Have a language expert improve your writing
Run a free plagiarism check in 10 minutes, generate accurate citations for free.
- Knowledge Base
Methodology
- How to Write a Literature Review | Guide, Examples, & Templates

How to Write a Literature Review | Guide, Examples, & Templates
Published on January 2, 2023 by Shona McCombes . Revised on September 11, 2023.
What is a literature review? A literature review is a survey of scholarly sources on a specific topic. It provides an overview of current knowledge, allowing you to identify relevant theories, methods, and gaps in the existing research that you can later apply to your paper, thesis, or dissertation topic .
There are five key steps to writing a literature review:
- Search for relevant literature
- Evaluate sources
- Identify themes, debates, and gaps
- Outline the structure
- Write your literature review
A good literature review doesn’t just summarize sources—it analyzes, synthesizes , and critically evaluates to give a clear picture of the state of knowledge on the subject.
Instantly correct all language mistakes in your text
Upload your document to correct all your mistakes in minutes
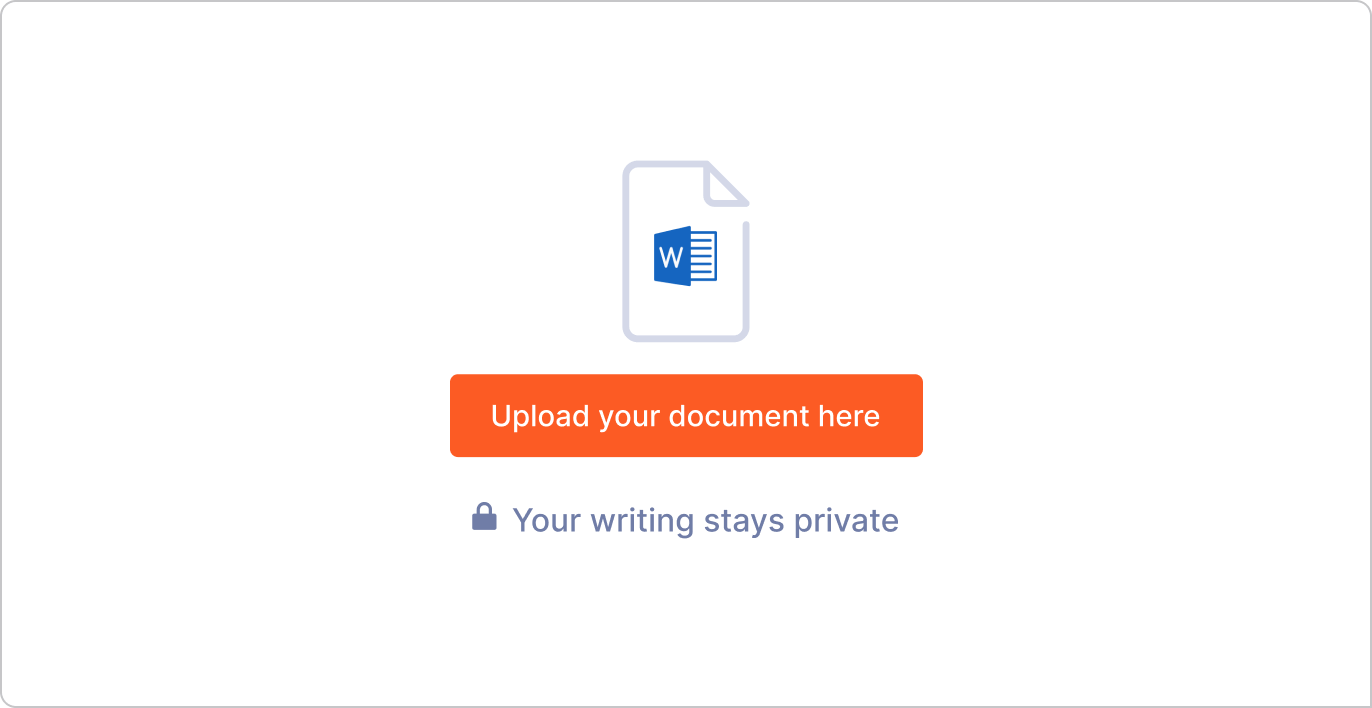
Table of contents
What is the purpose of a literature review, examples of literature reviews, step 1 – search for relevant literature, step 2 – evaluate and select sources, step 3 – identify themes, debates, and gaps, step 4 – outline your literature review’s structure, step 5 – write your literature review, free lecture slides, other interesting articles, frequently asked questions, introduction.
- Quick Run-through
- Step 1 & 2
When you write a thesis , dissertation , or research paper , you will likely have to conduct a literature review to situate your research within existing knowledge. The literature review gives you a chance to:
- Demonstrate your familiarity with the topic and its scholarly context
- Develop a theoretical framework and methodology for your research
- Position your work in relation to other researchers and theorists
- Show how your research addresses a gap or contributes to a debate
- Evaluate the current state of research and demonstrate your knowledge of the scholarly debates around your topic.
Writing literature reviews is a particularly important skill if you want to apply for graduate school or pursue a career in research. We’ve written a step-by-step guide that you can follow below.
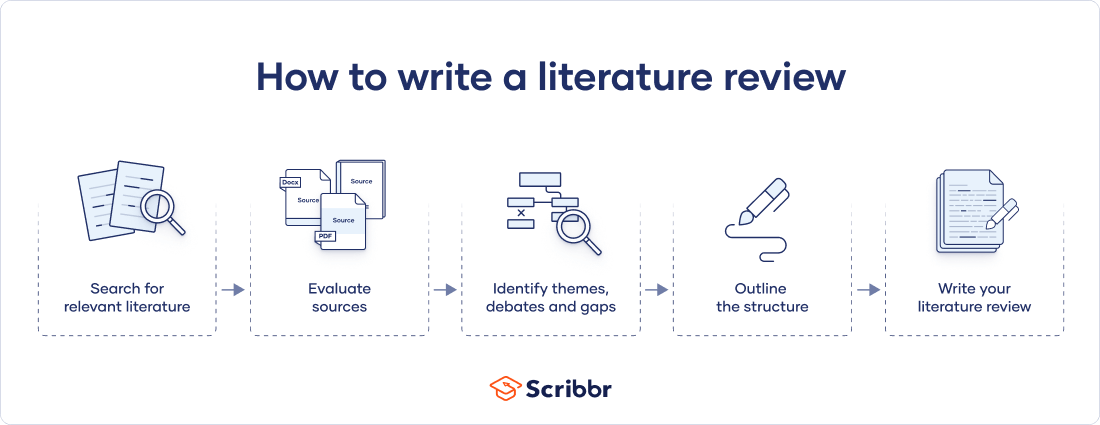
Receive feedback on language, structure, and formatting
Professional editors proofread and edit your paper by focusing on:
- Academic style
- Vague sentences
- Style consistency
See an example
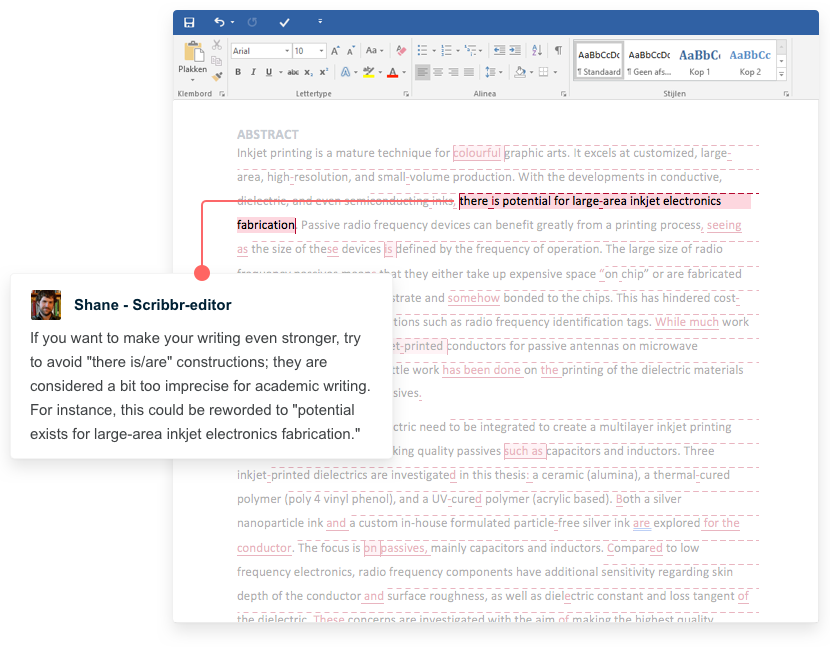
Writing literature reviews can be quite challenging! A good starting point could be to look at some examples, depending on what kind of literature review you’d like to write.
- Example literature review #1: “Why Do People Migrate? A Review of the Theoretical Literature” ( Theoretical literature review about the development of economic migration theory from the 1950s to today.)
- Example literature review #2: “Literature review as a research methodology: An overview and guidelines” ( Methodological literature review about interdisciplinary knowledge acquisition and production.)
- Example literature review #3: “The Use of Technology in English Language Learning: A Literature Review” ( Thematic literature review about the effects of technology on language acquisition.)
- Example literature review #4: “Learners’ Listening Comprehension Difficulties in English Language Learning: A Literature Review” ( Chronological literature review about how the concept of listening skills has changed over time.)
You can also check out our templates with literature review examples and sample outlines at the links below.
Download Word doc Download Google doc
Before you begin searching for literature, you need a clearly defined topic .
If you are writing the literature review section of a dissertation or research paper, you will search for literature related to your research problem and questions .
Make a list of keywords
Start by creating a list of keywords related to your research question. Include each of the key concepts or variables you’re interested in, and list any synonyms and related terms. You can add to this list as you discover new keywords in the process of your literature search.
- Social media, Facebook, Instagram, Twitter, Snapchat, TikTok
- Body image, self-perception, self-esteem, mental health
- Generation Z, teenagers, adolescents, youth
Search for relevant sources
Use your keywords to begin searching for sources. Some useful databases to search for journals and articles include:
- Your university’s library catalogue
- Google Scholar
- Project Muse (humanities and social sciences)
- Medline (life sciences and biomedicine)
- EconLit (economics)
- Inspec (physics, engineering and computer science)
You can also use boolean operators to help narrow down your search.
Make sure to read the abstract to find out whether an article is relevant to your question. When you find a useful book or article, you can check the bibliography to find other relevant sources.
You likely won’t be able to read absolutely everything that has been written on your topic, so it will be necessary to evaluate which sources are most relevant to your research question.
For each publication, ask yourself:
- What question or problem is the author addressing?
- What are the key concepts and how are they defined?
- What are the key theories, models, and methods?
- Does the research use established frameworks or take an innovative approach?
- What are the results and conclusions of the study?
- How does the publication relate to other literature in the field? Does it confirm, add to, or challenge established knowledge?
- What are the strengths and weaknesses of the research?
Make sure the sources you use are credible , and make sure you read any landmark studies and major theories in your field of research.
You can use our template to summarize and evaluate sources you’re thinking about using. Click on either button below to download.
Take notes and cite your sources
As you read, you should also begin the writing process. Take notes that you can later incorporate into the text of your literature review.
It is important to keep track of your sources with citations to avoid plagiarism . It can be helpful to make an annotated bibliography , where you compile full citation information and write a paragraph of summary and analysis for each source. This helps you remember what you read and saves time later in the process.
Prevent plagiarism. Run a free check.
To begin organizing your literature review’s argument and structure, be sure you understand the connections and relationships between the sources you’ve read. Based on your reading and notes, you can look for:
- Trends and patterns (in theory, method or results): do certain approaches become more or less popular over time?
- Themes: what questions or concepts recur across the literature?
- Debates, conflicts and contradictions: where do sources disagree?
- Pivotal publications: are there any influential theories or studies that changed the direction of the field?
- Gaps: what is missing from the literature? Are there weaknesses that need to be addressed?
This step will help you work out the structure of your literature review and (if applicable) show how your own research will contribute to existing knowledge.
- Most research has focused on young women.
- There is an increasing interest in the visual aspects of social media.
- But there is still a lack of robust research on highly visual platforms like Instagram and Snapchat—this is a gap that you could address in your own research.
There are various approaches to organizing the body of a literature review. Depending on the length of your literature review, you can combine several of these strategies (for example, your overall structure might be thematic, but each theme is discussed chronologically).
Chronological
The simplest approach is to trace the development of the topic over time. However, if you choose this strategy, be careful to avoid simply listing and summarizing sources in order.
Try to analyze patterns, turning points and key debates that have shaped the direction of the field. Give your interpretation of how and why certain developments occurred.
If you have found some recurring central themes, you can organize your literature review into subsections that address different aspects of the topic.
For example, if you are reviewing literature about inequalities in migrant health outcomes, key themes might include healthcare policy, language barriers, cultural attitudes, legal status, and economic access.
Methodological
If you draw your sources from different disciplines or fields that use a variety of research methods , you might want to compare the results and conclusions that emerge from different approaches. For example:
- Look at what results have emerged in qualitative versus quantitative research
- Discuss how the topic has been approached by empirical versus theoretical scholarship
- Divide the literature into sociological, historical, and cultural sources
Theoretical
A literature review is often the foundation for a theoretical framework . You can use it to discuss various theories, models, and definitions of key concepts.
You might argue for the relevance of a specific theoretical approach, or combine various theoretical concepts to create a framework for your research.
Like any other academic text , your literature review should have an introduction , a main body, and a conclusion . What you include in each depends on the objective of your literature review.
The introduction should clearly establish the focus and purpose of the literature review.
Depending on the length of your literature review, you might want to divide the body into subsections. You can use a subheading for each theme, time period, or methodological approach.
As you write, you can follow these tips:
- Summarize and synthesize: give an overview of the main points of each source and combine them into a coherent whole
- Analyze and interpret: don’t just paraphrase other researchers — add your own interpretations where possible, discussing the significance of findings in relation to the literature as a whole
- Critically evaluate: mention the strengths and weaknesses of your sources
- Write in well-structured paragraphs: use transition words and topic sentences to draw connections, comparisons and contrasts
In the conclusion, you should summarize the key findings you have taken from the literature and emphasize their significance.
When you’ve finished writing and revising your literature review, don’t forget to proofread thoroughly before submitting. Not a language expert? Check out Scribbr’s professional proofreading services !
This article has been adapted into lecture slides that you can use to teach your students about writing a literature review.
Scribbr slides are free to use, customize, and distribute for educational purposes.
Open Google Slides Download PowerPoint
If you want to know more about the research process , methodology , research bias , or statistics , make sure to check out some of our other articles with explanations and examples.
- Sampling methods
- Simple random sampling
- Stratified sampling
- Cluster sampling
- Likert scales
- Reproducibility
Statistics
- Null hypothesis
- Statistical power
- Probability distribution
- Effect size
- Poisson distribution
Research bias
- Optimism bias
- Cognitive bias
- Implicit bias
- Hawthorne effect
- Anchoring bias
- Explicit bias
A literature review is a survey of scholarly sources (such as books, journal articles, and theses) related to a specific topic or research question .
It is often written as part of a thesis, dissertation , or research paper , in order to situate your work in relation to existing knowledge.
There are several reasons to conduct a literature review at the beginning of a research project:
- To familiarize yourself with the current state of knowledge on your topic
- To ensure that you’re not just repeating what others have already done
- To identify gaps in knowledge and unresolved problems that your research can address
- To develop your theoretical framework and methodology
- To provide an overview of the key findings and debates on the topic
Writing the literature review shows your reader how your work relates to existing research and what new insights it will contribute.
The literature review usually comes near the beginning of your thesis or dissertation . After the introduction , it grounds your research in a scholarly field and leads directly to your theoretical framework or methodology .
A literature review is a survey of credible sources on a topic, often used in dissertations , theses, and research papers . Literature reviews give an overview of knowledge on a subject, helping you identify relevant theories and methods, as well as gaps in existing research. Literature reviews are set up similarly to other academic texts , with an introduction , a main body, and a conclusion .
An annotated bibliography is a list of source references that has a short description (called an annotation ) for each of the sources. It is often assigned as part of the research process for a paper .
Cite this Scribbr article
If you want to cite this source, you can copy and paste the citation or click the “Cite this Scribbr article” button to automatically add the citation to our free Citation Generator.
McCombes, S. (2023, September 11). How to Write a Literature Review | Guide, Examples, & Templates. Scribbr. Retrieved August 26, 2024, from https://www.scribbr.com/dissertation/literature-review/
Is this article helpful?
Shona McCombes
Other students also liked, what is a theoretical framework | guide to organizing, what is a research methodology | steps & tips, how to write a research proposal | examples & templates, "i thought ai proofreading was useless but..".
