flores, P.; Martín, D.; de la Escalera, A. Software architecture for autonomous and coordinated navigation of uav swarms in forest and urban firefighting. Appl. Sci. 2021 , 11 , 1258. [ Google Scholar ] [ CrossRef ]
- Kekec, T.; Ustundag, B.C.; Guney, M.A.; Yildirim, A.; Unel, M. A modular software architecture for UAVs. In Proceedings of the IECON 2013-39th Annual Conference of the IEEE Industrial Electronics Society, Vienna, Austria, 10–13 November 2013; pp. 4037–4042. [ Google Scholar ]
- Pastor, E.; Lopez, J.; Royo, P. A hardware/Software architecture for UAV payload and mission control. In Proceedings of the 2006 Ieee/Aiaa 25TH Digital Avionics Systems Conference, Portland, OR, USA, 15–19 October 2006; pp. 1–8. [ Google Scholar ]
- York, D.D.; Al-Bayati, A.J.; Al-Shabbani, Z.Y. Potential Applications of UAV within the Construction Industry and the Challenges Limiting Implementation. In Construction Research Congress 2020: Project Management and Controls, Materials, and Contracts ; American Society of Civil Engineers: Reston, VA, USA, 2020; pp. 31–39. [ Google Scholar ]
- Opfer, N.D.; Shields, D.R. Unmanned aerial vehicle applications and issues for construction. In Proceedings of the 2014 ASEE Annual Conference & Exposition; 2014; pp. 24–1302. Available online: https://peer.asee.org/unmanned-aerial-vehicle-applications-and-issues-for-construction (accessed on 22 July 2023).
- Javaid, M.; Haleem, A.; Khan, I.H.; Singh, R.P.; Suman, R.; Mohan, S. Significant features and applications of Drones for Healthcare: An overview. J. Ind. Integr. Manag. 2022 , 2250024. [ Google Scholar ] [ CrossRef ]
- Ahirwar, S.; Swarnkar, R.; Bhukya, S.; Namwade, G. Application of drone in agriculture. Int. J. Curr. Microbiol. Appl. Sci. 2019 , 8 , 2500–2505. [ Google Scholar ] [ CrossRef ]
- Li, Y.; Liu, C. Applications of multirotor drone technologies in construction management. Int. J. Constr. Manag. 2019 , 19 , 401–412. [ Google Scholar ] [ CrossRef ]
- Manfreda, S.; McCabe, M.F.; Miller, P.E.; Lucas, R.; Pajuelo Madrigal, V.; Mallinis, G.; Ben Dor, E.; Helman, D.; Estes, L.; Ciraolo, G.; et al. On the use of unmanned aerial systems for environmental monitoring. Remote Sens. 2018 , 10 , 641. [ Google Scholar ] [ CrossRef ] [ Green Version ]
- Majeed, R.; Abdullah, N.A.; Mushtaq, M.F.; Kazmi, R. Drone security: Issues and challenges. Parameters 2021 , 2 , 5. [ Google Scholar ] [ CrossRef ]
- Mirri, S.; Prandi, C.; Salomoni, P. Human-Drone Interaction: State of the art, open issues and challenges. In Proceedings of the ACM SIGCOMM 2019 Workshop on Mobile AirGround Edge Computing, Systems, Networks, and Applications, Beijing, China, 19 August 2019; pp. 43–48. [ Google Scholar ]
- PX4, PIXHAWK Engineering Team. Available online: https://github.com/PX4/jMAVSim (accessed on 22 July 2023).
- He, L.; Aouf, N.; Song, B. Explainable Deep Reinforcement Learning for UAV autonomous path planning. Aerosp. Sci. Technol. 2021 , 118 , 107052. [ Google Scholar ] [ CrossRef ]
- Available online: https://ardupilot.org/ardupilot (accessed on 22 July 2023).
- TensorFlow. Available online: https://www.tensorflow.org (accessed on 22 July 2023).
- Topalli, M.T.; Yilmaz, M.; Çorapsiz, M.F. Real Time Implementation of Drone Detection using TensorFlow and MobileNetV2-SSD. In Proceedings of the 2021 7th International Conference on Electrical, Electronics and Information Engineering (ICEEIE), Malang, Indonesia, 2 October 2021; pp. 436–439. [ Google Scholar ]
- Jiang, Z.; Liu, Y.; Wu, B.; Zhu, Q. Monocular Vision Based UAV Target Detection and Ranging System Implemented on OpenCV and Tensor Flow. In Proceedings of the 2019 18th International Symposium on Distributed Computing and Applications for Business Engineering and Science (DCABES), Wuhan, China, 8–10 November 2019; pp. 88–91. [ Google Scholar ]
- Available online: https://wiki.paparazziuav.org (accessed on 1 August 2023).
- Remes, B.; Hensen, D.; Van Tienen, F.; De Wagter, C.; Van der Horst, E.; De Croon, G. Paparazzi: How to make a swarm of parrot ar drones fly autonomously based on gps. In Proceedings of the International Micro Air Vehicle Conference and Flight Competition (IMAV2013), Toulouse, France, 17–20 September 2013; pp. 17–20. [ Google Scholar ]
- Garcia, J.; Brock, A.; Saporito, N.; Hattenberger, G.; Paris, X.; Gorraz, M.; Jestin, Y. Designing human-drone interactions with the Paparazzi UAV System. In Proceedings of the 1st International Workshop on Human-Drone Interaction-CHI’19, Glasgow, UK, 14 May 2019. [ Google Scholar ]
- Available online: http://www.qgroundcontrol.org (accessed on 22 July 2023).
- Mission Planner. Available online: http://ardupilot.org/planner (accessed on 22 July 2023).
- APM Planner 2. Available online: https://ardupilot.org/planner2/ (accessed on 22 July 2023).
- UgCS. Available online: https://www.ugcs.com (accessed on 22 July 2023).
- Ramirez-Atencia, C.; Camacho, D. Extending QGroundControl for automated mission planning of UAVs. Sensors 2018 , 18 , 2339. [ Google Scholar ] [ CrossRef ] [ Green Version ]
- Dardoize, T.; Ciochetto, N.; Hong, J.H.; Shin, H.S. Implementation of ground control system for autonomous multi-agents using qgroundcontrol. In Proceedings of the 2019 Workshop on Research, Education and Development of Unmanned Aerial Systems (RED UAS), Cranfield, UK, 25–27 November 2019; pp. 24–30. [ Google Scholar ]
- Vachtsevanos, G.; Tang, L.; Drozeski, G.; Gutierrez, L. From mission planning to flight control of unmanned aerial vehicles: Strategies and implementation tools. Annu. Rev. Control 2005 , 29 , 101–115. [ Google Scholar ] [ CrossRef ]
- AirSim. Available online: https://microsoft.github.io/AirSim (accessed on 22 July 2023).
- Madaan, R.; Gyde, N.; Vemprala, S.; Brown, M.; Nagami, K.; Taubner, T.; Cristofalo, E.; Scaramuzza, D.; Schwager, M.; Kapoor, A. Airsim drone racing lab. In Proceedings of the Neurips 2019 Competition and Demonstration Track, PMLR; 2020; pp. 177–191. Available online: http://proceedings.mlr.press/v123/madaan20a.html (accessed on 22 July 2023).
- Villota Miranda, D.; Gil Martínez, M.; Rico-Azagra, J. A3C for drone autonomous driving using Airsim. In XLII Jornadas de Automática ; Universidade da Coruña, Servizo de Publicacións: A Coruña, Spain, 2021; pp. 203–209. [ Google Scholar ]
- Ma, C.; Zhou, Y.; Li, Z. A new simulation environment based on AirSim, ROS, and PX4 for quadcopter aircrafts. In Proceedings of the 2020 6th International Conference on Control, Automation and Robotics (ICCAR), Singapore, 20–23 April 2020; pp. 486–490. [ Google Scholar ]
- JdeRobot. Available online: https://jderobot.github.io/projects/drones/drones (accessed on 1 August 2023).
- Cañas, J.M.; Perdices, E.; García-Pérez, L.; Fernández-Conde, J. A ROS-based open tool for intelligent robotics education. Appl. Sci. 2020 , 10 , 7419. [ Google Scholar ] [ CrossRef ]
- Arias-Perez, P.; Fernández-Conde, J.; Martin Gomez, D.; Cañas, J.M.; Campoy, P. A Middleware Infrastructure for Programming Vision-Based Applications in UAVs. Drones 2022 , 6 , 369. [ Google Scholar ] [ CrossRef ]
- DroneKit. Available online: https://dronekit.io (accessed on 22 July 2023).
- DroneKit-Python. Available online: dronekit-python.readthedocs.io (accessed on 22 July 2023).
- Gaber, A.M.; Rashid, R.A.; Nasir, N.; Rahim, R.A.; Sarijari, M.A.; Abdullah, A.S.; Aziz, O.A.; Hamid, S.Z.A.; Ali, S. Development of an Autonomous IoT-Based Drone for Campus Security. ELEKTRIKA-J. Electr. Eng. 2021 , 20 , 70–76. [ Google Scholar ]
- Lee, M.T.; Chuang, M.L.; Kuo, S.T.; Chen, Y.R. UAV swarm real-time rerouting by edge computing D* lite algorithm. Appl. Sci. 2022 , 12 , 1056. [ Google Scholar ] [ CrossRef ]
- Ingle, P.Y.; Kim, Y.; Kim, Y.G. Dvs: A drone video synopsis towards storing and analyzing drone surveillance data in smart cities. Systems 2022 , 10 , 170. [ Google Scholar ] [ CrossRef ]
- MAVLink. Available online: https://mavlink.io/ (accessed on 22 July 2023).
- Pratik, P.; Agarwal, P. Review on MAV Link for Unmanned Air Vehicle to Ground Control Station Communication. Think India J. 2019 , 22 , 7093–7103. [ Google Scholar ]
- Badole, S.; Choudhary, S.; Titarmare, A.; Khergade, P. Review On Ground Control Station Design for Remotely Piloted Aircraft System. In Proceedings of the 2022 10th International Conference on Emerging Trends in Engineering and Technology-Signal and Information Processing (ICETET-SIP-22), Nagpur, India, 29–30 April 2022; pp. 1–6. [ Google Scholar ]
- Mogili, U.R.; Deepak, B. An intelligent drone for agriculture applications with the aid of the MAVlink protocol. In Innovative Product Design and Intelligent Manufacturing Systems: Select Proceedings of ICIPDIMS 2019 ; Springer: Berlin/Heidelberg, Germany, 2020; pp. 195–205. [ Google Scholar ]
- Koubâa, A.; Allouch, A.; Alajlan, M.; Javed, Y.; Belghith, A.; Khalgui, M. Micro air vehicle link (mavlink) in a nutshell: A survey. IEEE Access 2019 , 7 , 87658–87680. [ Google Scholar ] [ CrossRef ]
- ROS Robot Operating System. Available online: https://www.ros.org (accessed on 22 July 2023).
- Abbyasov, B.; Lavrenov, R.; Zakiev, A.; Tsoy, T.; Magid, E.; Svinin, M.; Martinez-Garcia, E.A. Comparative analysis of ROS-based centralized methods for conducting collaborative monocular visual SLAM using a pair of UAVs. In Proceedings of the 23rd International Conference on Climbing and Walking Robots and Support Technologies for Mobile Machines, Moscow, Russia, 24–26 August 2020; pp. 113–120. [ Google Scholar ]
- Canpolat Tosun, D.; Işık, Y. A ROS-based hybrid algorithm for the UAV path planning problem. Aircr. Eng. Aerosp. Technol. 2023 , 95 , 784–798. [ Google Scholar ] [ CrossRef ]
- Megalingam, R.K.; Prithvi, D.V.; Kumar, N.C.S.; Egumadiri, V. Drone Stability Simulation Using ROS and Gazebo. In Proceedings of the Advanced Computing and Intelligent Technologies: Proceedings of ICACIT 2021 ; Springer: New Delhi, India, 2022; pp. 131–143. [ Google Scholar ]
- Mardiyanto, R.; Hisak, M.N.; Mujiono, T.; Suryoatmojo, H. Robot Operating System (ROS) Framework for Swarm Drone Flight Controller. In Proceedings of the 2022 International Seminar on Intelligent Technology and Its Applications (ISITIA), Surabaya, Indonesia, 20–21 July 2022; pp. 297–303. [ Google Scholar ]
- Jain, N.; Gupta, A.K.; Mathur, P. Autonomous drone using ROS for surveillance and 3D mapping using satellite map. In Proceedings of the Second International Conference on Information Management and Machine Intelligence: ICIMMI 2020 ; Springer: Jaipur, India, 2021; pp. 255–266. [ Google Scholar ]
- Yu, Y.; Wang, X.; Zhong, Z.; Zhang, Y. ROS-based UAV control using hand gesture recognition. In Proceedings of the 2017 29th Chinese Control Furthermore, Decision Conference (CCDC), Chongqing, China, 28–30 May 2017; pp. 6795–6799. [ Google Scholar ]
- Zhao, T.; Jiang, H. Landing system for AR. Drone 2.0 using onboard camera and ROS. In Proceedings of the 2016 IEEE Chinese Guidance, Navigation and Control Conference (CGNCC), Nanjing, China, 12–14 August 2016; pp. 1098–1102. [ Google Scholar ]
- Meng, W.; Hu, Y.; Lin, J.; Lin, F.; Teo, R. ROS+ unity: An efficient high-fidelity 3D multi-UAV navigation and control simulator in GPS-denied environments. In Proceedings of the IECON 2015-41st Annual Conference of the IEEE Industrial Electronics Society, Yokohama, Japan, 9–12 November 2015; pp. 002562–002567. [ Google Scholar ]
- Lamping, A.P.; Ouwerkerk, J.N.; Cohen, K. Multi-UAV control and supervision with ROS. In Proceedings of the 2018 Aviation Technology, Integration, and Operations Conference, Atlanta, GA, USA, 25–29 June 2018; p. 4245. [ Google Scholar ]
- Gazebosim, Gazebo. Available online: https://gazebosim.org/home (accessed on 22 July 2023).
- Webots, CyberBotics. Available online: https://cyberbotics.com (accessed on 22 July 2023).
- Morse, LAAS and ONERA Lab. Available online: https://github.com/morse-simulator/morse (accessed on 22 July 2023).
- Paparazzi. Available online: https://github.com/paparazzi (accessed on 22 July 2023).
- HackflightSim. Available online: https://github.com/simondlevy/HackflightSim (accessed on 22 July 2023).
- UAV Toolbox. Available online: https://www.mathworks.com/products/uav.html (accessed on 22 July 2023).
- Horri, N.; Pietraszko, M. A Tutorial and Review on Flight Control Co-Simulation Using Matlab/Simulink and Flight Simulators. Automation 2022 , 3 , 486–510. [ Google Scholar ] [ CrossRef ]
- Aliane, N.; Muñoz, C.Q.G.; Sánchez-Soriano, J. Web and MATLAB-Based Platform for UAV Flight Management and Multispectral Image Processing. Sensors 2022 , 22 , 4243. [ Google Scholar ] [ CrossRef ]
- Xing, Z.; He, Y.; Jian, C. Design and implementation of UAV flight simulation based on Matlab/Simulink. In Proceedings of the 2015 International Conference on Advances in Mechanical Engineering and Industrial Informatics; 2015; pp. 190–193. Available online: https://www.atlantis-press.com/proceedings/ameii-15/21706 (accessed on 22 July 2023).
- Pineda-Torres, F.; Arias-Barragán, L.A. PRM Navigation in Trading Drone and Gazebo Simulation. Vis. Electr. 2020 , 14 , 43–50. [ Google Scholar ] [ CrossRef ]
- Ivaldi, S.; Padois, V.; Nori, F. Tools for dynamics simulation of robots: A survey based on user feedback. arXiv 2014 , arXiv:1402.7050. [ Google Scholar ]
- Multiwii Series. Available online: https://github.com/multiwii (accessed on 22 July 2023).
- Cleanflight. Available online: http://cleanflight.com/ (accessed on 22 July 2023).
- Betaflight. Available online: https://github.com/betaflight/betaflight/wiki/670 (accessed on 22 July 2023).
- INAV, iNav Flight. Available online: https://github.com/iNavFlight/inav/wiki (accessed on 22 July 2023).
- OpenPilot, OpenPilot wiki. Available online: https://opwiki.readthedocs.io/en/latest (accessed on 22 July 2023).
- LibrePilot Project. Available online: http://librepilot.org (accessed on 22 July 2023).
- dRonin. Available online: http://dronin.org/ (accessed on 22 July 2023).
- OpenCV. Available online: https://opencv.org (accessed on 22 July 2023).
- Pytorch. Available online: https://pytorch.org (accessed on 22 July 2023).
- Azmat, M.; Kummer, S. Potential applications of unmanned ground and aerial vehicles to mitigate challenges of transport and logistics-related critical success factors in the humanitarian supply chain. Asian J. Sustain. Soc. Responsib. 2020 , 5 , 3. [ Google Scholar ] [ CrossRef ] [ Green Version ]
- Vattapparamban, E.; Güvenç, I.; Yurekli, A.I.; Akkaya, K.; Uluağaç, S. Drones for smart cities: Issues in cybersecurity, privacy, and public safety. In Proceedings of the 2016 International Wireless Communications and Mobile Computing Conference (IWCMC), Paphos, Cyprus, 5–9 September 2016; pp. 216–221. [ Google Scholar ]
- Shakhatreh, H.; Sawalmeh, A.H.; Al-Fuqaha, A.; Dou, Z.; Almaita, E.; Khalil, I.; Othman, N.S.; Khreishah, A.; Guizani, M. Unmanned aerial vehicles (UAVs): A survey on civil applications and key research challenges. IEEE Access 2019 , 7 , 48572–48634. [ Google Scholar ] [ CrossRef ]
- Kirschstein, T. Energy demand of parcel delivery services with a mixed fleet of electric vehicles. Clean. Eng. Technol. 2021 , 5 , 100322. [ Google Scholar ] [ CrossRef ]
- Gautam, T.; Johari, R. Drone: A Systematic Review of UAV Technologies. In Proceedings of the International Conference on Computing, Communications, and Cyber-Security ; Springer: Delhi, India, 2022; pp. 147–158. [ Google Scholar ]
- Elmeseiry, N.; Alshaer, N.; Ismail, T. A detailed survey and future directions of unmanned aerial vehicles (uavs) with potential applications. Aerospace 2021 , 8 , 363. [ Google Scholar ] [ CrossRef ]
- Heidari, A.; Navimipour, N.J.; Unal, M. A Secure Intrusion Detection Platform Using Blockchain and Radial Basis Function Neural Networks for Internet of Drones. IEEE Internet Things J. 2023 , 10 , 8445–8454. [ Google Scholar ] [ CrossRef ]
- Himeur, Y.; Sayed, A.; Alsalemi, A.; Bensaali, F.; Amira, A.; Varlamis, I.; Eirinaki, M.; Sardianos, C.; Dimitrakopoulos, G. Blockchain-based recommender systems: Applications, challenges and future opportunities. Comput. Sci. Rev. 2022 , 43 , 100439. [ Google Scholar ] [ CrossRef ]
Click here to enlarge figure
Research Direction | Antennas | Aircraft Detection | Remote Sensing | AI | IoT | Aircraft Control |
---|---|---|---|---|---|---|
Antennas | 22.150 | 3749 | 2176 | 3380 | 1092 | 1707 |
Aircraft Detection | 3749 | 5604 | 0462 | 1343 | 0133 | 0758 |
Remote Sensing | 2176 | 0462 | 3983 | 0707 | 0063 | 0 |
AI | 4231 | 2203 | 0777 | 7010 | 0512 | 0294 |
IoT | 1092 | 0133 | 0063 | 0365 | 1720 | 0043 |
Aircraft Control | 1707 | 1152 | 0 | 0311 | 0043 | 2504 |
Technology | Standard | Data Rate | Range |
---|---|---|---|
, , , , ] | 802.11 [ ] | Up to 2 Mbps | Up to 100 m |
LCY < 5 ms|DM:Y| | 802.11 a [ ] | Up to 54 Mbps | Up to 120 m |
UL NT:WLAN | 802.11 b [ ] | Up to 11 Mbps | Up to 140 m |
802.11 n [ ] | Up to 600 Mbps | Up to 250 m | |
802.11 g [ ] | Up to 54 Mbps | Up to 140 m | |
802.11 ac [ ] | Up to 866.7 Mbps | Up to 120 m | |
, ] | 802.15.4 | Up to 25 kbps | Up to 100 m |
LCY < 15 ms|DM:Y|UL | |||
] | 802.15.1 | Up to 2 Mbps | Up to 200 m |
LCY < 3 ms|DM:Y|UL | |||
] | IEEE | Up to 50 kbps | Up to 15 km |
DCD|DM:Y|UL | 802.15.4 g | ||
NT:WPAN | |||
] | - | Up to 100 bps | Up to 30 km |
LCY about 2 s|DM:Y|UL | |||
] | - | Up to 250 kbps | Up to 35 km |
LCY:1.6 to 10 s|DM:Y|L | |||
3G [ , , ] | HSPA+ | Up to 21.1 Mbps | Wide area |
LTE/LTEM | Up to 100 Mbps | ||
LCY:500 ms NT:LPWAN | |||
4G [ ] LCY:4ms | HSPA+ | Up to 100 Mbps | Wide area |
5G [ , , , ] | mMTC | Up to 1 Gbps | Wide area |
LCY:1 ms |DM:Y|L | URLLC | Up to 1 Gbps | Wide area |
B5G [ ] | eMBB/hybrid | Up to 100 Gbps | Wide area |
LCY:1 ms |DM:Y|L | URLLC | Up to 100 Gbps | Wide area |
6G [ , ] | MBRLLC | Up to 1 Tbps | Wide area |
LCY< 1 ms|DM:Y|L | mURLLC | Up to 1 Tbps | Wide area |
HCS / MPS | Up to 1 Tbps | Wide area |
Research Direction | Rate of Growth/Year (Number of Documents/Year) | Acceleration of Growth |
---|---|---|
Antenna | 980 | 1.20 |
Aircraft detection | 447 | 1.19 |
Remote sensing | 93 | 9.08 |
AI | 865 | 1.33 |
IoT | 155 | 1.34 |
Energy efficiency | 289 | 2.86 |
Control | 197 | 2.43 |
Swarm | 54 | 0.81 |
Control Technique | Advantage | Disadvantage |
---|---|---|
PID [ , , , , , , , , , ] | (1) Implementation is simple. (2) The reduction in steady state error can be achieved by increasing parameter gains. (3) It consumes minimal memory. (4) The design is user-friendly and it responds well. | (1) Conducting experiments can be a time-consuming process. (2) In certain cases, aggressive gain and overshooting may occur. (3) There is a possibility of overshoot occurrences when adjusting the parameters. |
SMC [ , , , , , , , , ] | (1) It exhibits high insensitivity to variations in parameters and disturbances. (2) It is capable of delivering significant implementation efforts. (3) Linearization of dynamics is not necessary for its operation. (4) It is efficient in terms of time. (5) Filtering techniques can be employed to reduce chattering effects. | (1) Severe chattering effects occur during switching. (2) The process of designing such a controller is intricate. (3) The sliding control scheme heavily depends on the sliding surface, and an incorrect design can result in unsatisfactory performance. |
LQR [ , , , , , , , ] | (1) Achieves robust stability while minimizing energy consumption. (2) Demonstrates computational efficiency. (3) The effectiveness of the system is enhanced by incorporating the Kalman filter. | (1) Complete access to the system states is necessary, but this is not always feasible. (2) There is no assurance regarding the speed of response. (3) It is not suitable for systems that demand a consistently minimal steady-state error. |
Gain Scheduling [ , , , , , , , ] | (1) Facilitates the rapid response of the controller to dynamic changes in operating conditions. (2) The design approach seamlessly integrates with the overall problem, even when dealing with challenging nonlinear problems. | (1) It is not time-efficient. (2) Gain scheduling heavily relies on conducting extensive simulations. (3) There are no guaranteed performance outcomes. |
Backstepping [ , , , , , , , ] | (1) Demonstrates robustness in the face of constant external disturbances. (2) Handles all states within the system and is capable of dealing with nonlinear systems. | (1) It is not efficient in terms of time. (2) It is sensitive to variations in parameters. (3) Implementation can be challenging. |
H-Infinity [ , , , , , , , , ] | (1) Capable of operating in the presence of uncertainties within a system. (2) Complex control problems are addressed in two subsections: stability and performance. (3) Offers robust performance. | (1) Involves intricate mathematical algorithms. (2) Implementation can be challenging. (3) It necessitates a reasonably accurate model of the system to be controlled. |
Adaptive control [ , , , , , , , , , , , ] | (1) Capable of handling systems with unpredictable parameter variations and disturbances. (2) Capable of handling unmodeled dynamics. (3) Exhibits rapid responsiveness to varying parameters. | (1) An accurate model of the system is necessary. (2) Implementing the design can be time-consuming. (3) It requires extensive design work before final implementations. |
AI: Fuzzy Logic and Neural Network [ , , , , , , , , , , ] | (1) The control action is heavily influenced by the provided rules. (2) The controller can be manually prepared. (3) Capable of withstanding unknown disturbances. (4) Offers adaptive parameters for uncertain models. (5) The selected control system can be trained. | (1) Stability cannot be guaranteed. (2) Continuous tuning is necessary for critical systems. (3) It consumes a significant amount of computational power. (4) Offline learning may fail when uncertainties are present. |
Simulation Software | Pros | Cons |
---|---|---|
UAV Toolbox MATLAB [ , , , ] PL: SOS: L: | ||
GazeBoSim [ , , ] PL: SOS: L: | ||
AirSim [ , , , , ] PL: SOS: L: |
The statements, opinions and data contained in all publications are solely those of the individual author(s) and contributor(s) and not of MDPI and/or the editor(s). MDPI and/or the editor(s) disclaim responsibility for any injury to people or property resulting from any ideas, methods, instructions or products referred to in the content. |
Share and Cite
Telli, K.; Kraa, O.; Himeur, Y.; Ouamane, A.; Boumehraz, M.; Atalla, S.; Mansoor, W. A Comprehensive Review of Recent Research Trends on Unmanned Aerial Vehicles (UAVs). Systems 2023 , 11 , 400. https://doi.org/10.3390/systems11080400
Telli K, Kraa O, Himeur Y, Ouamane A, Boumehraz M, Atalla S, Mansoor W. A Comprehensive Review of Recent Research Trends on Unmanned Aerial Vehicles (UAVs). Systems . 2023; 11(8):400. https://doi.org/10.3390/systems11080400
Telli, Khaled, Okba Kraa, Yassine Himeur, Abdelmalik Ouamane, Mohamed Boumehraz, Shadi Atalla, and Wathiq Mansoor. 2023. "A Comprehensive Review of Recent Research Trends on Unmanned Aerial Vehicles (UAVs)" Systems 11, no. 8: 400. https://doi.org/10.3390/systems11080400
Article Metrics
Article access statistics, further information, mdpi initiatives, follow mdpi.
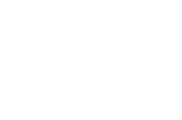
Subscribe to receive issue release notifications and newsletters from MDPI journals
Help | Advanced Search
Computer Science > Robotics
Title: a comprehensive review of recent research trends on uavs.
Abstract: The growing interest in unmanned aerial vehicles (UAVs) from both scientific and industrial sectors has attracted a wave of new researchers and substantial investments in this expansive field. However, due to the wide range of topics and subdomains within UAV research, newcomers may find themselves overwhelmed by the numerous options available. It is therefore crucial for those involved in UAV research to recognize its interdisciplinary nature and its connections with other disciplines. This paper presents a comprehensive overview of the UAV field, highlighting recent trends and advancements. Drawing on recent literature reviews and surveys, the review begins by classifying UAVs based on their flight characteristics. It then provides an overview of current research trends in UAVs, utilizing data from the Scopus database to quantify the number of scientific documents associated with each research direction and their interconnections. The paper also explores potential areas for further development in UAVs, including communication, artificial intelligence, remote sensing, miniaturization, swarming and cooperative control, and transformability. Additionally, it discusses the development of aircraft control, commonly used control techniques, and appropriate control algorithms in UAV research. Furthermore, the paper addresses the general hardware and software architecture of UAVs, their applications, and the key issues associated with them. It also provides an overview of current open-source software and hardware projects in the UAV field. By presenting a comprehensive view of the UAV field, this paper aims to enhance understanding of this rapidly evolving and highly interdisciplinary area of research.
Comments: | 32 pages, 4 figures, and 5 tables |
Subjects: | Robotics (cs.RO); Systems and Control (eess.SY) |
Cite as: | [cs.RO] |
(or [cs.RO] for this version) | |
Focus to learn more arXiv-issued DOI via DataCite |
Submission history
Access paper:.
- Other Formats

References & Citations
- Google Scholar
- Semantic Scholar
BibTeX formatted citation