I've been using Scribbr for years now and I know it's a service that won't disappoint. It does a good job spotting mistakes”
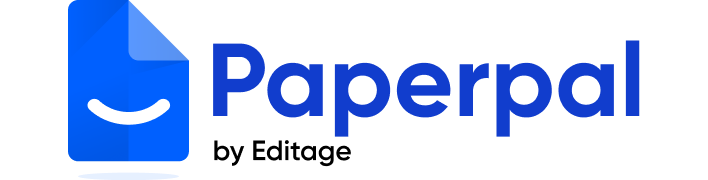
What is a Literature Review? How to Write It (with Examples)
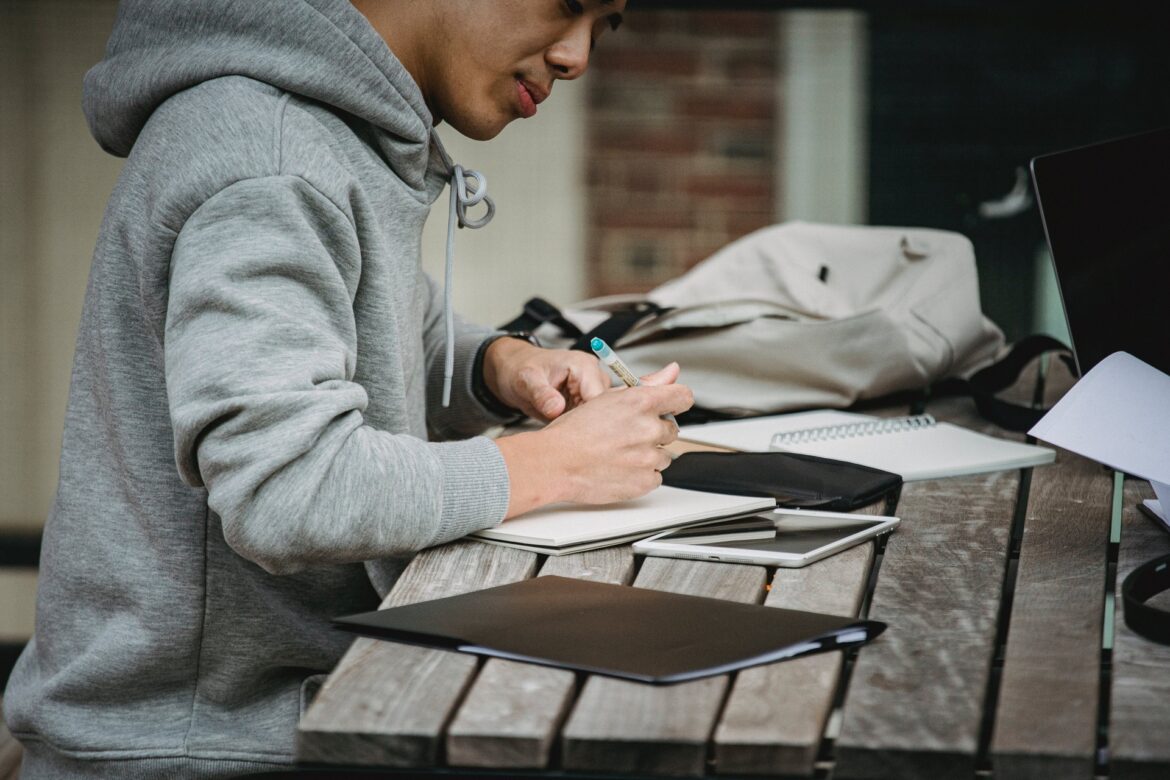
A literature review is a critical analysis and synthesis of existing research on a particular topic. It provides an overview of the current state of knowledge, identifies gaps, and highlights key findings in the literature. 1 The purpose of a literature review is to situate your own research within the context of existing scholarship, demonstrating your understanding of the topic and showing how your work contributes to the ongoing conversation in the field. Learning how to write a literature review is a critical tool for successful research. Your ability to summarize and synthesize prior research pertaining to a certain topic demonstrates your grasp on the topic of study, and assists in the learning process.
Table of Contents
- What is the purpose of literature review?
- a. Habitat Loss and Species Extinction:
- b. Range Shifts and Phenological Changes:
- c. Ocean Acidification and Coral Reefs:
- d. Adaptive Strategies and Conservation Efforts:
How to write a good literature review
- Choose a Topic and Define the Research Question:
- Decide on the Scope of Your Review:
- Select Databases for Searches:
- Conduct Searches and Keep Track:
- Review the Literature:
- Organize and Write Your Literature Review:
- How to write a literature review faster with Paperpal?
- Frequently asked questions
What is a literature review?
A well-conducted literature review demonstrates the researcher’s familiarity with the existing literature, establishes the context for their own research, and contributes to scholarly conversations on the topic. One of the purposes of a literature review is also to help researchers avoid duplicating previous work and ensure that their research is informed by and builds upon the existing body of knowledge.
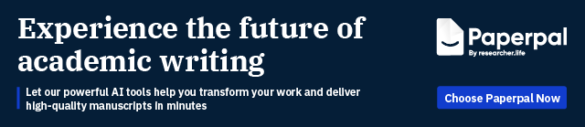
What is the purpose of literature review?
A literature review serves several important purposes within academic and research contexts. Here are some key objectives and functions of a literature review: 2
1. Contextualizing the Research Problem: The literature review provides a background and context for the research problem under investigation. It helps to situate the study within the existing body of knowledge.
2. Identifying Gaps in Knowledge: By identifying gaps, contradictions, or areas requiring further research, the researcher can shape the research question and justify the significance of the study. This is crucial for ensuring that the new research contributes something novel to the field.
Find academic papers related to your research topic faster. Try Research on Paperpal
3. Understanding Theoretical and Conceptual Frameworks: Literature reviews help researchers gain an understanding of the theoretical and conceptual frameworks used in previous studies. This aids in the development of a theoretical framework for the current research.
4. Providing Methodological Insights: Another purpose of literature reviews is that it allows researchers to learn about the methodologies employed in previous studies. This can help in choosing appropriate research methods for the current study and avoiding pitfalls that others may have encountered.
5. Establishing Credibility: A well-conducted literature review demonstrates the researcher’s familiarity with existing scholarship, establishing their credibility and expertise in the field. It also helps in building a solid foundation for the new research.
6. Informing Hypotheses or Research Questions: The literature review guides the formulation of hypotheses or research questions by highlighting relevant findings and areas of uncertainty in existing literature.
Literature review example
Let’s delve deeper with a literature review example: Let’s say your literature review is about the impact of climate change on biodiversity. You might format your literature review into sections such as the effects of climate change on habitat loss and species extinction, phenological changes, and marine biodiversity. Each section would then summarize and analyze relevant studies in those areas, highlighting key findings and identifying gaps in the research. The review would conclude by emphasizing the need for further research on specific aspects of the relationship between climate change and biodiversity. The following literature review template provides a glimpse into the recommended literature review structure and content, demonstrating how research findings are organized around specific themes within a broader topic.
Literature Review on Climate Change Impacts on Biodiversity:
Climate change is a global phenomenon with far-reaching consequences, including significant impacts on biodiversity. This literature review synthesizes key findings from various studies:
a. Habitat Loss and Species Extinction:
Climate change-induced alterations in temperature and precipitation patterns contribute to habitat loss, affecting numerous species (Thomas et al., 2004). The review discusses how these changes increase the risk of extinction, particularly for species with specific habitat requirements.
b. Range Shifts and Phenological Changes:
Observations of range shifts and changes in the timing of biological events (phenology) are documented in response to changing climatic conditions (Parmesan & Yohe, 2003). These shifts affect ecosystems and may lead to mismatches between species and their resources.
c. Ocean Acidification and Coral Reefs:
The review explores the impact of climate change on marine biodiversity, emphasizing ocean acidification’s threat to coral reefs (Hoegh-Guldberg et al., 2007). Changes in pH levels negatively affect coral calcification, disrupting the delicate balance of marine ecosystems.
d. Adaptive Strategies and Conservation Efforts:
Recognizing the urgency of the situation, the literature review discusses various adaptive strategies adopted by species and conservation efforts aimed at mitigating the impacts of climate change on biodiversity (Hannah et al., 2007). It emphasizes the importance of interdisciplinary approaches for effective conservation planning.
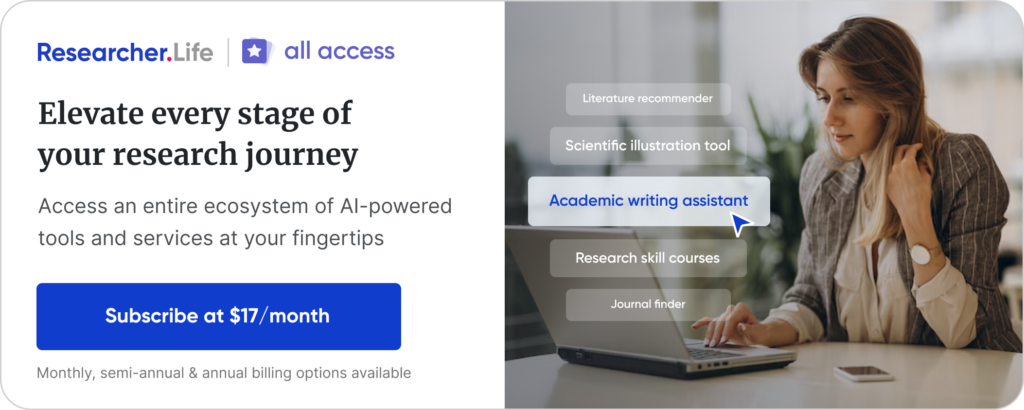
Strengthen your literature review with factual insights. Try Research on Paperpal for free!
Writing a literature review involves summarizing and synthesizing existing research on a particular topic. A good literature review format should include the following elements.
Introduction: The introduction sets the stage for your literature review, providing context and introducing the main focus of your review.
- Opening Statement: Begin with a general statement about the broader topic and its significance in the field.
- Scope and Purpose: Clearly define the scope of your literature review. Explain the specific research question or objective you aim to address.
- Organizational Framework: Briefly outline the structure of your literature review, indicating how you will categorize and discuss the existing research.
- Significance of the Study: Highlight why your literature review is important and how it contributes to the understanding of the chosen topic.
- Thesis Statement: Conclude the introduction with a concise thesis statement that outlines the main argument or perspective you will develop in the body of the literature review.
Body: The body of the literature review is where you provide a comprehensive analysis of existing literature, grouping studies based on themes, methodologies, or other relevant criteria.
- Organize by Theme or Concept: Group studies that share common themes, concepts, or methodologies. Discuss each theme or concept in detail, summarizing key findings and identifying gaps or areas of disagreement.
- Critical Analysis: Evaluate the strengths and weaknesses of each study. Discuss the methodologies used, the quality of evidence, and the overall contribution of each work to the understanding of the topic.
- Synthesis of Findings: Synthesize the information from different studies to highlight trends, patterns, or areas of consensus in the literature.
- Identification of Gaps: Discuss any gaps or limitations in the existing research and explain how your review contributes to filling these gaps.
- Transition between Sections: Provide smooth transitions between different themes or concepts to maintain the flow of your literature review.
Write and Cite as you go with Paperpal Research. Start now for free.
Conclusion: The conclusion of your literature review should summarize the main findings, highlight the contributions of the review, and suggest avenues for future research.
- Summary of Key Findings: Recap the main findings from the literature and restate how they contribute to your research question or objective.
- Contributions to the Field: Discuss the overall contribution of your literature review to the existing knowledge in the field.
- Implications and Applications: Explore the practical implications of the findings and suggest how they might impact future research or practice.
- Recommendations for Future Research: Identify areas that require further investigation and propose potential directions for future research in the field.
- Final Thoughts: Conclude with a final reflection on the importance of your literature review and its relevance to the broader academic community.
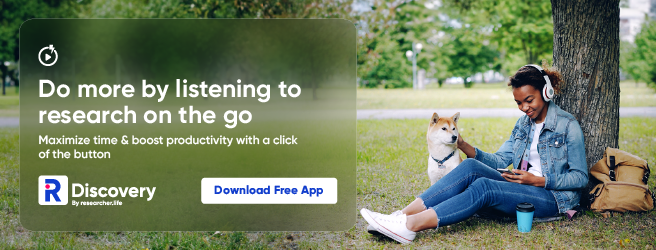
Conducting a literature review
Conducting a literature review is an essential step in research that involves reviewing and analyzing existing literature on a specific topic. It’s important to know how to do a literature review effectively, so here are the steps to follow: 1
Choose a Topic and Define the Research Question:
- Select a topic that is relevant to your field of study.
- Clearly define your research question or objective. Determine what specific aspect of the topic do you want to explore?
Decide on the Scope of Your Review:
- Determine the timeframe for your literature review. Are you focusing on recent developments, or do you want a historical overview?
- Consider the geographical scope. Is your review global, or are you focusing on a specific region?
- Define the inclusion and exclusion criteria. What types of sources will you include? Are there specific types of studies or publications you will exclude?
Select Databases for Searches:
- Identify relevant databases for your field. Examples include PubMed, IEEE Xplore, Scopus, Web of Science, and Google Scholar.
- Consider searching in library catalogs, institutional repositories, and specialized databases related to your topic.
Conduct Searches and Keep Track:
- Develop a systematic search strategy using keywords, Boolean operators (AND, OR, NOT), and other search techniques.
- Record and document your search strategy for transparency and replicability.
- Keep track of the articles, including publication details, abstracts, and links. Use citation management tools like EndNote, Zotero, or Mendeley to organize your references.
Review the Literature:
- Evaluate the relevance and quality of each source. Consider the methodology, sample size, and results of studies.
- Organize the literature by themes or key concepts. Identify patterns, trends, and gaps in the existing research.
- Summarize key findings and arguments from each source. Compare and contrast different perspectives.
- Identify areas where there is a consensus in the literature and where there are conflicting opinions.
- Provide critical analysis and synthesis of the literature. What are the strengths and weaknesses of existing research?
Organize and Write Your Literature Review:
- Literature review outline should be based on themes, chronological order, or methodological approaches.
- Write a clear and coherent narrative that synthesizes the information gathered.
- Use proper citations for each source and ensure consistency in your citation style (APA, MLA, Chicago, etc.).
- Conclude your literature review by summarizing key findings, identifying gaps, and suggesting areas for future research.
Whether you’re exploring a new research field or finding new angles to develop an existing topic, sifting through hundreds of papers can take more time than you have to spare. But what if you could find science-backed insights with verified citations in seconds? That’s the power of Paperpal’s new Research feature!
How to write a literature review faster with Paperpal?
Paperpal, an AI writing assistant, integrates powerful academic search capabilities within its writing platform. With the Research feature, you get 100% factual insights, with citations backed by 250M+ verified research articles, directly within your writing interface with the option to save relevant references in your Citation Library. By eliminating the need to switch tabs to find answers to all your research questions, Paperpal saves time and helps you stay focused on your writing.
Here’s how to use the Research feature:
- Ask a question: Get started with a new document on paperpal.com. Click on the “Research” feature and type your question in plain English. Paperpal will scour over 250 million research articles, including conference papers and preprints, to provide you with accurate insights and citations.
- Review and Save: Paperpal summarizes the information, while citing sources and listing relevant reads. You can quickly scan the results to identify relevant references and save these directly to your built-in citations library for later access.
- Cite with Confidence: Paperpal makes it easy to incorporate relevant citations and references into your writing, ensuring your arguments are well-supported by credible sources. This translates to a polished, well-researched literature review.
The literature review sample and detailed advice on writing and conducting a review will help you produce a well-structured report. But remember that a good literature review is an ongoing process, and it may be necessary to revisit and update it as your research progresses. By combining effortless research with an easy citation process, Paperpal Research streamlines the literature review process and empowers you to write faster and with more confidence. Try Paperpal Research now and see for yourself.
Frequently asked questions
A literature review is a critical and comprehensive analysis of existing literature (published and unpublished works) on a specific topic or research question and provides a synthesis of the current state of knowledge in a particular field. A well-conducted literature review is crucial for researchers to build upon existing knowledge, avoid duplication of efforts, and contribute to the advancement of their field. It also helps researchers situate their work within a broader context and facilitates the development of a sound theoretical and conceptual framework for their studies.
Literature review is a crucial component of research writing, providing a solid background for a research paper’s investigation. The aim is to keep professionals up to date by providing an understanding of ongoing developments within a specific field, including research methods, and experimental techniques used in that field, and present that knowledge in the form of a written report. Also, the depth and breadth of the literature review emphasizes the credibility of the scholar in his or her field.
Before writing a literature review, it’s essential to undertake several preparatory steps to ensure that your review is well-researched, organized, and focused. This includes choosing a topic of general interest to you and doing exploratory research on that topic, writing an annotated bibliography, and noting major points, especially those that relate to the position you have taken on the topic.
Literature reviews and academic research papers are essential components of scholarly work but serve different purposes within the academic realm. 3 A literature review aims to provide a foundation for understanding the current state of research on a particular topic, identify gaps or controversies, and lay the groundwork for future research. Therefore, it draws heavily from existing academic sources, including books, journal articles, and other scholarly publications. In contrast, an academic research paper aims to present new knowledge, contribute to the academic discourse, and advance the understanding of a specific research question. Therefore, it involves a mix of existing literature (in the introduction and literature review sections) and original data or findings obtained through research methods.
Literature reviews are essential components of academic and research papers, and various strategies can be employed to conduct them effectively. If you want to know how to write a literature review for a research paper, here are four common approaches that are often used by researchers. Chronological Review: This strategy involves organizing the literature based on the chronological order of publication. It helps to trace the development of a topic over time, showing how ideas, theories, and research have evolved. Thematic Review: Thematic reviews focus on identifying and analyzing themes or topics that cut across different studies. Instead of organizing the literature chronologically, it is grouped by key themes or concepts, allowing for a comprehensive exploration of various aspects of the topic. Methodological Review: This strategy involves organizing the literature based on the research methods employed in different studies. It helps to highlight the strengths and weaknesses of various methodologies and allows the reader to evaluate the reliability and validity of the research findings. Theoretical Review: A theoretical review examines the literature based on the theoretical frameworks used in different studies. This approach helps to identify the key theories that have been applied to the topic and assess their contributions to the understanding of the subject. It’s important to note that these strategies are not mutually exclusive, and a literature review may combine elements of more than one approach. The choice of strategy depends on the research question, the nature of the literature available, and the goals of the review. Additionally, other strategies, such as integrative reviews or systematic reviews, may be employed depending on the specific requirements of the research.
The literature review format can vary depending on the specific publication guidelines. However, there are some common elements and structures that are often followed. Here is a general guideline for the format of a literature review: Introduction: Provide an overview of the topic. Define the scope and purpose of the literature review. State the research question or objective. Body: Organize the literature by themes, concepts, or chronology. Critically analyze and evaluate each source. Discuss the strengths and weaknesses of the studies. Highlight any methodological limitations or biases. Identify patterns, connections, or contradictions in the existing research. Conclusion: Summarize the key points discussed in the literature review. Highlight the research gap. Address the research question or objective stated in the introduction. Highlight the contributions of the review and suggest directions for future research.
Both annotated bibliographies and literature reviews involve the examination of scholarly sources. While annotated bibliographies focus on individual sources with brief annotations, literature reviews provide a more in-depth, integrated, and comprehensive analysis of existing literature on a specific topic. The key differences are as follows:
Annotated Bibliography | Literature Review | |
Purpose | List of citations of books, articles, and other sources with a brief description (annotation) of each source. | Comprehensive and critical analysis of existing literature on a specific topic. |
Focus | Summary and evaluation of each source, including its relevance, methodology, and key findings. | Provides an overview of the current state of knowledge on a particular subject and identifies gaps, trends, and patterns in existing literature. |
Structure | Each citation is followed by a concise paragraph (annotation) that describes the source’s content, methodology, and its contribution to the topic. | The literature review is organized thematically or chronologically and involves a synthesis of the findings from different sources to build a narrative or argument. |
Length | Typically 100-200 words | Length of literature review ranges from a few pages to several chapters |
Independence | Each source is treated separately, with less emphasis on synthesizing the information across sources. | The writer synthesizes information from multiple sources to present a cohesive overview of the topic. |
References
- Denney, A. S., & Tewksbury, R. (2013). How to write a literature review. Journal of criminal justice education , 24 (2), 218-234.
- Pan, M. L. (2016). Preparing literature reviews: Qualitative and quantitative approaches . Taylor & Francis.
- Cantero, C. (2019). How to write a literature review. San José State University Writing Center .
Paperpal is an AI writing assistant that help academics write better, faster with real-time suggestions for in-depth language and grammar correction. Trained on millions of research manuscripts enhanced by professional academic editors, Paperpal delivers human precision at machine speed.
Try it for free or upgrade to Paperpal Prime , which unlocks unlimited access to premium features like academic translation, paraphrasing, contextual synonyms, consistency checks and more. It’s like always having a professional academic editor by your side! Go beyond limitations and experience the future of academic writing. Get Paperpal Prime now at just US$19 a month!
Related Reads:
- Empirical Research: A Comprehensive Guide for Academics
- How to Write a Scientific Paper in 10 Steps
- How Long Should a Chapter Be?
- How to Use Paperpal to Generate Emails & Cover Letters?
6 Tips for Post-Doc Researchers to Take Their Career to the Next Level
Self-plagiarism in research: what it is and how to avoid it, you may also like, academic integrity vs academic dishonesty: types & examples, dissertation printing and binding | types & comparison , what is a dissertation preface definition and examples , the ai revolution: authors’ role in upholding academic..., the future of academia: how ai tools are..., how to write a research proposal: (with examples..., how to write your research paper in apa..., how to choose a dissertation topic, how to write a phd research proposal, how to write an academic paragraph (step-by-step guide).
Learning and Teaching: Literature Review
- Course Texts
- Action Research
- TESOL, Literacy, and Culture
Literature Review
- Citation Resources
- Award-Winning Book Lists This link opens in a new window
- Publishing Your Work This link opens in a new window
Literature Reviews: An Overview for Graduate Students
1. Definition
Not to be confused with a book review, a literature review surveys scholarly articles, books and other sources (e.g. dissertations, conference proceedings) relevant to a particular issue, area of research, or theory, providing a description, summary, and critical evaluation of each work. The purpose is to offer an overview of significant literature published on a topic.
2. Components
Similar to primary research, development of the literature review requires four stages:
- Problem formulation—which topic or field is being examined and what are its component issues?
- Literature search—finding materials relevant to the subject being explored
- Data evaluation—determining which literature makes a significant contribution to the understanding of the topic
- Analysis and interpretation—discussing the findings and conclusions of pertinent literature
Literature reviews should comprise the following elements:
- An overview of the subject, issue or theory under consideration, along with the objectives of the literature review
- Division of works under review into categories (e.g. those in support of a particular position, those against, and those offering alternative theses entirely)
- Explanation of how each work is similar to and how it varies from the others
- Conclusions as to which pieces are best considered in their argument, are most convincing of their opinions, and make the greatest contribution to the understanding and development of their area of research
In assessing each piece, consideration should be given to:
- Provenance—What are the author's credentials? Are the author's arguments supported by evidence (e.g. primary historical material, case studies, narratives, statistics, recent scientific findings)?
- Objectivity—Is the author's perspective even-handed or prejudicial? Is contrary data considered or is certain pertinent information ignored to prove the author's point?
- Persuasiveness—Which of the author's theses are most/least convincing?
- Value—Are the author's arguments and conclusions convincing? Does the work ultimately contribute in any significant way to an understanding of the subject?
3. Definition and Use/Purpose
A literature review may constitute an essential chapter of a thesis or dissertation, or may be a self-contained review of writings on a subject. In either case, its purpose is to:
- Place each work in the context of its contribution to the understanding of the subject under review
- Describe the relationship of each work to the others under consideration
- Identify new ways to interpret, and shed light on any gaps in, previous research
- Resolve conflicts amongst seemingly contradictory previous studies
- Identify areas of prior scholarship to prevent duplication of effort
- Point the way forward for further research
- Place one's original work (in the case of theses or dissertations) in the context of existing literature
The literature review itself, however, does not present new primary scholarship.
All of the above information was obtained from UC Santa Cruz University Library http://guides.library.ucsc.edu/write-a-literature-review
Selected LitReview Resources at Copley
- << Previous: TESOL, Literacy, and Culture
- Next: Citation Resources >>
- Last Updated: May 22, 2024 2:28 PM
- URL: https://libguides.sandiego.edu/educ
Libraries | Research Guides
Literature reviews, what is a literature review, learning more about how to do a literature review.
- Planning the Review
- The Research Question
- Choosing Where to Search
- Organizing the Review
- Writing the Review
A literature review is a review and synthesis of existing research on a topic or research question. A literature review is meant to analyze the scholarly literature, make connections across writings and identify strengths, weaknesses, trends, and missing conversations. A literature review should address different aspects of a topic as it relates to your research question. A literature review goes beyond a description or summary of the literature you have read.
- Sage Research Methods Core This link opens in a new window SAGE Research Methods supports research at all levels by providing material to guide users through every step of the research process. SAGE Research Methods is the ultimate methods library with more than 1000 books, reference works, journal articles, and instructional videos by world-leading academics from across the social sciences, including the largest collection of qualitative methods books available online from any scholarly publisher. – Publisher
- Next: Planning the Review >>
- Last Updated: Jul 8, 2024 11:22 AM
- URL: https://libguides.northwestern.edu/literaturereviews

An official website of the United States government
The .gov means it’s official. Federal government websites often end in .gov or .mil. Before sharing sensitive information, make sure you’re on a federal government site.
The site is secure. The https:// ensures that you are connecting to the official website and that any information you provide is encrypted and transmitted securely.
- Publications
- Account settings
Preview improvements coming to the PMC website in October 2024. Learn More or Try it out now .
- Advanced Search
- Journal List
- Clinics (Sao Paulo)
Approaching literature review for academic purposes: The Literature Review Checklist
Debora f.b. leite.
I Departamento de Ginecologia e Obstetricia, Faculdade de Ciencias Medicas, Universidade Estadual de Campinas, Campinas, SP, BR
II Universidade Federal de Pernambuco, Pernambuco, PE, BR
III Hospital das Clinicas, Universidade Federal de Pernambuco, Pernambuco, PE, BR
Maria Auxiliadora Soares Padilha
Jose g. cecatti.
A sophisticated literature review (LR) can result in a robust dissertation/thesis by scrutinizing the main problem examined by the academic study; anticipating research hypotheses, methods and results; and maintaining the interest of the audience in how the dissertation/thesis will provide solutions for the current gaps in a particular field. Unfortunately, little guidance is available on elaborating LRs, and writing an LR chapter is not a linear process. An LR translates students’ abilities in information literacy, the language domain, and critical writing. Students in postgraduate programs should be systematically trained in these skills. Therefore, this paper discusses the purposes of LRs in dissertations and theses. Second, the paper considers five steps for developing a review: defining the main topic, searching the literature, analyzing the results, writing the review and reflecting on the writing. Ultimately, this study proposes a twelve-item LR checklist. By clearly stating the desired achievements, this checklist allows Masters and Ph.D. students to continuously assess their own progress in elaborating an LR. Institutions aiming to strengthen students’ necessary skills in critical academic writing should also use this tool.
INTRODUCTION
Writing the literature review (LR) is often viewed as a difficult task that can be a point of writer’s block and procrastination ( 1 ) in postgraduate life. Disagreements on the definitions or classifications of LRs ( 2 ) may confuse students about their purpose and scope, as well as how to perform an LR. Interestingly, at many universities, the LR is still an important element in any academic work, despite the more recent trend of producing scientific articles rather than classical theses.
The LR is not an isolated section of the thesis/dissertation or a copy of the background section of a research proposal. It identifies the state-of-the-art knowledge in a particular field, clarifies information that is already known, elucidates implications of the problem being analyzed, links theory and practice ( 3 - 5 ), highlights gaps in the current literature, and places the dissertation/thesis within the research agenda of that field. Additionally, by writing the LR, postgraduate students will comprehend the structure of the subject and elaborate on their cognitive connections ( 3 ) while analyzing and synthesizing data with increasing maturity.
At the same time, the LR transforms the student and hints at the contents of other chapters for the reader. First, the LR explains the research question; second, it supports the hypothesis, objectives, and methods of the research project; and finally, it facilitates a description of the student’s interpretation of the results and his/her conclusions. For scholars, the LR is an introductory chapter ( 6 ). If it is well written, it demonstrates the student’s understanding of and maturity in a particular topic. A sound and sophisticated LR can indicate a robust dissertation/thesis.
A consensus on the best method to elaborate a dissertation/thesis has not been achieved. The LR can be a distinct chapter or included in different sections; it can be part of the introduction chapter, part of each research topic, or part of each published paper ( 7 ). However, scholars view the LR as an integral part of the main body of an academic work because it is intrinsically connected to other sections ( Figure 1 ) and is frequently present. The structure of the LR depends on the conventions of a particular discipline, the rules of the department, and the student’s and supervisor’s areas of expertise, needs and interests.

Interestingly, many postgraduate students choose to submit their LR to peer-reviewed journals. As LRs are critical evaluations of current knowledge, they are indeed publishable material, even in the form of narrative or systematic reviews. However, systematic reviews have specific patterns 1 ( 8 ) that may not entirely fit with the questions posed in the dissertation/thesis. Additionally, the scope of a systematic review may be too narrow, and the strict criteria for study inclusion may omit important information from the dissertation/thesis. Therefore, this essay discusses the definition of an LR is and methods to develop an LR in the context of an academic dissertation/thesis. Finally, we suggest a checklist to evaluate an LR.
WHAT IS A LITERATURE REVIEW IN A THESIS?
Conducting research and writing a dissertation/thesis translates rational thinking and enthusiasm ( 9 ). While a strong body of literature that instructs students on research methodology, data analysis and writing scientific papers exists, little guidance on performing LRs is available. The LR is a unique opportunity to assess and contrast various arguments and theories, not just summarize them. The research results should not be discussed within the LR, but the postgraduate student tends to write a comprehensive LR while reflecting on his or her own findings ( 10 ).
Many people believe that writing an LR is a lonely and linear process. Supervisors or the institutions assume that the Ph.D. student has mastered the relevant techniques and vocabulary associated with his/her subject and conducts a self-reflection about previously published findings. Indeed, while elaborating the LR, the student should aggregate diverse skills, which mainly rely on his/her own commitment to mastering them. Thus, less supervision should be required ( 11 ). However, the parameters described above might not currently be the case for many students ( 11 , 12 ), and the lack of formal and systematic training on writing LRs is an important concern ( 11 ).
An institutional environment devoted to active learning will provide students the opportunity to continuously reflect on LRs, which will form a dialogue between the postgraduate student and the current literature in a particular field ( 13 ). Postgraduate students will be interpreting studies by other researchers, and, according to Hart (1998) ( 3 ), the outcomes of the LR in a dissertation/thesis include the following:
- To identify what research has been performed and what topics require further investigation in a particular field of knowledge;
- To determine the context of the problem;
- To recognize the main methodologies and techniques that have been used in the past;
- To place the current research project within the historical, methodological and theoretical context of a particular field;
- To identify significant aspects of the topic;
- To elucidate the implications of the topic;
- To offer an alternative perspective;
- To discern how the studied subject is structured;
- To improve the student’s subject vocabulary in a particular field; and
- To characterize the links between theory and practice.
A sound LR translates the postgraduate student’s expertise in academic and scientific writing: it expresses his/her level of comfort with synthesizing ideas ( 11 ). The LR reveals how well the postgraduate student has proceeded in three domains: an effective literature search, the language domain, and critical writing.
Effective literature search
All students should be trained in gathering appropriate data for specific purposes, and information literacy skills are a cornerstone. These skills are defined as “an individual’s ability to know when they need information, to identify information that can help them address the issue or problem at hand, and to locate, evaluate, and use that information effectively” ( 14 ). Librarian support is of vital importance in coaching the appropriate use of Boolean logic (AND, OR, NOT) and other tools for highly efficient literature searches (e.g., quotation marks and truncation), as is the appropriate management of electronic databases.
Language domain
Academic writing must be concise and precise: unnecessary words distract the reader from the essential content ( 15 ). In this context, reading about issues distant from the research topic ( 16 ) may increase students’ general vocabulary and familiarity with grammar. Ultimately, reading diverse materials facilitates and encourages the writing process itself.
Critical writing
Critical judgment includes critical reading, thinking and writing. It supposes a student’s analytical reflection about what he/she has read. The student should delineate the basic elements of the topic, characterize the most relevant claims, identify relationships, and finally contrast those relationships ( 17 ). Each scientific document highlights the perspective of the author, and students will become more confident in judging the supporting evidence and underlying premises of a study and constructing their own counterargument as they read more articles. A paucity of integration or contradictory perspectives indicates lower levels of cognitive complexity ( 12 ).
Thus, while elaborating an LR, the postgraduate student should achieve the highest category of Bloom’s cognitive skills: evaluation ( 12 ). The writer should not only summarize data and understand each topic but also be able to make judgments based on objective criteria, compare resources and findings, identify discrepancies due to methodology, and construct his/her own argument ( 12 ). As a result, the student will be sufficiently confident to show his/her own voice .
Writing a consistent LR is an intense and complex activity that reveals the training and long-lasting academic skills of a writer. It is not a lonely or linear process. However, students are unlikely to be prepared to write an LR if they have not mastered the aforementioned domains ( 10 ). An institutional environment that supports student learning is crucial.
Different institutions employ distinct methods to promote students’ learning processes. First, many universities propose modules to develop behind the scenes activities that enhance self-reflection about general skills (e.g., the skills we have mastered and the skills we need to develop further), behaviors that should be incorporated (e.g., self-criticism about one’s own thoughts), and each student’s role in the advancement of his/her field. Lectures or workshops about LRs themselves are useful because they describe the purposes of the LR and how it fits into the whole picture of a student’s work. These activities may explain what type of discussion an LR must involve, the importance of defining the correct scope, the reasons to include a particular resource, and the main role of critical reading.
Some pedagogic services that promote a continuous improvement in study and academic skills are equally important. Examples include workshops about time management, the accomplishment of personal objectives, active learning, and foreign languages for nonnative speakers. Additionally, opportunities to converse with other students promotes an awareness of others’ experiences and difficulties. Ultimately, the supervisor’s role in providing feedback and setting deadlines is crucial in developing students’ abilities and in strengthening students’ writing quality ( 12 ).
HOW SHOULD A LITERATURE REVIEW BE DEVELOPED?
A consensus on the appropriate method for elaborating an LR is not available, but four main steps are generally accepted: defining the main topic, searching the literature, analyzing the results, and writing ( 6 ). We suggest a fifth step: reflecting on the information that has been written in previous publications ( Figure 2 ).

First step: Defining the main topic
Planning an LR is directly linked to the research main question of the thesis and occurs in parallel to students’ training in the three domains discussed above. The planning stage helps organize ideas, delimit the scope of the LR ( 11 ), and avoid the wasting of time in the process. Planning includes the following steps:
- Reflecting on the scope of the LR: postgraduate students will have assumptions about what material must be addressed and what information is not essential to an LR ( 13 , 18 ). Cooper’s Taxonomy of Literature Reviews 2 systematizes the writing process through six characteristics and nonmutually exclusive categories. The focus refers to the reviewer’s most important points of interest, while the goals concern what students want to achieve with the LR. The perspective assumes answers to the student’s own view of the LR and how he/she presents a particular issue. The coverage defines how comprehensive the student is in presenting the literature, and the organization determines the sequence of arguments. The audience is defined as the group for whom the LR is written.
- Designating sections and subsections: Headings and subheadings should be specific, explanatory and have a coherent sequence throughout the text ( 4 ). They simulate an inverted pyramid, with an increasing level of reflection and depth of argument.
- Identifying keywords: The relevant keywords for each LR section should be listed to guide the literature search. This list should mirror what Hart (1998) ( 3 ) advocates as subject vocabulary . The keywords will also be useful when the student is writing the LR since they guide the reader through the text.
- Delineating the time interval and language of documents to be retrieved in the second step. The most recently published documents should be considered, but relevant texts published before a predefined cutoff year can be included if they are classic documents in that field. Extra care should be employed when translating documents.
Second step: Searching the literature
The ability to gather adequate information from the literature must be addressed in postgraduate programs. Librarian support is important, particularly for accessing difficult texts. This step comprises the following components:
- Searching the literature itself: This process consists of defining which databases (electronic or dissertation/thesis repositories), official documents, and books will be searched and then actively conducting the search. Information literacy skills have a central role in this stage. While searching electronic databases, controlled vocabulary (e.g., Medical Subject Headings, or MeSH, for the PubMed database) or specific standardized syntax rules may need to be applied.
In addition, two other approaches are suggested. First, a review of the reference list of each document might be useful for identifying relevant publications to be included and important opinions to be assessed. This step is also relevant for referencing the original studies and leading authors in that field. Moreover, students can directly contact the experts on a particular topic to consult with them regarding their experience or use them as a source of additional unpublished documents.
Before submitting a dissertation/thesis, the electronic search strategy should be repeated. This process will ensure that the most recently published papers will be considered in the LR.
- Selecting documents for inclusion: Generally, the most recent literature will be included in the form of published peer-reviewed papers. Assess books and unpublished material, such as conference abstracts, academic texts and government reports, are also important to assess since the gray literature also offers valuable information. However, since these materials are not peer-reviewed, we recommend that they are carefully added to the LR.
This task is an important exercise in time management. First, students should read the title and abstract to understand whether that document suits their purposes, addresses the research question, and helps develop the topic of interest. Then, they should scan the full text, determine how it is structured, group it with similar documents, and verify whether other arguments might be considered ( 5 ).