Bibliographic and Citation Tools
Code, data and media associated with this article, recommenders and search tools.
- Institution
arXivLabs: experimental projects with community collaborators
arXivLabs is a framework that allows collaborators to develop and share new arXiv features directly on our website.
Both individuals and organizations that work with arXivLabs have embraced and accepted our values of openness, community, excellence, and user data privacy. arXiv is committed to these values and only works with partners that adhere to them.
Have an idea for a project that will add value for arXiv's community? Learn more about arXivLabs .
Advertisement
Unmanned aerial vehicles (UAVs): practical aspects, applications, open challenges, security issues, and future trends
- Review Article
- Published: 16 January 2023
- Volume 16 , pages 109–137, ( 2023 )
Cite this article
- Syed Agha Hassnain Mohsan ORCID: orcid.org/0000-0002-5810-4983 1 ,
- Nawaf Qasem Hamood Othman 2 ,
- Yanlong Li 1 , 3 ,
- Mohammed H. Alsharif 4 &
- Muhammad Asghar Khan 5
66k Accesses
127 Citations
4 Altmetric
Explore all metrics
Recently, unmanned aerial vehicles (UAVs) or drones have emerged as a ubiquitous and integral part of our society. They appear in great diversity in a multiplicity of applications for economic, commercial, leisure, military and academic purposes. The drone industry has seen a sharp uptake in the last decade as a model to manufacture and deliver convergence, offering synergy by incorporating multiple technologies. It is due to technological trends and rapid advancements in control, miniaturization, and computerization, which culminate in secure, lightweight, robust, more-accessible and cost-efficient UAVs. UAVs support implicit particularities including access to disaster-stricken zones, swift mobility, airborne missions and payload features. Despite these appealing benefits, UAVs face limitations in operability due to several critical concerns in terms of flight autonomy, path planning, battery endurance, flight time and limited payload carrying capability, as intuitively it is not recommended to load heavy objects such as batteries. As a result, the primary goal of this research is to provide insights into the potentials of UAVs, as well as their characteristics and functionality issues. This study provides a comprehensive review of UAVs, types, swarms, classifications, charging methods and regulations. Moreover, application scenarios, potential challenges and security issues are also examined. Finally, future research directions are identified to further hone the research work. We believe these insights will serve as guidelines and motivations for relevant researchers.
Similar content being viewed by others
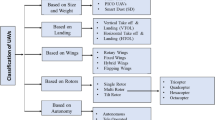
Review on design, development, and implementation of an unmanned aerial vehicle for various applications
Evolution and Significance of Unmanned Aerial Vehicles
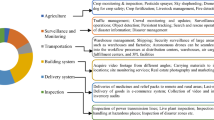
Recent Advances in Unmanned Aerial Vehicles: A Review
Explore related subjects.
- Artificial Intelligence
Avoid common mistakes on your manuscript.
1 Introduction
Unmanned aerial vehicles (UAVs), also known as drones, are being widely used and have gained significant attention in the last decade. Most of the studies report multirotors due to their simplicity in control mechanisms and high-precision in positioning. Other types of UAVs are also reported, but their numbers are comparably low. There are several limitations to the practical implementation of UAVs in different application scenarios. The main critical limitation among UAVs is flight endurance, which is limited due to the limited power supply provided by batteries. This issue can be mitigated through the design of different types of batteries using hybrid systems or internal combustion engines. Another promising solution is a docking station, which can recharge or swap batteries, store and even perform communication tasks with UAVs [ 1 ]. Docking stations can solve the battery endurance issue and put UAVs one step ahead in autonomous systems.
At present, UAVs are being used in multiple military, industrial and commercial applications [ 2 ], as shown in Fig. 1 . In the military, UAVs offer navigation, secure communication and reconnaissance. Furthermore, they are being used in mobile edge computing, cellular communication, package delivery, smart healthcare, intelligent transportation systems, video surveillance missions, precision agriculture, power-line inspection, remote sensing, search and rescue, and performing relief operations in disaster environments [ 3 ]. UAVs have the capability to access remote or dangerous areas, facilitate environmental monitoring and capture high-resolution imagery [ 4 ]. These flying objects are helpful in monitoring as they bridge the constraints in limited-access, dynamic, harsh and complex environments. Furthermore, they reduce the labor and time required to survey, inspect and sample on ground, provide collision avoidance and quick convergence to reach targets, and offer more time for intended managerial operations. However, there are multiple critical limits on the design, deployment and performance of UAVs. Some of them have limited flight time, limited autonomy, less mobility and limited battery endurance. Harsh weather conditions and environments also impose limitations of UAV performance. Limited mission time is due to low battery endurance, harsh atmospheric conditions and sensor accuracy challenges. Several promising solutions are presented in the literature to mitigate these challenges, including high-quality devices such as manufacturing materials and motors, UAV geometry, wings and batteries. Some studies also address optimization strategies to look for shortest trajectory for UAV flight to reach the target destination [ 5 ].
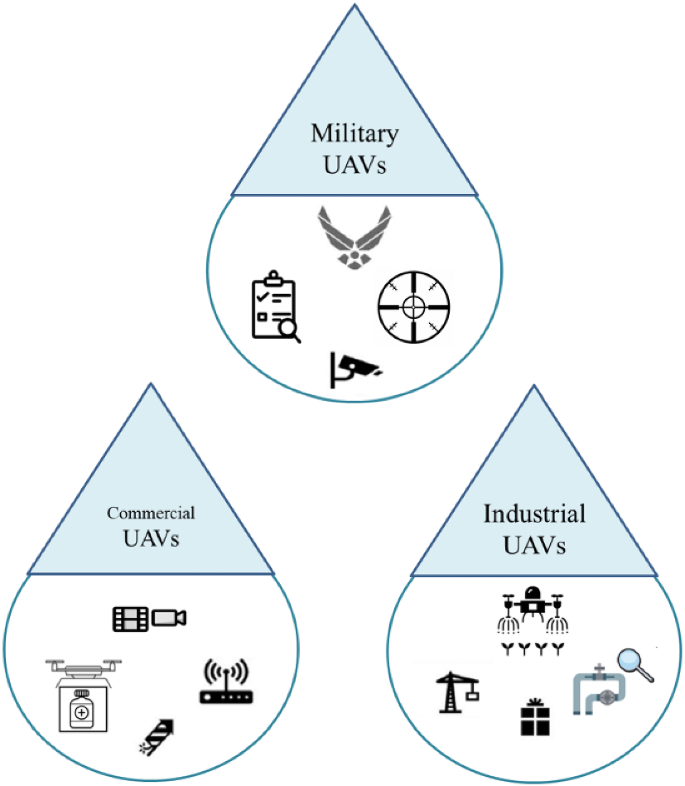
Commercial, industrial and military applications of UAVs
1.1 Scope and contributions
This review is devoted to UAV technology and will help readers understand ongoing research and development activities. Our goal is to support readers in getting an overview of UAV types, swarms, configurations, standardizations and charging techniques, etc. We provide a comprehensive review of UAV challenges and security issues. We also provide realistic recommendations and solutions to overcome these challenges. We carry out our extensive analysis to empower the use of UAVs in several application scenarios. In the end, we discuss future research directions to further hone the research work dedicated to this promising technology.
1.2 Organization of the paper
We have structured this study as follows: Sect. 2 reviews the research contributions on UAVs, types, classifications, payload, flight time, swarms and standardizations. Section 3 presents UAV battery charging techniques. Section 4 briefly discusses the UAV application areas. We examine open challenges in Sect. 5 , while security issues and mitigating solutions are addressed in Sect. 6 . We also present future research directions in Sect. 7 . Finally, this paper is concluded in Sect. 8 . The organization of this review can be seen in Fig. 2 .
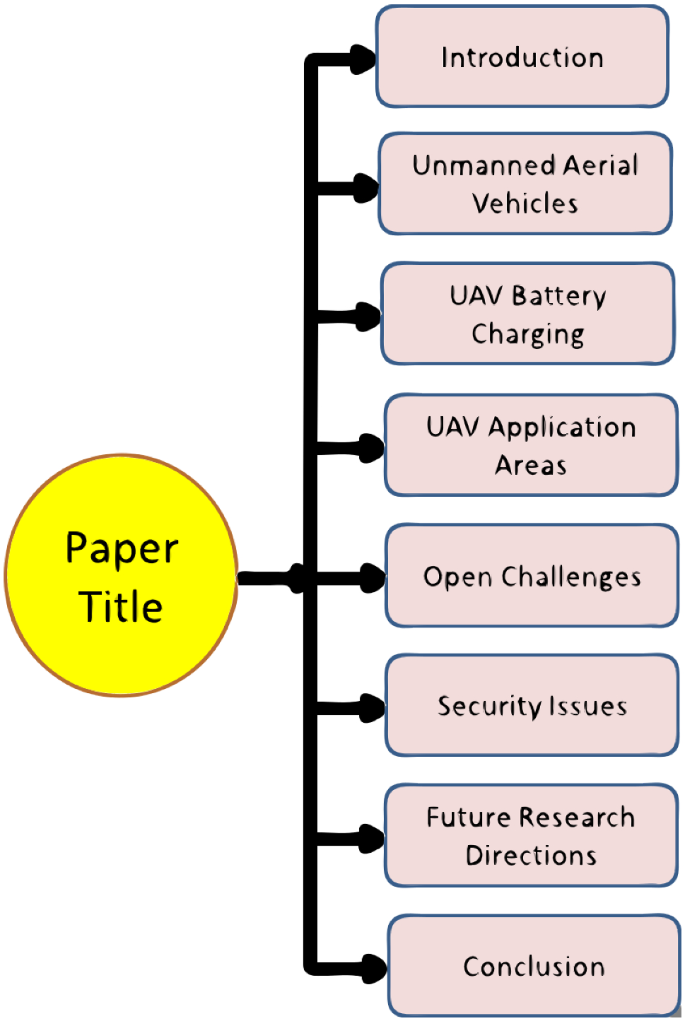
Organization of this paper
2 Unmanned aerial vehicles (UAVs)
UAVs refer to controlled aerial vehicles, which perform several missions without human assistance. They can be remotely operated through different electronic gadgets such as microprocessors and sensors [ 6 ]. UAVs can perform operations autonomously in such areas where human intervention is dangerous [ 7 ]. Figure 3 presents a general architecture of UAVs where they utilize communication links to establish connectivity with satellite or ground control system (GCS) such as a laptop or smart phone. A human operator is needed to operate and control the UAV remotely. In literature, several works have been presented to address different features of UAVs. For instance, Zhang et al. [ 8 ] have focused on mobile charging features for drones to enhance their battery life. Aldhaher et al. [ 9 ] the use of a wirelessly powered receiver in order to decrease the weight of UAVs. Several wireless charging techniques have been suggested in [ 10 ] to increase the UAV’s flight time. Raciti et al. [ 11 ] reported a charging system empowered by a wireless power transfer (WPT) system. This study also highlights misalignment losses and associated mitigation solutions. Rohan et al. [ 12 ], proposed an intelligent drone charging system empowered by WPT. Shin et al. [ 13 ] addressed charge scheduling for multi-drones networks based on deep learning algorithms. In Table 1 , we have summarized different surveys and reviews reporting on multiple aspects of UAVs.
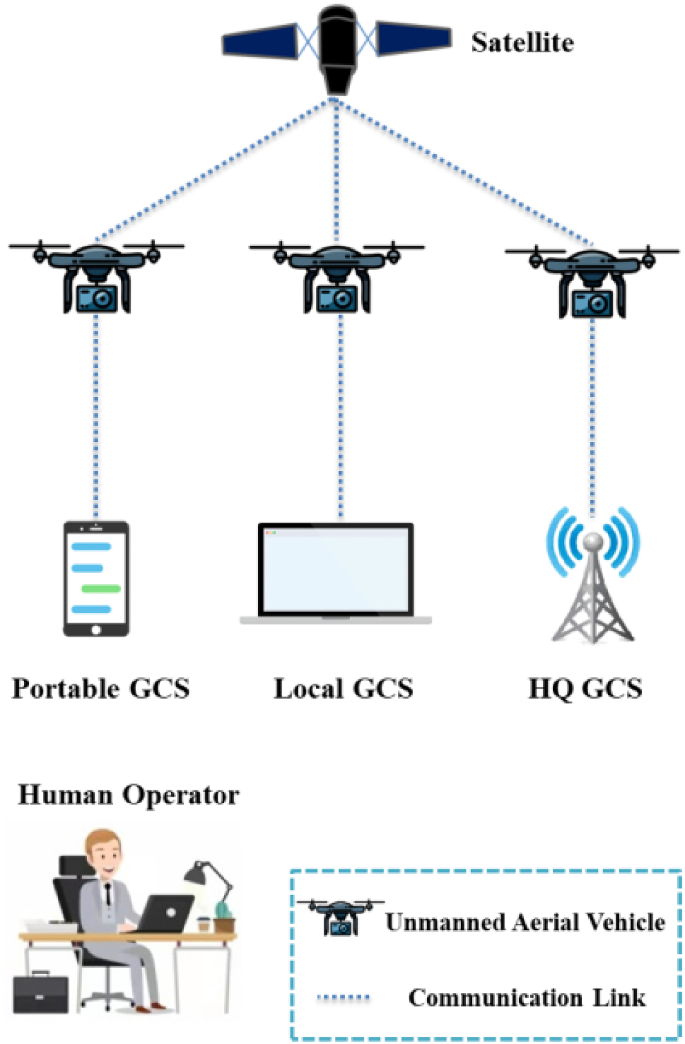
Architecture of UAV system
2.1 Classification of UAVs
UAVs come in a variety of specs, equipment, sizes, ranges, and forms. Different types of UAVs can be found in the literature: fixed-wing, single rotor, fixed-wing hybrid, and multirotor [ 31 ]. Fixed-wing UAVs contain a main body, wings, propeller and motor. These UAVs need special training to control and they remain useless for aerial surveillance. A fixed-wing UAV is depicted in Fig. 4 a [ 32 ]. These unmanned aerial vehicles are often used for aerial mapping and power line inspection. Such UAVs are not capable of hovering or forward flight. Figure 4 b depicts a hybrid fixed-wing UAV [ 33 ]. Figure 4c depicts a single-rotor UAV [ 34 ]. However, single-rotor UAVs are costly to produce and need skill training. These unmanned aerial vehicles are mechanically difficult and susceptible to obstacles such as vibrations. Furthermore, multirotor UAVs are the most affordable and simple to build. These unmanned aerial vehicles are often employed for image and video surveillance. Quadcopters are the most often utilized UAVs, as seen in Fig. 4 d. Quadcopters have gained popularity for their vertical landing, or quick maneuverability, low cost, and compact size. We have illustrated basic UAV types in Table 2 . While Table 3 provides a comparison of different UAVs considering various characteristics.