Third step: Analyzing the results
Critical reading and thinking skills are important in this step. This step consists of the following components:
- Reading documents: The student may read various texts in depth according to LR sections and subsections ( defining the main topic ), which is not a passive activity ( 1 ). Some questions should be asked to practice critical analysis skills, as listed below. Is the research question evident and articulated with previous knowledge? What are the authors’ research goals and theoretical orientations, and how do they interact? Are the authors’ claims related to other scholars’ research? Do the authors consider different perspectives? Was the research project designed and conducted properly? Are the results and discussion plausible, and are they consistent with the research objectives and methodology? What are the strengths and limitations of this work? How do the authors support their findings? How does this work contribute to the current research topic? ( 1 , 19 )
- Taking notes: Students who systematically take notes on each document are more readily able to establish similarities or differences with other documents and to highlight personal observations. This approach reinforces the student’s ideas about the next step and helps develop his/her own academic voice ( 1 , 13 ). Voice recognition software ( 16 ), mind maps ( 5 ), flowcharts, tables, spreadsheets, personal comments on the referenced texts, and note-taking apps are all available tools for managing these observations, and the student him/herself should use the tool that best improves his/her learning. Additionally, when a student is considering submitting an LR to a peer-reviewed journal, notes should be taken on the activities performed in all five steps to ensure that they are able to be replicated.
Fourth step: Writing
The recognition of when a student is able and ready to write after a sufficient period of reading and thinking is likely a difficult task. Some students can produce a review in a single long work session. However, as discussed above, writing is not a linear process, and students do not need to write LRs according to a specific sequence of sections. Writing an LR is a time-consuming task, and some scholars believe that a period of at least six months is sufficient ( 6 ). An LR, and academic writing in general, expresses the writer’s proper thoughts, conclusions about others’ work ( 6 , 10 , 13 , 16 ), and decisions about methods to progress in the chosen field of knowledge. Thus, each student is expected to present a different learning and writing trajectory.
In this step, writing methods should be considered; then, editing, citing and correct referencing should complete this stage, at least temporarily. Freewriting techniques may be a good starting point for brainstorming ideas and improving the understanding of the information that has been read ( 1 ). Students should consider the following parameters when creating an agenda for writing the LR: two-hour writing blocks (at minimum), with prespecified tasks that are possible to complete in one section; short (minutes) and long breaks (days or weeks) to allow sufficient time for mental rest and reflection; and short- and long-term goals to motivate the writing itself ( 20 ). With increasing experience, this scheme can vary widely, and it is not a straightforward rule. Importantly, each discipline has a different way of writing ( 1 ), and each department has its own preferred styles for citations and references.
Fifth step: Reflecting on the writing
In this step, the postgraduate student should ask him/herself the same questions as in the analyzing the results step, which can take more time than anticipated. Ambiguities, repeated ideas, and a lack of coherence may not be noted when the student is immersed in the writing task for long periods. The whole effort will likely be a work in progress, and continuous refinements in the written material will occur once the writing process has begun.
LITERATURE REVIEW CHECKLIST
In contrast to review papers, the LR of a dissertation/thesis should not be a standalone piece or work. Instead, it should present the student as a scholar and should maintain the interest of the audience in how that dissertation/thesis will provide solutions for the current gaps in a particular field.
A checklist for evaluating an LR is convenient for students’ continuous academic development and research transparency: it clearly states the desired achievements for the LR of a dissertation/thesis. Here, we present an LR checklist developed from an LR scoring rubric ( 11 ). For a critical analysis of an LR, we maintain the five categories but offer twelve criteria that are not scaled ( Figure 3 ). The criteria all have the same importance and are not mutually exclusive.

First category: Coverage
1. justified criteria exist for the inclusion and exclusion of literature in the review.
This criterion builds on the main topic and areas covered by the LR ( 18 ). While experts may be confident in retrieving and selecting literature, postgraduate students must convince their audience about the adequacy of their search strategy and their reasons for intentionally selecting what material to cover ( 11 ). References from different fields of knowledge provide distinct perspective, but narrowing the scope of coverage may be important in areas with a large body of existing knowledge.
Second category: Synthesis
2. a critical examination of the state of the field exists.
A critical examination is an assessment of distinct aspects in the field ( 1 ) along with a constructive argument. It is not a negative critique but an expression of the student’s understanding of how other scholars have added to the topic ( 1 ), and the student should analyze and contextualize contradictory statements. A writer’s personal bias (beliefs or political involvement) have been shown to influence the structure and writing of a document; therefore, the cultural and paradigmatic background guide how the theories are revised and presented ( 13 ). However, an honest judgment is important when considering different perspectives.
3. The topic or problem is clearly placed in the context of the broader scholarly literature
The broader scholarly literature should be related to the chosen main topic for the LR ( how to develop the literature review section). The LR can cover the literature from one or more disciplines, depending on its scope, but it should always offer a new perspective. In addition, students should be careful in citing and referencing previous publications. As a rule, original studies and primary references should generally be included. Systematic and narrative reviews present summarized data, and it may be important to cite them, particularly for issues that should be understood but do not require a detailed description. Similarly, quotations highlight the exact statement from another publication. However, excessive referencing may disclose lower levels of analysis and synthesis by the student.
4. The LR is critically placed in the historical context of the field
Situating the LR in its historical context shows the level of comfort of the student in addressing a particular topic. Instead of only presenting statements and theories in a temporal approach, which occasionally follows a linear timeline, the LR should authentically characterize the student’s academic work in the state-of-art techniques in their particular field of knowledge. Thus, the LR should reinforce why the dissertation/thesis represents original work in the chosen research field.
5. Ambiguities in definitions are considered and resolved
Distinct theories on the same topic may exist in different disciplines, and one discipline may consider multiple concepts to explain one topic. These misunderstandings should be addressed and contemplated. The LR should not synthesize all theories or concepts at the same time. Although this approach might demonstrate in-depth reading on a particular topic, it can reveal a student’s inability to comprehend and synthesize his/her research problem.
6. Important variables and phenomena relevant to the topic are articulated
The LR is a unique opportunity to articulate ideas and arguments and to purpose new relationships between them ( 10 , 11 ). More importantly, a sound LR will outline to the audience how these important variables and phenomena will be addressed in the current academic work. Indeed, the LR should build a bidirectional link with the remaining sections and ground the connections between all of the sections ( Figure 1 ).
7. A synthesized new perspective on the literature has been established
The LR is a ‘creative inquiry’ ( 13 ) in which the student elaborates his/her own discourse, builds on previous knowledge in the field, and describes his/her own perspective while interpreting others’ work ( 13 , 17 ). Thus, students should articulate the current knowledge, not accept the results at face value ( 11 , 13 , 17 ), and improve their own cognitive abilities ( 12 ).
Third category: Methodology
8. the main methodologies and research techniques that have been used in the field are identified and their advantages and disadvantages are discussed.
The LR is expected to distinguish the research that has been completed from investigations that remain to be performed, address the benefits and limitations of the main methods applied to date, and consider the strategies for addressing the expected limitations described above. While placing his/her research within the methodological context of a particular topic, the LR will justify the methodology of the study and substantiate the student’s interpretations.
9. Ideas and theories in the field are related to research methodologies
The audience expects the writer to analyze and synthesize methodological approaches in the field. The findings should be explained according to the strengths and limitations of previous research methods, and students must avoid interpretations that are not supported by the analyzed literature. This criterion translates to the student’s comprehension of the applicability and types of answers provided by different research methodologies, even those using a quantitative or qualitative research approach.
Fourth category: Significance
10. the scholarly significance of the research problem is rationalized.
The LR is an introductory section of a dissertation/thesis and will present the postgraduate student as a scholar in a particular field ( 11 ). Therefore, the LR should discuss how the research problem is currently addressed in the discipline being investigated or in different disciplines, depending on the scope of the LR. The LR explains the academic paradigms in the topic of interest ( 13 ) and methods to advance the field from these starting points. However, an excess number of personal citations—whether referencing the student’s research or studies by his/her research team—may reflect a narrow literature search and a lack of comprehensive synthesis of ideas and arguments.
11. The practical significance of the research problem is rationalized
The practical significance indicates a student’s comprehensive understanding of research terminology (e.g., risk versus associated factor), methodology (e.g., efficacy versus effectiveness) and plausible interpretations in the context of the field. Notably, the academic argument about a topic may not always reflect the debate in real life terms. For example, using a quantitative approach in epidemiology, statistically significant differences between groups do not explain all of the factors involved in a particular problem ( 21 ). Therefore, excessive faith in p -values may reflect lower levels of critical evaluation of the context and implications of a research problem by the student.

Fifth category: Rhetoric
12. the lr was written with a coherent, clear structure that supported the review.
This category strictly relates to the language domain: the text should be coherent and presented in a logical sequence, regardless of which organizational ( 18 ) approach is chosen. The beginning of each section/subsection should state what themes will be addressed, paragraphs should be carefully linked to each other ( 10 ), and the first sentence of each paragraph should generally summarize the content. Additionally, the student’s statements are clear, sound, and linked to other scholars’ works, and precise and concise language that follows standardized writing conventions (e.g., in terms of active/passive voice and verb tenses) is used. Attention to grammar, such as orthography and punctuation, indicates prudence and supports a robust dissertation/thesis. Ultimately, all of these strategies provide fluency and consistency for the text.
Although the scoring rubric was initially proposed for postgraduate programs in education research, we are convinced that this checklist is a valuable tool for all academic areas. It enables the monitoring of students’ learning curves and a concentrated effort on any criteria that are not yet achieved. For institutions, the checklist is a guide to support supervisors’ feedback, improve students’ writing skills, and highlight the learning goals of each program. These criteria do not form a linear sequence, but ideally, all twelve achievements should be perceived in the LR.
CONCLUSIONS
A single correct method to classify, evaluate and guide the elaboration of an LR has not been established. In this essay, we have suggested directions for planning, structuring and critically evaluating an LR. The planning of the scope of an LR and approaches to complete it is a valuable effort, and the five steps represent a rational starting point. An institutional environment devoted to active learning will support students in continuously reflecting on LRs, which will form a dialogue between the writer and the current literature in a particular field ( 13 ).
The completion of an LR is a challenging and necessary process for understanding one’s own field of expertise. Knowledge is always transitory, but our responsibility as scholars is to provide a critical contribution to our field, allowing others to think through our work. Good researchers are grounded in sophisticated LRs, which reveal a writer’s training and long-lasting academic skills. We recommend using the LR checklist as a tool for strengthening the skills necessary for critical academic writing.
AUTHOR CONTRIBUTIONS
Leite DFB has initially conceived the idea and has written the first draft of this review. Padilha MAS and Cecatti JG have supervised data interpretation and critically reviewed the manuscript. All authors have read the draft and agreed with this submission. Authors are responsible for all aspects of this academic piece.
ACKNOWLEDGMENTS
We are grateful to all of the professors of the ‘Getting Started with Graduate Research and Generic Skills’ module at University College Cork, Cork, Ireland, for suggesting and supporting this article. Funding: DFBL has granted scholarship from Brazilian Federal Agency for Support and Evaluation of Graduate Education (CAPES) to take part of her Ph.D. studies in Ireland (process number 88881.134512/2016-01). There is no participation from sponsors on authors’ decision to write or to submit this manuscript.
No potential conflict of interest was reported.
1 The questions posed in systematic reviews usually follow the ‘PICOS’ acronym: Population, Intervention, Comparison, Outcomes, Study design.
2 In 1988, Cooper proposed a taxonomy that aims to facilitate students’ and institutions’ understanding of literature reviews. Six characteristics with specific categories are briefly described: Focus: research outcomes, research methodologies, theories, or practices and applications; Goals: integration (generalization, conflict resolution, and linguistic bridge-building), criticism, or identification of central issues; Perspective: neutral representation or espousal of a position; Coverage: exhaustive, exhaustive with selective citations, representative, central or pivotal; Organization: historical, conceptual, or methodological; and Audience: specialized scholars, general scholars, practitioners or policymakers, or the general public.
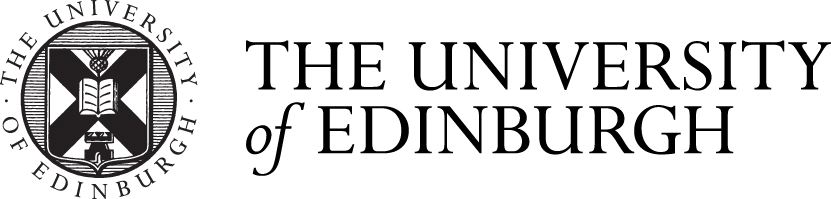
- Schools & departments
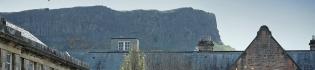
Literature review
A general guide on how to conduct and write a literature review.
Please check course or programme information and materials provided by teaching staff, including your project supervisor, for subject-specific guidance.
What is a literature review?
A literature review is a piece of academic writing demonstrating knowledge and understanding of the academic literature on a specific topic placed in context. A literature review also includes a critical evaluation of the material; this is why it is called a literature review rather than a literature report. It is a process of reviewing the literature, as well as a form of writing.
To illustrate the difference between reporting and reviewing, think about television or film review articles. These articles include content such as a brief synopsis or the key points of the film or programme plus the critic’s own evaluation. Similarly the two main objectives of a literature review are firstly the content covering existing research, theories and evidence, and secondly your own critical evaluation and discussion of this content.
Usually a literature review forms a section or part of a dissertation, research project or long essay. However, it can also be set and assessed as a standalone piece of work.
What is the purpose of a literature review?
…your task is to build an argument, not a library. Rudestam, K.E. and Newton, R.R. (1992)Surviving your dissertation: A comprehensive guide to content and process. California: Sage, p49.
In a larger piece of written work, such as a dissertation or project, a literature review is usually one of the first tasks carried out after deciding on a topic. Reading combined with critical analysis can help to refine a topic and frame research questions. Conducting a literature review establishes your familiarity with and understanding of current research in a particular field before carrying out a new investigation. After doing a literature review, you should know what research has already been done and be able to identify what is unknown within your topic.
When doing and writing a literature review, it is good practice to:
- summarise and analyse previous research and theories;
- identify areas of controversy and contested claims;
- highlight any gaps that may exist in research to date.
Conducting a literature review
Focusing on different aspects of your literature review can be useful to help plan, develop, refine and write it. You can use and adapt the prompt questions in our worksheet below at different points in the process of researching and writing your review. These are suggestions to get you thinking and writing.
Developing and refining your literature review (pdf)
Developing and refining your literature review (Word)
Writing a literature review has a lot in common with other assignment tasks. There is advice on our other pages about thinking critically, reading strategies and academic writing. Our literature review top tips suggest some specific things you can do to help you submit a successful review.
Literature review top tips (pdf)
Literature review top tips (Word rtf)
Our reading page includes strategies and advice on using books and articles and a notes record sheet grid you can use.
Reading at university
The Academic writing page suggests ways to organise and structure information from a range of sources and how you can develop your argument as you read and write.
Academic writing
The Critical thinking page has advice on how to be a more critical researcher and a form you can use to help you think and break down the stages of developing your argument.
Critical thinking
As with other forms of academic writing, your literature review needs to demonstrate good academic practice by following the Code of Student Conduct and acknowledging the work of others through citing and referencing your sources.
Good academic practice
As with any writing task, you will need to review, edit and rewrite sections of your literature review. The Editing and proofreading page includes tips on how to do this and strategies for standing back and thinking about your structure and checking the flow of your argument.
Editing and proofreading
Guidance on literature searching from the University Library
The Academic Support Librarians have developed LibSmart I and II, Learn courses to help you develop and enhance your digital research skills and capabilities; from getting started with the Library to managing data for your dissertation.
Searching using the library’s DiscoverEd tool: DiscoverEd
Finding resources in your subject: Subject guides
The Academic Support Librarians also provide one-to-one appointments to help you develop your research strategies.
1 to 1 support for literature searching and systematic reviews
Advice to help you optimise use of Google Scholar, Google Books and Google for your research and study: Using Google
Managing and curating your references
A referencing management tool can help you to collect and organise and your source material to produce a bibliography or reference list.
Referencing and reference management
Information Services provide access to Cite them right online which is a guide to the main referencing systems and tells you how to reference just about any source (EASE log-in may be required).
Cite them right
Published study guides
There are a number of scholarship skills books and guides available which can help with writing a literature review. Our Resource List of study skills guides includes sections on Referencing, Dissertation and project writing and Literature reviews.
Study skills guides
This article was published on 2024-02-26
- Research article
- Open access
- Published: 15 February 2021
Systematic literature review of machine learning methods used in the analysis of real-world data for patient-provider decision making
- Alan Brnabic 1 &
- Lisa M. Hess ORCID: orcid.org/0000-0003-3631-3941 2
BMC Medical Informatics and Decision Making volume 21 , Article number: 54 ( 2021 ) Cite this article
31k Accesses
57 Citations
3 Altmetric
Metrics details
Machine learning is a broad term encompassing a number of methods that allow the investigator to learn from the data. These methods may permit large real-world databases to be more rapidly translated to applications to inform patient-provider decision making.
This systematic literature review was conducted to identify published observational research of employed machine learning to inform decision making at the patient-provider level. The search strategy was implemented and studies meeting eligibility criteria were evaluated by two independent reviewers. Relevant data related to study design, statistical methods and strengths and limitations were identified; study quality was assessed using a modified version of the Luo checklist.
A total of 34 publications from January 2014 to September 2020 were identified and evaluated for this review. There were diverse methods, statistical packages and approaches used across identified studies. The most common methods included decision tree and random forest approaches. Most studies applied internal validation but only two conducted external validation. Most studies utilized one algorithm, and only eight studies applied multiple machine learning algorithms to the data. Seven items on the Luo checklist failed to be met by more than 50% of published studies.
Conclusions
A wide variety of approaches, algorithms, statistical software, and validation strategies were employed in the application of machine learning methods to inform patient-provider decision making. There is a need to ensure that multiple machine learning approaches are used, the model selection strategy is clearly defined, and both internal and external validation are necessary to be sure that decisions for patient care are being made with the highest quality evidence. Future work should routinely employ ensemble methods incorporating multiple machine learning algorithms.