a Fixed-wing, b fixed wing hybrid, c single rotor, and d multirotor UAV
2.2 UAV swarms
Figure 5 depicts the classification of UAV swarms as partly and completely autonomous. This categorization is also divided into single and multi-layered swarms. Each drone in the swarm provides real-time data recording and processing capabilities. While core processing takes place in the base station (BS) or in the clouds. UAV swarms with sophisticated monitoring mechanisms can cover a zone reliably and quickly by deploying several parallel-operating drones. Numerous studies have been reported on the usefulness of UAV swarms. Such as [ 35 ] analyzes swarms of drones to solve the charging process of many drones at the same time. It employs UAV swarms for smart power management. When considering the mission accomplishment of swarms of UAVs such as quadcopters, UAV flight coordination and hovering endurance become critical. Furthermore, the communication efficiency of the quadcopter swarm is critical for a successful operation. The authors devised a multi-robot coordination strategy in [ 36 ] to address the challenges of real-time synchronization of UAV swarms across a broad area network. Other investigations have looked at swarms of UAVs in the context of surveillance. [ 37 ], for example, describes quadcopter swarms for object location and tracking operations. Similarly, Leonard et al. [ 38 ] suggested tracking and detection algorithms for a quadcopter swarm-based monitoring program.
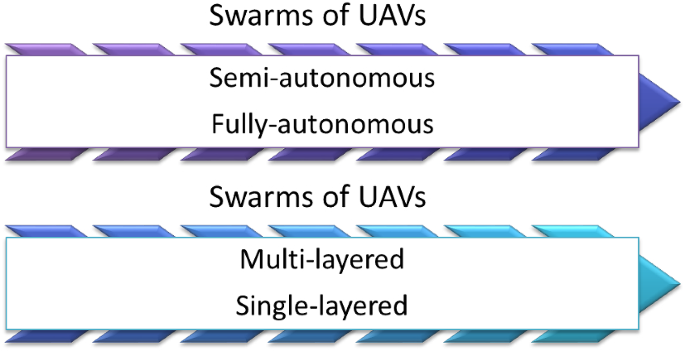
Classification of swarms
2.3 Developments in Navigation of UAVs
Navigation has a strong impact on UAV flight control. Several navigation technologies, such as Droppler, integrated, geometric, satellite and inertial navigation, perform multiple functions. The key navigation systems for UAVs are high-altitude, long-endurance navigation systems and tactical or medium range navigation systems. We can evaluate the development in navigation as follows:
High-performance navigation with data fusion: it plays a key role to determine the flight status to guarantee the normal UAV flight.
New inertial navigation system: it simplifies the volume and weight and takes less energy to refine the flight pliability.
Intelligent navigation system ability: information technology is used to enhance the UAV technology, along with updating the navigation system.
2.4 Formation control design
Motion control employee’s communication architecture needed for flow of information. Formation control strategies are as follows:
Leader–follower strategy: the main advantages of this technology are that it can be accomplished easily and simply. Owing to the leader dependency, it confronts single-point failures. The limitations of this strategy could be addressed by assigning virtual and multi-leaders.
Behavior-based strategy: the key benefit of this strategy is that it is greatly adaptable to even some unknown environments. However, it requires to be modeled mathematically which creates problems in analyzing stability of the system.
Virtual structure strategy: this strategy considers rigid structures for intended geometry or shape of the set of UAVs. The method fails to detect the faulty information in a UAV. The method also calls for a strong capability for computation.
2.5 UAV characteristics
2.5.1 speed and flight time.
Small-sized UAVs mostly fly at a speed of 15 m/s or below. On the other hand, large-sized UAVs can travel at higher speeds up to 100 m/s. Whenever any UAV is following any intended path to improve its energy or spectral efficiency, its speed should be taken into account at multiple turning points and locations along this path. In [ 38 ], authors have addressed this trade-off between UAV speed and turning agility.
Similarly, flight time indicates to the maximum time for which the UAV can fly until the batteries are drained out. It is of paramount significance along with cost. UAV weight, size and atmospheric conditions such as rain or wind have strong impact on battery endurance of UAVs which is closely associated to flight time. GPS and autopilot systems also play a crucial role in flight time. Small size UAVs can fly up to 20–30 min, while large size UAVs can fly up to several hours.
2.5.2 Payload
The lifting capability of a UAV to carry any weight is referred to as payload. Lifting capacity ranges from a few grams to several hundred kilos. Greater payloads allow for more accessories to be carried at the expense of shorter flying times, greater power capacity, and wider dimensions. Sensors and video cameras are common payloads for reconnaissance, surveillance, and commercial purposes. Cellular user equipment (UEs), such as tablets, mobile phones, and tablets weighing less than one kilogram, may also be carried by UAVs [ 39 ]. UAVs with high payloads are thought to have shorter flight duration. However, if a UAV has a larger surface area along with occupying more motors; it has the ability to store more power, which increases flying time. As a result, the payload's quality might let it move faster while maintaining the same precision and resolution. In Table 4 , we have illustrated payload capability of different UAVs.
2.5.3 Sensing equipment
Radiation detection has already been discussed in ground penetrating radar, and hyper-spectral cameras to Synthetic-Aperture Radar. The author identifies highly sensitive light sensors in the field of precision agriculture:
Fluorescence excitation
Infrared spectrum imaging, and
Visible spectrum imaging
The particular sensing arrays are highly precise but the specialized equipment is highly expensive with limited versatility. Table 5 presents an overview of some sensing devices in UAVs. While Table 6 provides comparison of different imaging and ranging sensors considering various parameters.
2.5.4 Software
UAVs are significantly capable of playing roles in data processing tasks and problem solving. Several algorithms are used for optimization, autonomy and path planning. These tools can minimize human assistance and the infrastructure required for various tasks, such as communication. It is challenging to figure out suitable off-the-shelf software, which requires time and resources. However, researchers are on the way to developing novel methods and algorithms to address a variety of such problems. A geometric correction algorithm can be employed to decrease image distortion. Image processing algorithms are used for remote sensing or to process images captured from UAV cameras. Similarly, an offline path planning tool can be employed for UAV- swarm aided persistent surveillance methods and a self-adapting multi-featured evolution approach offering trajectory planning for UAVs.
The software installed in UAVs has been validated as an essential factor in value creation. This concept has paralleled the expansion of industries and companies devoted to software development. Among these companies are Skyworks Aerial System (USA), SkyWards (USA), RedBird (France), PIX4D (Switzerland), MapBox (USA), DroneDeploy (USA), Dedrone (Germany), and Airware (USA). Most of these countries are from European countries and USA, outlining the emerging industry of this transformative technology. Some of these companies focus on processing and analysis through captured images through UAVs, using their results to various industries such as construction, surveying, agriculture and mining, etc. While others operate as flying simulators (Redbird) and developers of UAV detection (Dedrone).
2.5.5 Range and altitude
The range of a UAV relates to the area it can be operated remotely from. Small drones have a range of a few meters, whereas bigger drones have a range of hundreds of kilometers. In contrast, altitude points to the maximum height at which a drone may fly. Aerial platforms are usually split into two groups based on their altitude.
Low-altitude platforms (LAPs): LAPs are typically used to facilitate cellular connectivity since they are quick to deploy and inexpensive. LAPs also provide a line-of-sight route, which can boost communication performance greatly [ 49 ].
High-altitude platforms (HAPs): high-altitude platforms (HAPs), including balloons, are also employed for cellular communication. When compared to LAPs, HAPs provide more coverage. The implementation of HAPs is complex, and they are mostly used to facilitate Internet connectivity. Table 7 shows the various kinds of UAVs based on their altitude. Table 8 provides a comparison of several types of UAVs. Figures 6 a–d depict several UAV programs in various countries.
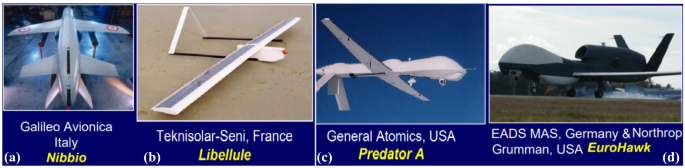
Different examples of UAVs on altitude a LADP, b LALE, c MALE, and d HALE [ 51 ]
2.5.6 Controllers of UAVs
Controllers are responsible for executing specific tasks for UAVs. They are defined as a series of actions that can affect UAVs and the physical world. The controllers vary according to the UAV type such as flapping wing, single rotor, multirotor and fixed-wing. These UAVs are designed for certain operations and face different aerodynamics. UAV control encompasses an extensive range of technologies and treatments. For some certain operations, the control may contain the macro- executions (such as formation control and path planning) and the micro flight control decisions of the actuators according to the provided commands. Due to the dynamic behavior of UAV configurations, the design and development of UAV control must follow an appropriate mechanism. As a result of the external disturbances, the operability of the flight control system is critical to following the stable response. The key objective of the controller is to reduce the error between the estimated and desired states, which can be altitude, velocity, or position of the UAV operating under varying atmospheric conditions. Next, we discussed the flight controller for UAVs in detail.
2.5.7 Flight controllers
Flight controllers are commonly known as autopilot systems, are the main elements of UAVs. They are generally designed to ensure autonomous flight control, such as mission planning, flight waypoint generation and attitude stabilization. To efficiently realize these operations, flight controllers require software or hardware assistance concurrently. The latter mostly consists of power control modules, communication entities, GPS modules, onboard sensors, inertial measurement units and onboard computers, and the former mainly contains signal processing algorithms, attitude control, path planning and task allocation.
The flight controller is based on three major components. The first one is named "kernel control law," which is responsible for the asymptotic stability of the UAV’s mobility within the air. The second component is called the command generator, which is used to generate references for the kernel control. The last part is the flight schedule, which is responsible for generating a group of flight arrangements to realize the desired mission. Flight control of a UAV can be achieved by controlling the altitude in order to maintain the UAV at the desired height and controlling the orientation and velocity to follow specific trajectories. Different linear and non-linear controllers are used for UAVs. The linear controllers include H¥, LRQ, PD, and PID, and non-linear controllers include NDI, gain-scheduling, fuzzy logic control, nested saturation, sliding mode, and backstepping. The linear controllers are as follows:
The H¥ Loop-Forming technique, which integrates conventional loop forming control with robust control, provides high robustness than other techniques. However, it is not feasible for large-scale UAV control.
A linear quadratic regulator (LQR) controller is an optimal controller which operates in dynamic systems with quadratic costs. It is regarded robust concerning technique uncertainty with a viable stability margin to error. However, it is not feasible always as it needs the full state of the system.
A proportional–integral–derivative (PID) controller is the most commonly used controller in UAVs. They are very popular due to their ease of use. However, they also impose limitation in optimality and robustness.
Nonlinear controllers are required to tackle with coupling components and non-linearity of UAV state variables. Nonlinear controllers are as follows:
Robust Control Algorithms are utilized to realize the effective behavior of the controller within an acceptable disturbance ratio. It can measure the performance changes with respect to the changing system parameters. It is feasible approach as it needs only the information about the limit in uncertainty.
The Backstepping Controller is a recursive design process which divides the controller task into various steps to ensure gradual stability in each sub-system. It can tackle external uncertainty and utilize less computational resources.
The Sliding Mode Controller (SMC) follows Lyapunov stability principles to force system state trajectory through a discontinuous control signal. It is inherently robust to any changes in parametric uncertainty, external disturbances and modeling errors. It is very feasible to analyze the second order dynamic systems. Table 9 provides a comparison of different flight controller.
2.6 UAV standardizationsp
2.6.1 uavs 3gpp standardization.
In this technological era, UAVs have gotten a lot of attention in the line-of-sight (LoS) and non-line-of-sight (NLoS) environments [ 56 ]. The third generation partnership group (3GPP) completed numerous investigations in 2017 and produced Release-15 to recognize long-term evolution (LTE)-powered UAVs. The key objective of this research was to concentrate on UAV traffic demands, channel modeling methodologies for air-to-ground dissemination views, reuse of existing cellular networks to facilitate LTE support, UAV communication, and innovations necessary to incorporate LTE into UAVs. The 3GPP's goals also include determining which traffic categories require current cellular networks to accommodate UAVs flying more than 300 m above the ground. Table 10 highlights the following UAV communication needs: (i) synchronization and radio control; (ii) command and control; and (iii) application data [ 18 ].
2.6.2 UAVs standardization outside the 3GPP
Aside from 3GPP standardization, numerous additional regulatory bodies have specified distinct UAV requirements to assure effective, consistent, and standardized communication.
The Institute of Electrical and Electronics Engineers (IEEE) established the Drones Working Group (DWG) in 2015. The primary goal of this group was to create a taxonomy for consumer drones in order to emphasize privacy and security issues. In order to do this, the DWG creates techniques, systems, specifications, testing, and evaluations for consumer drones to protect the privacy and security of the public and their properties. Similarly, the IEEE Conformity Assessment Program (ICAP) and OM/AerCom SC are collaboratively designing a conformity assessment program such that UAV approaches can show conformance with IEEE standardizations. The IEEE drone application certificate program is also introduced which is comprised of industry stakeholders, that will support regulators, system integrators, solution providers, manufacturers, users and other potential beneficiaries. It also includes the academic community and test laborite to demonstrate to buyers that UAV systems or products conform to IEEE standardizations.
The European Telecommunications Standards Institute (ETSI) investigates UAV operations, use cases, and knowledge of the to-be-built Internet Protocol (IP) suite framework, as well as the spectrum rules required to accommodate UAVs in current LTE networks [ 57 ]. ETSI has also developed European standards under EC regulation requests to ensure the extensive use of services such as Data Link Services (DLS), Advanced Surface Movement Guidance and Control along with Airport Collaborative Decision Making procedure. Furthermore, Radio Equipment Directive has been developed to employ on UAVs operating in conformity with the spectrum management. ETSI can also be utilized to show compliance with certain aspects of the directive.
The International Telecommunication Union (ITU-T) made work item (WI) Y.UAV.arch so that unmanned aerial vehicles (UAVs) and unmanned aerial vehicle controllers (UAVs) can have a stable and functional architecture over IMT-2020 networks [ 58 ]. IMT-2020 is employed for UAV communication. As UAVs need simultaneous applications with different aspects, thus a new kind of user terminal to IMT-2020 is needed. Likewise, the novel communication network IMT-2020 is designed for ground coverage of UAVs. A group of functionalities is required to fill the gap for interoperability between IMT-202 networks and UAVs in order to improve the quality of UAV operations and implement the feature of civilian UAV operations in IMT-2020. This recommendation supports an operational architecture for UAV operators through IMT-2020 networks and functionalities defined in the physical layer, application layer, and application support layer.
Federal aviation authority (FAA) is responsible for civil aircraft operations in national airspace (NAS) in the USA. It aims to provide operational and regulatory framework for unmanned traffic management (UTM). It interacts with UTM for data or information exchange. In March 2020, FAA released an updated UTM concept in order to define the technical and operational requirements for UTM ecosystem to ensure the operations of unmanned airspace systems in all existing airspaces. FAA has also set a limit of weight less than 55 pounds to include as small UAS. In general, FAA divides the existing airspace in six classes A to G. G refers to uncontrolled while class A-G is for controlled airspace. It means class G will not be monitored by air traffic control will be overseen by ATC entities. According to FAA rules, UTM operation can be performed till 400ft above ground. In case of uncontrolled airspace, the remote pilots are exempted to get approval from authorized training center (ATC) authorities. However, while entering into controlled airspace, they must inform ATC about their intent. Moreover, the UAV operator should guarantee that the foreseen UTM operations are compliant with the certain rules, e.g., e-registration of both operator and UAV and must satisfy safety requirements including communication abilities and drone airworthiness [ 59 ].
European Aviation Safety Agency (EASA) has also implemented regulations to operate UAVs. The EASA concepts are based on a regulatory framework considering operation-centric and proportional strategy which addresses conditions and the way of the operation rather than just focusing on UAV parameters. These actions are guided and accompanied by the European Roadmap to integrate civil remotely-piloted aircraft into the European Aviation System. The document identified several levels of integration and harmonization. It also contains three annexes with a clear strategy to implement and improve regulations, foreseeable research initiatives and societal impacts on UAV services. The major difference between proposed concepts is the application of three EASA drone categories such as OPEN, SPECIFIC and CERTIFIED [ 59 ]. Thus, UAV operations performed under both SPECIFIC and CERTIFIED must be authorized by the national aviation authorization (NAA). This authorization will allow UAV operations with high risk levels such as flying over populated area. Consequently, specific system capabilities and operational requirements must be satisfied by the operator to perform these operations.
2.7 UAV docking station: preliminary description
Docking stations are multipurpose to facilitate safe landing, recharge, or take off, payload and data transfer. Some docking systems are capable of storing UAVs safely, protecting them from harsh environmental conditions. Docking stations can be categorized regarding [ 1 ]:
Charging method
Automatic battery exchange
Positioning
Drone storage
Package delivery
Landing type
Landing platform type
Docking station comprises multiple subsystems, for example, power supply, a landing platform, battery recharging setup, drone storage system and many more. Docking stations should satisfy specific criteria to meet their goals. The classification of UAV docking stations is illustrated in Fig. 7 . We have summarized some commercially available docking stations in Table 11 . In next section, we will comprehensively discuss the UAV charging techniques.
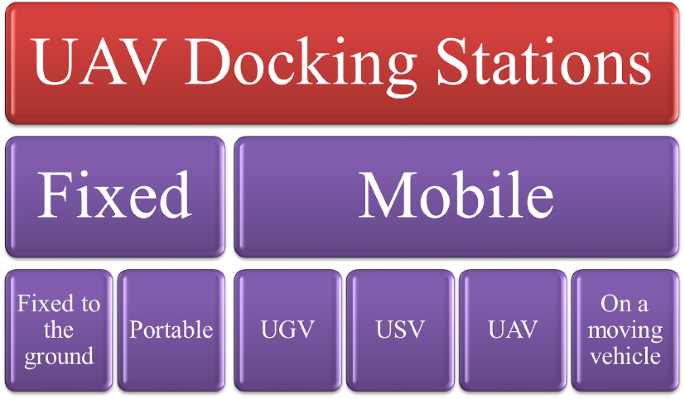
Classification of UAV docking stations [ 1 ]
3 UAV battery charging
One major issue in UAV performance is limited battery capacity. It is not suggested to increase battery weight or size as ultimately it will enhance payload which is another crucial issue. Charging can be obtained through wired or wireless power transfer (WPT) system [ 65 ] as discussed below:
3.1 Wireless power tranfer (WPT)
According to an analysis [ 66 ], the estimated revenue of WPT is around $2000 million till 2020. Figure 8 provides the estimated growth market for WPT and drones till 2025. It is envisaged that total sales with a Compound Annual Growth Rate (CARG) of 13.8% by 2025 will hit $43 billion [ 67 ]. This extensive growth is due to several applications in electronic industry with multiple advantages in terms of autonomy, reliability, convenience, security and safety. All these advantages can be obtained using various WPT techniques. One key benefit of using WPT techniques is the operability in those environments where wired medium power transfer is impossible or dangerous. WPT techniques are organized as radiative electromagnetic (EM) and non-EM techniques. In non-EM, power is transferred through optical or acoustic sources. In EM techniques; resonance coupling, magnetic coupling, inductive coupling and capacitive coupling are used. At present, these WPT technologies are commercially used for several applications such as implantable medical devices, smart phones and UAVs etc.
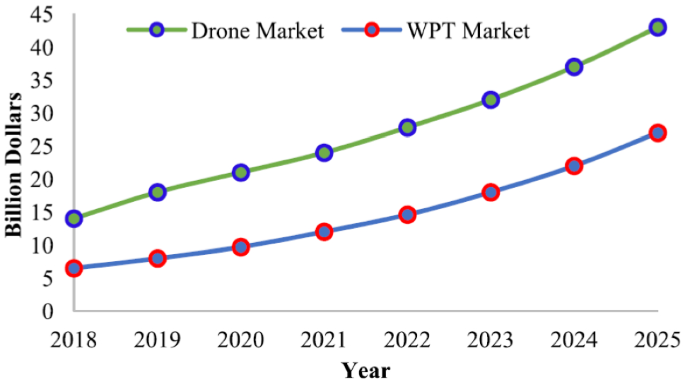
Statistics of WPT and Drones market growth [ 68 ]
WPT techniques offer reliable and efficient power transmission for UAVs. However, WPT techniques for charging UAVs must consider critical limitations under payload, interference, harsh weather conditions and misalignment. Among these critical factors, misalignment is a dominant challenge as UAVs mostly suffer from landing accuracy issues. UAV-empowered WPT techniques must be lightweight. These techniques should ensure efficient charging and high precision landing along with tolerance to misalignment factor. Several research works have been carried out for UAV charging through different WPT techniques [ 69 , 70 ]. We have summarized some of these studies addressing UAV-based WPT systems in Table 12 .
3.1.1 Photovoltaic (PV) cell-based UAV charging
The PV cells are used to charge batteries by using sunlight and they can significantly enhance the flight time of UAVs. In the presence of sunlight, the PV cells provide the required power to UAVs. However, in the absence of sunlight, the required power is delivered through batteries. Several research studies have been reported on solar-powered UAVs [ 79 , 80 ]. According to Fazelpour et al. [ 79 ], various parameters play a major role in solar-powered energy transfer such as type of PV cell, position, orientation, geometry, temperature intensity, weather conditions and angle of incidence of sun. However, this technique is critically limited in the absence of sunlight. Atmospheric conditions such as clouds, fog, rain, temperature, humidity can substantially reduce the UAV’s efficiency and reliability. Thus, it is suggested to investigate alternative solutions to continue the UAV mission under such scenarios. Controlling PV cell position based on incident sunlight, increasing PV cell size or UAV batteries, and carrying additional power supplies are some of these strategies.
Currently, different types of PV cells are available to ensure high-efficiency and cost-effectiveness. Some studies have focused on mono-crystalline silicon PV cells as they support high flexibility and easy integration with UAV wings [ 81 ]. Research fraternity is still looking for novel designs and energy management strategies with enhanced efficiency, high availability and reliability. There is a need to focus on research activities in material perspective as low-efficiency of these PV cells is a major limit [ 82 ]. In [ 83 ], authors proposed optimization approach for solar-powered UAV trajectory in order to attain high solar radiation at low usage of mechanical energy. In [ 84 ], authors reported that solar power may be beneficial for small size aircraft. They plotted a graph as shown in Fig. 9 considering PV cell size, drone size and mass for a continuous hovering flight. To plot this graph, authors assumed 30% efficient cells, 1/3 sunlight and drones capable of sensor-autonomous hovering. Table 13 shows the PV cell size required for persistent solar-powered hovering for different UAVs.
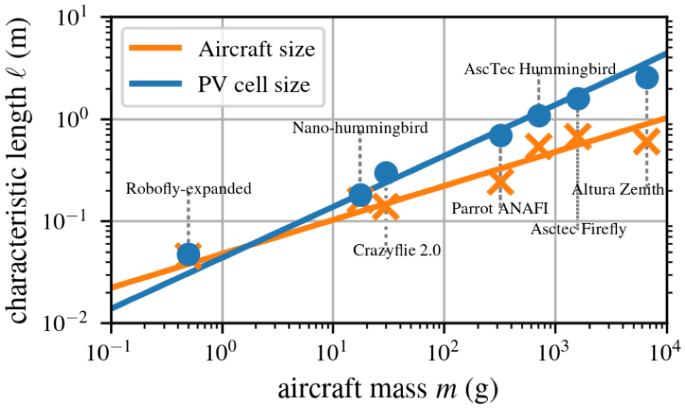
PV-powered hovering favors small aircraft [ 84 ]
Solar-powered UAVs have gained significant attention in both academia and industrial sectors. Several research and industrial activities have been initiated on solar-powered UAVs. Figure 10 shows solar-powered drones projects by technology giants e.g., Google and Facebook. Google started using these UAVs to provide internet access in remote areas. At present, such UAVs are being used for forest fire fighting, internet coverage, high-altitude communication, border monitoring, and power-line inspection.
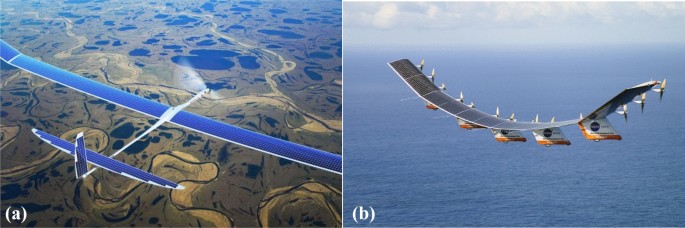
Solar-powered drones a Google’s project, b Facebook’s project [ 92 ]
3.1.2 Charging with laser beaming
Laser power transfer (LPT) is another promising charging technique, which is commonly used in space and military missions [ 93 ]. In this charging method, laser diodes of different wavelengths are used to deliver power to PV cells integrated on UAVs. These PV cells harvest energy from laser beams to charge batteries or deliver the required power to UAVs. This charging technique is mostly used for fixed wing and rotary wing UAVs. Laser power transfer is considered a promising approach to ensure unlimited endurance. It can deliver high energy to support various energy-hungry remote operations of UAVs [ 94 ]. Several studies have been reported on LPT techniques [ 95 , 96 ]. In [ 96 ], the authors introduced a controller design mechanism considering laser PV module to adjust the power conversion of LPT system. In another reported work [ 97 ], the authors discussed results pertaining to voltage, current and efficiency considering a LPT system. Furthermore, author in [ 98 ] carried out analysis of wavelength and temperature output of PV cells in a laser-based charging system. In [ 99 ], authors discussed UAV-based missions for longer durations considering different techniques. Authors discussed laser charging based on low-power laser source and precise energy consumption for UAV along with investigating its dynamics in a mission environment. In another reported study [ 100 ], authors investigated the joint problem of trajectory and power optimization in a rotary-wing UAV-empowered mobile relaying network. In order to support sustainable and convenient energy supply, they considered wireless charging of UAV through a power beacon and properly realized through a laser charging system. Apart from different advantages of LPT systems, there are several critical issues such as mobility, blockage and limited performance on long-distance flight. Moreover, this system is not feasible in airports and military areas where laser beam usage can be harmful. In Fig. 11 , we present a LPT system to charge multi-UAVs.
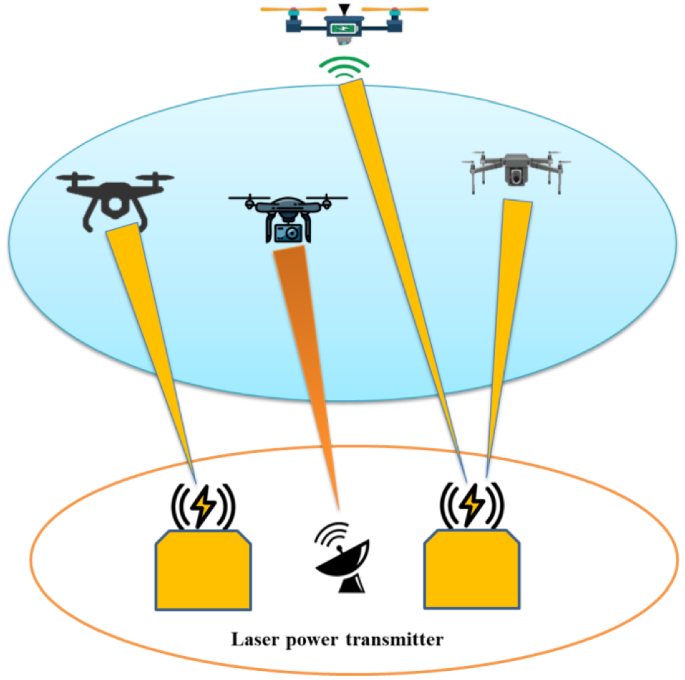
LPT system to charge multi-UAVs
4 UAV application areas
The ongoing activities on UAV deployment have created a new breed of promising applications to carry out autonomous missions. This section highlights several prominent application areas of UAVs.
4.1 Disaster management
UAVs can visit disaster regions that are unsafe for manned action in the event of a man-made or environmental catastrophe, such as terrorist strikes, tsunamis, and flooding. Power, telecommunications infrastructure, water utilities, and transportation are all vulnerable to these calamities. UAVs can assist in the collection of data, the need for quick answers, and the navigation of debris. UAVs equipped with sensors, radars, and high-resolution cameras can aid rescue teams in identifying damage, launching urgent recovery efforts, and dispatching supplies including first-aid manned helicopters and medical kits. UAVs can aid with catastrophe assessment, disaster alarms, and discovering preventative measures in real-time. A swarm of drones equipped with firefighting equipment can watch, analyze, and track any region in the event of a wildfire without jeopardizing human life. As a result, UAVs may help with real-time surveillance of a vast region without jeopardizing the safety and security of anyone involved. Unmanned aerial vehicles (UAVs) can help to find people and animals in danger so they can be saved.
4.2 Remote sensing
Drone technology is increasingly being applied by amateurs to collect high-resolution imaging data of isolated places, such as mountaintops, coastlines, and islands. The usage of UAVs serves as a link between aerial, ground-based and space-borne remotely sensed data. UAVs' cost-effectiveness and ultralight features enable spatial and temporal resolution observation. Disease diagnosis, water quality inspection, famine monitoring, gas and oil yield estimations, conservation of natural resources, geological calamity survey, topographical survey, woodland mapping, hydrological modeling, and crop management are all possible applications of UAV remotely sensed. Poor water quality because of wastewater discharge and nutrient pollution is a critical concern in some marine and coastal environments. Particularly, nutrient flow from agricultural, urban, and industrial activities can cause harmful algal blooms (HABs), ultimately producing the harmful toxins. Some research labs in Virginia and Florida, USA are focusing on the use of UAVs to early trace and detect HABs through hyperspectral sensors. However, there are several challenges which must be tackled to make this technology practical for coastal monitoring activities. Moreover, this technique is also being utilized in archaeological and cartography for crowd-sourced mapping and the generation of 3D atmospheric maps. Drones may deliver current data at low cost, allowing land planners to avoid depending on obsolete mapping sources.
4.3 Search and rescue (SAR)
Unmanned aerial vehicles (UAVs) are considered critical in areas like disaster risk management, rescue missions, and public security. UAVs may save a lot of time and resources by providing real-time imaging data of desired sites. Resulting that, the SAR group is able to detect and pinpoint precisely where aid is necessary. Drones, for example, can be used to follow lost mountaineers on any trip or to defend humans in any isolated forest or desert. Drones may therefore aid in the tracking of unlucky victims as well as any difficult terrain or harsh weather conditions. Drones can deliver critical medical equipment before an emergency or physician arrives. Drones containing food supplies and medical supplies such as vaccines, medical kits and life-saving jackets can be sent to disaster-stricken communities and isolated locations. Drones, for example, can deliver clothing, water, and other essentials to stranded people in difficult regions before rescue workers arrive. This technology can help speed up SAR efforts in disasters such as mission personnel, avalanches, forest fires, and deadly gas penetration.
4.4 Infrastructure and construction inspection
UAVs have made as-built maps, project monitoring, and surveys more efficient, simple, and quick. Tracking the development of the building project from start to finish ensures that the work on the site is of high quality. It may deliver reports to prospective stakeholders that include pictures, video, and 3D mapping. Infrastructure and construction inspection applications can benefit greatly from this approach. UAVs are gaining high popularity for evaluating the global system for mobile communication (GSM) towers, keeping an eye on gas pipelines, inspecting power cables, and keeping an eye on building projects [ 101 ].
4.5 Precision agriculture
UAVs could be installed in smart agriculture to obtain specific information through ground sensors (quality of water, soil composition, humidity, and so on), spray pesticides, diagnose illness, schedule irrigation, detect weeds, and monitor and manage crops. The applicability of UAVs in precision agriculture is a cost-effective and time-saving strategy that can improve agricultural systems' revenue, performance, and agricultural production. Furthermore, UAVs aid in insect damage, weed monitoring, chemical spraying, and farm management, resulting in higher crop yields to handle these difficulties quickly. UAVs combined with remote sensing have the potential to revolutionize smart farming. It offers temporal, geographical, and spectral resolution, as well as multi-angular observation and comprehensive vegetation height data. By undertaking sophisticated aerial mapping, UAVs can have a huge influence on the agricultural system. UAVs outfitted with the necessary cameras and sensors can analyze crop health status, including leaf area, foreign pollutants, chlorophyll content, and temperatures [ 102 ]. WH Maes et al. evaluated the advancement of remote sensing through drones in growth vigor evaluation, nutritional status, disease and weed identification, and drought stress [ 103 ]. With more UAV photos, digital image methods will be able to study plant diseases and other things in the future.
4.6 Real-time monitoring of road traffic
The integration of UAVs with road traffic monitoring (RTM) systems has piqued the curiosity of many. UAVs can accomplish 100% automation of the transportation industry in RTM [ 104 ]. Rescue squads, roadway surveyors, traffic cops, and field support personnel will all be automated. Reliable and intelligent UAVs can support with the automation of these parts. UAVs have emerged as a new viable instrument for gathering data on highway traffic situations. As compared to traditional monitoring systems e.g., surveillance cameras, ultrasonic sensors, and circuit analyzers, low-cost UAVs, or drones, can inspect large sections of road [ 101 ]. Local police can use drones to gain a clear picture of road accidents or to conduct a large security crackdown on illegal activities along the highway, including car theft. Some of the other implications include vehicle recognition; raids on suspect cars; pursuing hijackers and armed robbers, or anybody who breaches traffic regulations. It may also be used to monitor driving and incidents in vehicles and probably prevent traffic bottlenecks and overcrowding [ 105 ]. Figure 12 depicts an overview of UAV service on the roadway.
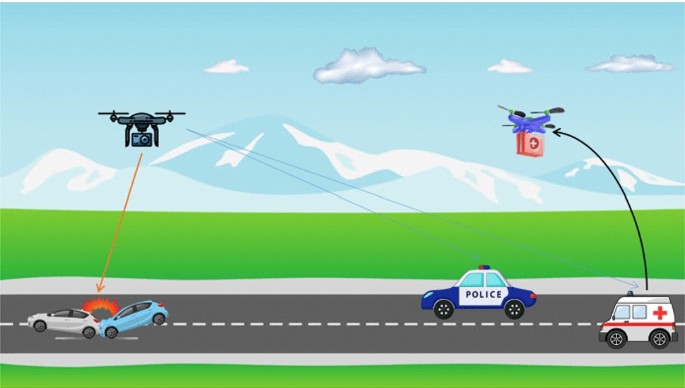
UAV assistance on highway
Likewise, UAVs may be used to monitor road conditions, such as detecting fractures and providing early warning to avert traffic accidents and reduce damage. At present, road inspection and monitoring are carried out using human vehicles, and the level of automation must be increased. The combination of road inspection technologies with UAVs has the potential to drastically minimize road damage. UAVs can take pictures of road cracks and use target detection algorithms to figure out what they are.