Peer Review reports
Traditional methods of analyzing large real-world databases (big data) and other observational studies are focused on the outcomes that can inform at the population-based level. The findings from real-world studies are relevant to populations as a whole, but the ability to predict or provide meaningful evidence at the patient level is much less well established due to the complexity with which clinical decision making is made and the variety of factors taken into account by the health care provider [ 1 , 2 ]. Using traditional methods that produce population estimates and measures of variability, it is very challenging to accurately predict how any one patient will perform, even when applying findings from subgroup analyses. The care of patients is nuanced, and multiple non-linear, interconnected factors must be taken into account in decision making. When data are available that are only relevant at the population level, health care decision making is less informed as to the optimal course of care for a given patient.
Clinical prediction models are an approach to utilizing patient-level evidence to help inform healthcare decision makers about patient care. These models are also known as prediction rules or prognostic models and have been used for decades by health care professionals [ 3 ]. Traditionally, these models combine patient demographic, clinical and treatment characteristics in the form of a statistical or mathematical model, usually regression, classification or neural networks, but deal with a limited number of predictor variables (usually below 25). The Framingham Heart Study is a classic example of the use of longitudinal data to build a traditional decision-making model. Multiple risk calculators and estimators have been built to predict a patient’s risk of a variety of cardiovascular outcomes, such as atrial fibrillation and coronary heart disease [ 4 , 5 , 6 ]. In general, these studies use multivariable regression evaluating risk factors identified in the literature. Based on these findings, a scoring system is derived for each factor to predict the likelihood of an adverse outcome based on a patient’s score across all risk factors evaluated.
With the advent of more complex data collection and readily available data sets for patients in routine clinical care, both sample sizes and potential predictor variables (such as genomic data) can exceed the tens of thousands, thus establishing the need for alternative approaches to rapidly process a large amount of information. Artificial intelligence (AI), particularly machine learning methods (a subset of AI), are increasingly being utilized in clinical research for prediction models, pattern recognition and deep-learning techniques used to combine complex information for example genomic and clinical data [ 7 , 8 , 9 ]. In the health care sciences, these methods are applied to replace a human expert to perform tasks that would otherwise take considerable time and expertise, and likely result in potential error. The underlying concept is that a machine will learn by trial and error from the data itself, to make predictions without having a pre-defined set of rules for decision making. Simply, machine learning can simply be better understood as “learning from data.” [ 8 ].
There are two types of learning from the data, unsupervised and supervised. Unsupervised learning is a type of machine learning algorithm used to draw inferences from datasets consisting of input data without labelled responses. The most common unsupervised learning method is cluster analysis, which is used for exploratory data analysis to find hidden patterns or grouping in data. Supervised learning involves making a prediction based on a set of pre-specified input and output variables. There are a number of statistical tools used for supervised learning. Some examples include traditional statistical prediction methods like regression models (e.g. regression splines, projection pursuit regression, penalized regression) that involve fitting a model to data, evaluating the fit and estimating parameters that are later used in a predictive equation. Other tools include tree-based methods (e.g. classification and regression trees [CART] and random forests), which successively partition a data set based on the relationships between predictor variables and a target (outcome) variable. Other examples include neural networks, discriminant functions and linear classifiers, support vector classifiers and machines. Often, predictive tools are built using various forms of model aggregation (or ensemble learning) that may combine models based on resampled or re-weighted data sets. These different types of models can be fitted to the same data using model averaging.
Classical statistical regression methods used for prediction modeling are well understood in the statistical sciences and the scientific community that employs them. These methods tend to be transparent and are usually hypothesis driven but can overlook complex associations with limited flexibility when a high number of variables are investigated. In addition, when using classic regression modeling, choosing the ‘right’ model is not straightforward. Non-traditional machine learning algorithms, and machine learning approaches, may overcome some of these limitations of classical regression models in this new era of big data, but are not a complete solution as they must be considered in the context of the limitations of data used in the analysis [ 2 ].
While machine learning methods can be used for both population-based models as well as for informed patient-provider decision making, it is important to note that the data, model, and outputs used to inform the care of an individual patient must meet the highest standards of research quality, as the choice made will likely have an impact on both the long- and short-term patient outcomes. While a range of uncertainty can be expected for population-based estimates, the risk of error for patient level models must be minimized to ensure quality patient care. The risks and concerns of utilizing machine learning for individual patient decision making have been raised by ethicists [ 10 ]. The risks are not limited to the lack of transparency, limited data regarding the confidence of the findings, and the risk of reducing patient autonomy in choice by relying on data that may foster a more paternalistic model of healthcare. These are all important and valid concerns, and therefore the role of machine learning for patient care must meet the highest standards to ensure that shared, not simply informed, evidence-based decision making be supported by these methods.
A systematic literature review was published in 2018 that evaluated the statistical methods that have been used to enable large, real-world databases to be used at the patient-provider level [ 11 ]. Briefly, this study identified a total of 115 articles that evaluated the use of logistic regression (n = 52, 45.2%), Cox regression (n = 24, 20.9%), and linear regression (n = 17, 14.8%). However, an interesting observation noted several studies utilizing novel statistical approaches such as machine learning, recursive partitioning, and development of mathematical algorithms to predict patient outcomes. More recently, publications are emerging describing the use of Individualized Treatment Recommendation algorithms and Outcome Weighted Learning for personalized medicine using large observational databases [ 12 , 13 ]. Therefore, this systematic literature review was designed to further pursue this observation to more comprehensively evaluate the use of machine learning methods to support patient-provider decision making, and to critically evaluate the strengths and weaknesses of these methods. For the purposes of this work, data supporting patient-provider decision making was defined as that which provided information specifically on a treatment or intervention choice; while both population-based and risk estimator data are certainly valuable for patient care and decision making, this study was designed to evaluate data that would specifically inform a choice for the patient with the provider. The overarching goal is to provide evidence of how large datasets can be used to inform decisions at the patient level using machine learning-based methods, and to evaluate the quality of such work to support informed decision making.
This study originated from a systematic literature review that was conducted in MEDLINE and PsychInfo; a refreshed search was conducted in September 2020 to obtain newer publications (Table 1 ). Eligible studies were those that analyzed prospective or retrospective observational data, reported quantitative results, and described statistical methods specifically applicable to patient-level decision making. Specifically, patient-level decision making referred to studies that provided data for or against a particular intervention at the patient level, so that the data could be used to inform decision making at the patient-provider level. Studies did not meet this criterion if only a population-based estimates, mortality risk predictors, or satisfaction with care were evaluated. Additionally, studies designed to improve diagnostic tools and those evaluating health care system quality indicators did not meet the patient-provider decision-making criterion. Eligible statistical methods for this study were limited to machine learning-based approaches. Eligibility was assessed by two reviewers and any discrepancies were discussed; a third reviewer was available to serve as a tie breaker in case of different opinions. The final set of eligible publications were then abstracted into a Microsoft Excel document. Study quality was evaluated using a modified Luo scale, which was developed specifically as a tool to standardize high-quality publication of machine learning models [ 14 ]. A modified version of this tool was utilized for this study; specifically, the optional item were removed, and three terms were clarified: item 6 (define the prediction problem) was redefined as “define the model,” item 7 (prepare data for model building) was renamed “model building and validation,” and item 8 (build the predictive model) was renamed “model selection” to more succinctly state what was being evaluated under each criterion. Data were abstracted and both extracted data and the Luo checklist items were reviewed and verified by a second reviewer to ensure data comprehensiveness and quality. In all cases of differences in eligibility assessment or data entry, the reviewers met and ensured agreement with the final set of data to be included in the database for data synthesis, with a third reviewer utilized as a tie breaker in case of discrepancies. Data were summarized descriptively and qualitatively, based on the following categories: publication and study characteristics; patient characteristics; statistical methodologies used, including statistical software packages; strengths and weaknesses; and interpretation of findings.
The search strategy was run on September 1, 2020 and identified a total of 34 publications that utilized machine learning methods for individual patient-level decision making (Fig. 1 ). The most common reason for study exclusion, as expected, was due to the study not meeting the patient-level decision making criterion. A summary of the characteristics of eligible studies and the patient data are included in Table 2 . Most of the real-world data sources included retrospective databases or designs (n = 27, 79.4%), primarily utilizing electronic health records. Six analyses utilized prospective cohort studies and one utilized data from a cross sectional study.
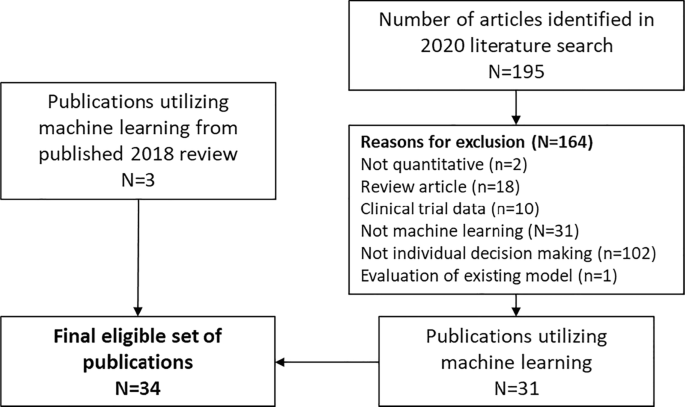
Prisma diagram of screening and study identification
General approaches to machine learning
The types of classification or prediction machine learning algorithms are reported in Table 2 . These included decision tree/random forest analyses (19 studies) [ 15 , 16 , 17 , 18 , 19 , 20 , 21 , 22 , 23 , 24 , 25 , 26 , 27 , 28 , 29 , 30 , 31 , 32 , 33 ] and neural networks (19 studies) [ 24 , 25 , 26 , 27 , 28 , 29 , 30 , 32 , 34 , 35 , 36 , 37 , 38 , 39 , 40 , 41 , 42 , 43 , 44 ]. Other approaches included latent growth mixture modeling [ 45 ], support vector machine classifiers [ 46 ], LASSO regression [ 47 ], boosting methods [ 23 ], and a novel Bayesian approach [ 26 , 40 , 48 ]. Within the analytical approaches to support machine learning, a variety of methods were used to evaluate model fit, such as Akaike Information Criterion, Bayesian Information Criterion, and the Lo-Mendel-Rubin likelihood ratio test [ 22 , 45 , 47 ], and while most studies included the area under the curve (AUC) of receiver-operator characteristic (ROC) curves (Table 3 ), analyses also included sensitivity/specificity [ 16 , 19 , 24 , 30 , 41 , 42 , 43 ], positive predictive value [ 21 , 26 , 32 , 38 , 40 , 41 , 42 , 43 ], and a variety of less common approaches such as the geometric mean [ 16 ], use of the Matthews correlation coefficient (ranges from -1.0, completely erroneous information, to + 1.0, perfect prediction) [ 46 ], defining true/false negatives/positives by means of a confusion matrix [ 17 ], calculating the root mean square error of the predicted versus original outcome profiles [ 37 ], or identifying the model with the best average performance training and performance cross validation [ 36 ].
Statistical software packages
The statistical programs used to perform machine learning varied widely across these studies, no consistencies were observed (Table 2 ). As noted above, one study using decision tree analysis used Quinlan’s C5.0 decision tree algorithm [ 15 ] while a second used an earlier version of this program (C4.5) [ 20 ]. Other decision tree analyses utilized various versions of R [ 18 , 19 , 22 , 24 , 27 , 47 ], International Business Machines (IBM) Statistical Package for the Social Sciences (SPSS) [ 16 , 17 , 33 , 47 ], the Azure Machine Learning Platform [ 30 ], or programmed the model using Python [ 23 , 25 , 46 ]. Artificial neural network analyses used Neural Designer [ 34 ] or Statistica V10 [ 35 ]. Six studies did not report the software used for analysis [ 21 , 31 , 32 , 37 , 41 , 42 ].
Families of machine learning algorithms
Also as summarized in Table 2 , more than one third of all publications (n = 13, 38.2%) applied only one family of machine learning algorithm to model development [ 16 , 17 , 18 , 19 , 20 , 34 , 37 , 41 , 42 , 43 , 46 , 48 ]; and only four studies utilized five or more methods [ 23 , 25 , 28 , 45 ]. One applied an ensemble of six different algorithms and the software was set to run 200 iterations [ 23 ], and another ran seven algorithms [ 45 ].
Internal and external validation
Evaluation of study publication quality identified the most common gap in publications as the lack of external validation, which was conducted by only two studies [ 15 , 20 ]. Seven studies predefined the success criteria for model performance [ 20 , 21 , 23 , 35 , 36 , 46 , 47 ], and five studies discussed the generalizability of the model [ 20 , 23 , 34 , 45 , 48 ]. Six studies [ 17 , 18 , 21 , 22 , 35 , 36 ] discussed the balance between model accuracy and model simplicity or interpretability, which was also a criterion of quality publication in the Luo scale [ 14 ]. The items on the checklist that were least frequently met are presented in Fig. 2 . The complete quality assessment evaluation for each item in the checklist is included in Additional file 1 : Table S1.
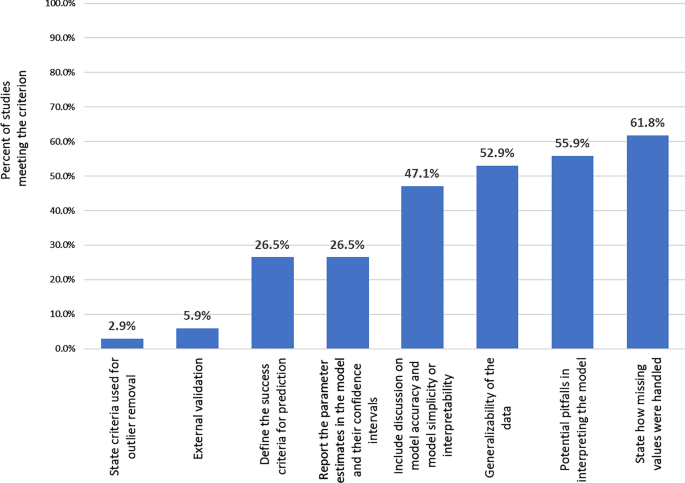
Least frequently met study quality items, modified Luo Scale [ 14 ]
There were a variety of approaches taken to validate the models developed (Table 3 ). Internal validation with splitting into a testing and validation dataset was performed in all studies. The cohort splitting approach was conducted in multiple ways, using a 2:1 split [ 26 ], 60/40 split [ 21 , 36 ], a 70/30 split [ 16 , 17 , 22 , 30 , 33 , 35 ], 75/25 split [ 27 , 40 ], 80/20 split [ 46 ], 90/10 split [ 25 , 29 ], splitting the data based on site of care [ 48 ], a 2/1/1 split for training, testing and validation [ 38 ], and splitting 60/20/20, where the third group was selected for model selection purposes prior to validation [ 34 ]. Nine studies did not specifically mention the form of splitting approach used [ 15 , 18 , 19 , 20 , 24 , 29 , 39 , 45 , 47 ], but most of those noted the use of k fold cross validation. One training set corresponded to 90% of the sample [ 23 ], whereas a second study was less clear, as input data were at the observation level with multiple observations per patient, and 3 of the 15 patients were included in the training set [ 37 ]. The remaining studies did not specifically state splitting the data into testing and validation samples, but most specified they performed five-fold cross validation (including one that generally mentioned cohort splitting) [ 18 , 45 ] or ten-fold cross validation strategies [ 15 , 19 , 20 , 28 ].
External validation was conducted by only two studies (5.9%). Hische and colleagues conducted a decision tree analysis, which was designed to identify patients with impaired fasting glucose [ 20 ]. Their model was developed in a cohort study of patients from the Berlin Potsdam Cohort Study (n = 1527) and was found to have a positive predictive value of 56.2% and a negative predictive value of 89.1%. The model was then tested on an independent from the Dresden Cohort (n = 1998) with a family history of type II diabetes. In external validation, positive predictive value was 43.9% and negative predictive value was 90.4% [ 20 ]. Toussi and colleagues conducted both internal and external validation in their decision tree analysis to evaluate individual physician prescribing behaviors using a database of 463 patient electronic medical records [ 15 ]. For the internal validation step, the cross-validation option was used from Quinlan’s C5.0 decision tree learning algorithm as their study sample was too small to split into a testing and validation sample, and external validation was conducted by comparing outcomes to published treatment guidelines. Unfortunately, they found little concordance between physician behavior and guidelines potentially due to the timing of the data not matching the time period in which guidelines were implemented, emphasizing the need for a contemporaneous external control [ 15 ].
Handling of missing values
Missing values were addressed in most studies (n = 21, 61.8%) in this review, but there were thirteen remaining studies that did not mention if there were missing data or how they were handled (Table 3 ). For those that reported methods related to missing data, there were a wide variety of approaches used in real-world datasets. The full information maximum likelihood method was used for estimating model parameters in the presence of missing data for the development of the model by Hertroijs and colleagues, but patients with missing covariate values at baseline were excluded from the validation of the model [ 45 ]. Missing covariate values were included in models as a discrete category [ 48 ]. Four studies removed patients from the model with missing data [ 46 ], resulting in the loss of 16%-41% of samples in three studies [ 17 , 36 , 47 ]. Missing data from primary outcome variables were reported among with 59% (men) and 70% (women) within a study of diabetes [ 16 ]. In this study, single imputation was used; for continuous variables CART (IBM SPSS modeler V14.2.03) and for categorical variables the authors used the weighted K-Nearest Neighbor approach using RapidMiner (V.5) [ 16 ]. Other studies reported exclusion but not specifically the impact on sample size [ 29 , 31 , 38 , 44 ]. Imputation was conducted in a variety of ways for studies with missing data [ 22 , 25 , 28 , 33 ]. Single imputation was used in the study by Bannister and colleagues, but followed by multiple imputation in the final model to evaluate differences in model parameters [ 22 ]. One study imputed with a standard last-imputation-forward approach [ 26 ]. Spline techniques were used to impute missing data in the training set of one study [ 37 ]. Missingness was largely retained as an informative variable, and only variables missing for 85% or more of participants were excluded by Alaa et al. [ 23 ] while Hearn et al. used a combination of imputation and exclusion strategies [ 40 ]. Lastly, missing or incomplete data were imputed using a model-based approach by Toussi et al. [ 15 ] and using an optimal-impute algorithm by Bertsimas et al. [ 21 ].