4.7 UAVs for automated forest restoration
One more developing study area is the use of UAVs for controlled forest restoration (AFR). UAVs could be used to aid in the accomplishment of re-vegetation activities e.g., site infrastructure, site inspection, restoration plan, seedling supply, site maintenance (germinating and weeding, for example), and bio-diversity survey after restoring interventions [ 106 ]. Existing technology, such as GPS and image sensors, assists UAVs in performing specific tasks, such as fundamental pre-restoration site inspections and monitoring various aspects of biodiversity revival. UAVs could evaluate any alterations in temperature, forest functions, and ecological composition, hence assisting in the surveillance of replanting [ 107 ]. High-resolution cameras mounted on UAVs can give useful data on natural forests to aid in forest restoration initiatives. Because of their simplicity of data acquisition and mobility, UAV cameras with suitable resolution can aid in the characterization and study of forest landscapes. Likewise, optical sensors mounted on UAVs are being used to obtain geometric properties of forests, such as vegetation cover, diameter, and length [ 108 ]. UAVs can also be used for remote sensing, which is a reliable and effective way to monitor forests that is different from the way it has been done in the past.
4.8 UAVs for monitoring of overhead power lines
Power line fault detection and avoidance are critical for the reliability and quality of generation capacity. Traditional procedures have several disadvantages, including expensive costs, inconvenient deployment, and dangerous dangers. As a result, researchers are interested in UAV-based power line distribution and inspection, as illustrated in Fig. 13 . The safety of a power transmission system is sometimes referred to as power line inspection. The use of a digital camera installed on UAV to photograph power line corridors is a practical way to perform inspection duties [ 109 ]. UAVs can also be installed to look for broken bolts, rust or corrosion, and lightning strikes on electrical pylons. Short-circuiting issue of electricity lines is most commonly caused by severe weather, bushfires, and tree falls. Authors of a recent research [ 110 ] examined the use of UAVs to detect and monitor defects in overhead transmission lines. To find defects, both climbing robots and flying UAVs can be utilized. These inspection tasks may be carried out by UAVs at a cheaper cost than helicopters and with a lower risk than traditional foot patrol. Fixed-wing UAVs, which can fly at high altitude and speed than other types of UAVs, are most commonly used for rough inspection. Multirotor UAVs, on the other hand, obtain photographs in the air at a closer distance from the objects by hovering. Because of their excellent 3D mobility, multirotor UAVs are ideal. Despite these advantages, autonomous mission planning and piloting of a multirotor UAV in a limited or complicated area is difficult. Advanced data collection, exchange, and processing tools for cooperative UAV networks should be researched in the future to provide reliable, efficient, and speedier inspections.
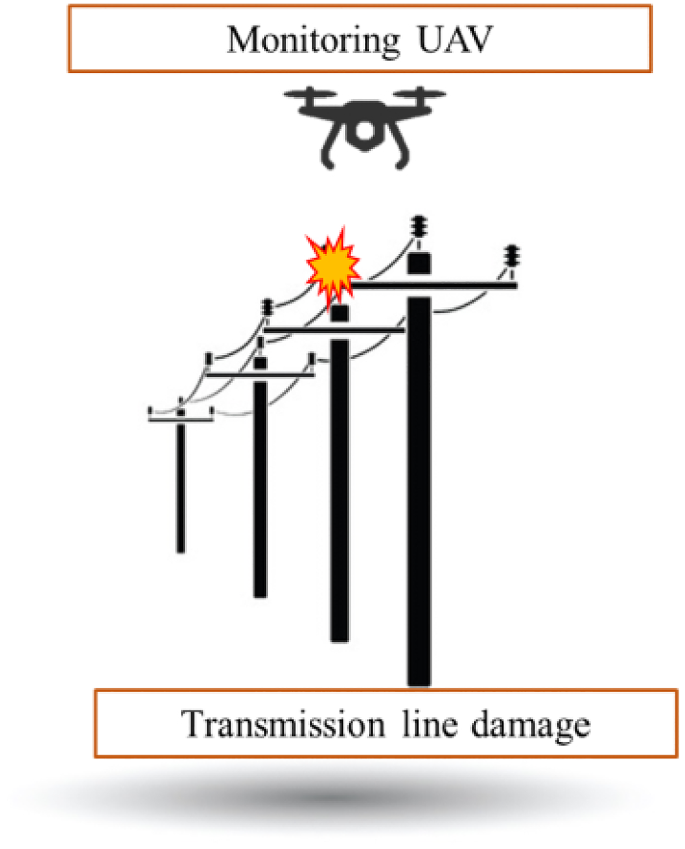
Inspection of power lines through UAV
4.9 UAVs for monitoring and assessing plant stress
UAVs have become an integral element of data collection in multiple applications. The use of UAVs in agriculture is highly suitable in multiple scenarios. Currently, the use of UAVs is steadily expanding to monitor and assess crops, forests and orchards. They play a major role to manage plant stress such as pests, nutrition deficiencies, disease and water quality and quantity. Several studies have been reported on plant height assessment, canopy cover estimation, vegetation classification, biomass estimation, yield prediction and stress detection [ 111 ]. Every domain has certain aspects which must be kept into account to address the impact of UAV-enabled services. Among these domains, plant stress detection and quantification has received high attention.
Several UAV-based approached are being used to assess plant stress. However, there are several shortcomings which prevent their usage at a high-scale. For instance, the techniques based on thermal imagery are limited by negative impact from various factors including stomatal conductance patterns, canopy architecture, shadows and soil background. Similarly, methods based on hyperspectral and multispectral images suffer from spatial resolutions, angel of capture, illumination and canopy structure. Moreover, UAV-based red, green, and blue (RGB) images are only feasible in clear visibility scenarios. Although image-based techniques are useful for pesticide applications, irrigation practices, disease prediction and prevention and weather information, but they are not feasible yet, regardless of the deployment techniques and sensing devices. There is a need to develop sophisticated ML and computer vision techniques along with novel sensors for UAVs to overcome aforementioned limitations. At the same time, research fraternity should perform more experiments to study plant physiology and impact of various stresses on biological process. It will be an excellent opportunity to contribute in this domain and find viable solutions.
4.10 UAVs for space exploration
There is an emerging trend to utilize UAVs for planetary exploration from last few years. UAVs offer tremendous potentials to carry our space missions such as study about moon surface and atmosphere. Although several planetary exploration techniques are available to perform these missions including rovers, landers, orbiters, flying balloons, flying spacecraft, probes and telescopes [ 112 ]. However, these techniques are restricted by resolution, limited information and versatility. Therefore, UAVs have recently got focus due to several benefits in such missions. Several government bodies and space agencies including National Aeronautics and Space Administration (NASA) has started using UAVs to other solar bodies. Researchers have focused on solar exploration works through autonomous, semi-autonomous and UAVs. According to Sharma et al. [ 112 ], UAVs have good chances to fly in the atmosphere of Mars. It is due to the potential features of hovering, VTOL and low-speed forward flight. In particular, UAVs offer a wide coverage area as compared to exiting orbiters and rovers. As gravity is less at Mars surface and it has low density, so UAV can achieve an optimal height of 380 m. However, some challenges still exist in terms of efficiency and cost. Thus, there is a need to develop compatible propulsion system, suitable aerodynamic design, and optimal flight trajectories.
4.11 Aquaculture farm monitoring and management
UAVs have the ability to monitor fish farms in aquaculture, particularly on offshore sites. The mobility and affordability of UAVs ensures accessibility to remote areas. The automation and mechanization to monitor farms through UAVs, sensors and artificial intelligence (AI) approaches will support farmers to gather information about farms and interact efficiently. Moreover, due to the extensive advancements in aquaculture industry, UAVs can be used to achieve target results and effectively monitor the expanding farm sites. UAVs can significantly reduce cost and labors in the aquaculture industry, thus providing stability in fish form through minimizing farm deaths. In aquaculture, UAVs can be used to capture real-time images on underwater species, observing fish behavior, remote sensing, site surveillance, fish feeding management, and for assessment of the species [ 113 ].
UAVs offer enormous benefits for monitoring offshore kelp aquaculture farms. Giant kelps are effective aquaculture crops which needs regular monitoring in order to achieve maximum production, and optimizing biomass and nutritional content. For this purpose, a small UAV carrying a lightweight optical sensor can be used to monitor these farms. It can estimate the tissue nitrogen quantity, density and canopy area based on space and time scales, which plays significant role to observe any changes in kelps. UAVs with integrated sensors such as hyperspectral, multispectral and RGB cameras can be used to provide a natural image of kelp forest canopy. Similarly, UAVs can be used to observe and characterize the mobility of the pellets. Through collected information, farmers can attain feeding optimization.
4.12 UAVs in emergency medical services
UAVs have shown great potential in smart cities worldwide. Smart cities have smart healthcare system based on telemetry, implantable medical equipments, and medical drones to quickly deliver first-aid supplies. Currently, UAVs have proven their stature to tackle with COVID-19 pandemic in different countries. However, it is worth noting that the leading organization to tackle with COVID-19 is the national EMS institution along with several parties such as EMS personnel, nurses, and medical doctors. In addition, several policy makers are considering different preventive measure to fight against COVID-19 including wearing surgical masks, avoiding facial-touching, regular hand-washing, city lockdown, high risk area avoidance, social gathering avoidance and implementing health codes [ 114 ]. Policy makers should consider the balance between economy and public safety before introducing any new measures. Currently, UAVs are being used to perform various tasks to prevent COVID-19 such as:
Transport of patients
Public announcements
Crowd surveillance
Spraying disinfection
Mass screening
Crown aerial monitoring
Delivering vaccines and other medical supplies
A very common application of UAVs is to supervise or monitor any specific area. UAV-integrated cameras can assist in real-time surveillance. Thermal cameras can be implemented to trace febrile patients. It helps to quickly implement isolation rules in any certain area in order to reduce the risk of further disease spread. Similarly, on-board loudspeaker can help to deliver official policies and give appropriate instructions in any community [ 115 ]. UAVs can help to deliver daily life items, ensuring reduction in mass contact at crowded markets or other public places. Furthermore, UAVs can support in transportation tasks such as medicament supply deliver and COVID test sampling collecting, ultimately reducing the risk of contact with infectious person and virus spread. UAVs can also play their role in nucleic acid testing, collecting samples and delivering reports. They can also be used to spray disinfection in order to mitigate the viability of the virus on the surface. In 2020, UAVs have been used during the COVID-19 crises to deliver medical supplies in USA, China, Chile etc. Recently, Zipline, working collaboratively with Novant-Health, has been providing COVID-19 vaccines in North Carolina, USA. UAVs have been used to provide personal protective equipment to frontline staff fighting against this pandemic. Different applications of UAVs to cope with COVID-19 are presented in Fig. 14 .
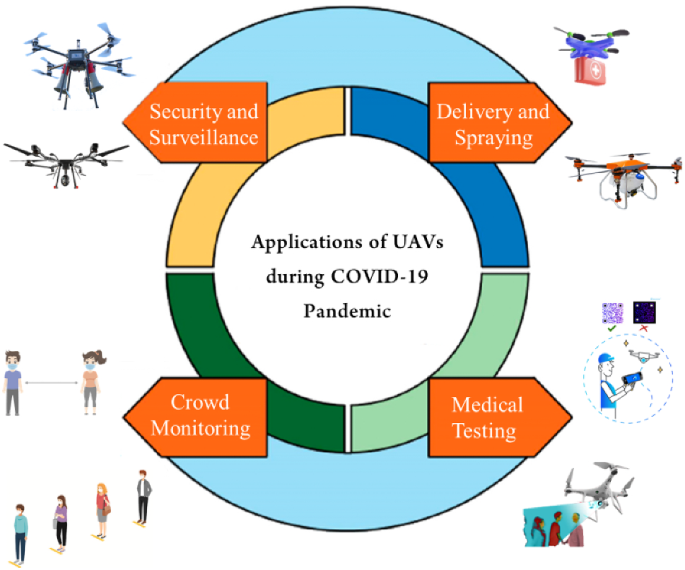
Role of UAVs in COVID-19 pandemic
4.13 UAVs for maritime communication and surveillance
Aerial platform include the use of high-altitude platform Stations (HAPS), flying up to 20 km from the ground and UAVs flying at a few hundred meters above the sea surface [ 116 ]. Some UAVs can be installed and remotely operated from small boats, while larger systems rely on onshore architectures to launch and operate. This technology is complementary to surface and underwater vehicles since it can be easily controlled in the air at some distance and altitude, and thus communicate at a different speed and position. In maritime networks, UAVs can assist to forward the data from ground station to mobile vessel even in the absence of LoS path. In addition, UAVs can relay data from IoT sensors located in ocean and relay data from/to USVs. The main limitation in the operation of UAVs is limited battery capacity and payload. This limitation can be relieved through a tethered UAVs connected with a power supply. However, it can pose another issue of limited mobility. There is a need to find optimal path to collect data from the sensor nodes in maritime environment. Some recent works have been reported on tethered UAVs fixed on buoys [ 117 ]. Tethered UAVs can hover at a limited range above the water surface with limited mobility and coverage. Also, optical fibers can be connected with tethered UAVs to ensure high transmission rates. However, HAPs are more suitable as they offer extended coverage. Moreover, HAPs can carry large antennas, removing less weight limitations and offering autonomy up to several months.
Due to enormous benefits of UAVs in maritime communication, UAV-to-ship wireless channels must be developed. Furthermore, efficient system testing must be conducted for the promising UAV-to-ship networks. In maritime environments, UAV communication faces different channel characteristics, including temperature of troposphere above the ocean, pressure, waveguide impact due to humidity, signal attenuation due to climate change and seawater irregular fluctuations. In contrast to terrestrial UAV-to-ground channels, the scatterers effect on UAV-to-Ship is uneven due to irregular sea waves. Similarly, waveguide propagation is another essential factor in UAV-to-ship channels, which ultimate introduces different channel characteristics. In order to ensure sophisticated UAV communication in such maritime environments, the related channel modeling is an open research area for future contributions. In US, both the coastal guard and Navy have deployed a group of small UAVs to assist manned assets, supporting in many aspects to support law enforcement, surveillance and military missions in marine and coastal environments. Moreover, two US-based systems ScanEagle and MQ-4C Triton are being used for naval missions. The former is deployed with radar as well as infrared (IR) and electro-optical (EO) sensors, making is capable to perform persistent maritime surveillance missions. It has the ability to identify ships through installed AIR receivers.
4.14 Flying cars and eVTOLs
Rapidly expanding high infrastructure costs, land space limitations and urban population put a critical challenge for the future ground-based transportation systems. Flying cars, no more confined to the realm of fiction, are a remarkable step in transportation industry. Flying cars and eVTOL (electric vertical take-off and landing) aircraft is envisaged to revolutionize the future transportation system, which can substantially minimize greenhouse gas emissions and carbon footprint of personal vehicles as well as travel time. Currently, over 250 companies have started initiatives on eVTOLs and flying cars, and few vehicles will pave their way to commercial market soon [ 118 ]. From last few decades, there has been a notable expansion in the use of personal vehicles worldwide, causing traffic congestion, a rapid climate change, and enhanced commuting duration, particularly in metropolitan areas. In this regard, eVTOLs and flying cars are expected to be the future of smart transportation system as they will support reduced emission and traffic congestion along with improving security. These disruptive technologies will have diverse requirements based on their application scenario. For example, personal vehicles should be economical, lightweight, and should contain fewer parking spaces, while piloted taxi services are envisaged to have range and speed according to their Rural Air Mobility (RAM) and Urban Air Mobility (UAM) missions.
Flying car manufacturers are finding innovative designs to shrink the vehicle size through detachable propellers or retractable wings for different tasks such as drive mode or garage parking. Several flight mechanisms and wing configuration are being proposed for the eVTOLs and flying cars to ensure forward flight for the cruise and vertical lift for both take-off and landing. At present, mostly available flying cars have hybrid energy supply system or only gasoline and do not have VTOL capabilities. However, ensuring a complete electric flying car is yet to be explored. In a recent study [ 118 ], authors discussed fully electric powertrain design with dual energy sources, such as hybrid fuel cells and batteries, to support extended range. Moreover, authors also discussed the use of a single propeller for both flight and drive modes in order to aid VTOL capability. Despite these technical advancements, initiating widespread deployment of flying cars will impose an immense standardization challenges and sustainability implications [ 119 ]. Despite these challenges, several companies have started innovative strategies to introduce these smart flying vehicles in future. With expanding features supporting timesaving, environment, and economic potentials, it’s time for regulatory bodies to make the life-changing leap to seed the architecture development for personal urban airspace utility. With these advancements in aviation regulations as well as processing and sensor technologies, we can expect a transition toward futuristic smart and automatic transportation systems.
5 Open challenges
UAVs are prone to several critical challenges and limitations, which require future investigations. Some limitations in UAV usage are presented in Fig. 15 while potential challenges related to UAVs are as follow:
One of the critical challenge is to ensure the security of sensitive data such as position, location etc., from drones or UAVs. As there is no encryption on UAV, so there is risk to be hijacked. Hacking and cyber liability are critical issues of using UAVs. In military operations, UAVs are vulnerable to potential threats of data leakage. Hackers may usurp complete control of UAV to steal data, invasion of privacy and any illegal activity such as for smuggling.
Despite the extensive emergence of UAVs, there is a dire need to devise standardizations from regulatory bodies for the operations of UAVs in geographic area of different countries. A major hindrance in the widespread use of UAVs is the ambiguity or lack of significant standards and regulations for UAV operations, allowed airspace, allowed weight and size, allowed height, privacy or secrecy considerations, safety requirements and characteristics. A lack in heterogeneity of government rules for the implementation of UAVs can be observed. UAVs can affect the navigation of commercial airplanes. So countries should implement regulations and rules for proper operations of UAVs. For instance, in the US, the FAA is responsible to issue certificates and air traffic regulations for UAVs. Similarly, international collaboration or coordination can also assist to introduce global rules, as different countries have different regulations and standards. For example, at present, there are three different regions, such as (a) region 1 contains Africa, Europe and some part of Middle East, (b) region 2 contains US, and (c) region 3 is based on Asia and the Pacific, operating at different frequency ranges for UAV operations. In short, several concerns are associated to UAV regulations, such as poorly documented legal processes and delay in flight approvals limiting the widespread use, availability and flexibility of this technology. In this regard, a viable approach can be AI algorithms for standard compliance, which supports risk mitigation, enhanced security of confidential data, and quick response to new standardization requirements. In this way, we can see AI-empowered UAVs authorized for domestic use in various countries, meeting the operational regulations of each certain geographic location.
Smooth and successful operation of UAVs can be performed using wireless sensors. For instance, smart traffic control system can be attained by monitoring and surveillance from wireless sensors.
Limited transmission range, processing capability and slower speed are also some major concerns in UAVs which need more research contributions and investigations to mature this technology.
Due to UAV path constraints and battery limitations, resource allocation has become a crucial concern. It is noticed in three aspects: UAV hovering, local computing and task offloading. Thus, designing accurate path planning can consequently compromise the operational cost and calculated performance. Efficient resource allocation can improve fairness, task completion time reduction, cost reduction, power consumption reduction and computational efficiency maximization.
The speed of some UAVs is slower than cars and other vehicles on highway. A possible solution to overcome this issue is to allow UAVs to travel at high altitudes by regulatory bodies. In this way, UAVs can get broad field-of-view which can overcome the limitation of speed.
The high speed of some UAVs is also a critical issue. UAVs flying on speed between 35–70 Kmph should involve obstacle avoidance feature to prevent from any possible collision [ 120 ].
Another hurdle for UAV operation performance is power limitation, energy consumption or limited battery life. Usually UAVs are battery-powered and suffer from short battery life, generally below 1 h. UAV batteries are consumed for image analysis, data processing, wireless communication and UAV hovering. Usually UAVs need to travel over large areas and need to return multiple times to charging stations. In SAR operations, UAVs fly for longer time periods over disaster stricken areas. Due to these limitations, a decision should be taken weather UAVs carry out image or data analysis in real-time or not. One possible solution is to form swarms of drones through coordination algorithms which can overcome the limitation of single drone in terms of energy efficiency [ 101 ]. Other interesting approaches are to investigate novel designs for recharging stations and efficient WPT methods such as laser power transfer (LPT). In [ 121 ], energy-aware installation of UAVs with low power and lossy networks (LLT) technique is proposed to overcome these issues.
Complete autonomous and safe operation of swarms of drones is critically important as its prone to human error, machine error and obstacles collisions. Thus, there is a need to swarm intelligence algorithms to avoid collisions. Cooperative formation control algorithms have been proposed to avoid collisions for the multi-UAVs [ 122 ]. These algorithms can fuse data from different deployed sources such as LIDARs, RADARs, gyroscopes, accelerometers, digital cameras, weather and location sensors.
There are several issues in using UAVs for infrastructure and construction monitoring such as limited processing capability, short flight time and limited energy. There is a research gap in multiple UAVs cooperation for infrastructure and construction inspection. Multiple UAVs cooperation can ensure speedy project completion, high error tolerance and broad inspection scope.
Another key challenge is limited payload capability of lightweight UAVs. It limits the ability of UAVs to carry on-board load such as digital, stereo vision and thermal cameras, multiple sensors such as temperature, GPS and gat detection etc. UAVs are required to carry sensors like laser scanner, ultrasonic, RADAR and LADAR which are heavy [ 120 ].
Some cameras used in UAVs are very expensive and low resolution. For example, most of the thermal cameras are of resolution from 640 pixels by 480 pixels and price ranges from 2000 to 5000$. In addition, thermal aerial imaging suffers from several factors including emitted/reflected thermal radiations, shooting distance and atmospheric moisture.
In case of adverse weather conditions such as storm, rain and wind, UAV’s deployment for different applications such as precision agriculture is difficult due to unwanted deviations in predetermined trajectories. Weather conditions also affect operation time, path elevation, UAV altitude and flight direction. In natural disaster conditions e.g., typhoons, hurricanes or Tsunamis, atmospheric condition tends to be a cardinal challenge for UAV missions. In these detrimental conditions, UAVs cannot hover and cannot take accurate readings or data and cannot operate in extreme conditions. Therefore, researchers should address the specifications and UAV capabilities to withstand these adverse weather conditions and can complete weather-sensitive missions efficiently and safely. Specifically, wind speed should be taken into account for smooth UAV operations and it should be involved in UAV’s strategic mission plan and deployment phase.
There should be proper insurance liability due to damages caused by UAVs. Several media reports describe soft tissue injuries, eye loss and severe lacerations due to UAV accidents. In addition to property damages and injuries due to UAV crash, UAV also cause accident with aircrafts, liability doe damaged goods and dropped cargo. Liability for UAV use also contains an enormous threat to individual privacy.
Another major concern of privacy arises with the use of UAVs. UAVs are incorporated with cameras or other equipment which can capture photos or record videos; which may result in violation of individual’s privacy. To tackle this problem in USA, Center of Democracy and Technology (CDT) informed Federal Aviation Administration (FAA) to develop specific regulations to preserve privacy. For this purpose, Privacy by Design (PbD) was introduced which supports compensations for privacy violations [ 123 ]. PbD regulations notably restrict the privacy intrusion. Moreover, consistent UAVs flights can damage the market value of some companies by revealing their trade strategic plans and secrets.
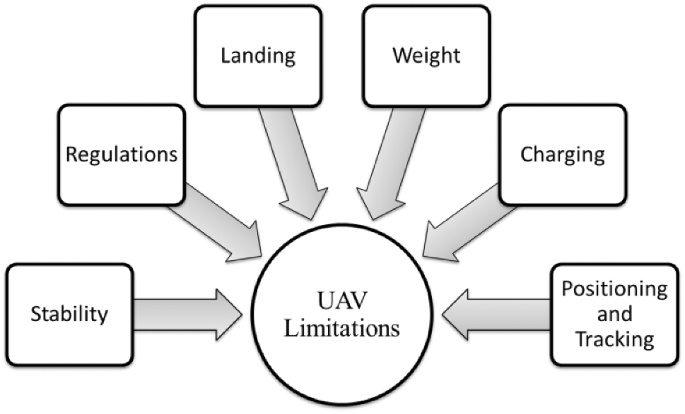
Limitations of current stage of UAV usage
6 Security issues
In this section, we have discussed different cyber security issues on UAVs such as:
UAVs mostly suffer from Hijacking, denial-of-service (DoS) and distributed DoS attacks because of the unavailability of appropriate DoS/DDoS resistant strategies. Signal spoofing trough hijacking can harm the behavior of some UAVs. GPS Signal spoofing attacks occur due to inlaying or injecting wrong information from the GPS channels by the miscreant as illustrated in Fig. 16 . In hijacking, full control of UAV can be stolen by the through inserting extra commands. Similarly, session hijacking can severely damage communication links of UAVs. Moreover, DoS attacks impose severe availability challenges as attacker can cause network congestion by sending multiple requests. DoS attacks occur due to overflow data in communication links to cause interruptions, putting extra load on processing units and by depleting the batteries. In DDos, the attacker overwhelms the UAV by sending traffic from several sources to introduce unreachability issues. These attacks can be mitigated by sensing, tracing signal distortion and high authentication.
Current countermeasure algorithms are available for single UAV networks. Thus, there is a need to develop or modify existing algorithms for multi-UAVs.
According to Shakhatreh et al. [ 101 ], existing simulation test beds are not complete mature. Similarly, available emulators for security analysis are only suitable for specific software and hardware designs. Thus, there is a need to develop customized simulators and tools.
Existing security analyses neglect the software and hardware differences for different types of UAVs. In contrast, some attacks only occur in particular software or hardware design. In contrast, security measures are suitable for any specific UAV and cannot be implemented to different UAV systems. Thus, there is a need to design unified security measure which can be implemented to all UAVs.
Among various security threats, GCS threats are very harmful as sensitive data can be leaked through software tools and malicious operating commands. A compromised GCS receives erroneous commands from attacker. These attacks usually occur due to viruses, key loggers and malwares. Mitigative solutions such as GCS authentications are needed to secure UAV data to be leaked to unauthorized processes, entities and users. Figure 17 illustrates some GCS attacks.
In some scenarios, attacker can misguide UAVs by sending some erroneous warning texts. It can cause network traffic jams. The attacker can masquerade as a legitimate user to give false information, wrong commands or corrupt data to substantially degrade UAV performance. These attacks occur due to the unavailability of authentication strategies as any adversary can pretend like a legitimate user to cause interference in the network.
In some cases, the attackers can steal the UAV path planning information or monitor UAV trajectories for any illegal activity by using this data. These threats can leverage the attacker UAV around the legitimate UAV.
Eavesdropping is another major concern which occurs due to the absence of preventive strategies like data encryption. In such scenario, the adversary can access the legitimate UAV’s data. Non-repudiation can be used to strengthen the security measures [ 124 ]. In such case, specific information is needed for any confirmation to prevent from security breaches.
Another important factor is the integrity of UAV mission which relates with data accuracy and transfer without any interference. If integrity protection strategies are absent, the data becomes invalid as attacker can damage the original data.
In some scenarios, UAV hardware components do not respond accurately and change their target behavior [ 125 ]. Such kinds of attacks are performed to cause UAV mission failure or steal confidential data.
Another critical attack is flight control computer attack which can change mission instructions or parameters to interrupt UAV flight control. On-board software or hardware tools can be used to protect from such attacks. It may contain warning generation, controller estimation or real-time monitoring in order to respond immediately against any possible risk.
In some cases, the attacker misguides UAV to stop following any intended trajectory through intended tampering on onboard navigational devices. Due to these attacks, the UAVs cannot provide accurate position and location to the control system.
It is highly critical to develop advanced mechanisms to combat ever evolving security risks against UAV communication, infrastructure and reliability. Major security concern related to delivery-based UAVs is hijacking, where attacker can steal or damage its load. In case of multimedia-, entertainment- or commercial-based UAVs, disruption its transmission can severely impact the whole network performance. In such scenario, UAV authentication is required, which can cause excessive delays. To cope with different security challenges, ML algorithms are proposed. ML-enabled physical layer security adopted to reduce the impact of malicious UAVs [ 127 ]. Furthermore, anti-jamming strategies can be implemented to enhance physical layer security (PLS) performance against potential jammers. In Table 14 , we have summarized security issues in ML-based UAV networks.
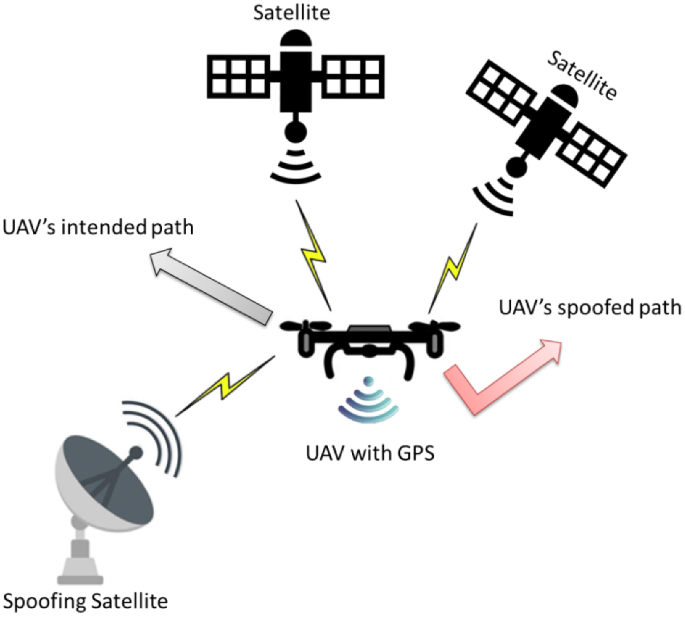
GPS spoofing attack
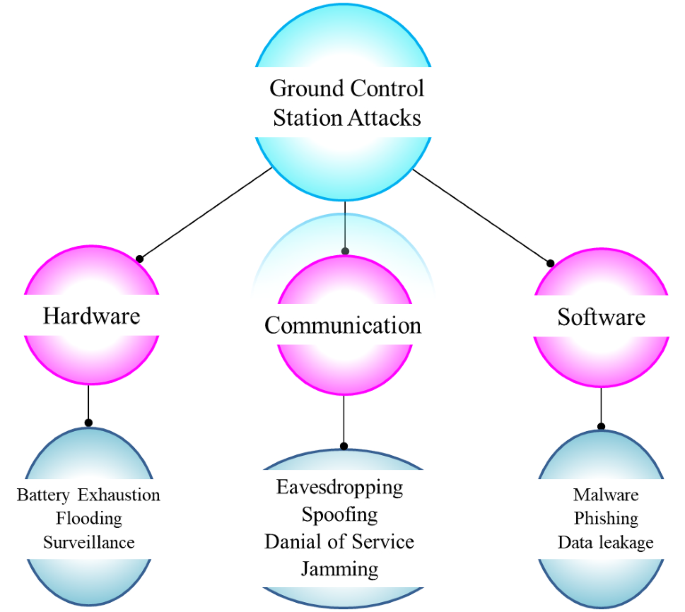
Ground control station (GCS) attacks, motivated by Mansfield et al. [ 126 ]
7 Future research directions
In this section, we present several future research directions for further contributions on UAV research as follows:
Even though AI schemes including machine learning strategies and neural networks have been used in UAVs, but deep learning and reinforcement learning strategies are yet to be implemented fully [ 138 , 139 , 140 , 141 , 142 , 143 ]. It is due to limited power constraints and processing utilities. Hence, researchers should find deep-learning-based novel strategies for UAVs, particularly for SAR mission [ 144 ]. These schemes can support contextual decisions and learning on the basis of trajectory information. The gathered information can be utilized to ensure efficient autonomous piloting of UAVs. As UAVs suffer from both storage and energy limitations, thus, lightweight and portable ML, DL, and RL approaches can be adopted to tackle these limitations. AI/ML-based solution can be implemented in a wide variety of scenarios for UAV communication as shown in Fig. 18 .
Novel strategies for UAV energy harvesting and novel material for UAV batteries must be investigated to achieve extended missions [ 145 ]. Researchers should find new lightweight and efficient batteries to support enhance flight time for UAVs. Future studies must address efficient power control and energy consumption mechanism for UAVs.
Researchers should focus on finding power-efficient algorithms to process UAV’s data e.g., aerial imaging, video and sensing data in real-time. In [ 146 ], authors proposed a convolutional neural network method to assist avalanches search and rescue operation through UAV captured images. Similarly, algorithms for UAVs swarms, swarms optimization [ 147 , 148 ], coordination, collision avoidance and UAVs trajectory plans are also needed.
It is envisaged that with the further development of UAVs with efficient standardizations, privacy rules, image processing algorithms, low-cost sensors, enhanced flight time and larger payload, there is a need to integrate UAVs in diverse applications such as field crop phenotyping [ 149 ].
Imaging processing techniques for UAVs face several critical issues such as varying image orientation, higher overlaps, variable scales and varying altitudes. Research fraternity should address these challenges in future investigations.
There is a need to propose efficient and real-time transmission lines inspection methods through UAVs, such as data analysis tools, cooperative platforms, robust tracking [ 150 ] and detection means, vision-based inspection and UAV low altitude photogrammetry approaches.
Researchers should focus on the implementation of new sensing devices, special cameras, multi decision making algorithms, multispectral imagery, coexistence of edge/fog computing [ 151 , 152 ] or remote sensing and positioning mechanism to support efficient detection of soil, mapping crop status and other farming characteristics [ 153 ].
There is a need to propose more strategies for sensing, guidance, navigation and localization. Any problem in these techniques can cause failure in accuracy and timely delivery of parcels. Thus, researchers should investigate low-cost, efficient sensing devices and localization systems.
Further research contributions should be made toward using UAVs in resilient public safety networks. Future studies should address public safety communications, public health in disaster scenario, blockchain integration in UAVs to enhance health monitoring systems. In a recent study [ 154 ], authors addressed public safety network using UAVs.
Researchers should find adaptive control [ 155 , 156 , 157 , 158 , 159 , 160 ] and cooperative algorithms for multi-UAVs system. In current era, thousands of UAVs in the air form a network to perform various tasks such as drones light show, QR code generation and company’s logo design in air for promotion. There is a need to find robust coordination mechanisms to support such applications.