Strengths and weaknesses noted by authors
Publications summarized the strengths and weaknesses of the machine learning methods employed. Low complexity and simplicity of machine-based learning models were noted as strengths of this approach [ 15 , 20 ]. Machine learning approaches were both powerful and efficient methods to apply to large datasets [ 19 ]. It was noted that parameters in this study that were significant at the patient level were included, even if at the broader population-based level using traditional regression analysis model development they would have not been significant and therefore would have been otherwise excluded using traditional approaches [ 34 ]. One publication noted the value of machine learning being highly dependent on the model selection strategy and parameter optimization, and that machine learning in and of itself will not provide better estimates unless these steps are conducted properly [ 23 ].
Even when properly planned, machine learning approaches are not without issues that deserve attention in future studies that employ these techniques. Within the eligible publications, weaknesses included overfitting the model with the inclusion of too much detail [ 15 ]. Additional limitations are based on the data sources used for machine learning, such as the lack of availability of all desired variables and missing data that can affect the development and performance of these models [ 16 , 34 , 36 , 48 ]. The lack of all relevant variables was noted as a particular concern for retrospective database studies, where the investigator is limited to what has been recorded [ 26 , 28 , 29 , 38 , 40 ]. Importantly and as observed in the studies included in this review, the lack of external validation was stated as a limitation of studies included in this review [ 28 , 30 , 38 , 42 ].
Limitations can also be on the part of the research team, as the need for both clinical and statistical expertise in the development and execution of studies using machine learning-based methodology, and users are warned against applying these methods blindly [ 22 ]. The importance of the role of clinical and statistical experts in the research team was noted in one study and highlighted as a strength of their work [ 21 ].
This study systematically reviewed and summarized the methods and approaches used for machine learning as applied to observational datasets that can inform patient-provider decision making. Machine learning methods have been applied much more broadly across observational studies than in the context of individual decision making, so the summary of this work does not necessarily apply to all machine learning-based studies. The focus of this work is on an area that remains largely unexplored, which is how to use large datasets in a manner that can inform and improve patient care in a way that supports shared decision making with reliable evidence that is applicable to the individual patient. Multiple publications cite the limitations of using population-based estimates for individual decisions [ 49 , 50 , 51 ]. Specifically, a summary statistic at the population level does not apply to each person in that cohort. Population estimates represent a point on a potentially wide distribution, and any one patient could fall anywhere within that distribution and be far from the point estimate value. On the other extreme, case reports or case series provide very specific individual-level data, but are not generalizable to other patients [ 52 ]. This review and summary provides guidance and suggestions of best practices to improve and hopefully increase the use of these methods to provide data and models to inform patient-provider decision making.
It was common for single modeling strategies to be employed within the identified publications. It has long been known that single algorithms to estimation can produce a fair amount of uncertainty and variability [ 53 ]. To overcome this limitation, there is a need for multiple algorithms and multiple iterations of the models to be performed. This, combined with more powerful analytics in recent years, provides a new standard for machine learning algorithm choice and development. While in some cases, a single model may fit the data well and provide an accurate answer, the certainty of the model can be supported through novel approaches, such as model averaging [ 54 ]. Few studies in this review combined multiple families of modeling strategies along with multiple iterations of the models. This should become a best practice in the future and is recommended as an additional criterion to assess study quality among machine learning-based modeling [ 54 ].
External validation is critical to ensure model accuracy, but was rarely conducted in the publications included in this review. The reasons for this could be many, such as lack of appropriate datasets or due to the lack of awareness of the importance of external validation [ 55 ]. As model development using machine learning increases, there is a need for external validation prior to application of models in any patient-provider setting. The generalizability of models is largely unknown without these data. Publications that did not conduct external validation also did not note the need for this to be completed, as generalizability was discussed in only five studies, one of which had also conducted the external validation. Of the remaining four studies, the role of generalizability was noted in terms of the need for future external validation in only one study [ 48 ]. Other reviews that were more broadly conducted to evaluate machine learning methods similarly found a low rate of external validation (6.6% versus 5.9% in this study) [ 56 ]. It was shown that there was lower prediction accuracy by external validation than simply by cross validation alone. The current review, with a focus on machine learning to support decision making at a practical level, suggests external validation is an important gap that should be filled prior to using these models for patient-provider decision making.
Luo and others suggest that k -fold validation may be used with proper stratification of the response variable as part of the model selection strategy [ 14 , 55 ]. The studies identified in this review generally conducted 5- or tenfold validation. There is no formal rule for the selection for the value of k , which is typically based on the size of the dataset; as k increases, bias will be reduced, but in turn variance will increase. While the tradeoff has to be accounted for, k = 5–10 has been found to be reasonable for most study purposes [ 57 ].
The evidence from identified publications suggests that the ethical concerns of lack of transparency and failure to report confidence in the findings are largely warranted. These limitations can be addressed through the use of multiple modeling approaches (to clarify the ‘black box’ nature of these approaches) and by including both external and high k-fold validation (to demonstrate the confidence in findings). To ensure these methods are used in a manner that improves patient care, the expectations of population-based risk prediction models of the past are no longer sufficient. It is essential that the right data, the right set of models, and appropriate validation are employed to ensure that the resulting data meet standards for high quality patient care.
This study did not evaluate the quality of the underlying real-world data used to develop, test or validate the algorithms. While not directly part of the evaluation in this review, researchers should be aware that all limitations of real-world data sources apply regardless of the methodology employed. However, when observational datasets are used for machine learning-based research, the investigator should be aware of the extent to which the methods they are using depend on the data structure and availability, and should evaluate a proposed data source to ensure it is appropriate for the machine learning project [ 45 ]. Importantly, databases should be evaluated to fully understand the variables included, as well as those variables that may have prognostic or predictive value, but may not be included in the dataset. The lack of important variables remains a concern with the use of retrospective databases for machine learning. The concerns with confounding (particularly unmeasured confounding), bias (including immortal time bias), and patient selection criteria to be in the database must also be evaluated [ 58 , 59 ]. These are factors that should be considered prior to implementing these methods, and not always at the forefront of consideration when applying machine learning approaches. The Luo checklist is a valuable tool to ensure that any machine-learning study meets high research standards for patient care, and importantly includes the evaluation of missing or potentially incorrect data (i.e. outliers) and generalizability [ 14 ]. This should be supplemented by a thorough evaluation of the potential data to inform the modeling work prior to its implementation, and ensuring that multiple modeling methods are applied.
This review found a wide variety of approaches, methods, statistical software and validation strategies that were employed in the application of machine learning methods to inform patient-provider decision making. Based on these findings, there is a need to ensure that multiple modeling approaches are employed in the development of machine learning-based models for patient care, which requires the highest research standards to reliably support shared evidence-based decision making. Models should be evaluated with clear criteria for model selection, and both internal and external validation are needed prior to applying these models to inform patient care. Few studies have yet to reach that bar of evidence to inform patient-provider decision making.
Availability of data and materials
All data generated or analyzed during this study are included in this published article and its supplementary information files.
Abbreviations
Artificial intelligence
Area under the curve
Classification and regression trees
Logistic least absolute shrinkage and selector operator
Steyerberg EW, Claggett B. Towards personalized therapy for multiple sclerosis: limitations of observational data. Brain. 2018;141(5):e38-e.
Fröhlich H, Balling R, Beerenwinkel N, Kohlbacher O, Kumar S, Lengauer T, et al. From hype to reality: data science enabling personalized medicine. BMC Med. 2018;16(1):150.
Article PubMed PubMed Central Google Scholar
Steyerberg EW. Clinical prediction models. Berlin: Springer; 2019.
Book Google Scholar
Schnabel RB, Sullivan LM, Levy D, Pencina MJ, Massaro JM, D’Agostino RB Sr, et al. Development of a risk score for atrial fibrillation (Framingham Heart Study): a community-based cohort study. Lancet. 2009;373(9665):739–45.
D’Agostino RB, Wolf PA, Belanger AJ, Kannel WB. Stroke risk profile: adjustment for antihypertensive medication. Framingham Study Stroke. 1994;25(1):40–3.
Article CAS PubMed Google Scholar
Framingham Heart Study: Risk Functions 2020. https://www.framinghamheartstudy.org/ .
Gawehn E, Hiss JA, Schneider G. Deep learning in drug discovery. Mol Inf. 2016;35:3–14.
Article CAS Google Scholar
Vamathevan J, Clark D, Czodrowski P, Dunham I, Ferran E, Lee G, et al. Applications of machine learning in drug discovery and development. Nat Rev Drug Discov. 2019;18(6):463–77.
Article CAS PubMed PubMed Central Google Scholar
Marcus G. Deep learning: A critical appraisal. arXiv preprint arXiv:180100631. 2018.
Grote T, Berens P. On the ethics of algorithmic decision-making in healthcare. J Med Ethics. 2020;46(3):205–11.
Article PubMed Google Scholar
Brnabic A, Hess L, Carter GC, Robinson R, Araujo A, Swindle R. Methods used for the applicability of real-world data sources to individual patient decision making. Value Health. 2018;21:S102.
Article Google Scholar
Fu H, Zhou J, Faries DE. Estimating optimal treatment regimes via subgroup identification in randomized control trials and observational studies. Stat Med. 2016;35(19):3285–302.
Liang M, Ye T, Fu H. Estimating individualized optimal combination therapies through outcome weighted deep learning algorithms. Stat Med. 2018;37(27):3869–86.
Luo W, Phung D, Tran T, Gupta S, Rana S, Karmakar C, et al. Guidelines for developing and reporting machine learning predictive models in biomedical research: a multidisciplinary view. J Med Internet Res. 2016;18(12):e323.
Toussi M, Lamy J-B, Le Toumelin P, Venot A. Using data mining techniques to explore physicians’ therapeutic decisions when clinical guidelines do not provide recommendations: methods and example for type 2 diabetes. BMC Med Inform Decis Mak. 2009;9(1):28.
Ramezankhani A, Hadavandi E, Pournik O, Shahrabi J, Azizi F, Hadaegh F. Decision tree-based modelling for identification of potential interactions between type 2 diabetes risk factors: a decade follow-up in a Middle East prospective cohort study. BMJ Open. 2016;6(12):e013336.
Pei D, Zhang C, Quan Y, Guo Q. Identification of potential type II diabetes in a Chinese population with a sensitive decision tree approach. J Diabetes Res. 2019;2019:4248218.
Neefjes EC, van der Vorst MJ, Verdegaal BA, Beekman AT, Berkhof J, Verheul HM. Identification of patients with cancer with a high risk to develop delirium. Cancer Med. 2017;6(8):1861–70.
Mubeen AM, Asaei A, Bachman AH, Sidtis JJ, Ardekani BA, Initiative AsDN. A six-month longitudinal evaluation significantly improves accuracy of predicting incipient Alzheimer’s disease in mild cognitive impairment. J Neuroradiol. 2017;44(6):381–7.
Hische M, Luis-Dominguez O, Pfeiffer AF, Schwarz PE, Selbig J, Spranger J. Decision trees as a simple-to-use and reliable tool to identify individuals with impaired glucose metabolism or type 2 diabetes mellitus. Eur J Endocrinol. 2010;163(4):565.
Bertsimas D, Dunn J, Pawlowski C, Silberholz J, Weinstein A, Zhuo YD, et al. Applied informatics decision support tool for mortality predictions in patients with cancer. JCO Clin Cancer Inform. 2018;2:1–11.
Bannister CA, Halcox JP, Currie CJ, Preece A, Spasic I. A genetic programming approach to development of clinical prediction models: a case study in symptomatic cardiovascular disease. PLoS ONE. 2018;13(9):e0202685.
Article PubMed PubMed Central CAS Google Scholar
Alaa AM, Bolton T, Di Angelantonio E, Rudd JHF, van der Schaar M. Cardiovascular disease risk prediction using automated machine learning: a prospective study of 423,604 UK Biobank participants. PLoS ONE. 2019;14(5):e0213653.
Baxter SL, Marks C, Kuo TT, Ohno-Machado L, Weinreb RN. Machine learning-based predictive modeling of surgical intervention in glaucoma using systemic data from electronic health records. Am J Ophthalmol. 2019;208:30–40.
Dong Y, Xu L, Fan Y, Xiang P, Gao X, Chen Y, et al. A novel surgical predictive model for Chinese Crohn’s disease patients. Medicine (Baltimore). 2019;98(46):e17510.
Hill NR, Ayoubkhani D, McEwan P, Sugrue DM, Farooqui U, Lister S, et al. Predicting atrial fibrillation in primary care using machine learning. PLoS ONE. 2019;14(11):e0224582.
Kang AR, Lee J, Jung W, Lee M, Park SY, Woo J, et al. Development of a prediction model for hypotension after induction of anesthesia using machine learning. PLoS ONE. 2020;15(4):e0231172.
Karhade AV, Ogink PT, Thio Q, Cha TD, Gormley WB, Hershman SH, et al. Development of machine learning algorithms for prediction of prolonged opioid prescription after surgery for lumbar disc herniation. Spine J. 2019;19(11):1764–71.
Kebede M, Zegeye DT, Zeleke BM. Predicting CD4 count changes among patients on antiretroviral treatment: Application of data mining techniques. Comput Methods Programs Biomed. 2017;152:149–57.
Kim I, Choi HJ, Ryu JM, Lee SK, Yu JH, Kim SW, et al. A predictive model for high/low risk group according to oncotype DX recurrence score using machine learning. Eur J Surg Oncol. 2019;45(2):134–40.
Kwon JM, Jeon KH, Kim HM, Kim MJ, Lim S, Kim KH, et al. Deep-learning-based out-of-hospital cardiac arrest prognostic system to predict clinical outcomes. Resuscitation. 2019;139:84–91.
Kwon JM, Lee Y, Lee Y, Lee S, Park J. An algorithm based on deep learning for predicting in-hospital cardiac arrest. J Am Heart Assoc. 2018;7(13):26.
Scheer JK, Smith JS, Schwab F, Lafage V, Shaffrey CI, Bess S, et al. Development of a preoperative predictive model for major complications following adult spinal deformity surgery. J Neurosurg Spine. 2017;26(6):736–43.
Lopez-de-Andres A, Hernandez-Barrera V, Lopez R, Martin-Junco P, Jimenez-Trujillo I, Alvaro-Meca A, et al. Predictors of in-hospital mortality following major lower extremity amputations in type 2 diabetic patients using artificial neural networks. BMC Med Res Methodol. 2016;16(1):160.
Rau H-H, Hsu C-Y, Lin Y-A, Atique S, Fuad A, Wei L-M, et al. Development of a web-based liver cancer prediction model for type II diabetes patients by using an artificial neural network. Comput Methods Programs Biomed. 2016;125:58–65.
Ng T, Chew L, Yap CW. A clinical decision support tool to predict survival in cancer patients beyond 120 days after palliative chemotherapy. J Palliat Med. 2012;15(8):863–9.
Pérez-Gandía C, Facchinetti A, Sparacino G, Cobelli C, Gómez E, Rigla M, et al. Artificial neural network algorithm for online glucose prediction from continuous glucose monitoring. Diabetes Technol Therapeut. 2010;12(1):81–8.
Azimi P, Mohammadi HR, Benzel EC, Shahzadi S, Azhari S. Use of artificial neural networks to decision making in patients with lumbar spinal canal stenosis. J Neurosurg Sci. 2017;61(6):603–11.
Bowman A, Rudolfer S, Weller P, Bland JDP. A prognostic model for the patient-reported outcome of surgical treatment of carpal tunnel syndrome. Muscle Nerve. 2018;58(6):784–9.
Hearn J, Ross HJ, Mueller B, Fan CP, Crowdy E, Duhamel J, et al. Neural networks for prognostication of patients with heart failure. Circ. 2018;11(8):e005193.
Google Scholar
Isma’eel HA, Cremer PC, Khalaf S, Almedawar MM, Elhajj IH, Sakr GE, et al. Artificial neural network modeling enhances risk stratification and can reduce downstream testing for patients with suspected acute coronary syndromes, negative cardiac biomarkers, and normal ECGs. Int J Cardiovasc Imaging. 2016;32(4):687–96.
Isma’eel HA, Sakr GE, Serhan M, Lamaa N, Hakim A, Cremer PC, et al. Artificial neural network-based model enhances risk stratification and reduces non-invasive cardiac stress imaging compared to Diamond-Forrester and Morise risk assessment models: a prospective study. J Nucl Cardiol. 2018;25(5):1601–9.
Jovanovic P, Salkic NN, Zerem E. Artificial neural network predicts the need for therapeutic ERCP in patients with suspected choledocholithiasis. Gastrointest Endosc. 2014;80(2):260–8.
Zhou HF, Huang M, Ji JS, Zhu HD, Lu J, Guo JH, et al. Risk prediction for early biliary infection after percutaneous transhepatic biliary stent placement in malignant biliary obstruction. J Vasc Interv Radiol. 2019;30(8):1233-41.e1.
Hertroijs DF, Elissen AM, Brouwers MC, Schaper NC, Köhler S, Popa MC, et al. A risk score including body mass index, glycated haemoglobin and triglycerides predicts future glycaemic control in people with type 2 diabetes. Diabetes Obes Metab. 2018;20(3):681–8.
Oviedo S, Contreras I, Quiros C, Gimenez M, Conget I, Vehi J. Risk-based postprandial hypoglycemia forecasting using supervised learning. Int J Med Inf. 2019;126:1–8.
Khanji C, Lalonde L, Bareil C, Lussier MT, Perreault S, Schnitzer ME. Lasso regression for the prediction of intermediate outcomes related to cardiovascular disease prevention using the TRANSIT quality indicators. Med Care. 2019;57(1):63–72.
Anderson JP, Parikh JR, Shenfeld DK, Ivanov V, Marks C, Church BW, et al. Reverse engineering and evaluation of prediction models for progression to type 2 diabetes: an application of machine learning using electronic health records. J Diabetes Sci Technol. 2016;10(1):6–18.
Patsopoulos NA. A pragmatic view on pragmatic trials. Dialogues Clin Neurosci. 2011;13(2):217–24.
Lu CY. Observational studies: a review of study designs, challenges and strategies to reduce confounding. Int J Clin Pract. 2009;63(5):691–7.
Morgenstern H. Ecologic studies in epidemiology: concepts, principles, and methods. Annu Rev Public Health. 1995;16(1):61–81.
Vandenbroucke JP. In defense of case reports and case series. Ann Intern Med. 2001;134(4):330–4.