More research works should be carried out to assess adverse weather effects on UAV robustness to ensure successful mission implementation.
Data filtering techniques should be embedded in UAVs to prevent from redundant data, limit duplicate, illegal access and false locations. UAV can be integrated with emerging intelligent reflecting surfaces (IRS) to enhance PLS of IRS-assisted UAV system in different scenarios while maintaining computational intricacy and system performance [ 161 ].
In future, UAVs may be recharged through different types of energy resources such as fuel cell, solar cell and batteries to prolong flight time and endurance in persistent missions [ 162 , 163 , 164 ]. Considering hybrid power supply feature, it will important to effectively control UAV’s charging characteristics. In addition, UAVs can offload traffic and computation tasks to nearby ground vehicles in order to save power consumption. Thus, collaborative scheduling of hybrid power supply, communication and computation is an interesting research topic for further contributions.
UAV-empowered aerial caching can be adopted to achieve enhanced data throughput in IoT applications. In this context, size of caching contents, users mutual distances and optimizations of UAVs are the primary concerns to ensure feasibility.
There is a need to implement security algorithms such as blockchain in UAVs swarms, means putting more computation ability and storage capacity on UAVs. However, it can reduce UAV flight time and cause latency. Hence, further investigates are required to implement the security algorithms taking the resource-constrained nature of drones into account.
Cooperative path planning is highly significant for intelligent rendezvous generation and energy reduction for UAVs. Due to geographic limitations and highly dynamic network topology, smartly monitoring UAV’s flight in a collision-free and real-time manner has become a crucial issue. To overcome such issues for distributed and intelligent routing scheduling, deep reinforcement algorithms can be used through efficient transfer learning and accelerating the learning speed.
Present studies on UAVs mainly rely on trusted hardware or a third party integration, whose malfunction can severely damage the system. Therefore, there is a need to propose trust-free mechanisms to avoid illegal activity and develop trust for UAVs in an untrusted environment. In this regard, blockchain technology is a viable approach to ensure security. However, a major hurdle is to develop robust and lightweight blockchain system to withstand network fragmentation due to UAV’s constrained resources and high mobility. Moreover, it can cause complex incorporation problem and requires rigorous testing. Thus, a highly devoted architecture integrating features of both UAVs and blockchain technology is needed.
Existing blockchain-assisted UAV applications need a permissioned or private blockchain networks. These networks are critically vulnerable to threats in case of multi-UAVs networks. Moreover, due to increasing number of potential attacks such as game-theory-based attacks, machine learning (ML), and quantum-based attacks, it is essential to secure blockchain. Thus, research efforts are needed to make private blockchain networks safer, secure and immutable.
New set of regulations and policies for UAVs must be introduced and implemented to ensure efficient, reliable, secure and safe operations of the vehicles. Developing new standardizations is one of the major concerns as UAVs must be interoperable with the current technologies. In order to manage EM spectrum and its bandwidth, it is critically essential for UAVs not to be operating in the congested bandwidth and frequency spectrum. It is also crucial to keep the knowledge of published regulatory agreements by NATO for UAVs. This regulation defines the standard data protocols and message formats. It also enables a standard interface between ground coalitions and UAVs. Additionally, it indicates the coalition-shared database which permits data sharing between smart sources. In the US, the Federal Aviation Administration (FAA) provides certification for remote piloting, even for commercial operators. Commercial UAVs should follow the rules set by the FAA for reliable operations. For example, commercial UAVs must have a weight of 55 pounds and their operational range must be below 400 feet above the ground in case of uncontrolled airspace as indicated by Class G. On the other hand, certain authorizations and permissions must be attained to fly in controlled airspace as indicated by Class C, D and E [ 165 ]. UAVs which will be utilized for public operations must have a certificate issued from the FAA, operating body must comply with all regulations, rules, laws and federal of each specific area, city, province, state and country.
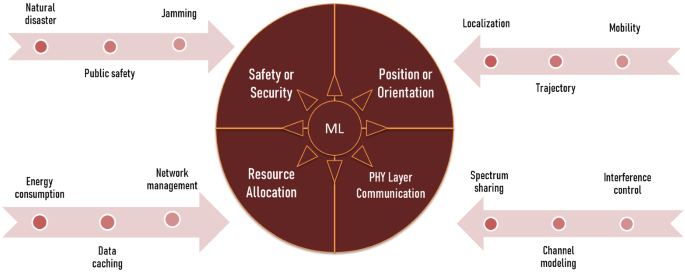
AI/ML-based solutions for UAV communication
8 Conclusion
As UAVs have gained significant research attention, more and more patents and scientific articles are being published. The rapidly expanding research and development of UAVs is a consequence of these innovations. Furthermore, the demand for high mobility, more autonomy and bigger range of UAVs resulted in the design of novel systems for battery swapping, docking stations and precision landing. The application of these is already being used for fire detection and prevention, disaster monitoring, precision agriculture, wireless communication, remote sensing, power-line monitoring, and highway traffic control etc. In this regard, we comprehensively review ongoing developments of UAVs, accomplished by both academia and industrial sectors. We review academic contributions pertinent to UAV types, classifications, standardizations, swarms and charging methods. Furthermore, this study outlines the rapidly expanding interest of researchers, state authorities and business bodies to further harness and utilize the complete features of this promising technology. We briefly discuss UAV characteristics such as payload, altitude, range, speed, and flight time. Moreover, application scenarios, potential challenges and security issues are also examined. Finally, future research directions are identified to further hone the research work.
Grlj CG, Krznar N, Pranjić M (2022) A decade of UAV docking stations: a brief overview of mobile and fixed landing platforms. Drones 6(1):17
Article Google Scholar
Rovira-Sugranes A, Razi A, Afghah F, Chakareski J (2022) A review of AI-enabled routing protocols for UAV networks: trends, challenges, and future outlook. Ad Hoc Netw 130:102790
Noor F, Khan MA, Al-Zahrani A, Ullah I, Al-Dhlan KA (2020) A review on communications perspective of flying ad-hoc networks: key enabling wireless technologies, applications, challenges and open research topics. Drones 4(4):65
Dronova I, Kislik C, Dinh Z, Kelly M (2021) A review of unoccupied aerial vehicle use in wetland applications: emerging opportunities in approach, technology, and data. Drones 5(2):45
Kim J, Kim S, Jeong J, Kim H, Park JS, Kim T (2018) CBDN: cloud-based drone navigation for efficient battery charging in drone networks. IEEE Trans Intell Transp Syst 20(11):4174–4191
Nourmohammadi A, Jafari M, Zander TO (2018) A survey on unmanned aerial vehicle remote control using brain–computer interface. IEEE Trans Hum-Mach Syst 48(4):337–348
Kanellakis C, Nikolakopoulos G (2017) Survey on computer vision for UAVs: current developments and trends. J Intell Rob Syst 87(1):141–168
Zhang S, Qian Z, Wu J, Kong F, Lu S (2016) Optimizing itinerary selection and charging association for mobile chargers. IEEE Trans Mob Comput 16(10):2833–2846
Aldhaher S, Mitcheson PD, Arteaga JM, Kkelis G, Yates DC (2017) Light-weight wireless power transfer for mid-air charging of drones. In: 2017 11th European conference on antennas and propagation (EUCAP). IEEE, pp 336–340
Lu M, Bagheri M, James AP, Phung T (2018) Wireless charging techniques for UAVs: a review, reconceptualization, and extension. IEEE Access 6:29865–29884
Raciti A, Rizzo SA, Susinni G (2018) Drone charging stations over the buildings based on a wireless power transfer system. In: 2018 IEEE/IAS 54th industrial and commercial power systems technical conference (I&CPS). IEEE, pp 1–6
Rohan A, Rabah M, Talha M, Kim SH (2018) Development of intelligent drone battery charging system based on wireless power transmission using hill climbing algorithm. Appl Syst Innov 1(4):44
Shin M, Kim J, Levorato M (2019) Auction-based charging scheduling with deep learning framework for multi-drone networks. IEEE Trans Veh Technol 68(5):4235–4248
Pham H, Smolka SA, Stoller SD, Phan D, Yang J (2015) A survey on unmanned aerial vehicle collision avoidance systems. arXiv preprint arXiv:1508.07723
Hayat S, Yanmaz E, Muzaffar R (2016) Survey on unmanned aerial vehicle networks for civil applications: a communications viewpoint. IEEE Commun Surv Tutor 18(4):2624–2661
Ebeid E, Skriver M, Jin J (2017) A survey on open-source flight control platforms of unmanned aerial vehicle. In: 2017 euromicro conference on digital system design (DSD). IEEE, pp 396–402
Geraci G, Garcia-Rodriguez A, Giordano LG, López-Pérez D, Björnson E (2018) Understanding UAV cellular communications: from existing networks to massive MIMO. IEEE Access 6:67853–67865
Fotouhi A, Qiang H, Ding M, Hassan M, Giordano LG, Garcia-Rodriguez A, Yuan J (2019) Survey on UAV cellular communications: practical aspects, standardization advancements, regulation, and security challenges. IEEE Commun Surv Tutor 21(4):3417–3442
Mozaffari M, Saad W, Bennis M, Nam YH, Debbah M (2019) A tutorial on UAVs for wireless networks: applications, challenges, and open problems. IEEE Commun Surv Tutor 21(3):2334–2360
Li B, Fei Z, Zhang Y (2018) UAV communications for 5G and beyond: recent advances and future trends. IEEE Internet Things J 6(2):2241–2263
Zhang L, Zhao H, Hou S, Zhao Z, Xu H, Wu X et al (2019) A survey on 5G millimeter wave communications for UAV-assisted wireless networks. IEEE Access 7:117460–117504
Ullah Z, Al-Turjman F, Mostarda L (2020) Cognition in UAV-aided 5G and beyond communications: a survey. IEEE Trans Cogn Commun Netw 6(3):872–891
Oubbati OS, Atiquzzaman M, Ahanger TA, Ibrahim A (2020) Softwarization of UAV networks: a survey of applications and future trends. IEEE Access 8:98073–98125
Zhi Y, Fu Z, Sun X, Yu J (2020) Security and privacy issues of UAV: a survey. Mob Netw Appl 25(1):95–101
Skorobogatov G, Barrado C, Salamí E (2020) Multiple UAV systems: a survey. Unmanned Syst 8(02):149–169
Jiang X, Sheng M, Zhao N, Xing C, Lu W, Wang X (2021) Green UAV communications for 6G: a survey. Chin J Aeronaut
Song Q, Zeng Y, Xu J, Jin S (2021) A survey of prototype and experiment for UAV communications. Sci China Inf Sci 64(4):1–21
Srivastava S, Narayan S, Mittal S (2021) A survey of deep learning techniques for vehicle detection from UAV images. J Syst Arch 117:102152
Haider SK, Nauman A, Jamshed MA, Jiang A, Batool S, Kim SW (2022) Internet of drones: routing algorithms. Tech Chall Math 10(9):1488
Google Scholar
Poudel S, Moh S (2022) Task assignment algorithms for unmanned aerial vehicle networks: a comprehensive survey. Veh Commun 100469
Tahir A, Böling J, Haghbayan MH, Toivonen HT, Plosila J (2019) Swarms of unmanned aerial vehicles—a survey. J Ind Inf Integr 16:100106
Mairaj A, Baba AI, Javaid AY (2019) Application specific drone simulators: recent advances and challenges. Simul Model Pract Theory 94:100–117
kalpa Gunarathna J, Munasinghe R (2018) Development of a quad-rotor fixed-wing hybrid unmanned aerial vehicle. In: 2018 Moratuwa engineering research conference (MERCon). IEEE, pp 72–77
Sheng WEN, Jie HAN, Yubin LAN, Xuanchun YIN, Yuhua LU (2018) Influence of wing tip vortex on drift of single rotor plant protection unmanned aerial vehicle. Nongye Jixie Xuebao/Trans Chin Soc Agric Mach 49(8)
Lee D, Zhou J, Lin WT (2015) Autonomous battery swapping system for quadcopter. In: 2015 international conference on unmanned aircraft systems (ICUAS). IEEE, pp 118–124
de Souza BJO, Endler M (2015) Coordinating movement within swarms of UAVs through mobile networks. In: 2015 IEEE international conference on pervasive computing and communication workshops (PerCom Workshops). IEEE, pp 154–159
Pestana J, Sanchez-Lopez JL, de la Puente P, Carrio A, Campoy P (2014) A vision-based quadrotor swarm for the participation in the 2013 international micro air vehicle competition. In: 2014 international conference on unmanned aircraft systems (ICUAS). IEEE, pp 617–622
Fotouhi A, Ding M, Hassan M (2017) Understanding autonomous drone maneuverability for internet of things applications. In: 2017 IEEE 18th international symposium on a world of wireless, mobile and multimedia networks (WoWMoM). IEEE, pp 1–6
Al-Hourani A, Gomez K (2017) Modeling cellular-to-UAV path-loss for suburban environments. IEEE Wirel Commun Lett 7(1):82–85
Saggiani G, Persiani F, Ceruti A, Tortora P, Troiani E, Giuletti F, et al. (2007) A UAV system for observing volcanoes and natural hazards. In: AGU fall meeting abstracts, vol 2007, pp GC11B-05
Berman ES, Fladeland M, Liem J, Kolyer R, Gupta M (2012) Greenhouse gas analyzer for measurements of carbon dioxide, methane, and water vapor aboard an unmanned aerial vehicle. Sens Actuators, B Chem 169:128–135
Khan A, Schaefer D, Tao L, Miller DJ, Sun K, Zondlo MA et al (2012) Low power greenhouse gas sensors for unmanned aerial vehicles. Remote Sens 4(5):1355–1368
Watai T, Machida T, Ishizaki N, Inoue G (2006) A lightweight observation system for atmospheric carbon dioxide concentration using a small unmanned aerial vehicle. J Atmos Ocean Tech 23(5):700–710
McGonigle AJS, Aiuppa A, Giudice G, Tamburello G, Hodson AJ, Gurrieri S (2008) Unmanned aerial vehicle measurements of volcanic carbon dioxide fluxes. Geophys Res Lett 35(6)
Hill AC, Laugier EJ, Casana J (2020) Archaeological remote sensing using multi-temporal, drone-acquired thermal and Near Infrared (NIR) Imagery: a case study at the Enfield Shaker Village. New Hamps Remote Sens 12(4):690
Miyoshi GT, Arruda MDS, Osco LP, Marcato Junior J, Gonçalves DN, Imai NN et al (2020) A novel deep learning method to identify single tree species in UAV-based hyperspectral images. Remote Sens 12(8):1294
Lin YC, Cheng YT, Zhou T, Ravi R, Hasheminasab SM, Flatt JE et al (2019) Evaluation of UAV LiDAR for mapping coastal environments. Remote Sens 11(24):2893
Liu Z, Zhang Y, Yu X, Yuan C (2016) Unmanned surface vehicles: an overview of developments and challenges. Annu Rev Control 41:71–93
Ding M, Wang P, López-Pérez D, Mao G, Lin Z (2015) Performance impact of LoS and NLoS transmissions in dense cellular networks. IEEE Trans Wirel Commun 15(3):2365–2380
Hempe D (2006) Unmanned aircraft systems in the United States. In: US/Europe international safety conference
EASA UAS Workshop [Online]. https://www.easa.europa.eu/sites/default/files/dfu/ws_prod-g-doc-Events-2008-February-1-Overview-of-the-UAV-Industry-(UVS).pdf
ArcturusUAV. Jump 20 [Online]. https://arcturus-uav.com/product/jump-20
AlphaUnmmanedSystems. Alpha 800 UAV Helicopter [Online]. https://alphaunmannedsystems.com/alpha-800-uav/
DJI. DJI Agras MG-1P Series [Online]. https://www.dji.com/mg-1p/infor#specs
AgEagle Aeriel Systems Inc. AgEagle RX-60 Taking Agriculture Intelligence to the Next Level [Online]. https://docs.wixstatic.com/ugd/89e3c5_e3de865b41b644fbb68adea13706723c.pdf?index=true
Technical Specification Group Radio Access Network (2017) Study on Enhanced LTE Support for Aerial Vehicles (Release 15), 3GPP Standard TS 36.777
Use cases and spectrum considerations for UAS (unmanned aircraft systems) (2018). ETSI, Sophia Antipolis, France, Rep. 103 373
Functional architecture for unmanned aerial vehicles and unmanned aerial vehicle controllers using IMT-2020 networks (2017). ITU-T, Geneva
Lieb J, Volkert A (2020) Unmanned aircraft systems traffic management: a comparsion on the FAA UTM and the European CORUS ConOps based on U-space. In: 2020 AIAA/IEEE 39th digital avionics systems conference (DASC). IEEE, pp 1–6
Dronehub. Autonomous drones-in-a-Box. https://dronehub.ai
HIVE. Autonomous Drone Port. https://hive.aero
Skycharge. SKYPORT DP5 drone box hangar. https://www.skycharge.de/drone-box-hangar
Percepto. Percepto Base. https://percepto.co/air-mobile/
Security, N. https://www.nightingalesecurity.com/specs-faqs/
Campi T, Cruciani S, Feliziani M (2018) Wireless power transfer technology applied to an autonomous electric UAV with a small secondary coil. Energies 11(2):352
Wireless power transmission: patent landscape analysis [Online]. https://www.wipo.int/edocs/plrdocs/en/lexinnova_plr_wireless_power.pdf
AirMed&Rescue [Online]. https://www.airmedandrescue.com
Drone Market Report (2024) Drone Ind. Insights UG, Germany
Xu J, Zeng Y, Zhang R (2018) UAV-enabled wireless power transfer: trajectory design and energy optimization. IEEE Trans Wireless Commun 17(8):5092–5106
Hu Y, Yuan X, Jie Xu, Schmeink A (2019) Optimal 1D trajectory design for UAV-enabled multiuser wireless power transfer. IEEE Trans Commun 67(8):5674–5688
Song C, Kim H, Kim Y, Kim D, Jeong S, Cho Y et al (2018) EMI reduction methods in wireless power transfer system for drone electrical charger using tightly coupled three-phase resonant magnetic field. IEEE Trans Ind Electron 65(9):6839–6849
Jawad AM, Jawad HM, Nordin R, Gharghan SK, Abdullah NF, Abu-Alshaeer MJ (2019) Wireless power transfer with magnetic resonator coupling and sleep/active strategy for a drone charging station in smart agriculture. IEEE Access 7:139839–139851
Li J, Yin F, Wang L, Cui B, Yang D (2019) Electromagnetic induction position sensor applied to anti-misalignment wireless charging for UAVs. IEEE Sens J 20(1):515–524
Liu CH, Piao C, Tang J (2020) Energy-efficient UAV crowdsensing with multiple charging stations by deep learning. In: IEEE INFOCOM 2020-IEEE conference on computer communications. IEEE, pp 199–208
Hassija V, Chamola V, Krishna DNG, Guizani M (2020) A distributed framework for energy trading between UAVs and charging stations for critical applications. IEEE Trans Veh Technol 69(5):5391–5402
Qin C, Li P, Liu J, Liu J (2021) Blockchain-enabled charging scheduling for unmanned vehicles in smart cities. J Internet Technol 22(2):327–337
Zhu K, Yang J, Zhang Y, Nie J, Lim WYB, Zhang H, Xiong Z (2022) Aerial refueling: scheduling wireless energy charging for UAV enabled data collection. IEEE Trans Green Commun Netw
Oubbati OS, Lakas A, Guizani M (2022) Multi-agent deep reinforcement learning for wireless-powered UAV networks. IEEE Internet Things J
Fazelpour F, Vafaeipour M, Rahbari O, Shirmohammadi R (2013) Considerable parameters of using PV cells for solar-powered aircrafts. Renew Sustain Energy Rev 22:81–91
Woźniak W, Jessa M (2021) Selection of solar powered unmanned aerial vehicles for a long range data acquisition chain. Sensors 21(8):2772
Thipyopas C, Sripawadkul V, Warin N (2019) Design and development of a small solar-powered UAV for environmental monitoring application. In: 2019 IEEE Eurasia conference on IOT, communication and engineering (ECICE). IEEE, pp 316–319
Wu J, Wang H, Huang Y, Su Z, Zhang M (2018) Energy management strategy for solar-powered UAV long-endurance target tracking. IEEE Trans Aerosp Electron Syst 55(4):1878–1891
Gao XZ, Hou ZX, Guo Z, Chen XQ (2015) Reviews of methods to extract and store energy for solar-powered aircraft. Renew Sustain Energy Rev 44:96–108
Elkunchwar N, Chandrasekaran S, Iyer V, Fuller SB (2021) Toward battery-free flight: duty cycled recharging of small drones. In: 2021 IEEE/RSJ international conference on intelligent robots and systems (IROS). IEEE, pp 5234–5241
Dhingra D, Chukewad YM, Fuller SB (2020) A device for rapid, automated trimming of insect-sized flying robots. IEEE Robot Autom Lett 5(2):1373–1380
Keennon M, Klingebiel K, Won H (2012) Development of the nano hummingbird: a tailless flapping wing micro air vehicle. In: 50th AIAA aerospace sciences meeting including the new horizons forum and aerospace exposition, p 588
Förster J (2015) System identification of the Crazyflie 2.0 nano quadrocopter. Bachelor's thesis, ETH Zurich
SAS PD (2018) Parrot anafi specsheet
Technologies A (2015) Ascending technologies hummingbird
Technologies A (2015) Payload options & accessories
Systems ACUA (2017) Aerialtronics altura zenith specsheet
Solar Tribune (online). https://solartribune.com/solar-powered-drones/
Achtelik, M. C., Stumpf, J., Gurdan, D., & Doth, K. M. (2011, September). Design of a flexible high performance quadcopter platform breaking the MAV endurance record with laser power beaming. In: 2011 IEEE/RSJ international conference on intelligent robots and systems. IEEE, pp 5166–5172
Ouyang J, Che Y, Xu J, Wu K (2018) Throughput maximization for laser-powered UAV wireless communication systems. In: 2018 IEEE international conference on communications workshops (ICC workshops). IEEE, pp 1–6
Chen Q, Zhang D, Zhu D, Shi Q, Gu J, Ai Y (2015) Design and experiment for realization of laser wireless power transmission for small unmanned aerial vehicles. In: AOPC 2015: advances in laser technology and applications, vol 9671. International Society for Optics and Photonics, p 96710N
Lee S, Lim N, Choi W, Lee Y, Baek J, Park J (2020) Study on battery charging converter for MPPT control of laser wireless power transmission system. Electronics 9(10):1745
Kim Y, Shin HB, Lee WH, Jung SH, Kim CZ, Kim H et al (2019) 1080 nm InGaAs laser power converters grown by MOCVD using InAlGaAs metamorphic buffer layers. Sol Energy Mater Sol Cells 200:109984
Zhang Q, Fang W, Liu Q, Wu J, Xia P, Yang L (2018) Distributed laser charging: a wireless power transfer approach. IEEE Internet Things J 5(5):3853–3864
Jaafar W, Yanikomeroglu H (2020) Dynamics of laser-charged UAVs: a battery perspective. IEEE Internet Things J 8(13):10573–10582
Zhao MM, Shi Q, Zhao MJ (2020) Efficiency maximization for UAV-enabled mobile relaying systems with laser charging. IEEE Trans Wirel Commun 19(5):3257–3272
Article MathSciNet Google Scholar
Shakhatreh H, Sawalmeh AH, Al-Fuqaha A, Dou Z, Almaita E, Khalil I et al (2019) Unmanned aerial vehicles (UAVs): a survey on civil applications and key research challenges. IEEE Access 7:48572–48634
Reinecke M, Prinsloo T (2017) The influence of drone monitoring on crop health and harvest size. In: 2017 1st international conference on next generation computing applications (NextComp). IEEE, pp 5–10
Maes WH, Steppe K (2019) Perspectives for remote sensing with unmanned aerial vehicles in precision agriculture. Trends Plant Sci 24(2):152–164
Menouar H, Guvenc I, Akkaya K, Uluagac AS, Kadri A, Tuncer A (2017) UAV-enabled intelligent transportation systems for the smart city: applications and challenges. IEEE Commun Mag 55(3):22–28
Elloumi M, Dhaou R, Escrig B, Idoudi H, Saidane LA (2018) Monitoring road traffic with a UAV-based system. In: 2018 IEEE wireless communications and networking conference (WCNC). IEEE, pp 1–6
Tiansawat P, Elliott S (2020) Unmanned aerial vehicles for automated forest restoration
De Almeida DRA, Broadbent EN, Ferreira MP, Meli P, Zambrano AMA, Gorgens EB et al (2021) Monitoring restored tropical forest diversity and structure through UAV-borne hyperspectral and lidar fusion. Remote Sens Environ 264:112582
Moura MM, de Oliveira LES, Sanquetta CR, Bastos A, Mohan M, Corte APD (2021) Towards Amazon forest restoration: automatic detection of species from UAV imagery. Remote Sens 13(13):2627
Zhang Y, Yuan X, Li W, Chen S (2017) Automatic power line inspection using UAV images. Remote Sens 9(8):824
Foudeh HA, Luk PCK, Whidborne JF (2021) An advanced unmanned aerial vehicle (UAV) approach via learning-based control for overhead power line monitoring: a comprehensive review. IEEE Access
Barbedo JGA (2019) A review on the use of unmanned aerial vehicles and imaging sensors for monitoring and assessing plant stresses. Drones 3(2):40
Sharma M, Gupta A, Gupta SK, Alsamhi SH, Shvetsov AV (2021) Survey on unmanned aerial vehicle for Mars exploration: deployment use case. Drones 6(1):4
Ubina NA, Cheng SC (2022) A review of unmanned system technologies with its application to aquaculture farm monitoring and management. Drones 6(1):12
Pulsiri N, Vatananan-Thesenvitz R (2021) Drones in emergency medical services: a systematic literature review with bibliometric analysis. Int J Innov Technol Manag 18(04):2097001
Mohsan SAH, Khan MA, Alsharif MH, Elhaty IA, Jahid A (2022) Role of drone technology helping in alleviating the COVID-19 pandemic. Micromachines 13(10):1593
Alqurashi FS, Trichili A, Saeed N, Ooi BS, Alouini MS (2022) Maritime communications: a survey on enabling technologies, opportunities, and challenges. arXiv preprint arXiv:2204.12824
Kourani A, Daher N (2021) Marine locomotion: a tethered UAV-Buoy system with surge velocity control. Robot Auton Syst 145:103858
Swaminathan N, Reddy SRP, Rajashekara K, Haran KS (2022) Flying cars and eVTOLs-technology advancements, powertrain architectures and design. IEEE Trans Transp Electr
Kasliwal A, Furbush NJ, Gawron JH, McBride JR, Wallington TJ, De Kleine RD et al (2019) Role of flying cars in sustainable mobility. Nat Commun 10(1):1–9
Griffiths S, Saunders J, Curtis A, Barber B, McLain T, Beard R (2007) Obstacle and terrain avoidance for miniature aerial vehicles. In: Advances in unmanned aerial vehicles. Springer, Dordrecht, pp 213–244
Winter T, Thubert P, Brandt A, Hui JW, Kelsey R, Levis P et al (2012) RPL: IPv6 routing protocol for low-power and lossy networks. RFC 6550:1–157
Kuriki Y, Namerikawa T (2014) Consensus-based cooperative formation control with collision avoidance for a multi-UAV system. In: 2014 American control conference. IEEE, pp 2077–2082
Vattapparamban E, Güvenç I, Yurekli AI, Akkaya K, Uluağaç S (2016) Drones for smart cities: issues in cybersecurity, privacy, and public safety. In: 2016 international wireless communications and mobile computing conference (IWCMC). IEEE, pp 216–221
He D, Chan S, Guizani M (2016) Communication security of unmanned aerial vehicles. IEEE Wirel Commun 24(4):134–139
Birnbaum Z, Dolgikh A, Skormin V, O'Brien E, Muller D, Stracquodaine C (2015) Unmanned aerial vehicle security using behavioral profiling. In: 2015 international conference on unmanned aircraft systems (ICUAS). IEEE, pp 1310–1319
Mansfield K, Eveleigh T, Holzer TH, Sarkani S (2013) Unmanned aerial vehicle smart device ground control station cyber security threat model In: 2013 IEEE international conference on technologies for homeland security (HST). IEEE, pp 722–728
Bithas PS, Michailidis ET, Nomikos N, Vouyioukas D, Kanatas AG (2019) A survey on machine-learning techniques for UAV-based communications. Sensors 19(23):5170
Yue X, Liu Y, Wang J, Song H, Cao H (2018) Software defined radio and wireless acoustic networking for amateur drone surveillance. IEEE Commun Mag 56(4):90–97
Liao Q, Fischer T, Gao J, Hafeez F, Oechsner C, Knode J (2018) A secure end-to-end cloud computing solution for emergency management with UAVs. In: 2018 IEEE global communications conference (GLOBECOM). IEEE, pp 1–7
Shoufan A, Al-Angari HM, Sheikh MFA, Damiani E (2018) Drone pilot identification by classifying radio-control signals. IEEE Trans Inf Forens Secur 13(10):2439–2447
Zohdi T (2020) The Game of Drones: rapid agent-based machine-learning models for multi-UAV path planning. Comput Mech 65(1):217–228
Article MathSciNet MATH Google Scholar
Min M, Xiao L, Xu D, Huang L, Peng M (2018) Learning-based defense against malicious unmanned aerial vehicles. In: 2018 IEEE 87th vehicular technology conference (VTC Spring). IEEE, pp 1–5
Hoang TM, Nguyen NM, Duong TQ (2019) Detection of eavesdropping attack in UAV-aided wireless systems: unsupervised learning with one-class SVM and k-means clustering. IEEE Wirel Commun Lett 9(2):139–142
Manesh MR, Kenney J, Hu WC, Devabhaktuni VK, Kaabouch N (2019) Detection of GPS spoofing attacks on unmanned aerial systems. In: 2019 16th IEEE annual consumer communications and networking conference (CCNC). IEEE, pp 1–6
Xiao L, Xie C, Min M, Zhuang W (2017) User-centric view of unmanned aerial vehicle transmission against smart attacks. IEEE Trans Veh Technol 67(4):3420–3430
Xiao L, Lu X, Xu D, Tang Y, Wang L, Zhuang W (2018) UAV relay in VANETs against smart jamming with reinforcement learning. IEEE Trans Veh Technol 67(5):4087–4097
Li C, Xu Y, Xia J, Zhao J (2018) Protecting secure communication under UAV smart attack with imperfect channel estimation. IEEE Access 6:76395–76401
Lv Z, Li Y, Feng H, Lv H (2021) Deep learning for security in digital twins of cooperative intelligent transportation systems. IEEE Trans Intell Transp Syst. https://doi.org/10.1109/TITS.2021.3113779
Liu F, Zhang G, Lu J (2020) Multi-source heterogeneous unsupervised domain adaptation via fuzzy-relation neural networks. IEEE Trans Fuzzy Syst. https://doi.org/10.1109/TFUZZ.2020.3018191
Zhang L, Zheng H, Cai G, Zhang Z, Wang X, et al. (2022) Power-frequency oscillation suppression algorithm for AC microgrid with multiple virtual synchronous generators based on fuzzy inference system. IET Renew Power Gener. https://doi.org/10.1049/rpg2.12461
Zhang L, Gao T, Cai G, Hai KL (2022) Research on electric vehicle charging safety warning model based on back propagation neural network optimized by improved gray wolf algorithm. J Energy Storage. https://doi.org/10.1016/j.est.2022.104092
Wu X, Zheng W, Chen X, Zhao Y, Yu T et al (2021) Improving high-impact bug report prediction with combination of interactive machine learning and active learning. Inf Softw Technol 133:106530. https://doi.org/10.1016/j.infsof.2021.106530
Chen P, Pei J, Lu W, Li M (2022) A deep reinforcement learning based method for real-time path planning and dynamic obstacle avoidance. Neurocomputing (Amsterdam) 497:64–75. https://doi.org/10.1016/j.neucom.2022.05.006
Carrio A, Sampedro C, Rodriguez-Ramos A, Campoy P (2017) A review of deep learning methods and applications for unmanned aerial vehicles. J Sensors 2017
Vergouw B, Nagel H, Bondt G, Custers B (2016) Drone technology: types, payloads, applications, frequency spectrum issues and future developments. In: The future of drone use. TMC Asser Press, The Hague, pp 21–45
Bejiga MB, Zeggada A, Nouffidj A, Melgani F (2017) A convolutional neural network approach for assisting avalanche search and rescue operations with UAV imagery. Remote Sens 9(2):100
Cao B, Gu Y, Lv Z, Yang S, Zhao, J., et al. (2021) RFID reader anticollision based on distributed parallel particle swarm optimization. IEEE Internet Things J 8(5):3099–3107. https://doi.org/10.1109/JIOT.2020.3033473
Hu Y, Qing JX, Liu ZH, Conrad ZJ, Cao JN et al (2021) Hovering efficiency optimization of the ducted propeller with weight penalty taken into account. Aerosp Sci Technol. https://doi.org/10.1016/j.ast.2021.106937
Yang G, Liu J, Zhao C, Li Z, Huang Y, Yu H et al (2017) Unmanned aerial vehicle remote sensing for field-based crop phenotyping: current status and perspectives. Front Plant Sci 8:1111
Yan J, Jiao H, Pu W, Shi C, Dai, J., et al. (2022) Radar sensor network resource allocation for fused target tracking: a brief review. Inf Fus 86–87:104–115. https://doi.org/10.1016/j.inffus.2022.06.009
Cao B, Fan S, Zhao J, Tian S, Zheng Z, Yan Y, et al. (2021) Large-scale many-objective deployment optimization of edge servers. IEEE Trans Intell Transp Syst 22(6):3841–3849. https://doi.org/10.1109/TITS.2021.3059455
Cao B, Sun Z, Zhang J, Gu Y (2021) Resource allocation in 5G IoV architecture based on SDN and Fog-cloud computing. IEEE Trans Intell Transp Syst 22(6):3832–3840. https://doi.org/10.1109/TITS.2020.3048844
Mogili UR, Deepak BBVL (2018) Review on application of drone systems in precision agriculture. Procedia Comput Sci 133:502–509
Wan S, Lu J, Fan P, Letaief KB (2017) To smart city: public safety network design for emergency. IEEE Access 6:1451–1460
Cao B, Zhang W, Wang X, Zhao J, Gu Y et al (2021) A memetic algorithm based on two_Arch2 for multi-depot heterogeneous-vehicle capacitated arc routing problem. Swarm Evolut Comput 63:100864. https://doi.org/10.1016/j.swevo.2021.100864
Li D, Ge SS, Lee TH (2021) Simultaneous-arrival-to-origin convergence: sliding-mode control through the norm-normalized sign function. IEEE Trans Autom Control. https://doi.org/10.1109/TAC.2021.3069816
Article MATH Google Scholar
Li D, Yu H, Tee KP, Wu Y, Ge SS et al (2021) On time-synchronized stability and control. IEEE Trans Syst Man Cybern-Syst. https://doi.org/10.1109/TSMC.2021.3050183
Wang J, Tian J, Zhang X, Yang B, Liu S, Yin L, et al. (2022) Control of time delay force feedback teleoperation system with finite time convergence. Front Neurorobot. https://doi.org/10.3389/fnbot.2022.877069
Gong X, Wang L, Mou Y, Wang H, Wei X, Zheng W, et al. (2022) Improved four-channel PBTDPA control strategy using force feedback bilateral teleoperation system. Int J Control 20(3):1002–1017. https://doi.org/10.1007/s12555-021-0096-y
Lu S, Ban Y, Zhang X, Yang B, Liu S, Yin L, Zheng W (2022) Adaptive control of time delay teleoperation system with uncertain dynamics. Front Neurorobot 16:928863. https://doi.org/10.3389/fnbot.2022.928863
Mohsan SAH, Khan MA, Alsharif MH, Uthansakul P, Solyman AA (2022) Intelligent reflecting surfaces assisted UAV communications for massive networks: current trends, challenges, and research directions. Sensors 22(14):5278
Mohsan SAH, Khan MA, Noor F, Ullah I, Alsharif MH (2022) Towards the unmanned aerial vehicles (UAVs): a comprehensive review. Drones 6(6):147
Mohsan SAH, Othman NQH, Khan MA, Amjad H, Żywiołek J (2022) A comprehensive review of micro UAV charging techniques. Micromachines 13(6):977
Khan MA, Kumar N, Mohsan SAH, Khan WU, Nasralla MM, Alsharif MH, et al. (2022) Swarm of UAVs for network management in 6G: a technical review. IEEE Trans Netw Serv Manag
Regulation & Policies [Online]. https://www.faa.gov/regulations_policies
Download references
Acknowledgements
This work is supported by the National Natural Science Foundation of China under grant 62261009, Ministry of Education Key Laboratory of Cognitive Radio and Information Processing (CRKL200106)
Author information
Authors and affiliations.
Optical Communications Laboratory, Ocean College, Zhejiang University, Zheda Road 1, Zhoushan, 316021, Zhejiang, China
Syed Agha Hassnain Mohsan & Yanlong Li
School of Telecommunications Engineering, Xidian University, Xi’an, China
Nawaf Qasem Hamood Othman
Ministry of Education Key Laboratory of Cognitive Radio and Information Processing, Guilin University of Electronic Technology, Guilin, 541004, China
Department of Electrical Engineering, College of Electronics and Information Engineering, Sejong University, Seoul, 05006, Korea
Mohammed H. Alsharif
Department of Electrical Engineering, Hamdard Institute of Engineering & Technology, Islamabad, 44000, Pakistan
Muhammad Asghar Khan
You can also search for this author in PubMed Google Scholar
Corresponding author
Correspondence to Syed Agha Hassnain Mohsan .
Ethics declarations
Conflict of interest.
The authors declare no conflict of interest.
Additional information
Publisher's note.
Springer Nature remains neutral with regard to jurisdictional claims in published maps and institutional affiliations.
Rights and permissions
Springer Nature or its licensor (e.g. a society or other partner) holds exclusive rights to this article under a publishing agreement with the author(s) or other rightsholder(s); author self-archiving of the accepted manuscript version of this article is solely governed by the terms of such publishing agreement and applicable law.
Reprints and permissions

About this article
Mohsan, S.A.H., Othman, N.Q.H., Li, Y. et al. Unmanned aerial vehicles (UAVs): practical aspects, applications, open challenges, security issues, and future trends. Intel Serv Robotics 16 , 109–137 (2023). https://doi.org/10.1007/s11370-022-00452-4
Download citation
Received : 08 February 2022
Accepted : 21 December 2022
Published : 16 January 2023
Issue Date : March 2023
DOI : https://doi.org/10.1007/s11370-022-00452-4
Share this article
Anyone you share the following link with will be able to read this content:
Sorry, a shareable link is not currently available for this article.
Provided by the Springer Nature SharedIt content-sharing initiative
- Path planning
- Applications
- Find a journal
- Publish with us
- Track your research
- Engineering
- Aerospace Engineering
- Unmanned Aerial Vehicles
Unmanned Aerial Vehicle Classification, Applications and Challenges: A Review
- November 2018

- Council of Scientific and Industrial Research (CSIR), New Delhi

- Central Scientific Instruments Organization
- This person is not on ResearchGate, or hasn't claimed this research yet.
Precision wildlife monitoring using unmanned aerial vehicles
- Jarrod C. Hodgson 1 nAff2 ,
- Shane M. Baylis 1 ,
- Rowan Mott 1 ,
- Ashley Herrod 1 &
- Rohan H. Clarke 1
Scientific Reports volume 6 , Article number: 22574 ( 2016 ) Cite this article
31k Accesses
284 Citations
317 Altmetric
Metrics details
- Biological techniques
Unmanned aerial vehicles (UAVs) represent a new frontier in environmental research. Their use has the potential to revolutionise the field if they prove capable of improving data quality or the ease with which data are collected beyond traditional methods. We apply UAV technology to wildlife monitoring in tropical and polar environments and demonstrate that UAV-derived counts of colony nesting birds are an order of magnitude more precise than traditional ground counts. The increased count precision afforded by UAVs, along with their ability to survey hard-to-reach populations and places, will likely drive many wildlife monitoring projects that rely on population counts to transition from traditional methods to UAV technology. Careful consideration will be required to ensure the coherence of historic data sets with new UAV-derived data and we propose a method for determining the number of duplicated (concurrent UAV and ground counts) sampling points needed to achieve data compatibility.
Similar content being viewed by others
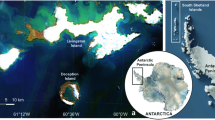
ShetlandsUAVmetry: unmanned aerial vehicle-based photogrammetric dataset for Antarctic environmental research
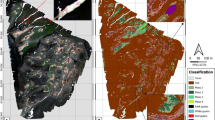
Applications of unmanned aerial vehicles in Antarctic environmental research
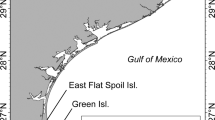
Drone Surveys Do Not Increase Colony-wide Flight Behaviour at Waterbird Nesting Sites, But Sensitivity Varies Among Species
Introduction.
The uptake of UAVs in environmental research has been remarkable. In their short history UAVs have been used in applications as diverse as monitoring breeding success of canopy-nesting birds 1 to surveying elephants 2 . Predictions of ‘big things to come’ for UAV technology are based on the perception that the collection efficiency, cost effectiveness and/or accuracy of data collection using UAVs exceeds that of traditional methods 3 , 4 , 5 , 6 . In wildlife population monitoring applications it is desirable for population counts to be accurate; that is resulting in an estimate that is close to the true population number 7 . In wild populations where the true population size is fundamentally unknown 8 , it is not possible to directly assess the accuracy of any count method. However, it is possible to assess the precision of a count method, defined as the variance between replicated counts by different counters attempting to count the same sample 7 . Regular precise counts facilitate the detection of small magnitude population fluctuations owing to the lower type II error rate in statistical analysis that comes with comparing measures with smaller variance 9 . Reduction in the overall variance exhibited by repeat count data may be obtained by reducing the number of sources of variance in the counting process or by reducing the magnitudes of those variances ( Fig. 1 ). If, as has been predicted, UAV technology results in more precise count estimates than traditional ground-based counting methods then it is likely that many wildlife population monitoring projects will transition from traditional methods to UAV technology for data collection purposes. Transition will require an understanding of how UAV-derived count data compares with that from traditional counting methods so that meaningful comparison can be made between the historical data set and data collected into the future using UAVs. Small UAVs have been used in wildlife monitoring projects to obtain imagery to retrospectively estimate the number of individuals captured in images 10 , 11 . Here, we compare the precision of UAV-derived image counts with counts made concurrently by ground counters for colonies of three seabird taxa – frigatebirds (n = 5), terns (n = 4) and penguins (n = 3) – in tropical and polar environments ( Fig. 2 ). Further, we investigate whether the two count methods return data of comparable magnitude, and propose a method for determining how many times UAV-derived counts and ground counts would need to be carried out in duplicate to ensure compatibility of the two data sets.
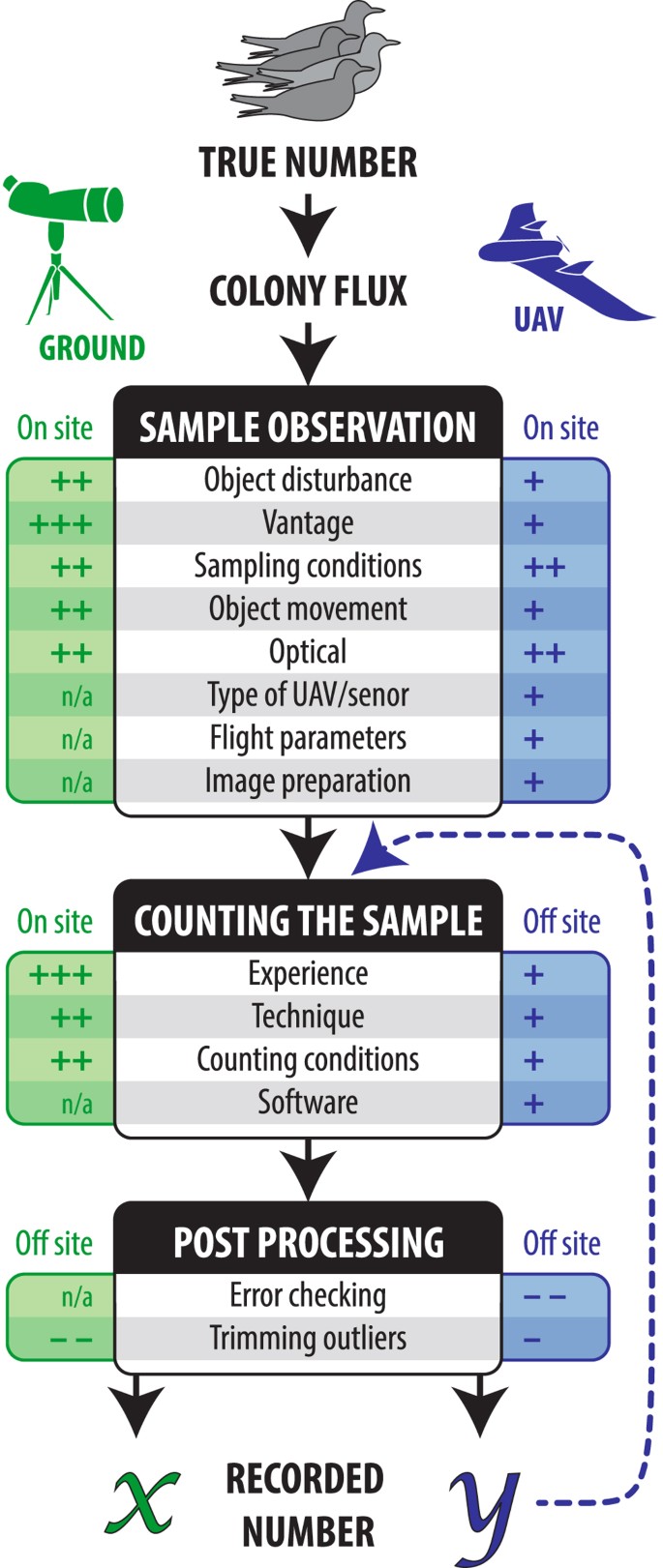
The estimated magnitude of variance for each element for each count type either increases (+) or reduces (−) variance in estimates at a minor (+), moderate (++) or major (+++) scale. We categorised elements into three main stages which occur on or off site: (a) observing the sample, (b) counting the sample and (c) post processing. The hashed blue arrow indicates the ability for UAV-derived counts to be repeated using the existing sample. Figure created by the authors.
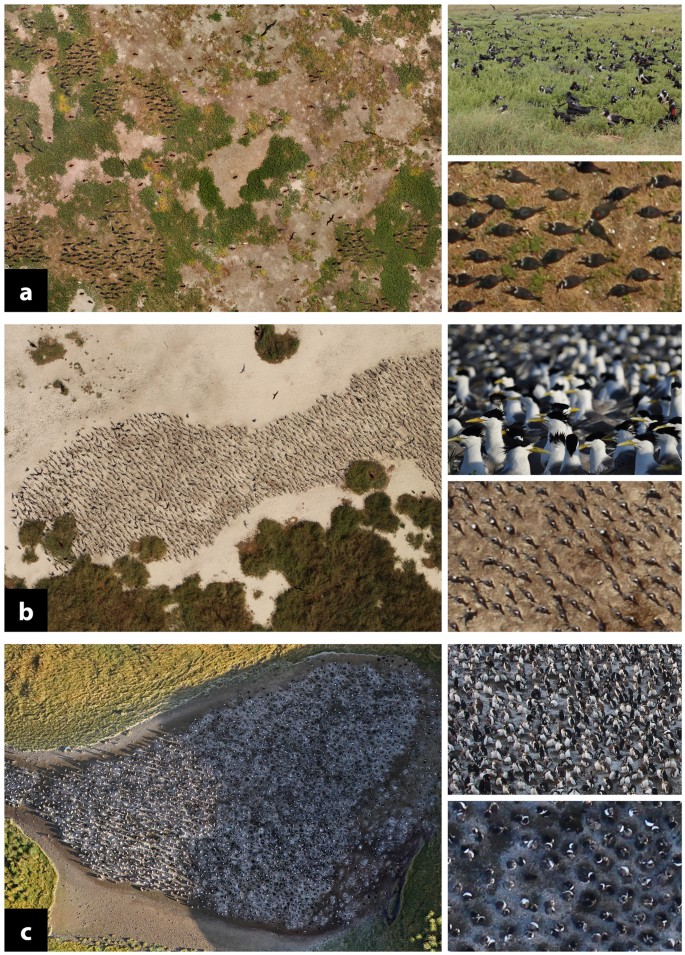
The main image for each species is comprised of several photographs captured by a UAV and merged to make estimates of the number of individuals within the colony. The lower inset of each panel is a magnified view of the main image. Upper insets illustrate the vantage of a ground counter. Images are not to scale and are by Jarrod Hodgson.
UAV-derived counts had significantly lower variance within colonies than ground counts for all species (frigatebirds: F 1,44 = 12.294, p = 0.001, Fig. 3a ; terns: F 1,44 = 19.607, p ≪ 0.001, Fig. 3b ; penguins: F 1,31 = 16.075, p < 0.001, Fig. 3c ). UAV-derived counts were consistently larger than ground counts for both frigatebirds ( Fig. 3a ) and penguins ( Fig. 3c ) in colonies after accounting for variance between colonies (t 40 = −7.257, p ≪ 0.001; t 29 = −0.377, p ≪ 0.001, respectively), however, the magnitude of the difference between ground and UAV-derived counts varied substantially between penguin colonies ( Fig. 3c ). There was no significant difference between ground and UAV-derived counts of terns in colonies (t 41 = 0.694, p = 0.492; Fig. 3b ) although median ground counts were lower than median UAV-derived counts for all colonies.
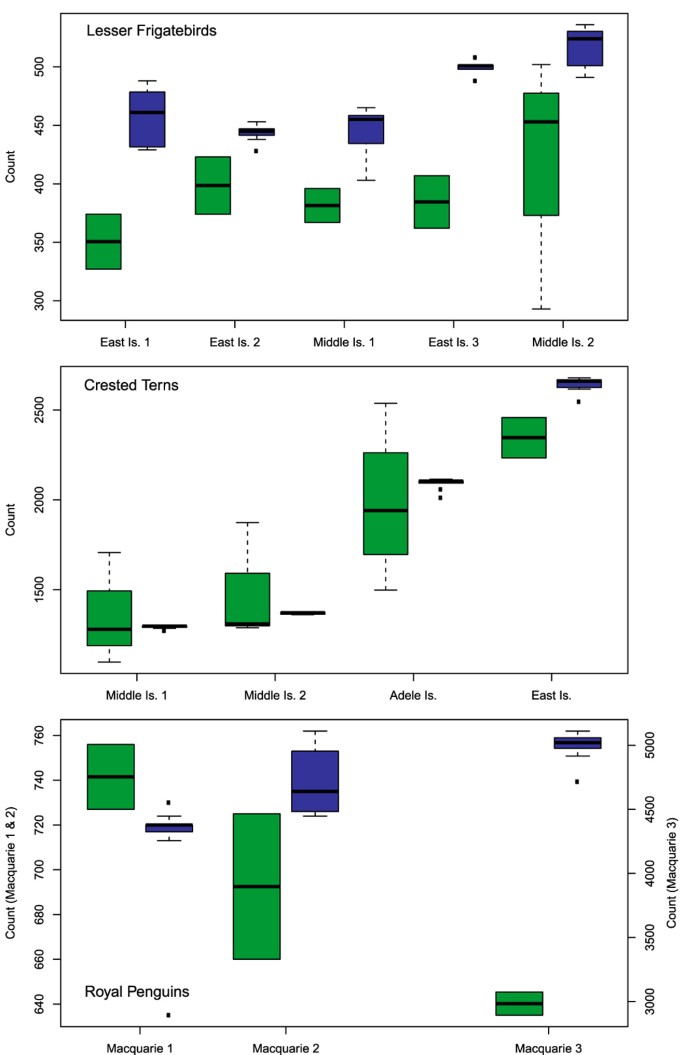
Colonies of varying size were sampled on Australian islands: breeding Lesser Frigatebirds Fregata ariel (a) and Crested Terns Thalasseus bergii (b) at the tropical Ashmore Reef Commonwealth Marine Reserve as well as on the nearby Adele Island in April 2014 and moulting Royal Penguins Eudyptes schlegeli (c) on the subantarctic Macquarie Island in April 2015.
Simulated data matching our observed ratio of ground counts to UAV-derived counts (G:U) and variances of count magnitudes for frigatebirds and terns indicated approximately 15 duplicate counts are required before estimation error below +/− 10% of the true G:U is attained ( Fig. 4 , Table S1 ). For monitoring projects aiming to maintain error at or below +/− 5% during transition from ground counts to UAV-derived counts, 50 duplicate counts were required in the frigatebird simulation whereas approximately 80 duplicate counts were required in the tern simulation. In interpreting these simulations, we stress that they are approximations only, informed by a single field season and are expected to vary by species and with environmental conditions. However, if seasonal or colony-specific variations in G:U are small, then these models will give a good approximation of the number of samples necessary to make interpretations between ground and UAV-derived counts at a desired degree of accuracy.
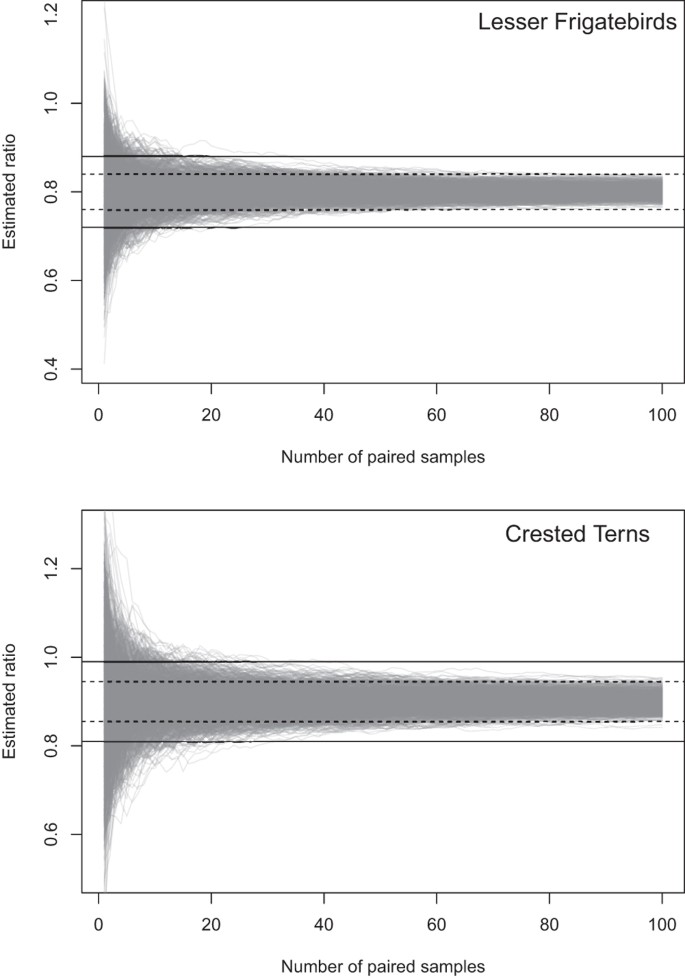
For Lesser Frigatebirds Fregata ariel and Crested Terns Thalasseus bergii , the ground and UAV-derived count means and variances are set at the values provided in Supplementary Table 1 . Each grey line represents the cumulative estimate of the G:U ratio over an increasing number of paired samples. Dashed black lines represent +/−5% of the true ratio, and solid black lines represent +/− 10% of the true ratio.
We demonstrate that UAV-derived estimates of colony size result in smaller cumulative variance compared to conventional ground-based approaches ( Fig. 1 ). While enhanced precision does not guarantee greater estimate accuracy, it does increase the power of our statistical ability to detect population trends 9 . We expect this same benefit to extend to other animal groups and geographic contexts. We also find that UAV-derived counts are consistently similar to or significantly larger than ground counts. The nadir (downward-facing) perspective of UAV imagery reduces the likelihood of missed counts due to topography and birds obscuring the counters’ line of sight. Additionally, still imagery from UAVs presents the option of separating the count area into manageable subsets and completing counts in multiple sittings.
However, the transition from traditional to new UAV-based monitoring methods requires careful consideration, particularly in terms of maintaining the relevance of historical data that have been collected at substantial time and financial cost 12 , 13 . Our simulations indicated that the true ratio of the magnitude of ground count to UAV-derived count (G:U) can be estimated to an accuracy of +/− 10% after completing approximately 15 duplicate counts for frigatebirds and terns. Substantially more counts are required to reduce this error margin to within +/− 5%. However, this simulation was not carried out for penguins as the ratio of ground to UAV-derived counts was not consistent between colonies. Although our data indicate that ground surveys typically resulted in smaller estimates than UAV-derived counts, other studies indicate the converse is possible 14 , 15 . Regardless of the direction of the relationship, if a consistent G:U and a corresponding error metric can be established, as with our terns and frigatebirds, then data continuity is ensured.
This research demonstrates that the precision of population estimates of seabirds in both tropical and polar environments can be improved using UAV technology compared to ground counts. We also present an analytical model to facilitate the transition of monitoring programs to this more precise technique. Our findings suggest that population estimates using UAVs could be a powerful new tool in the ecologist’s tool kit for precision wildlife monitoring.
Study sites and species
Five Lesser Frigatebird Fregata ariel (frigatebird) and two Crested Tern Thalasseus bergii (tern) colonies were sampled at two tropical islands at Ashmore Reef Commonwealth Marine Reserve, Australian (12°16′S 123°2′E), and one tern colony at nearby Adele Island, Western Australia (tropical; 15°31′S 123°9′E). Frigatebirds nest on the ground among herbaceous vegetation at these locations while terns nest in a simple sand scrape in colonies situated on un-vegetated, sandy shorelines.
Three colonies of moulting Royal Penguins Eudyptes schlegeli (penguins) were sampled at subantarctic Macquarie Island, Australia (54°30′S 158°56′E) in April 2015. Sampled colonies were situated on a bare soil substrate. Individuals were at various stages of moult.
Research was conducted in accordance with wildlife research (Parks Australia: 066 ARRR-110217-01; Department of Parks and Wildlife: CE004403, Tasmania Parks and Wildlife Service: RAA 2925) and animal ethics (Monash University Animal Ethics Committee: BSCI/2010/08, BSCI/2012/08 and BSCI/2014/09) permits. Experimental protocols were approved by the Monash University Animal Ethics Committee (see permits details above).
Ground counts
Ground counts were made by experienced seabird counters using standard techniques 16 . Counters used tripod-mounted spotting scopes, binoculars or the naked eye as appropriate. Hand-held two-bank tally counters were used to assist counting. Observation viewpoints were at the same altitude as the sample except one colony (Macquarie 3) which had a 5 m altitude vantage. Counting commenced immediately post UAV recovery. Two to four ground counters each made a single count of the number of individuals within a defined colony. The duration of each count varied (10–30 minutes) depending on colony size. Although there may have been some flux of individual birds over the short period between obtaining aerial imagery and completing ground counts (15–45 minutes), we assumed that the colony numbers were constant given individuals within the sample were either tending a nest (tropical colonies) or moulting (polar colonies).
UAV flight parameters
Tropical colonies were photographed using a small, off-the-shelf octocopter (X8, 3D Robotics – approximate cost: US$1,500). Missions were flown autonomously along a pre-programmed flight path (Mission Planner, planner.ardupilot.com) at a speed of 2–3 m/s and altitude of 75 m above surface level (asl), with launch and recovery greater than 100 m from sampled colonies. Two passes were made over the target colony before returning to the launch site. Total flight time for each colony was 4–12 minutes.
Polar colonies were sampled using a custom flying wing conservation drone (FX79 airframe; in association with HornbillSurveys.com – approximate cost: US$3,000) flown at approximately 13 m/s at 120 m asl. Ground speed was variable relative to weather conditions and pre-programmed, autonomous missions (as per tropical colonies but with manual launch and recovery) were executed. Total flight for each colony was approximately 10–20 minutes.
For all colonies, imagery was captured using a mirrorless digital SLR camera (EOS M, Canon – resolution: 5184 × 3456 px; sensor: CMOS; sensor size: 22.3 × 14.9 mm; approximate cost: US$450) with 40 mm lens (EF, Canon – approximate cost: US$150) and UV filter (Hoya). The manufacturer’s firmware was replaced (MagicLantern, magiclantern.fm) to control the camera’s intervalometer and photograph successively in flight. Photographs were taken at 2–3 second intervals with 1/400–1/1600 second shutter speed, and shot at the highest possible quality in jpeg format. The camera was mounted facing downward and vibration blur was mitigated using a commercial vibration dampening plate for the octocopter and iSPONGE 3 for the flying wing.
Colonies were observed by counters leading up to and during the UAV overflight, as any group startle response which resulted in birds taking flight would obviously affect recorded counts. No group startle response was observed during UAV counts.
Counting aerial images
Unaltered images of a colony were merged in Adobe Photoshop (CS6) to form a composite of the entire colony. Geometric corrections were not considered important and may have distorted the shape of birds in the image. Counts were conducted on a desktop computer. As not all counters were bird specialists, all counters were shown high resolution close up photographs of each species to ensure they could effectively differentiate them from other birds. A grid was overlaid on all files and systematic counts, gridcell-by-gridcell, were then made (left to right, top to bottom) in a laboratory environment using Photoshop’s count tool. Flying birds were ignored. Counters were encouraged to zoom in to each cell as they progressed and, upon completion, review their count at different levels of zoom until they were satisfied they had counted all individuals. Seven to nine people made blind counts of each colony. The time taken to complete each colony count varied (15–90 minutes). Some ground counters were also UAV counters, although not every ground counter counted every drone image, or vice-versa.
Statistical methods
All analyses were carried out in R 3.1.2 17 . Ground and UAV-derived counts were considered linked – i.e., for each flight, ground counters counted the colony immediately after UAV recovery, so analyses treated these as paired samples.
The equality of variances between ground and UAV-derived counts was assessed using a Levene’s test to test for a difference in variance of the residuals from the models for count size between ground and UAV-derived counts. Each species was tested separately.
In order to determine whether ground counts and UAV-derived counts were equal in magnitude, we fitted a mixed-effects Poisson model 18 using the glmmPQL function in the MASS package 19 , with counts modelled as a function of count type (ground or UAV-derived count). Colony identity was included in the model as a random effect. Because an early model showed a significant interaction effect between species and count type on count, each species was modelled separately.
We carried out a simple simulation model to determine how many duplicate counts using both count techniques should be undertaken so that trends are interpretable between the two data collection methods. UAV-derived counts had a higher median than ground counts for all but one of the colonies. This simulation was not carried out for penguins as the ratio of ground to UAV-derived counts was not consistent between colonies. Ground counts of frigatebirds recorded approximately 80% of the individuals in a matched UAV-derived count, whereas ground counts of terns recorded approximately 90% of the individuals in a matched UAV-derived count. As our counts were large (>100 s), we employed a Normal approximation for our simulations. The model simulated paired samples of ground and UAV-derived counts with the underlying ratio of ground counts to UAV-derived counts, and the variance of ground counts and UAV-derived counts, set to reflect the ratios and variances in our data. For each set of samples, we plotted the cumulative ratio of ground counts to UAV-derived counts across increasing numbers of paired samples. For each of 1000 iterations, we simulated 100 paired samples to estimate how many paired samples were necessary in order for the cumulative ratio to reflect the underlying ratio within a given tolerance range of the true ratio with a given probability.
Additional Information
How to cite this article : Hodgson, J. C. et al . Precision wildlife monitoring using unmanned aerial vehicles. Sci. Rep . 6 , 22574; doi: 10.1038/srep22574 (2016).
Weissensteiner, M. H., Poelstra, J. W. & Wolf, J. B. W. Low-budget ready-to-fly unmanned aerial vehicles: an effective tool for evaluating the nesting status of canopy-breeding bird species. J. of Avian Biol. 46 , 425–430, doi: 10.1111/jav.00619 (2015).
Article Google Scholar
Vermeulen, C., Lejeune, P., Lisein, J., Sawadogo, P. & Bouche, P. Unmanned aerial survey of elephants. PLoS One 8 , e54700, doi: 10.1371/journal.pone.0054700 (2013).
Article CAS ADS PubMed PubMed Central Google Scholar
Koh, L. P. & Wich, S. A. Dawn of drone ecology: low-cost autonomous aerial vehicles for conservation. Trop. Conserv. Sci. 5 , 121–132 (2012).
Anderson, K. & Gaston, K. J. Lightweight unmanned aerial vehicles will revolutionize spatial ecology. Front. Ecol. Environ. 11 , 138–146, doi: 10.1890/120150 (2013).
Marris, E. Fly, and bring me data. Nature 498 , 156–158 (2013).
CAS ADS PubMed Google Scholar
Bryson, M., Reid, A., Hung, C., Ramos, F. T. & Sukkarieh, S. Cost-effective mapping using unmanned aerial vehicles in ecology monitoring applications in Experimental Robotics: The 12th International Symposium on Experimental Robotics (eds Khatib, O., Kumar, V. & Sukhatme, G. ) 509–523 (Springer Berlin Heidelberg, 2014).
Chapter Google Scholar
Gregory, R. D., Gibbons, D. W. & Donald, P. F. Bird census and survey techniques in Bird ecology and conservation: a handbook of techniques (eds Sutherland, W. J., Newton, I. & Green, R. ) 1–35 (Oxford University Press, 2004).
Yoccoz, N. G., Nichols, J. D. & Boulinier, T. Monitoring of biological diversity in space and time. Trends Ecol. Evol. 16 , 446–453 (2001).
Gerrodette, T. A power analysis for detecting trends. Ecology 68 , 1364–1372, doi: 10.2307/1939220 (1987).
Chabot, D. & Bird, D. M. Evaluation of an off-the-shelf unmanned aircraft system for surveying flocks of geese. Waterbirds 35 , 170–174, doi: 10.1675/063.035.0119 (2012).
Sarda-Palomera, F. et al. Fine‐scale bird monitoring from light unmanned aircraft systems. Ibis 154 , 177–183 (2012).
Guttman, N. B. & Baker, C. B. Exploratory analysis of the difference between temperature observations recorded by ASOS and conventional methods. Bull. Am. Meteorol. Soc. 77 , 2865–2873 (1996).
Article ADS Google Scholar
Beard, G., Scott, W. & Adamson, J. The value of consistent methodology in long-term environmental monitoring. Environ. Monit. Assess. 54 , 239–258 (1999).
Chabot, D., Craik, S. R. & Bird, D. M. Population census of a large common tern colony with a small unmanned aircraft. PLoS One 10 , e0122588, doi: 10.1371/journal.pone.0122588 (2015).
Article CAS PubMed PubMed Central Google Scholar
van Andel, A. C. et al. Locating chimpanzee nests and identifying fruiting trees with an unmanned aerial vehicle. Am. J. Primatol. 77 , 1122–1134 doi: 10.1002/ajp.22446 (2015).
Article PubMed Google Scholar
Clarke, R., Carter, M., Swann, G. & Thomson, J. The status of breeding seabirds and herons at Ashmore Reef, off the Kimberley coast, Australia. J. R. Soc. West. Aust. 94 , 171–182 (2011).
Google Scholar
R Development Core Team. R: A language and environment for statistical computing. http://www.R-project.org/ (R Foundation for Statistical Computing, Vienna, Austria 2015).
O’Hara, R. B. & Kotze, D. J. Do not log-transform count data. Methods Ecol. Evol. 1 , 118–122, doi: 10.1111/j.2041-210X.2010.00021.x (2010).
Venables, W. N. & Ripley, B. D. Modern Applied Statistics with S. Fourth Edition (Springer, 2002).
Download references
Acknowledgements
This research was funded in part by the Norman Wettenhall Foundation and Macquarie Island access was via the Australian Antarctic Division. We recognise the support of the Tasmanian Department of Primary Industries, Parks, Water and Environment, particularly Rachael Alderman and Kris Carlyon. We appreciate the contribution of counters including George Swann and Amanda Lilleyman, as well as Lian Pin Koh, Keeyen Pang, Kate Lawrence and Andrea Turbett. We thank Brendan Hodgson and an anonymous reviewer for providing comments on an earlier draft of the manuscript.
Author information
Jarrod C. Hodgson
Present address: Present address: School of Biological Sciences, The University of Adelaide, SA 5005, Australia.,
Authors and Affiliations
School of Biological Sciences, Monash University, VIC, 3800, Australia
Jarrod C. Hodgson, Shane M. Baylis, Rowan Mott, Ashley Herrod & Rohan H. Clarke
You can also search for this author in PubMed Google Scholar
Contributions
J.H. designed the study with input from all authors. J.H., S.B., R.M., A.H. and R.H. collected the data. S.B. analysed the data. J.H., S.B. and R.M. wrote the manuscript. All authors discussed results and commented on the manuscript.
Corresponding author
Correspondence to Jarrod C. Hodgson .
Ethics declarations
Competing interests.
The authors declare no competing financial interests.
Supplementary information
Supplementary information (pdf 82 kb), rights and permissions.
This work is licensed under a Creative Commons Attribution 4.0 International License. The images or other third party material in this article are included in the article’s Creative Commons license, unless indicated otherwise in the credit line; if the material is not included under the Creative Commons license, users will need to obtain permission from the license holder to reproduce the material. To view a copy of this license, visit http://creativecommons.org/licenses/by/4.0/
Reprints and permissions
About this article
Cite this article.
Hodgson, J., Baylis, S., Mott, R. et al. Precision wildlife monitoring using unmanned aerial vehicles. Sci Rep 6 , 22574 (2016). https://doi.org/10.1038/srep22574
Download citation
Received : 28 October 2015
Accepted : 17 February 2016
Published : 17 March 2016
DOI : https://doi.org/10.1038/srep22574
Share this article
Anyone you share the following link with will be able to read this content:
Sorry, a shareable link is not currently available for this article.
Provided by the Springer Nature SharedIt content-sharing initiative
This article is cited by
Cues to individuality in greylag goose faces: algorithmic discrimination and behavioral field tests.
- Sonia Kleindorfer
- Benedikt Heger
- Diane Colombelli-Négrel
Journal of Ornithology (2024)
The broad scale impact of climate change on planning aerial wildlife surveys with drone-based thermal cameras
- Annalysa M. Camacho
- Humberto L. Perotto-Baldivieso
- Shad D. Nelson
Scientific Reports (2023)
Fusion of visible and thermal images improves automated detection and classification of animals for drone surveys
- B. Santhana Krishnan
- Landon R. Jones
- Raymond B. Iglay
The first use of a photogrammetry drone to estimate population abundance and predict age structure of threatened Sumatran elephants
- Dede Aulia Rahman
- Riki Herliansyah
- Nyoto Santoso
Integrated Population Models: Achieving Their Potential
- Rachel McCrea
- Elise Zipkin
Journal of Statistical Theory and Practice (2023)
By submitting a comment you agree to abide by our Terms and Community Guidelines . If you find something abusive or that does not comply with our terms or guidelines please flag it as inappropriate.
Quick links
- Explore articles by subject
- Guide to authors
- Editorial policies
Sign up for the Nature Briefing: Anthropocene newsletter — what matters in anthropocene research, free to your inbox weekly.


An official website of the United States government
The .gov means it’s official. Federal government websites often end in .gov or .mil. Before sharing sensitive information, make sure you’re on a federal government site.
The site is secure. The https:// ensures that you are connecting to the official website and that any information you provide is encrypted and transmitted securely.
- Publications
- Account settings
Preview improvements coming to the PMC website in October 2024. Learn More or Try it out now .
- Advanced Search
- Journal List
- Springer Nature - PMC COVID-19 Collection

Recent Advances in Unmanned Aerial Vehicles: A Review
Faiyaz ahmed.
1 Department of Mechanical Engineering, Motilal Nehru National Institute of Technology, Allahabad, India
J. C. Mohanta
Anupam keshari.
2 National Institute of Industrial Engineering, Mumbai, India
Pankaj Singh Yadav
In recent decades, aerial robots especially small UAVs and drones have witnessed tremendous improvements in terms of their structure, working methodology, flying features and navigation control. UAVs are highly utilized in a wide range of services such as photography, path planning, search and rescue, inspection of power lines and civil constructions, etc. This manuscript reports a wide overview and comprehensive survey of recent developments in commercially available UAV’s and gives a brief note on the progress and research covered in last 10 years. The research presents a roadmap to understand the successive development of advanced drones/ UAVs in terms of their geometric structure, flying mechanism, sensing and vision ability, aviation quality, path planning, intelligent behaviour and adoptability. A literature survey is conducted systematically on 254 retrieved articles published in the last 10 years and scaled down to 96 relevant articles. In these shortlisted articles, path planning, neural network, artificial intelligence, inspection, surveillance, tracking and identification, etc. are the most relevant methodologies or applications presented. The current research is concerned about the growth and impact of UAVs/drones in the society and also inspires the newbies to carry research in this field and propose new methods to select or equip the flying robot for a specific application in various fields. This article also assists researchers in understanding and evaluating their research work in the context of existing solutions. It also helps newcomers and pilots/practitioners to quickly gain an overview of the existing vast literature in the related fields.
Introduction
Unmanned Aerial Vehicle (UAV) or pilotless aircraft operates with advanced components including a physical model, Ground Control Station (GCS), modern sensors and a platform for ease of communication between them. In the past, UAVs are used for civilian and military operations such as rescue and search, climate monitoring, surveillance, weather forecasting and mapping. Since the evolution of modern technology and innovations in internet, UAVs has completely changed. Nowadays, UAVs are also used in emergency evacuations during natural disasters like storms, floods and bush fires, etc.
An expected figure of applications of UAVs from 2010 to 2022 in various fields is represented in Fig . 1 . They have better performance in maximum roll angle, turn angle, path length, and flexibility in maneuvering, high scalability, portability and mobility. Apart from this, UAVs are also widely used in civil constructions such as the delivery of materials to an inaccessible location in construction sites and monitoring the constructed buildings for finding damages to achieve the purpose of smart cities. Its usage is also increasing in various domains of real estate management and smart cities. The communication methods have completely evolved from the most advanced platform starting from the first generation (1G) with no data capabilities to the fifth-generation (5G) with end-to-end connectivity and IP networking. 5G presents a cutting-edge future archetype that will ubiquitously and completely link all across the internet as shown in Fig. 2 [ 1 ].