Buckland ST, Burnham KP, Augustin NH. Model selection: an integral part of inference. Biometrics. 1997;53:603–18.
Zagar A, Kadziola Z, Lipkovich I, Madigan D, Faries D. Evaluating bias control strategies in observational studies using frequentist model averaging 2020 (submitted).
Kang J, Schwartz R, Flickinger J, Beriwal S. Machine learning approaches for predicting radiation therapy outcomes: a clinician’s perspective. Int J Radiat Oncol Biol Phys. 2015;93(5):1127–35.
Scott IM, Lin W, Liakata M, Wood J, Vermeer CP, Allaway D, et al. Merits of random forests emerge in evaluation of chemometric classifiers by external validation. Anal Chim Acta. 2013;801:22–33.
Kuhn M, Johnson K. Applied predictive modeling. Berlin: Springer; 2013.
Hess L, Winfree K, Muehlenbein C, Zhu Y, Oton A, Princic N. Debunking Myths While Understanding Limitations. Am J Public Health. 2020;110(5):E2-E.
Thesmar D, Sraer D, Pinheiro L, Dadson N, Veliche R, Greenberg P. Combining the power of artificial intelligence with the richness of healthcare claims data: Opportunities and challenges. PharmacoEconomics. 2019;37(6):745–52.
Download references
Acknowledgements
Not applicable.
No funding was received for the conduct of this study.
Author information
Authors and affiliations.
Eli Lilly and Company, Sydney, NSW, Australia
Alan Brnabic
Eli Lilly and Company, Indianapolis, IN, USA
Lisa M. Hess
You can also search for this author in PubMed Google Scholar
Contributions
AB and LMH contributed to the design, implementation, analysis and interpretation of the data included in this study. AB and LMH wrote, revised and finalized the manuscript for submission. AB and LMH have both read and approved the final manuscript.
Corresponding author
Correspondence to Lisa M. Hess .
Ethics declarations
Ethics approval and consent to participate, consent for publication, competing interests.
Authors are employees of Eli Lilly and Company and receive salary support in that role.
Additional information
Publisher's note.
Springer Nature remains neutral with regard to jurisdictional claims in published maps and institutional affiliations.
Supplementary Information
Additional file 1..
Table S1. Study quality of eligible publications, modified Luo scale [14].
Rights and permissions
Open Access This article is licensed under a Creative Commons Attribution 4.0 International License, which permits use, sharing, adaptation, distribution and reproduction in any medium or format, as long as you give appropriate credit to the original author(s) and the source, provide a link to the Creative Commons licence, and indicate if changes were made. The images or other third party material in this article are included in the article's Creative Commons licence, unless indicated otherwise in a credit line to the material. If material is not included in the article's Creative Commons licence and your intended use is not permitted by statutory regulation or exceeds the permitted use, you will need to obtain permission directly from the copyright holder. To view a copy of this licence, visit http://creativecommons.org/licenses/by/4.0/ . The Creative Commons Public Domain Dedication waiver ( http://creativecommons.org/publicdomain/zero/1.0/ ) applies to the data made available in this article, unless otherwise stated in a credit line to the data.
Reprints and permissions
About this article
Cite this article.
Brnabic, A., Hess, L.M. Systematic literature review of machine learning methods used in the analysis of real-world data for patient-provider decision making. BMC Med Inform Decis Mak 21 , 54 (2021). https://doi.org/10.1186/s12911-021-01403-2
Download citation
Received : 07 July 2020
Accepted : 20 January 2021
Published : 15 February 2021
DOI : https://doi.org/10.1186/s12911-021-01403-2
Share this article
Anyone you share the following link with will be able to read this content:
Sorry, a shareable link is not currently available for this article.
Provided by the Springer Nature SharedIt content-sharing initiative
- Machine learning
- Decision making
- Decision tree
- Random forest
- Automated neural network
BMC Medical Informatics and Decision Making
ISSN: 1472-6947
- General enquiries: [email protected]
arXiv's Accessibility Forum starts next month!
Help | Advanced Search
Computer Science > Machine Learning
Title: multimodal methods for analyzing learning and training environments: a systematic literature review.
Abstract: Recent technological advancements have enhanced our ability to collect and analyze rich multimodal data (e.g., speech, video, and eye gaze) to better inform learning and training experiences. While previous reviews have focused on parts of the multimodal pipeline (e.g., conceptual models and data fusion), a comprehensive literature review on the methods informing multimodal learning and training environments has not been conducted. This literature review provides an in-depth analysis of research methods in these environments, proposing a taxonomy and framework that encapsulates recent methodological advances in this field and characterizes the multimodal domain in terms of five modality groups: Natural Language, Video, Sensors, Human-Centered, and Environment Logs. We introduce a novel data fusion category -- mid fusion -- and a graph-based technique for refining literature reviews, termed citation graph pruning. Our analysis reveals that leveraging multiple modalities offers a more holistic understanding of the behaviors and outcomes of learners and trainees. Even when multimodality does not enhance predictive accuracy, it often uncovers patterns that contextualize and elucidate unimodal data, revealing subtleties that a single modality may miss. However, there remains a need for further research to bridge the divide between multimodal learning and training studies and foundational AI research.
Comments: | Submitted to ACM Computing Surveys. Currently under review |
Subjects: | Machine Learning (cs.LG); Multimedia (cs.MM) |
Cite as: | [cs.LG] |
(or [cs.LG] for this version) | |
Focus to learn more arXiv-issued DOI via DataCite |
Submission history
Access paper:.
- HTML (experimental)
- Other Formats
References & Citations
- Google Scholar
- Semantic Scholar
BibTeX formatted citation

Bibliographic and Citation Tools
Code, data and media associated with this article, recommenders and search tools.
- Institution
arXivLabs: experimental projects with community collaborators
arXivLabs is a framework that allows collaborators to develop and share new arXiv features directly on our website.
Both individuals and organizations that work with arXivLabs have embraced and accepted our values of openness, community, excellence, and user data privacy. arXiv is committed to these values and only works with partners that adhere to them.
Have an idea for a project that will add value for arXiv's community? Learn more about arXivLabs .
Research on K-12 maker education in the early 2020s – a systematic literature review
- Open access
- Published: 27 August 2024
Cite this article
You have full access to this open access article
- Sini Davies ORCID: orcid.org/0000-0003-3689-7967 1 &
- Pirita Seitamaa-Hakkarainen ORCID: orcid.org/0000-0001-7493-7435 1
This systematic literature review focuses on the research published on K-12 maker education in the early 2020s, providing a current picture of the field. Maker education is a hands-on approach to learning that encourages students to engage in collaborative and innovative activities, using a combination of traditional design and fabrication tools and digital technologies to explore real-life phenomena and create tangible artifacts. The review examines the included studies from three perspectives: characteristics, research interests and findings, previous research gaps filled, and further research gaps identified. The review concludes by discussing the overall picture of the research on maker education in the early 2020s and suggesting directions for further studies. Overall, this review provides a valuable resource for researchers, educators, and policymakers to understand the current state of K-12 maker education research.
Explore related subjects
- Artificial Intelligence
- Digital Education and Educational Technology
Avoid common mistakes on your manuscript.
Introduction
Maker culture developed through the pioneering efforts of Papert ( 1980 ) and his followers, such as Blikstein ( 2013 ), Kafai and Peppler ( 2011 ), and Resnick ( 2017 ). It has gained popularity worldwide as an educational approach to encourage student engagement in learning science, technology, engineering, arts, and mathematics (STEAM) (Martin, 2015 ; Papavlasopoulou et al., 2017 ; Vossoughi & Bevan, 2014 ). Maker education involves engaging students to collaborate and innovate together by turning their ideas into tangible creations through the use of conceptual ideas (whether spoken or written), visual representations such as drawings and sketches, and material objects like prototypes and models (Kangas et al., 2013 ; Koh et al., 2015 ). Another core aspect of maker education is combining traditional design and fabrication tools and methods with digital technologies, such as 3D CAD and 3D printing, electronics, robotics, and programming, which enables students to create multifaceted artifacts and hybrid solutions to their design problems that include both digital and virtual features (e.g., Blikstein, 2013 ; Davies et al., 2023 ; Riikonen, Seitamaa-Hakkarainen, et al., 2020 ). The educational value of such multi-dimensional, concrete making has become widely recognized (e.g., Blikstein, 2013 ; Kafai, 1996 ; Kafai et al., 2014 ; Martin, 2015 ).
Maker education has been studied intensively, as indicated by several previous literature reviews (Iivari et al., 2016 ; Lin et al., 2020 ; Papavlasopoulou et al., 2017 ; Rouse & Rouse, 2022 ; Schad & Jones, 2020 ; Vossoughi & Bevan, 2014 ; Yulis San Juan & Murai, 2022 ). These reviews have revealed how the field has been evolving and provided a valuable overall picture of the research on maker education before the 2020s, including only a few studies published in 2020 or 2021. However, the early years of the 2020s have been an extraordinary period in time in many ways. The world was hit by the COVID-19 pandemic, followed by the global economic crises, increasing geopolitical tensions, and wars that have had a major impact on societies, education, our everyday lives, and inevitably on academic research as well. Furthermore, 2023 was a landmark year in the development of artificial intelligence (AI). In late 2022, OpenAI announced the release of ChatGPT 3.5, a major update to their large language model that is able to generate human-like text. Since then, sophisticated AI systems have rushed into our lives at an accelerating speed and are now becoming integrated with other technologies and applications, shaping how we live, work, our cultures, and our environments irreversibly (see, e.g., World Economic Forum, 2023 ). Thus, it can be argued that towards the end of 2023, the world had transitioned into the era of AI. It is essential that researchers, educators, and policymakers have a fresh overall understanding and a current picture of research on K-12 maker education to develop new, research-based approaches to technology and design education in the present rapidly evolving technological landscape of AI. This is especially important in order to avoid falling back towards shallow epistemic and educational practices of repetition and reproduction. The present systematic review was conducted to provide a ‘big picture’ of the research on K-12 maker education published in the extraordinary times of the early 2020s and to act as a landmark between the research on the field before and after the transition to the AI era. The review was driven by one main research question: How has the research on maker education developed in the early 2020s? To answer this question, three specific research questions were set:
What were the characteristics of the studies in terms of geographical regions, quantity of publications, research settings, and research methods?.
What were the research interests and findings of the reviewed studies?.
How did the reviewed studies fulfill the research gaps identified in previous literature reviews, and what further research gaps they identified?.
The following will outline the theoretical background of the systematic literature review by examining previous literature reviews on maker culture and maker education. This will be followed by an explanation of the methodologies used and findings. Finally, the review will conclude by discussing the overall picture of the research on maker education in the early 2020s and suggesting directions for further studies.
Previous literature reviews on maker culture and maker education
Several literature reviews have been conducted on maker education over the past ten years. The first one by Vossoughi and Bevan ( 2014 ) concentrated on the impact of tinkering and making on children’s learning, design principles and pedagogical approaches in maker programs, and specific tensions and possibilities within the maker movement for equity-oriented teaching and learning. They approached the maker movement in the context of out-of-school time STEM from three perspectives: (1) entrepreneurship and community creativity, (2) STEM pipeline and workforce development, and (3) inquiry-based education. At the time of their review, the research on maker education was just emerging, and therefore, their review included only a few studies. The review findings highlighted how STEM practices were developed through tinkering and striving for equity and intellectual safety (Vossoughi & Bevan, 2014 ). Furthermore, they also revealed how making activities support new ways of learning and collaboration in STEM. Their findings also pointed out some tensions and gaps in the literature, especially regarding a focus that is too narrow on STEM, tools, and techniques, as well as a lack of maker projects conducted within early childhood education or families.
In subsequent literature reviews (Iivari et al., 2016 ; Lin et al., 2020 ; Papavlasopoulou et al., 2017 ; Rouse & Rouse, 2022 ; Schad & Jones, 2020 ; Yulis San Juan & Murai, 2022 ), the interests of the reviews were expanded. Iivari and colleagues ( 2016 ) reviewed the potential of digital fabrication and making for empowering children and helping them see themselves as future digital innovators. They analyzed the studies based on five conditions: conditions for convergence, entry, social support, competence, and reflection, which were initially developed to help with project planning (Chawla & Heft, 2002 ). Their findings revealed that most of the studies included in their review emphasized the conditions for convergence, entry, and competence. However, only a few studies addressed the conditions for social support and reflection (Iivari et al., 2016 ). The reviewed studies emphasized children’s own interests and their voluntary participation in the projects. Furthermore, the studies highlighted projects leading to both material and learning-related outcomes and the development of children’s competencies in decision-making, design, engineering, technology, and innovation through projects.
Papavlasopoulou and colleagues ( 2017 ) took a broader scope on their systematic literature review, characterizing the overall development and stage of research on maker education through analyzing research settings, interests, and methods, synthesizing findings, and identifying research gaps. They were specifically interested in the technology used, subject areas that implement making activities, and evaluation methods of making instruction across all levels of education and in both formal and informal settings. Their data comprised 43 peer-reviewed empirical studies on maker-centered teaching and learning with children in their sample, providing participants with any making experience. In Papavlasopoulou and colleagues’ ( 2017 ) review, the included studies were published between 2011 and November 2015 as journal articles, conference papers, or book chapters. Most of the studies were conducted with fewer than 50 participants ( n = 34), the most prominent age group being children from the beginning of primary school up to 14 years old ( n = 22). The analyzed studies usually utilized more than one data collection method, mainly focusing on qualitative ( n = 22) or mixed method ( n = 11) approaches. Most included studies focused on programming skills and computational thinking ( n = 32) or STEM subjects ( n = 6). The studies reported a wide range of positive effects of maker education on learning, the development of participants’ self-efficacy, perceptions, and engagement (Papavlasopoulou et al., 2017 ). There were hardly any studies reporting adverse effects.
Schad and Jones ( 2020 ) focused their literature review on empirical studies of the maker movement’s impacts on formal K12 educational environments, published between 2000 and 2018. Their Boolean search (maker movement AND education) to three major academic research databases resulted in 599 studies, of which 20 were included in the review. Fourteen of these studies focused on K12 students, and six on K12 teachers. All but three of the studies were published between 2014 and 2018. Similarly to the studies reported in the previous literature reviews (Iivari et al., 2016 ; Papavlasopoulou et al., 2017 ; Vossoughi & Bevan, 2014 ), the vast majority of the studies were qualitative studies that reported positive opportunities for maker-centered approaches in STEM learning and promotion of excitement and motivation. On the other hand, the studies on K12 in- and preservice teacher education mainly focused on the importance of offering opportunities for teachers to engage in making activities. Both, studies focused on students or teachers, promoting equity and offering equally motivating learning experiences regardless of participants’ gender or background was emphasized.
Lin and colleagues’ ( 2020 ) review focused on the assessment of maker-centered learning activities. After applying inclusion and exclusion criteria, their review consisted of 60 peer-reviewed empirical studies on making activities that included making tangible artifacts and assessments to measure learning outcomes. The studies were published between 2006 and 2019. Lin and colleagues ( 2020 ) also focused on all age groups and activities in both formal and informal settings. Most studies included applied STEM as their main subject domain and utilized a technology-based platform, such as LilyPad Arduino microcontroller, Scratch, or laser cutting. The results of the review revealed that in most studies, learning outcomes were usually measured through the assessment of artifacts, tests, surveys, interviews, and observations. The learning outcomes measured were most often cognitive skills on STEM-related content knowledge or students’ feelings and attitudes towards STEM or computing.
The two latest systematic reviews, published in 2022, also focused on specific research interests in maker education (Rouse & Rouse, 2022 ; Yulis San Juan & Murai, 2022 ). Rouse and Rouse ( 2022 ) reviewed studies that specifically investigated learning in preK-12 maker education in formal school-based settings. Their analysis included 22 papers from seven countries, all but two published between 2017 and 2019. Only two of the studies focused on early childhood education, and three involved participants from the elementary level. Like previous reviews, most studies were conducted with qualitative methods ( n = 17). On the other hand, in contrast to the earlier reviews (Lin et al., 2020 ; Papavlasopoulou et al., 2017 ; Schad & Jones, 2020 ), the studies included in the review did not concentrate on content-related outcomes on STEM or computing. Instead, a wide range of learning outcomes was investigated, such as 21st-century skills, agency, and materialized knowledge. On the other hand, they found that equity and inclusivity were not ubiquitously considered when researchers design makerspace interventions. Yulis San Juan and Murai’s ( 2022 ) literature review focused on frustration in maker-centered learning activities. Their analysis consisted of 28 studies published between 2013 and 2021. Their findings of the studies identified six factors that are most often recognized as the causes of frustration in makerspace activities: ‘unfamiliar pedagogical approach, time constraints, collaboration, outcome expectations, lack of skills and knowledge, and tool affordances and availability’ (Yulis San Juan & Murai, 2022 , p. 4).
From these previous literature reviews, five significant research gaps emerged that required further investigation and attention:
Teacher training, pedagogies, and orchestration of learning activities in maker education (Papavlasopoulou et al., 2017 ; Rouse & Rouse, 2022 ; Schad & Jones, 2020 ; Vossoughi & Bevan, 2014 ).
Wide variety of learning outcomes that potentially emerge from making activities, as well as the development of assessment methods and especially systematic ways to measure student learning (Lin et al., 2020 ; Rouse & Rouse, 2022 ; Schad & Jones, 2020 ).
Equity and inclusivity in maker education (Rouse & Rouse, 2022 ; Vossoughi & Bevan, 2014 ).
Practices, tools, and technologies used in makerspaces and digital fabrication (Iivari et al., 2016 ; Papavlasopoulou et al., 2017 ).
Implementation and effects of maker education in formal, school-based settings and specific age groups, especially early childhood education (Papavlasopoulou et al., 2017 ; Rouse & Rouse, 2022 ).
Methodology
This review was conducted following the Preferred Reporting Items for Systematic Reviews and Meta-Analyses (PRISMA) guidelines, adapting it to educational settings where studies are conducted with qualitative, quantitative, and mixed methods (Page et al., 2021 ; Tong et al., 2012 ). Review protocols were defined for data collection, inclusion, exclusion, and quality criteria and the data analysis. In the following, the method used for each stage of the review process will be defined in detail.