Expected reach of UAVs in various applications

Evolution of communication technology along with a 5G Drone system and possible upcoming technology/applications for drones [ 1 , 95 ]
UAVs are classified based on different parameters such as Size, Weight and Power (SWAP). These parameters refer to maximum flight time, altitude, computational speed and communication. Similarly, High Altitude Platform (HAP) refers to better and broader communication with double payload capacity. There must be a fundamental trade-off between the two altitudes platforms for incorporating storage capacity and maximum coverage area. According to the report of the world’s second-largest professional services enterprise Price water house-Coopers (PwC) and Interact Analysis, estimates that the global market for UAV applications will exceed $20 billion in 2022 [ 2 ]. In the year 2016–17, the United Business Media (UBM) has recorded a 30% growth in UAVs and a hike of 40% in 2017–2018 [ 3 ]. According to the forecast yearly report of the Aerospace and Federal Aviation Administration (FAA), in the United States of America, the purchase of UAVs has reached seven million in 2020 [ 4 ].
Despite the rapid growth of drone development and marketing, there are very limited reviews showing a road map of step-by-step growth in drone’s capabilities distinguishing their types, categories, design and functionality, application, specific developments and major domains of researches [ 5 ]. Such reviews are important inputs to select or equip the flying robots for a specific use, to realize the impact of drones in the upcoming society and to grasp important information of its successive growth and current researches and advancements [ 6 ]. Thus, for summarizing all such researches, a survey is carried-out over top journals and conference research articles published in the last decade. From the survey, it is observed that the types of UAVs are increasing quickly. This manuscript gives a brief overview of all types of aerial robotics including flapping wing, fixed-wing, spin copter, cyclo-copter, ornithopter, tri-copters, quad-copters, hexa-copters, and octa-copters, etc. along with the structural models of aerial robotics, navigation control and application aspects are also presented comprehensively. In line with the trend of drones, the use of small-scale rotorcraft robotic systems (SRURS) is also in its developing phase [ 7 ]. These SRURS are generally weighed less than 25 kg or up to 10 m in dimension and are widely used for the applications of surveillance of environment, photography, the response of nuclear disaster, monitoring of power lines and survey of agriculture, etc. [ 8 ]. The research in aerial manipulation also attracted researchers for its wide applications in inspections of bridges and power transmission lines, tool operations, transporting of objects, wall climbing, delivery of goods, etc. [ 9 – 11 ].
Definition of UAV/Drone
The abbreviation of DRONE is Dynamic Remotely Operated Navigation Equipment. A UAV is also defined as a drone without any onboard pilot and operates autonomously or with the help of a flight controller operated from the ground station [ 12 ]. The degree of freedom in UAV is varied for executing the special functions like stabilization and altitude lock, Inertial Measurement Unit (IMU), Global Navigation Satellite System (GNSS) is used for locking the position in mid-air. These additional components and sensors will make the UAV more autonomous and succeed in missions like path planning, obstacle avoidance, and autonomous take-off and landing. From the literature, it is noted that UAVs are named or presented with different names like Aerial-rotor, Vertical take-off and landing aircraft, multi-rotor and rotorcraft, etc. These keywords help in finding the research papers related to UAVs.
Types/Categories of UAVs/Drones
UAVs are generally classified by their flying principle because of their aerodynamic structure. UAV’s having heavier mass will depend on propulsive thrust to fly into the air. Further, they are categorized into two types, i.e., ‘rotor’ and ‘wing’ type. Rotor UAV will depend on multiple rotors and propellers attached to them to generate the required amount of thrust to lift upwards. Similarly, UAVs with wing types will depend on their wings to produce the aerodynamic effect for lifting upwards into the air. This is classified further into three sub-categories, i.e., flapping-wing, fixed-wing and flying-wing. The UAV with a light weight like a parachute, balloons and blimps will rely on forces of buoyancy to fly in the air.
Floreano et al. [ 13 ] discussed the different categories of UAV/Drones. Wherein, drones are categorized based on their mass and flight time. UAVs with heavier mass will have the capability to carry heavy payload and can perform autonomous and multiple tasks. Fixed-wing and Rotor-type UAVs are heavier in mass and relatively big in structure. Considering the aerodynamic efficiency, fixed-wing UAVs can have more flight time compared to rotor-type.
The structure of this manuscript is organized as follows: Sect. 1 gives a brief introduction to the current study and evaluation of UAV in various applications. In Sect. 2 the methodology followed to identify research articles in the field of UAVs are briefly explained. Section 3 describes the architecture and different components of UAVs. Section 4 shows an overview of UAVs yearly distribution, and types of UAV/drones with growth and need of autonomy. Finally, Sect. 5 concludes the manuscript with a glimpse of upcoming developments.
Methodology of Survey
This section explains the methodology and steps carried out in the survey and the scope of research related to UAV/drones. To identify relevant articles towards the scope of research, a well-defined process is carried. The overview of published papers with the methods and concepts are explained and compared with the other types of aerial robotics.
The Method Followed to Identify UAV Research Papers
To search high impact papers/research concerning aerial robotics or UAV, many titles and keywords are collected from the top journals and conferences which includes IEEE, Web of Science and Scopus index journals [ 14 ], etc. The collected list of keywords is grouped and referred to as A1, A2, A3, A4 and A5. A1 refers to the articles focussed on UAV with flapping, fixed wing and aerodynamic structure. The aim was to retract the articles with domain-specific. The keywords used for A2 are concentrated on UAV’s regarding cyclo-copter, blimp, glider and kite, etc. The keywords designed for A3 are used for retracting articles on tricopter, quad-copter, hexacopter and octacopter. The keywords in A4 are used for searching articles on multi-copters, UAS, UAV and Radio Control (RC) planes. Keywords linked to A5 are concerned with different components mounted on UAVs, and sensors attached for various applications. The different stages involved in the retrieval of articles with linked keywords are used in different search engines as shown in Fig. 3 .

Retrieval of the articles using keywords
As an output of this phase, highly cited articles published in high impact factor journals are collected from the search engines. The total number of articles retrieved is 254. As shown in Table 1 keywords are entered with their abbreviation and short-form e.g., UAV and its abbreviation (Unmanned Aerial Vehicle), MAV (Micro Aerial Vehicle), etc. These keywords will find the papers related to words like copter, aerial, robotics, quad-rotor/quad-copter and drones. A screening process is followed to filter the articles to match the relevant keyword. A manual step-by-step process is as follows:
- i. In the first step topics presented in posters and workshops are not considered because of the limitations of papers.
- ii. The articles or manuscripts should be published on or after January 2010.
- iii. Papers that have the most relevant information only on topics related to drones, aerial vehicles and quad-copter, etc. with modelling, methodology, fabrication and results are considered.
- iv. Research papers with experimental results of flying and hovering, path planning and surveillance are suggested. (A few papers will not show any experimental results to their method and proposes to implement these methods in UAVs. such papers are not considered.)
- v. In research papers like inspections, communication and image processing, it should be noted that the data of images and datasets of inspection should be collected by the author. Otherwise, such papers will not be useful for justifying the experimental results shown in the paper.
- vi. In the selection of a review paper, it is mostly observed that most of the authors will include methods that are irrelevant and cannot be implemented in UAVs or drones. Such papers are also not considered. Review papers with references or methods of the past five years are considered to be useful.
- vii. Modelling and analysis research papers are considered only if the author showed valid results. Otherwise, such papers are also not proposed to be useful.
- viii. All manuscripts must be in the English language.
- ix. Unique research work articles should be considered leaving duplicate ones.

Methodology of literature survey
List of keywords collected from the research papers of top journals/conferences
Sl. No | Keywords | ||
---|---|---|---|
1 | Unmanned Aerial Vehicle (UAV) | 12 | Aerial Manipulating |
2 | Micro Aerial Vehicle (MAV) | 13 | Modelling and control |
3 | Dynamic Remotely Operated Navigation Equipment (DRONE) | 14 | Flapping |
4 | Small-Scale Rotorcraft Robotic Systems (SRURS) | 15 | Rotor craft |
5 | Vertical Take-off and Landing (VTOL) | 16 | Fixed-wing |
6 | Unmanned Aerial Systems (UAS) | 17 | Gliding |
7 | Quad-copter | 18 | Flying |
8 | Surveillance and monitoring with UAS | 19 | Dragonfly |
9 | UAS path planning for disaster management and relief | 20 | Copter |
10 | UAS communication networks | 21 | Multi-copter |
11 | UAS path planning and trajectory planning | 22 | Propellers |
As discussed in the survey methodology, at first google trends are used to find the search string used in this manuscript. The different keywords used in the trend search are UAV path planning, UAV communication, UAV inspection, UAV surveillance and UAV industry as shown in Fig. 5 , and the growth rate in commercial market size of UAVs from 2010 to present and forecasted up to 2025 in terms of commercial drone sales and revenue generation worldwide is shown in Fig. 6 . From the figure, it can be observed that there is a spike in UAV communication from 2012 and 2017 onwards. UAV inspection has attained numerous growths since 2010 as it is widely implemented in the inspection of various applications such as power lines, solar panels, civil bridges and windmills, etc. UAVs especially used for surveillance is declining due to the non-availability of long-lasting power. UAV industries are also growing and maintaining consistency from the beginning of a search.

Analysis of keywords searched in google trends since 2010

Projected worldwide market growth for commercial drones [ 96 ]
Topics Covered on UAV
Liew et al. [ 15 ] conducted a vast survey on types and categories of UAVs. The information regarding the evolution of UAV is summarized in a pie chart as shown in Fig. 7 . It is observed from the pie chart that the number of research papers published is on the topics of hardware development (31%) and control and modelling (28%) as it covers more than 50% of the pie chart. But gradually the growth is observed in task planning, mapping and inspection as these are the emerging topics in the present era. Beyond this, the research papers also published on navigation, GPS location and collision avoidance. This is the most covered topic after 2016 in the top journals and conferences.

Research on evolving of UAV [ 15 ]
Components of UAV and its Architecture
In this section components of UAV and architecture are explained in brief along with their function and applications.
Drone/Quad-Copter
Drones are also categorized depending on their structure and weight, i.e. Macro aerial Vehicle, Micro Aerial Vehicle (MAV) and Nano Aerial Vehicle (NAV). Kumar et al. [ 16 ] carried research on quad-copters and discussed their flight time challenges.
In addition, the main quad-copter is equipped with different components for high-level functioning in different applications such as path and trajectory planning, inspection and evaluation, etc. The structure is mainly divided into data collection, data processing and data actuation or evaluation. The data collection system handles the functioning of onboard sensors, cameras, battery and smart devices. The data collected by these devices is processed by the central core unit of UAV for defined tasks like path planning, surveillance, inspection and detection, localization, etc.
Figure 8 depicts the area covered by components and sensors of UAV. These hardware onboard components cover the area of different applications such as path planning, collision avoidance and inspection, etc. during the hovering of UAV. LIDAR and infrared devices are mainly used for collision avoidance and mapping, whereas camera and GPS are used for surveillance of particular area or path of the UAV in front and rear direction. The central core system actuates the UAV for real-world environment applications as shown in Fig. 9 . UAVs are equipped with various components such as Electronic Speed Controller (ESC), Camera & Gimbal, Flight controller and Lithium-ion battery (LiPo), etc. as explained below.

Components of UAV

Functional components of UAV
Electronic Speed Controller
ESC is mainly used to offer power and high frequency to the UAV motors. The main function of ESC’s is it converts Direct Current (DC) to 3-phase Alternate Current (AC) and is also used for alternating the motors speed.
In UAVs, Lithium-ion Polymer batteries (LiPo) are used for powering the onboard components and sensors. Drone pilots always prefer to carry an extra battery in case of an emergency during the flight.
Flight and Radio Controllers (FC and RC)
The flight controller plays a key role in UAV during hovering in the mid-air. Improper calibration of flight controllers will lead to crashes or non-stability in the flight of the drone. A highly configured radio controller with switchable sticks will lead to maintaining connectivity for long-range and to attain vertical take-off landing. Lim et. al. [ 17 ] discussed the importance of flight controllers in quad-copters and carried a survey of FC’s available in the market. According to their survey, the open-source FC’s are KK 2.1.5, Arducopter, Aeroquad, Pixhawk, Multiwii, Arduino and Raspberry pi. Apart from the FC’s their survey also provides information about the structure and cost estimation of open-source FC’s. Development in FC’s has gradually increased over the past five years in terms of programming and operation. Pixhawk recently has released a more stable FC into the market known as Pixhawk-cube for Quad, Hexa and Octa-copters. For more information and survey regarding FC & RC for multiple-copters, researchers are suggested to go through this given reference for knowledge on the selection of components and controllers [ 18 , 19 ].
Camera and Gimbal
Wood et al. [ 20 ] discussed the steps involved in building a quad-copter with the different components and also gave a brief note on the various applications of quad-copter. The developed model from their study is used for the application of surveillance and hovering at a particular position. Their copter was much useful in societal applications like surveillance of traffic updates and crowded areas. Fabrication, control, actuation and battery capacity of flapping wing UAV’s and their applications are explained in the present field. Thermal cameras are also used in UAS for the inspection of solar panels or power transmission lines. These cameras are also used in agriculture for the detection of disease and the breeding of plants. Gimbal is used to rotate the camera in x, y and z directions without any disturbance in the tilt of the camera. It provides the stabilization for capturing or recording high-definition images and videos.
Besides the frame or the structure, many other system parts in a drone are gradually being improved, i.e., GPS/GNSS (Global Positioning System/Global Navigation Satellite System), motors to rotate propellers, ESC (Electronic Speed Controller); battery and its charging systems, onboard controller, receivers, transmitters, and propellers. Some commonly used examples of these components are shown in Table 2 .
Some examples of quad-copter system parts and advanced accessories used in modern commercial drones

Day by day, gradual improvements are also seen in the development/application of advanced accessories, i.e., camera (infrared, HD cameras and high-speed camera) and gimble system; sensor (gyroscope, Magnetometers, optical, ultra-sonic and accelerometer, etc.) and communication systems (Table 2 ).
First Person View (FPV) of UAV
Lupashin et al. [ 21 ] have demonstrated a video of quad-copters and their motion of flight indoor and outdoor. They have further used the technique of motion capture for explaining the topics of rhythmic flight, aggressive adaptive flight, unstable flight, iterative learning and balance of quad-copter for a perfect flight. Ollero et al. [ 22 ] also explained about a video and results of UAV from four European projects. Mellinger et al. [ 23 ] discussed the techniques of capturing motion, manipulation, perching and flying through the window with the quad-copter.
Development of UAV’s and Types
The information regarding types of UAV’s and growth are collected from the past two decades by observing in the results of Web of Science and Scopus [ 24 ]. The information is framed into a graph for better understanding as shown in Fig. 10 . It can be observed from the bar graph that the growth of helicopters is gradually decreasing starting from 48, 38, and 28 to 20. It is because of its design and control, maneuverability in air and cost. The number of Tri-copter papers remained 0 up to 2004 and increased to 8 in 2005–2009 and 15 in 2010–2014 and 39 in 2015–2021.2 (Up to 2021 February) because of its limited functions and carrying of payload.

Overview of distribution of UAV types with the change of research papers over the years (Best viewed in colour)
The most impressive transformation in the bar graph is the growth of quad-copters i.e. from 7 in 2000 to 2004 to 509 in 2021.2 because of its flexible design and multi-operational function in various applications. Nowadays, quad-copters are also used for the transportation of goods and medicines in the field of hospitals and firms. Multinational companies like Amazon, E-bay, McDonald’s and KFC are planning to use quad-copter as a means of transport for delivering the goods to the customers to save time [ 25 , 26 ]. Research papers in the field of hexa-copter remained 0 until 2009 and the value increased to 11 in 2010–2014, in 2015–2021.2 it went on increasing to 259. In the same way, the number of papers in octa-copter remained at 68 till 2014 but in the year 2015–2021.2, 359 articles were found on the topic of octa-copters. The reason for the growth of interest in hexa-copters and octa-copters is the mode of control is easy in these copters i.e. if one motor of these copters fails during hovering it does not lead to malfunctioning of the copter and can be landed safely. Further, the control algorithm is not complex. These copters can carry more payload and the researchers can easily attach extra hardware like robotic arms or LIDAR sensors for mapping, inspection and various applications. Researchers are also showing interest in fixed-wing aerial robots as the value reached 56 in 2021.2. The interest in the field of other aerial robotics gradually increased from 2005 to 09 and lost its place as the UAV’s were introduced [ 27 , 28 ].
Overview of UAV’s Yearly Distribution and Types
A survey is conducted in the ten top journals and international conferences from 2010 to date to identify the research papers with broad information on drones and aerial robotics as plotted in Fig. 11 . During the survey, it is noticed that the number of research papers has increased from year to year as compared to other topics. Improvements in technology and sensors, flight controllers and receivers have made it easy for the researchers to concentrate more on the field of aerial robotics. A broad area of research is still being carried in this field and the numbers of papers are increasing with the current trends in hobbyist, research and competitions held all across the globe.

Number of papers published from top journals and conferences from the year 2010–2021.2 with a moving average curve (Best viewed in colour)
The topic of motion capturing system is also increasing rapidly in the field of UAVs or drones since 2009 [ 29 ]. This system will help to record the smallest movement of reflective markers mounted on UAV with the help of high-speed action multiple cameras in real-time as shown in Fig. 12 .

UAV research papers over the years on motion capture systems
In this literature survey, research is also carried out on multiple countries mostly publishing the UAV papers in journals and conferences (National and International). Among all of them, the top twelve developed/developing countries such as USA, Switzerland, France, Australia, Japan, Germany, India, China, South Korea, Italy, United Arab Emirates and others are mostly researching on integration of various sensors and modifications in the structure of UAVs for multiple applications. The list of countries in ‘Others’ are Spain, North Korea, United Kingdom, Canada, Belgium, Mexico, Austria, Venezuela, Slovakia, Portugal, Brazil, Czech Republic, Netherlands, Singapore, Saudi Arabia, Malaysia, Cyprus, Philippines, Iran, Taiwan, Israel, Croatia, Sweden, Hungary, South Africa, Finland, Iran, Turkey, Greece and Denmark.
Types of Drones or UAV
In the previous sections, a discussion is carried on the topics of UAV papers and their distribution in terms of percentages and numerical numbers across the world. In this section, a brief discussion is shown on the types of UAVs and their applications presented by the researchers in the past.
In various domains of robotics, BIOMIMETIC robots are one of the most lucrative domains across the globe. The unique aviation concept used in Flapping-wing micro aerial vehicles (FWMAVs) has received a lot of attention for improving aviation quality. It is being accepted for a wide range of possible uses in military and civic domains, including catastrophe investigation, intelligence collection, anti-terrorism reconnaissance, etc. They are characterized by good maneuverability, camouflage ability and high flight efficiency [ 30 ]. Approximately 1000 flapping-wing flying robots with stable flight performance have been produced so far. They were employed for a variety of purposes, including scientific study, flight testing and high-tech entertainment at parties [ 31 ].
UAVs with flapping wings in the shape of insects are most common and they are also known as ‘Ornithopter’ and are about hand size. Ma et al. [ 32 ] have developed a novel based flapping-wing UAV (Fig. 13 a) in the shape of Bee with a mass of 380 mg. This mini UAV was hovering with stability in the air. Peterson et al. [ 33 ] fabricated a model known as ‘BOLT’-flapping-wing UAV and also known as a bipedal robot as it can move on the ground surface and can fly in the air (Fig. 13 b). Paranjape et al. [ 34 ] have designed a unique type of UAV with wing articulation for controlling the head angles and flight path of UAV. This type of UAV can perch naturally on a human hand or chair (Fig. 13 c). Rose et al. [ 35 ] have demonstrated a flapping-wing UAV with a bird shape known as H 2 bird (Fig. 14 d). It is widely used for comparing the data from wind tunnels and free flight with aerodynamic modelling.

Models of flapping-wing [ a – d ] [ 32 – 35 ] (Best viewed in colour)

Angle of attack and air speed of the FWMAVs wing is affected by flapping [ 36 ]
In general, birds are the inspiration for developing FWMAV and fixed-wing aircraft. When a bird is flying, the actual airspeed experienced by its wings is a mix of forwarding movement of the body and flapping motion of the wings. The airflow over wings will be altered by the combination of these two types of motion, allowing the birds to fully utilize the high lift devices (Fig. 14 ). The air flow surrounding the birds and FWMAV in-flight differs significantly resulting in improvement of flying efficiency and performance.
He et al. [ 36 ] presented a boundary control approach for controlling the two links rigid-flexible wing for FWMAV. It’s design is based on the bionics idea to improve aircraft movement and flexibility. The modelling and vibration restraint of a rigid-flexible wing are investigated in this study. The proposed control technique by the author solves these challenges and the controllers are seen to be built to suppress vibrations and deformations. In addition, the angular error appears to be decreasing.
Fixed-wing UAV’s are also known as airplanes. Due to their highest flight stability and longer flight time they are considered to be the dominant aircraft in the history of aviation. As compared to conventional multi-rotor aircraft it has the highest efficiency of energy and can stay and glide for a long time in the air irrespective of engine/controller failure. Bapst et al. [ 37 ] demonstrated a combination of UAV (Fig. 15 a) with their modelling and design to achieve flight of cruise alike fixed-wing and Vertical Take-off and Landing (VTOL) like conventional multi-rotor.

Models of fixed-wing [ a – h ] [ 37 – 44 ] (Best viewed in colour)
Sa et al. [ 38 ] developed a fixed-wing UAV with the configuration of a quad-copter to fly safely in the air and achieve VTOL (Fig. 15 b). Zufferey et al. [ 39 ] have fabricated a small autonomous fixed-wing UAV that weighs about 30 g (Fig. 15 c). With onboard sensors like ultra-sonic, IR and optical flow, this vehicle will navigate safely by avoiding obstacles in the indoor environment. Daler et al. [ 40 ] developed a multi-functional UAV that can hover in the air and move on the ground surface by rotating its wings (Fig. 15 d). Bryson et al. [ 41 ] fabricated a fixed-wing UAV with multiple sensors placed on it such as IMU, GPS, telemetry and monocular camera facing downwards direction for acquiring high-definition images and recording video (Fig. 15 e). These vehicles are mainly used for applications like surveillance and mapping. Papachristos et al. [ 42 ] also developed a multi-functional fixed-wing UAV that can hover in the midair like a tri-copter and execute cruise flight (Fig. 15 f). Kumara et al. [ 43 ] proposed a fixed-wing UAV for demonstrating the system identification and learning the model of complex dynamics with Gaussian process (Fig. 15 g). Morton et al. [ 44 ] developed a solar-powered fixed-wing UAV by giving detailed information regarding modelling, design and development of hardware (Fig. 15 h).
A cyclo-copter is a type of UAV with a cyclo-gyro wing and additional wings fixed on the edges of the cylindrical structure. The required amount of forces is generated by adjusting the angle of attack with a fixed servo motor on it. Hara et al. [ 45 ] developed and fabricated a model of cyclo-copter based on the structure of pantograph. In these vehicles, flight control is achieved by contracting or expanding the wings by varying their diameters concerning the rotational axis (Fig. 16 a). A blimp is a lightweight UAV (lighter than air) with an envelope structure that depends mostly on helium gas to generate lifting force. This envelope shape helps the blimp to maintain the internal pressure of the helium gas and achieve motion control using the units of actuation. Muller et al. [ 46 ] presented a blimp that can navigate autonomously with online motion planning systems in indoor environments (Fig. 16 b).

Models of Cyclo-copter, Blimp, Glider and Kite [ a – d ] [ 45 – 48 ] (Best viewed in colour)
A glider is a type of UAV that uses aerodynamics and its wings to glide freely in the air. It has a structure of fixed-wing UAV but it does not depend on a propulsion system for hovering. Woodward et al. [ 47 ] have built a glider inspired by a vampire bat, this glider will bound from the ground and use its wings to fly/hover in the midair (Fig. 16 c). Kites are also known as parafoil UAVs, Christoforou et al. [ 48 ] introduced a kite UAV with robotic technology to fly autonomously in the air (Fig. 16 d) for the application of aerial cargo delivery.
Kastelan et. al. [ 49 ] have developed a tricopter with three independent propellers and a rigid body for neglecting the dynamics of the vehicle as shown in Fig. 17 a. A method of a pilot supported control scheme is proposed for steering the tri-copter. Belal Sababha et al. [ 50 ] has proposed a novel design and control mechanism for tri-copter (Fig. 17 b). For stable maneuvering, the results of kinematic and dynamic modelling are simulated and corrected. Ziwei Song et al. [ 51 ] (Fig. 17 c) have compared three different designs of tri-copter and rotor configurations are selected based on the performance. PID simulation is carried in MATLAB for reducing the overshoot and peak time.

Models of Tri-copters [ a – c ] [ 50 , 51 ]
Driessens et al. [ 52 ] developed a simplified quadcopter in the name of ‘Y4’ (Fig. 18 a) to mix the energy efficiency of a helicopter with a traditional and conventional quad-copter. Oosedo et al. [ 53 ] have proposed a quad-copter of a unique type with various pitch angles for stable hovering in the air with four propellers and motors (Fig. 18 b). Mulgaonkar et al. [ 54 ] designed and developed a small and low weight quad-copter hardly weighing about twenty five grams (Fig. 18 c). Ishiki et al. [ 55 ] modelled a quad-copter with an array of microphones (Fig. 18 d) for performing localization of sound. Kalantari et al. [ 56 ] build the first-ever quad-copter that can hover in the air and can roll on the ground surface (Fig. 18 e). Okada et al. [ 57 ] showcased a quad-copter with the mechanism of gimbal with rotating shell (Fig. 18 f) and helps the vehicle for stable hovering in the air and avoids obstacles. These types of copters are much useful for applications like surveillance and inspection.

Models of Quad-copters [ a – l ] [ 53 – 63 ] (Best viewed in colour)
Kalantari et al. [ 58 ] developed a model of quad-copter with a gripper mechanism for autonomous take-off and perching on walls (Fig. 18 g).
Abeywardena et al. [ 59 ] demonstrated a quad-copter as shown in Fig. 18 h with Kalman filter, IMU sensor and monocular camera for generating high-frequency odometry. Shen et al. [ 60 ] is the first person to fly an autonomous quad-copter (Fig. 18 i) with a magnetometer, GPS sensor, altimeter, tiltable camera and sensors for hovering inside and outside for the application of surveillance, mission planning, search and rescue. Papachristos et al. [ 61 ] have demonstrated a (DIY) Do-It-Yourself quad-copter (Fig. 18 j) for avoiding obstacles and collisions, tracking and locating a moving target. Latscha et al. [ 62 ] developed a hybrid quad-copter with the combination of two snake structured mobile robots for safety and robustness to monitor the disaster scenarios (Fig. 18 k). Darivianakis et al. [ 63 ] designed and developed a quad-copter (Fig. 18 l) for inspecting the infrastructures and physically interacting with them.
Nguyen et al. [ 64 ] have developed a commercial hexa-copter for path planning, inspection and obstacle avoidance in real-time hovering (Fig. 19 a). Park et al. [ 65 ] built a hexa-copter (Fig. 19 b) with six bi-directional motors aligned asymmetrically for a stable flight. It has many advantages compared to conventional hexa-copter as resistance to wind disturbance, Human Drone Interaction (HDI), and accurate path of flight. Jannoura et al. [ 66 ] have developed a small low altitude and cheap hexa-copter for acquiring high-resolution images in agriculture (Fig. 19 c). Jeroen et al. [ 67 ] have proposed a unique Model Predictive Control (MPC) for hexa-copters (Fig. 19 d). A mathematical explanation is given for achieving a stable and fast response in maneuvering with a remote controller. Dmitrijs Lancovs et al. [ 68 ] have developed a model for collision avoidance using UGCS software and an aircraft emulator. It helps determining the path of the vehicle with the onboard sensors like IMU and telemetry, etc. (Fig. 19 e).

Models of Hexa-copters [ a – e ] [ 65 – 68 ] (Best viewed in colour)
- vii. Octa-copters
An Octa-copter is a UAV with eight motors and propellers fixed on them. Brescianini et al. [ 69 ] fabricated an octa-copter with 6-DOF (Degrees of Freedom) (Fig. 20 a) to generate a stable flight in the air. This type of vehicle is widely used for the applications like aerial surveillance and manipulation. Schneider et al. [ 70 ] developed an octa-copter with on-board multiple sensors and a fish-eyed camera (Fig. 20 b). This type of system is useful in the mapping of vegetation and surveillance of crowded areas.

Models of Octa-copters [ a – b ] [ 69 , 70 ]
Growth of UAVs in Future
In the forthcoming days, UAVs are rapidly emerging in various applications such as path planning, inspection, fire-fighting service, air ambulance, tracing and tracking, delivery and transport, etc. UAV’s are going to be equipped with high-definition multiple cameras and sensors to capture heterogeneous data such as ultraviolet and thermal images, video and audio recording in applications like surveillance [ 71 ] and monitoring, precision-agriculture, crowd management, transportation, etc. The present technology is not capable to handle such kind of heterogeneous data, so in the future modern algorithms and methods alike (Machine Learning, Artificial Intelligence, Big data, Internet of Things, edge computing and software-defined network) are used to tackle the difficulties and deployment of UAVs in various applications.
Need of Autonomy in UAVs
In a dynamic environment, UAVs might collide with obstacles while performing different operations in various applications. The present technologies are not capable of overcoming these issues. So there is a need for autonomy implementation in UAV or aerial robotics sector. Autonomy is used in different applications such as inspection, path planning, communication, delivery system, task scheduling, etc. [ 72 ]. As mentioned in [ 73 ] there are ten levels of autonomy that are used for different applications. These levels are used to define the control levels and autonomy as a metric in UAVs as shown in Fig. 21 .

Control levels of UAV autonomy in future [ 73 ]
So there is a need for autonomy implementation in UAV or aerial robotics sector. Autonomy is used in different applications such as inspection, path planning, communication, delivery system, task scheduling, etc. [ 72 ]. As mentioned in [ 73 ] there are ten levels of autonomy that are used for different applications. These levels are used to define the control levels and autonomy as a metric in UAVs as shown in Fig. 21 .
Domains of UAV Research
Based on the survey conducted on various research papers on the topic of UAV across the globe and from top international and national journals, conferences it is observed that the current trend is mainly focusing in the field of UAV’s. The top most categories or domains concentrated by researchers are shown in Fig. 22 . A vast survey and information regarding to drones/UAVs and robotics/aerial manipulation were collected, reviewed and extended from the top journals or international conferences like Journal of Electronics, Journal of Electrical Engineering, Array, Journal of Guidance, International journal of mechanical and production engineering research and development [ 74 ], Control and Dynamics [ 75 ], International Conference on Unmanned Aircraft Systems [ 76 ], International Journal of Robust and Non-linear Control [ 77 ]. The plethora of UAV domain research in various fields is briefly explained. Samad et. al. [ 78 ] explained a deep role of mapping with UAV in various applications like civil constructions, mapping of vegetation, mapping of power lines, etc. Mahjri et al. [ 79 ] has stated various applications of 3D UAV localization and path planning. The algorithm of path planning is divided mainly into five categories: Mathematical model-based, sampling-based, bio-inspired, multi-fusion and node-based. Various approaches are implemented in path planning approaches that include waypoint-based navigation, vision-based navigation and GPS based navigation. Howden et al. [ 80 ] has focussed on collective intelligence algorithm on localized control instead of centralized control. Danoy et al. [ 81 ] developed a homogeneous network of UAV’s carrying an upper layer (altitude, stability) and a lower layer (VTOL, low altitude) to form a swarm with the improved connecting network in a wide range of applications and operations. Roberts et al. [ 82 ] demonstrated the tracking position of VTOL UAV. It presents the interference of drone flight and data collecting. Nemra et al. [ 83 ] proposed a fusion of inertial navigation sensor and GPS sensor scheme for localization of UAVs with the help of state-dependent Riccati equations.

Domains of UAV research
In [ 84 ] a small UAV for collecting hyper-spectral images of vineyards and other crops is explained. The images are transmitted in near real-time to a base distance and are used to assess crop vitality from observations of the reluctance of canopies. Grenzdorffer et al. [ 85 ] also explained the potential of UAV’s photogrammetry in agriculture and forestry to form a GIS-based data acquisition system with input images. Mauriello et al. [ 86 ] also demonstrated the use of drones for thermal imaging on power lines and buildings to locate leakages, defects in conductors and insulators and heat loss. Customization and Applications is a domain with a high level of skills, precision and accuracy. Cho et al. [ 87 ] proposed a technique for estimation of wind and air speed with the help of a GPS antenna and a pitot tube. Ferworn et al. [ 88 ] have explained a method for the reconstruction of a collapsed building and civil constructions using an approach of game-engine based simulation. This simulation can be used for formulating the search and rescue operations with the help of UAVs. The wide applications of UAV’s in various categories include a survey in water with a water proof camera integrated with UAV were proposed by Ruangwiset et al. [ 89 ] and detection of water-stress in crops with UAVs by Tejada et al. [ 90 ]. The usage of drones in agriculture has got a significant growth such as precision agriculture by Das et al. [ 91 ], thermal imaging and monitoring of crop fields by Bellvert et al. [ 92 ] and in various aspects are extensively explored.
The top trending drones in society with advanced technology and features will also provide prolific information. For example, UAVs like DJ Mavic Pro, DJ Phantom 4, Matrice 100, 200, 210 and Parrot, etc., will provide featured solutions to many existing problems like inspection, mapping, surveying, tracking, path planning, autonomous hovering, and obstacle avoidance, etc. Conversely, some companies will not provide excessive information to the public regarding their models of UAVs for security and privacy reasons.
Indeed, the COVID-19 pandemic has sped up the drone's consumption in different applications. Peculiarly in logistics and delivery systems, drone applications have found wide utilities [ 93 , 94 ].
Conclusion and Future Scope
The main goal and intent of this paper are to review and present current aerial vehicles literature. In particular, this paper has mostly focussed on journals published from 2010 to till date. Throughout this literature, it has been observed and understood that to have a stable and strong foundation in the advancement of scientific and technical areas of UAVs/drones, a review of relevant literature is required. A review paper leads to identifying challenges and uncovers areas where essential research is needed. Inspired by this idea, an attempt has been made to identify the top approaches and attempts in this area and present them in detail. The data presented in Sect. 4 explains the aforementioned methods and concepts that could be an advantage for researchers to understand the existing technology or can contribute to this domain. An explanation of various drones designed and developed by various researchers is also explained in detail with different techniques, components used and their images. The trends of drones from 2010 to 2021.2 is presented with bar charts and graphs in terms of publishing ratios. A detailed explanation regarding current trends in drones and the interest of researchers in particular drones/UAVs are also explained.
In this study, Google Trends is used to represent various search strings used around the world. The top keywords from Web of Science indexed articles are also mentioned to find the relevant articles. Most of the articles retrieved are determined on the topics of path planning and task scheduling as compared to UAV communications and inspections. The numbers of articles in UAV inspections are found less which leads towards a research gap to be filled in future. Despite this vast survey, a rigorous study is yet to be required in the field of UAVs and new technologies implemented in them across the globe. This review regarding newly developed drones with their components, types of controller used, programming languages and techniques implemented will help the newly emerging researchers to concentrate and carry out their work in the right direction.
The overall approach is about the trend of drones/UAVs and the ratio of researches addressed in this field. It can be forecasted that researchers will continue their research on autonomous aerial vehicles in the future. In reality, a momentous amount of work remains to be carried out in the future. This includes hybrid aerial robotics, air-ground drones, long flight drones with smart technology batteries and VTOL drones with high accuracy, etc. Furthermore, only a small amount of information is presented regarding drones/UAVs with their applications. However, in the domain of UAVs, some issues and constraints need to be addressed properly. A few of these interesting topics are highlighted for future research directions. They are (i) UAVs communication, (ii) Dynamic path planning, (iii) Privacy and security, (iv) Integration of different segments, for network communication between air, ground and space UAVs, (v) Efficient energy for UAVs, (vi) Union of UAVs and AI, (vii) Indigenous programming for UAVs to tackle the multiple issues in inspection, communication and collision avoidance. There are still remains a significant amount of research in future on drones/UAVs and aerial robotics.
Acknowledgements
The authors would like to thank the project ‘Quad-copter application for live inspection of power transmission lines’ (Ref no: T-32) funded by DST-ICPS, India for supporting this research.
Declarations
The authors declare no conflict of interest.
Contributor Information
Faiyaz Ahmed, Email: ni.ca.tinnm@demham .
J. C. Mohanta, Email: ni.ca.tinnm@atnahomcj .
Anupam Keshari, Email: [email protected] .
Pankaj Singh Yadav, Email: ni.ca.tinnm@ysjaknap .
Purdue UAS Research and Test Facility - Purdue University

A recap to the 2023 IEEE UAV Chase Challenge
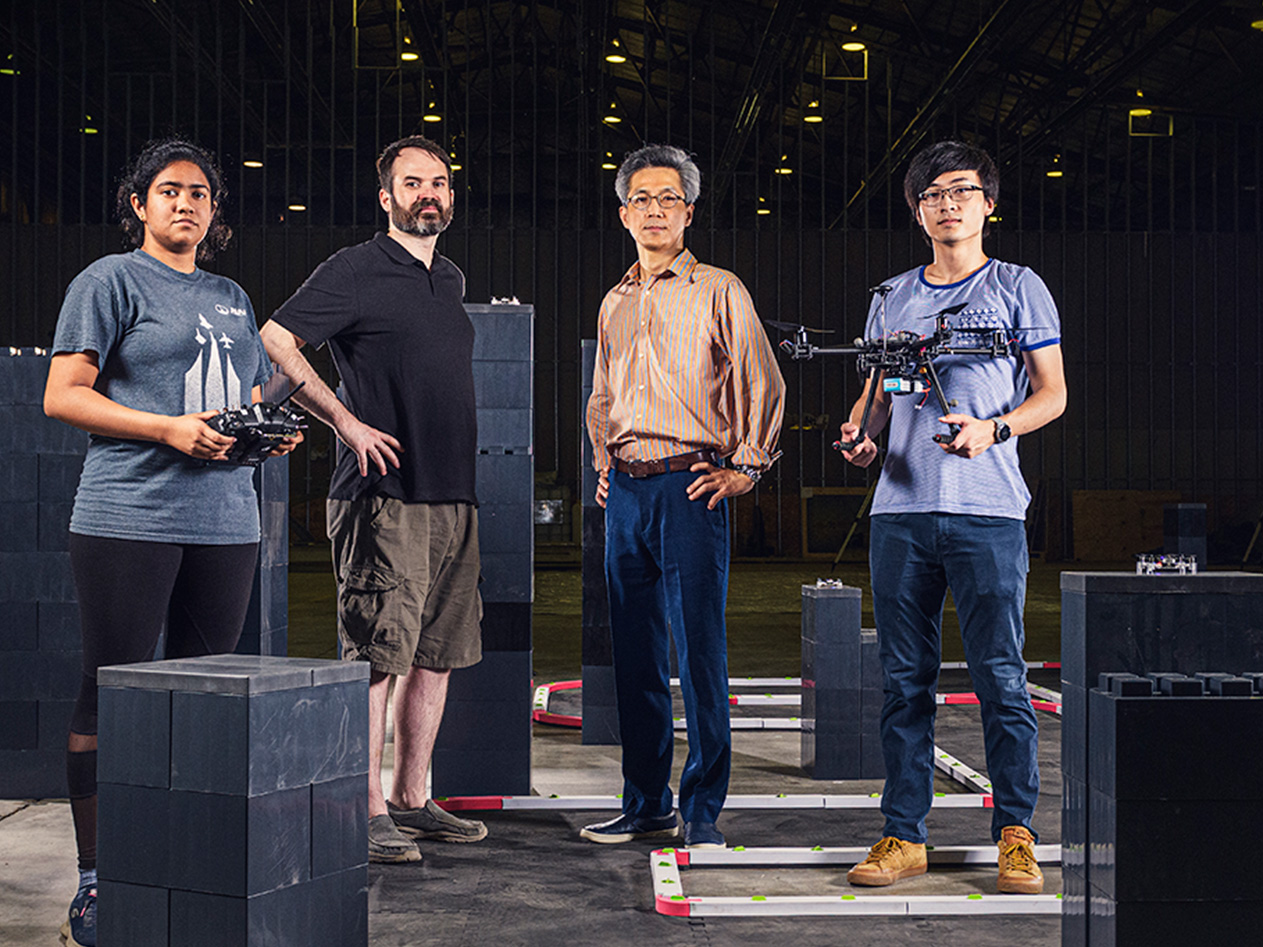
High-Tech Cameras for UAS research
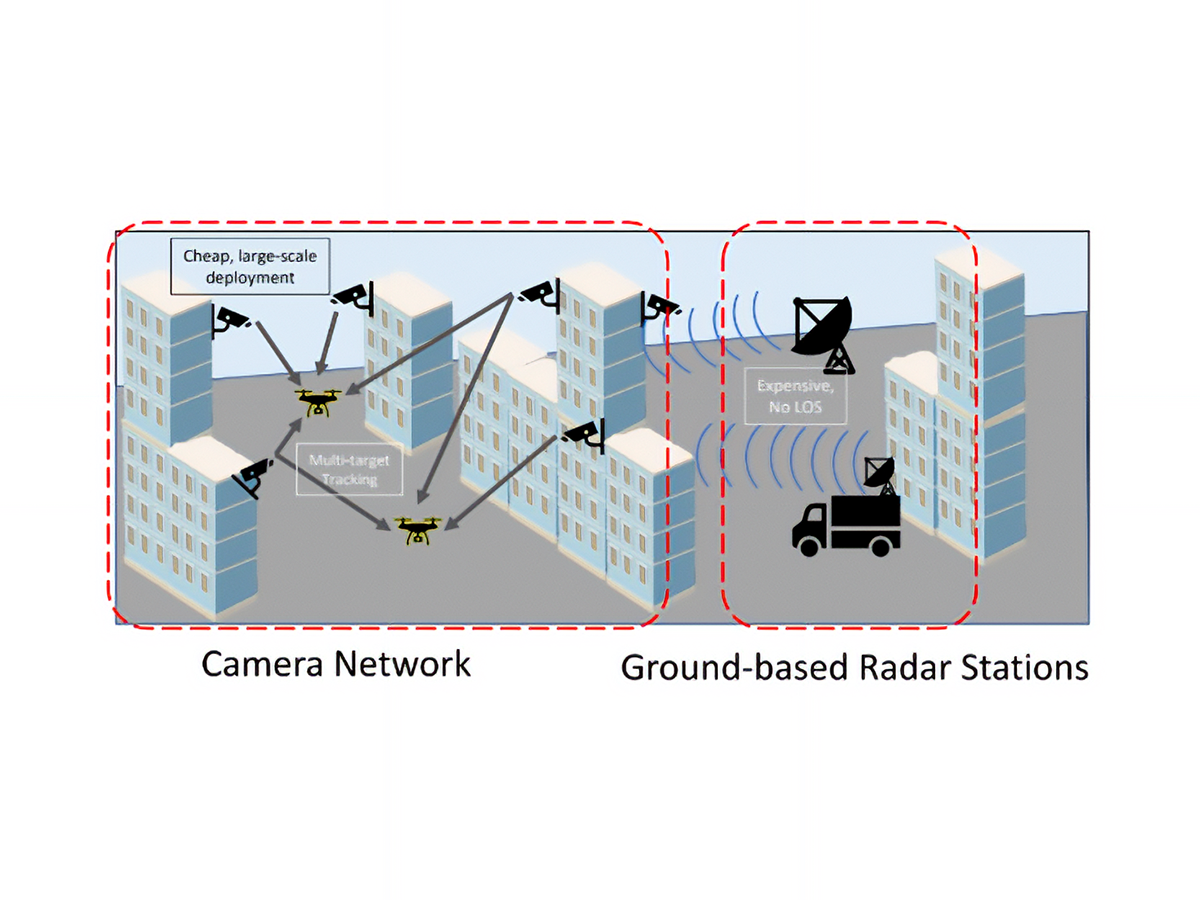
Cyber-Physical Security in a Smart City
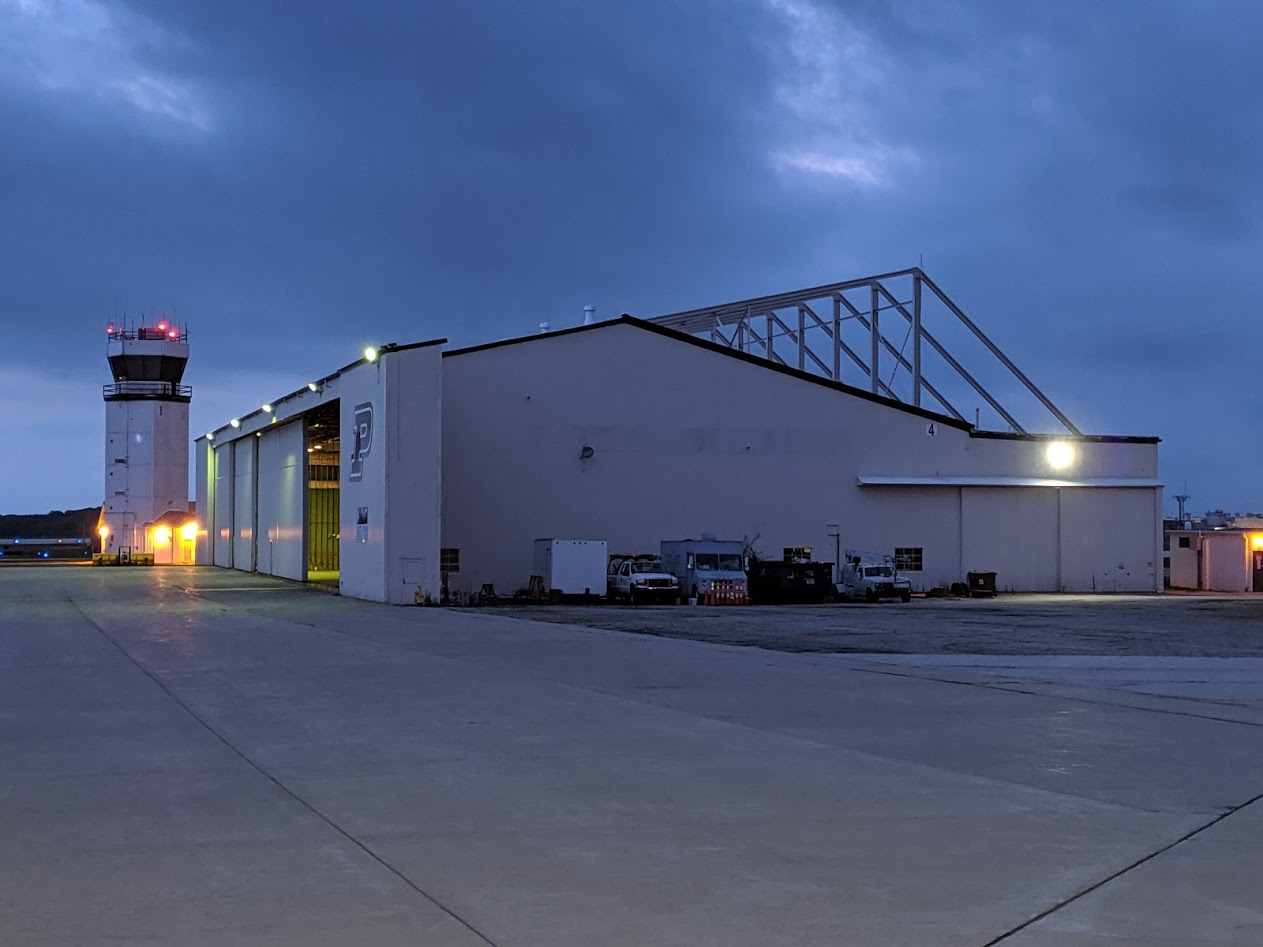
22,000 square feet of testing space
Purdue's home for drone autonomy and control.
The Purdue UAS Research and Test Facility (PURT) can do what others can't. Our motion-capture system is unmatched in its size, and is one hundred times more accurate than GPS. Our 600,000 cubic feet of volume allows us to fly many types of drones, including fixed-wing, in a space protected from weather. Our camera system gives Purdue researchers unmatched precision to develop the unmanned aerial systems essential to the future of mobility.
Research done at PURT enables unmanned aerial systems (UAS) technologies like:
- emergency delivery of overdose treatments or other critical medications
- finding victims trapped under collapsed buildings
- searching for missing persons in wooded areas
- automatic monitoring of plant growth
Motion-capture systems like this are frequently used for movie special effects, but it has a special purpose here. By tracking the exact locations of anything in our space, we can conduct research that develops drone navigation algorithms, corrects for shortcomings with GPS and much more. The capture and virtual reality systems here offer significantly higher precision than commercially available VR equipment, and in a space of a much larger volume: PURT's footprint has an area of 20,000 square feet, and extends to a 30 foot high ceiling.
Explore our capabilities and successes:
Facility Research Education
IEEE Autonomous UAV Chase Challenge, Spring 2023
Recap of 2023 competition Competition Website
"A very, very unique facility"

Read the cover story on PURT in the Fall 2021 issue of Aerogram magazine.
Penn State | College of Engineering

Search this site Search Penn State Search PSU People Search PSU Depts. Web
- Research Overview
- Meet Aerospace Engineering Research Faculty
- Aerospace Engineering Faculty
- Affiliate Faculty
- Emeritus Faculty
- Faculty Mentoring
- Aerospace Engineering Staff
- College-Level Communications Resources
- Undergraduate
- What is Aerospace Engineering?
- Prospective Students
- Majors, Minors, and Certificates
- Suggested Academic Plan
- Curriculum Flow Chart
- Undergraduate Guide
- Honors College
- Co-ops and Internships
- How to Apply
- Undergraduate Research Opportunities
- Degrees and Requirements
- Graduate Studies Guide
- Funding Opportunities
- Student Life
- Graduate Research Opportunities
- Student Organizations
- Career Resources
- Petition Submission
- Financial Aid and Scholarships
- Accreditation
- Student Computer Recommendations
- University Links
- Undergraduate Bulletin
- Graduate Bulletin
- Academic Calendar and Deadlines
- Thesis/Dissertation Deadlines
- Schedule of Courses
- University Registrar
- Quick Links
- About the Department
- Academic Positions
- Faculty Directory
- Staff Directory
- Partner with Us
- Industrial and Professional Advisory Council
Research Areas
- Employer Relations
- Outreach and Inclusion Programs
- Giving Opportunities
- Sponsorships
- Scholarships
- Continuing Education
- Intellectual Properties Policy
- In the News
- Aerospace Engineering Alumni Reception
- Penn State Engineering Alumni Society
- Penn State Alumni Association
- Department Newsletter
- Alumni Updates
- Recognitions
- Outstanding Engineering Alumni Award
- Penn State Engineering Alumni Society Awards
- McCormick Honorary Alumni Lecturers
- Alumni Achievement Award
- Alumni Fellow Award
- Update Your Information
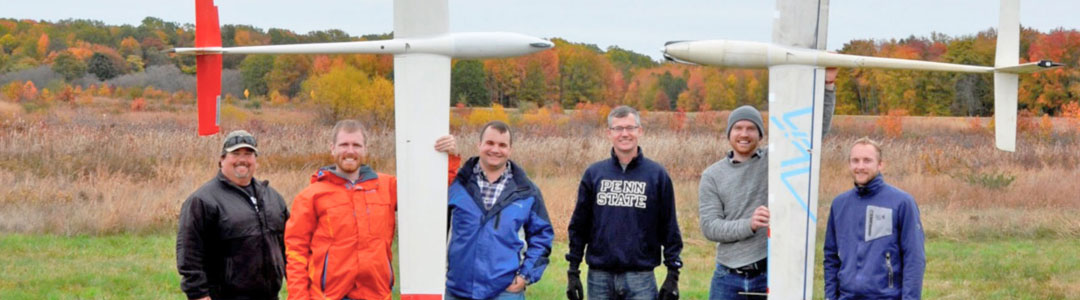
Autonomous Flight and Uninhabited Air Vehicles
In addition to military uses, unmanned aircraft (commonly known as drones) are seeing significant scientific and civil applications. Our research focuses on path planning, control, state estimation, and data fusion, applied especially to navigation, obstacle avoidance, and long-range flight of small uninhabited aircraft.
There are two main thrusts to this research: first, improving perception of the vehicle’s surroundings (i.e., turning sensor data into actionable intelligence); second, improving the vehicle’s persistence (i.e., the length of time it can remain on station and the distance that it can cover in flight).
In many cases, our research is bio-inspired. We are developing algorithms for autonomous flight that allow small UAVs to soar, harvesting energy from the atmosphere. Ultimately, we seek to develop a soaring-capable, autonomous, mini-UAV that is equipped with a sophisticated sensing system that will be able to follow a migrating bird and provide close-up in-flight video, as well as in situ atmospheric measurements.
Key Faculty:
- Eric Johnson
- Jack Langelaan
- Alan Wagner
Research Focus Areas
Our research has a strong focus on hardware implementation. Testbeds include multi-rotors, a helicopter, and fixed-wing aircraft. Specific research topics include:
- Vision-based obstacle avoidance and navigation
- Trajectory generation and trajectory following control
- Flight planning in complex wind fields
- Vision-based landing systems
- Atmospheric sensing
- Mapping algorithms
- Aeroacoustics
- Air-breathing Propulsion
- Astrodynamics
- Autonomous Flight and UAVs
- Computational and Experimental Fluid Dynamics
- Flight Science
- Multifunctional Structures and Nanomaterials
- Rotorcraft Engineering
- Space Propulsion and Plasmas
- Structural Dynamics and Adaptive Structures
- Vehicle Dynamics and Control
- Vehicle Systems Engineering
- Wind Energy
The Penn State Department of Aerospace Engineering, established in 1961 and the only aerospace engineering department in Pennsylvania, is consistently recognized as one of the top aerospace engineering departments in the nation, and is also an international leader in aerospace education, research, and engagement. Our undergraduate program is ranked 15 th and our graduate programs are ranked 15 th nationally by U.S. News & World Report , while one in 25 holders of a B.S. degree in aerospace engineering in the U.S. earned it from Penn State. Our students are consistently among the most highly recruited by industry, government, and graduate schools nationwide.
The department is built upon the fundamentals of academic integrity, innovation in research, and commitment to the advancement of industry. Through an innovative curriculum and world-class instruction that reflects current industry practice and embraces future trends, Penn State Aerospace Engineering graduates emerge as broadly educated, technically sound aerospace engineers who will become future leaders in a critical industry
- Privacy and Legal Statements
- Accessibility
- University Hotlines
- Email Webmaster

Department of Aerospace Engineering
308 Engineering Collaborative Research and Education (ECoRE) Building
556 White Course Drive
The Pennsylvania State University
University Park, PA 16802
Phone: 814-865-2569
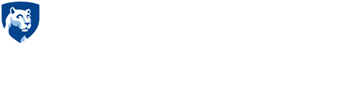
IEEE Account
- Change Username/Password
- Update Address
Purchase Details
- Payment Options
- Order History
- View Purchased Documents
Profile Information
- Communications Preferences
- Profession and Education
- Technical Interests
- US & Canada: +1 800 678 4333
- Worldwide: +1 732 981 0060
- Contact & Support
- About IEEE Xplore
- Accessibility
- Terms of Use
- Nondiscrimination Policy
- Privacy & Opting Out of Cookies
A not-for-profit organization, IEEE is the world's largest technical professional organization dedicated to advancing technology for the benefit of humanity. © Copyright 2024 IEEE - All rights reserved. Use of this web site signifies your agreement to the terms and conditions.

IMAGES
VIDEO
COMMENTS
The growing interest in unmanned aerial vehicles (UAVs) from both the scientific and industrial sectors has attracted a wave of new researchers and substantial investments in this expansive field. However, due to the wide range of topics and subdomains within UAV research, newcomers may find themselves overwhelmed by the numerous options available. It is therefore crucial for those involved in ...
A comprehensive survey of unmanned aerial vehicles, their applications, challenges, and future trends, based on the latest scientific literature.
Recently, unmanned aerial vehicles (UAVs) or drones have emerged as a ubiquitous and integral part of our society. They appear in great diversity in a multiplicity of applications for economic, commercial, leisure, military and academic purposes. The ...
The use of unmanned aerial vehicles (UAVs) is growing rapidly across many civil application domains, including real-time monitoring, providing wireless coverage, remote sensing, search and rescue, delivery of goods, security and surveillance, precision agriculture, and civil infrastructure inspection. Smart UAVs are the next big revolution in the UAV technology promising to provide new ...
In recent decades, aerial robots especially small UAVs and drones have witnessed tremendous improvements in terms of their structure, working methodology, flying features and navigation control. UAVs are highly utilized in a wide range of services such as photography, path planning, search and rescue, inspection of power lines and civil constructions, etc. This manuscript reports a wide ...
This paper analyzes the UAV communication technologies for both hardware and algorithm-based software, including antenna arrays and signal management, and utilization of centralized and decentralized techniques. Technologies such as FANET, NDN, AFW, and DTN help in synchronization and latency minimization.
With the rapid development of computer technology, automatic control technology and communication technology, research on unmanned aerial vehicles (UAVs) has attracted extensive attention from all over the world during the last decades. Particularly due to the demand of various civil applications, the conceptual design of UAV and autonomous flight control technology have been promoted and ...
These areas play a pivotal role in advancing UAV technology and are critical subjects of investigation for researchers and practitioners alike. Due to the wide range of subdomains and extensive scope of UAV research, coupled with significant invest-ments in this multifaceted field, several important research questions have arisen.
The growing interest in unmanned aerial vehicles (UAVs) from both scientific and industrial sectors has attracted a wave of new researchers and substantial investments in this expansive field. However, due to the wide range of topics and subdomains within UAV research, newcomers may find themselves overwhelmed by the numerous options available. It is therefore crucial for those involved in UAV ...
Abstract Unmanned Aerial Vehicles (UAVs) have become an integral part of modern smart cities and systems. However, the proliferation of UAVs has also brought a significant security concern. Therefore, UAVs security has attracted the interest of researchers in the field. To safeguard these leading devices, researchers and developers adopted artificial intelligence (AI) to improve the security ...
This survey article focuses on the different applications and the related algorithms for realizing aerial base stations by thoroughly reviewing each related research area. In a nutshell, this article provides key applications, challenges, and the technology used for the design and analysis of unmanned aerial vehicles as base stations.
Recently, unmanned aerial vehicles (UAVs) or drones have emerged as a ubiquitous and integral part of our society. They appear in great diversity in a multiplicity of applications for economic, commercial, leisure, military and academic purposes. The drone industry has seen a sharp uptake in the last decade as a model to manufacture and deliver convergence, offering synergy by incorporating ...
PDF | In past few years, unmanned aerial vehicles (UAV) or drones has been a hot topic encompassing technology, security issues, rules and regulations... | Find, read and cite all the research you ...
Metrics. Unmanned aerial vehicles (UAVs) represent a new frontier in environmental research. Their use has the potential to revolutionise the field if they prove capable of improving data quality ...
TRB blog post about drones. Commercial and public-sector demands are helping to accelerate advancements in unmanned systems. For instance, military services and federal agencies use the technology for aerial, surface, and underwater applications. A new National Academies of Sciences, Engineering, and Medicine report from TRB finds that the U.S. Coast Guard should proceed more aggressively and ...
An unmanned aerial vehicle (UAV) is an aircraft that carries no human pilot or passengers. UAVs--sometimes called drones--can be fully or partially autonomous but are more often controlled remotely by a human pilot. RAND research has contributed to the public discussion on the use of drones for warfare and surveillance.
UAV research papers over the years on motion capture systems In this literature survey, research is also carried out on multiple countries mostly publishing the UAV papers in journals and conferences (National and International).
However, the development of best practices for high quality of UAV mapping are often overlooked representing a drawback for their wider adoption. UAV solutions then require an inter-disciplinary research, integrating different expertise and combining several hardware and software components on the same platform.
Purdue's home for drone autonomy and control The Purdue UAS Research and Test Facility (PURT) can do what others can't. Our motion-capture system is unmatched in its size, and is one hundred times more accurate than GPS. Our 600,000 cubic feet of volume allows us to fly many types of drones, including fixed-wing, in a space protected from weather. Our camera system gives Purdue researchers ...
Lab. The UAV research group develops and supports a world class, low cost, open-source avionics platform enabling research and education within the department and worldwide. Our infrastructure is used to conduct flight research on navigation, guidance, and flight control systems to enable future commercial aircraft and small UAVs to be ...
Since the dawn of military aviation, the U.S. military has been interested in remotely piloted aircraft. Present-day unmanned aircraft systems (UAS) typically consist of an unmanned aircraft vehicle (UAV) paired with a ground control station. UAS have become ubiquitous in U.S. military operations since the 1990s with the introduction of the MQ-1 Predator.
Autonomous Flight and Uninhabited Air Vehicles In addition to military uses, unmanned aircraft (commonly known as drones) are seeing significant scientific and civil applications. Our research focuses on path planning, control, state estimation, and data fusion, applied especially to navigation, obstacle avoidance, and long-range flight of small uninhabited aircraft.
This continued misuse has led to research in preventing unauthorized UAVs from causing damage to life and property. In this paper, we present a literature review of UAVs, UAV attacks, and their prevention using anti-UAV techniques.
The intelligent unmanned mooring device adopts a multi-link mechanism and vacuum adsorption design, which can perform heave, surge, and sway actions to adapt to the mooring postures of different ships. The use of flow control valves and accumulators in hydraulic circuits can achieve appropriate dynamic response and oscillation reducing effects on ship oscillation without manual intervention ...