Data collection
To gather high-quality and comprehensive data, a search for peer-reviewed articles was conducted in three international online bibliographic databases: Scopus, Education Resources Information Center (ERIC), and Academic Search Complete (EBSCO). Scopus and EBSCO are extensive multi-disciplinary databases for research literature, covering research published in over 200 disciplines, including education, from over 6000 publishers. ERIC concentrates exclusively on educational-related literature, covering publications from over 1900 full-text journals. These three databases were considered to offer a broad scope to capture comprehensive new literature on K-12 maker education. The search aimed to capture peer-reviewed literature on maker education and related processes conducted in both formal and informal K-12 educational settings. The search was limited to articles published in English between 2020 and 2023. Major search terms and their variations were identified to conduct the search, and a Boolean search string was formed from them. The search was implemented in October 2023 with the following search string that was used to search on titles, abstracts, and keywords:
(“maker education” OR “maker pedagogy” OR “maker-centered learning” OR “maker centered learning” OR “maker-centred learning” OR “maker centred learning” OR “maker learning” OR “maker space*” OR makerspace* OR “maker culture” OR “design learning” OR “maker practices” OR “collaborative invention*” OR co-invention*) AND (“knowledge-creation” OR “knowledge creation” OR “knowledgecreation” OR maker* OR epistemic OR “technology education” OR “design-based learning” OR “design based learning” OR “designbased learning” OR “design learning” OR “design thinking” OR “codesign” OR “co-design” OR “co design” OR craft* OR tinker* OR “collaborative learning” OR inquiry* OR “STEAM” OR “project-based learning” OR “project based learning” OR “projectbased learning” OR “learning project*” OR “knowledge building” OR “making” OR creati* OR innovat* OR process*) AND (school* OR pedago* OR “secondary education” OR “pre-primary education” OR “primary education” OR “special education” OR “early childhood education” OR “elementary education” OR primary-age* OR elementary-age* OR “k-12” OR “youth” OR teen* OR adolescen* OR child* OR “tween”) .
Inclusion and exclusion criteria
The search provided 700 articles in total, 335 from Scopus, 345 from EBSCO, and 20 from ERIC that were aggregated to Rayyan (Ouzzani et al., 2016 ), a web and mobile app for systematic reviews, for further processing and analysis. After eliminating duplicates, 513 studies remained. At the next stage, the titles and abstracts of these studies were screened independently by two researchers to identify papers within the scope of this review. Any conference papers, posters, work-in-progress studies, non-peer-reviewed papers, review articles, and papers focusing on teacher education or teachers’ professional development were excluded from the review. To be included, the study had to meet all the following four inclusion criteria. It had to:
show empirical evidence.
describe any making experience or testing process conducted by the participants.
include participants from the K-12 age group in their sample.
have an educational purpose.
For example, studies that relied purely on statistical data collected outside a maker educational setting or studies that described a maker space design process but did not include any research data from an actual making experience conducted by participants from the K-12 age group were excluded. Studies conducted both in formal and informal settings were included in the review. Also, papers were included regardless of whether they were conducted using qualitative, quantitative, or mixed methods. After the independent screening process, the results were combined, and any conflicting assessments were discussed and settled. Finally, 149 studies were included to be retrieved for further evaluation of eligibility, of which five studies were not available for retrieval. Thus, the screening resulted in 144 included studies with full text retrieved to apply quality criteria and further analysis.
Quality criteria
The quality of each of the remaining 144 studies was assessed against the Critical Appraisal Skills Programme’s ( 2023 ) qualitative study checklist, which was slightly adjusted for the context of this review. The checklist consisted of ten questions that each address one quality criterion:
Was there a clear statement of the aims of the research?.
Are the methodologies used appropriate?.
Was the research design appropriate to address the research aims?.
Was the recruitment strategy appropriate to the aims of the research?.
Was the data collected in a way that addressed the research issue?.
Has the relationship between the researcher and participants been adequately considered?.
Have ethical issues been taken into consideration?.
Was the data analysis sufficiently rigorous?.
Is there a clear statement of findings?.
How valuable is the research?.
The first author assessed the quality by reading each study’s full text. To be included in the final analysis, the study had to meet both the inclusion-exclusion and the quality criteria. In this phase, the final assessment for eligibility, 50 studies were excluded due to not meeting the initial inclusion and exclusion criteria, and 32 studies for not filling the criteria for quality. A total of 62 studies were included in the final analysis of this literature review. The PRISMA flow chart (Haddaway et al., 2022 ; see also Page et al., 2021 ) of the study selection process is presented in Fig. 1 .
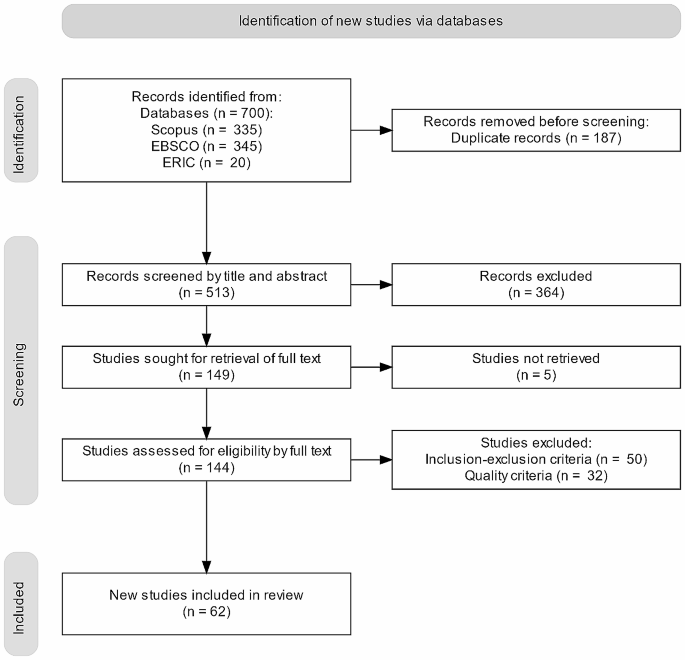
PRISMA study selection flow chart (Haddaway et al., 2022 )
Qualitative content analysis of the reviewed studies
The analysis of the studies included in the review was conducted through careful reading of the full texts of the articles by the first author. To answer the first research question: What were the characteristics of the studies in terms of geographical regions, quantity of publications, research settings, and methods; a deductive coding framework was applied that consisted of characterizing factors of the study, its research setting as well as data collection and analysis methods applied. The predetermined categories of the study characteristics and the codes associated with each category are presented in Table 1 . The educational level of the participants was determined by following The International Standard Classification of Education (ISCED) (UNESCO Institute for Statistics, 2012 ). Educational level was chosen instead of an age group as a coding category because, during the first abstract and title screening of the articles, it became evident that the studies describe their participants more often by their educational level than age. The educational levels were converted from national educational systems following the ISCED diagrams (UNESCO Institute for Statistics, 2021 ).
In addition to the deductive coding, the following analysis categories were gathered from the articles through inductive analysis: journal, duration of the project, number of participants, types of research data collected, and specific data analysis methods. Furthermore, the following characteristics of the studies were marked in the data when applicable: if the research was conducted as a case study, usage of control groups, specific focus on minority groups, gifted students, special needs students, or inclusion. Inductive coding and thematic analysis were applied to answer the second research question: what were the research interests and findings of the reviewed studies? The categorization of research interests was then combined with some aspects of the first part of the analysis to reveal further interesting characteristics about the latest developments in the research in maker education.
In the following, the findings of this systematic literature review will be presented for each research question separately.
Characteristics of research in K-12 maker education in the 2020s
Of the studies included in the review, presented in Table 2 and 20 studies were published in 2020, 17 in 2021, 12 in 2022, and 13 in 2023. The slight decline in publications does not necessarily indicate a decline in interest towards maker education but is more likely due to the COVID-19 pandemic that heavily limited hands-on activities and in situ data collection. Compared to the latest wide-scope review on maker education (Papavlasopoulou et al., 2017 ), the number of high-quality studies published yearly appears to be at similar levels to those in the previous reviews. The studies included in the present review were published in 34 different peer-reviewed academic journals, of which 13 published two or more articles.
Regarding the geographic distribution of studies conducted on maker education, the field seems to be becoming more internationally spread. In 2020, the studies mainly published research conducted in either the USA ( n = 6) or Finland ( n = 12), whereas in the subsequent years, the studies were distributed more evenly around the world. However, North America and Scandinavia remained the epicenters of research on maker education, conducting over half of the studies published each year.
Most of the reviewed studies used qualitative methods ( n = 42). Mixed methods were utilized in 13 studies, and quantitative methods in seven. Forty-four studies were described as case studies by their authors, and, on the other hand, a control group was used in four quantitative and two mixed methods studies. The analysis indicated an interesting research shift towards making activities part of formal educational settings instead of informal, extracurricular activities. Of the studies included in this review, 82% ( n = 51) were conducted exclusively in formal educational settings. This contrasts significantly with the previous literature review by Papavlasopoulou and colleagues ( 2017 ), where most studies were conducted in informal settings. Furthermore, Schad and Jones ( 2020 ) identified only 20 studies between 2000 and 2018 conducted in formal educational settings in K12-education, and Rouse and Rouse ( 2022 ) identified 22 studies in similar settings from 2014 to early 2020. In these reviews, nearly all studies done in formal educational settings were published in the last years of the 2010 decade. Thus, this finding suggests that the change in learning settings started to emerge in the latter half of the 2010s, and in the 2020s, maker education in formal settings has become the prominent focus of research. The need for further research in formal settings was one of the main research gaps identified in previous literature reviews (Papavlasopoulou et al., 2017 ; Rouse & Rouse, 2022 ).
In addition to the shift from informal to formal educational settings, the projects studied in the reviewed articles were conducted nearly as often in school and classroom environments ( n = 26) as in designated makerspaces ( n = 28). Only seven of the studied projects took place in other locations, such as youth clubs, libraries, or summer camps. One project was conducted entirely in an online learning environment. Most of the studied projects involved children exclusively from primary ( n = 27) or lower secondary ( n = 26) education levels. Only three studies were done with students in upper secondary education. Like the previous literature reviews, only a few studies concentrated on children in early childhood education (Papavlasopoulou et al., 2017 ; Rouse & Rouse, 2022 ). Three articles reported projects conducted exclusively on early childhood education age groups, and three studies had participants from early childhood education together with children from primary ( n = 2) or lower secondary education ( n = 1).
The number of child participants in the studies varied between 1 and 576, and 14 studies also included teachers or other adults in their sample. The number of participating children in relation to the methods used is presented in Fig. 2 . Most of the qualitative studies had less than 100 children in their sample. However, there were three qualitative studies with 100 to 199 child participants (Friend & Mills, 2021 ; Leskinen et al., 2021 ; Riikonen, Kangas, et al., 2020 ) and one study with 576 participating children (Forbes et al., 2021 ). Studies utilizing mixed methods were either conducted with a very large number of child participants or with less than 100 participants, ranging from 4 to 99. Studies using quantitative methods, on the other hand, in most cases had 50–199 participants ( n = 6). One quantitative study was conducted with 35 child participants (Yin et al., 2020 ). Many studies included participants from non-dominant backgrounds or with special educational needs. However, only two studies focused specifically on youth from non-dominant backgrounds (Brownell, 2020 ; Hsu et al., 2022 ), and three studies focused exclusively on inclusion and students with special needs (Giusti & Bombieri, 2020 ; Martin et al., 2020 ; Sormunen et al., 2020 ). In addition, one study specifically chose gifted students in their sample (Andersen et al., 2022 ).
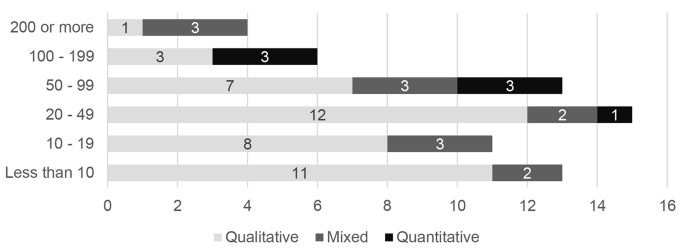
Child participants in the reviewed studies in relation to the methods used
Slightly over half of the studied projects had only collaborative tasks ( n = 36), 11 projects involved both collaborative and individual tasks, and in 11 projects, the participants worked on their own individual tasks. Four studies did not specify whether the project was built around collaborative or individual tasks. In most cases, the projects involved both traditional tangible tools and materials as well as digital devices and fabrication technologies ( n = 54). In five projects, the students worked entirely with digital design and making methods, and in 3 cases, only with traditional tangible materials. Similarly, the outcomes of the project tasks were mainly focused on designing and building artifacts that included both digital and material elements ( n = 31), or the project included multiple activities and building of several artifacts that were either digital, material, or had both elements ( n = 17). Eleven projects included digital exploration without an aim to build a design artifact as a preparatory activity, whereas one project was based solely on digital exploration as the making activity. Material artifacts without digital elements were made in seven of the studied projects, and six concentrated solely on digital artifact making.
The duration of the projects varied between two hours (Tisza & Markopoulos, 2021 ) and five years (Keune et al., 2022 ). The number of studies in each categorized project duration range, in relation to the methods used, is presented in Fig. 3 . Over half of the projects lasted between 1 month and one year ( n = 35), nine were longer, lasting between 1 and 5 years, and 14 were short projects lasting less than one month. Three qualitative studies and one quantitative study did not give any indication of the duration of the project. Most of the projects of qualitative studies took at least one month ( n = 32), whereas projects in mixed method studies usually were shorter than three months ( n = 10). On the other hand, quantitative studies usually investigated projects that were either shorter than three months ( n = 4) or longer than one year ( n = 2).
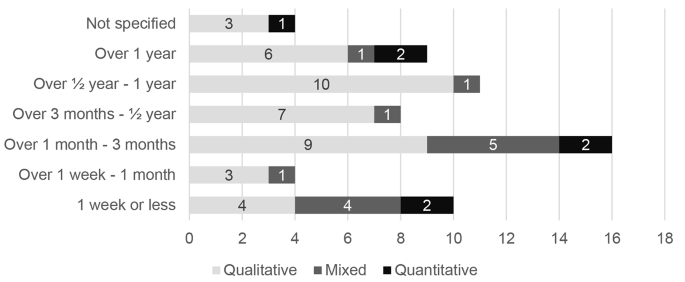
Duration of the studied projects in relation to the methods used
A multitude of different types of data was used in the reviewed studies. The data collection methods utilized by at least three reviewed studies are presented in Table 3 . Qualitative studies usually utilized several (2 to 6) different data gathering methods ( n = 31), and all mixed method studies used more than one type of data (2 to 6). The most common data collection methods in qualitative studies were video data, interviews, and ethnographic observations combined with other data, such as design artifacts, photographs, and student portfolios. In addition to the data types specified in Table 3 , some studies used more unusual data collection methods such as lesson plans (Herro et al., 2021b ), the think-aloud protocol (Friend & Mills, 2021 ; Impedovo & Cederqvist, 2023 ), and social networks (Tenhovirta et al., 2022 ). Eleven qualitative studies used only one type of data, mainly video recordings ( n = 9). Mixed method studies, on the other hand, relied often on interviews, pre-post measurements, surveys, and video data. In addition to the data types in Table 3 , mixed-method studies utilized biometric measurements (Hsu et al., 2022 ; Lee, 2021 ), lesson plans (Falloon et al., 2022 ), and teacher assessments (Doss & Bloom, 2023 ). In contrast to the qualitative and mixed method studies, all quantitative studies, apart from one (Yin et al., 2020 ), used only one form of research data, either pre-post measurements or surveys.
The findings of the data collection methods are similar to the previous literature review of Papavlasopoulou and colleagues ( 2017 ) regarding the wide variety of data types used in qualitative and mixed-method studies. However, when compared to their findings on specific types of research data used, video recordings have become the most popular way of collecting data in recent years, replacing interviews and ethnographic observations.
Research interests and findings of the reviewed studies
Seven categories of research interests emerged from the inductive coding of the reviewed studies. The categories are presented in Table 4 in relation to the research methods and educational levels of the participating children. Five qualitative studies, four mixed methods studies, and two quantitative studies had research interests from more than one category. Processes, activity, and practices, as well as sociomateriality in maker education, were studied exclusively with qualitative methods, and, on the other hand, nearly all studies on student motivation, interests, attitudes, engagement, and mindset were conducted with mixed or quantitative methods. In the two biggest categories, most of the studies utilized qualitative methods. Studies conducted with mixed or quantitative methods mainly concentrated on two categories: student learning and learning opportunities and student motivation, interests, attitudes, engagement, and mindset. In the following section, the research interests and findings for each category will be presented in detail.
Nearly half of the reviewed studies ( n = 30) had a research interest in either student learning through making activities in general or learning opportunities provided by such activities. Five qualitative case studies (Giusti & Bombieri, 2020 ; Hachey et al., 2022 ; Hagerman et al., 2022 ; Hartikainen et al., 2023 ; Morado et al., 2021 ) and two mixed method studies (Martin et al., 2020 ; Vuopala et al., 2020 ) investigated the overall educational value of maker education. One of these studies was conducted in early childhood education (Hachey et al., 2022 ), and two in the context of inclusion in primary and lower secondary education (Giusti & Bombieri, 2020 ; Martin et al., 2020 ). They all reported positive findings on the development of children’s identity formation and skills beyond subject-specific competencies, such as creativity, innovation, cultural literacy, and learning skills. The studies conducted in the context of inclusion especially emphasized the potential of maker education in pushing students with special needs to achieve goals exceeding their supposed cognitive abilities (Giusti & Bombieri, 2020 ; Martin et al., 2020 ). Three studies (Forbes et al., 2021 ; Kumpulainen et al., 2020 ; Xiang et al., 2023 ) investigated student learning through the Maker Literacies Framework (Marsh et al., 2018 ). They also reported positive findings on student learning and skill development in early childhood and primary education, especially on the operational dimension of the framework, as well as on the cultural and critical dimensions. These positive results were further confirmed by the reviewed studies that investigated more specific learning opportunities provided by maker education on developing young people’s creativity, innovation skills, design thinking and entrepreneurship (Liu & Li, 2023 ; Timotheou & Ioannou, 2021 ; Weng et al., 2022a , b ), as well as their 21st-century skills (Iwata et al., 2020 ; Tan et al., 2021 ), and critical data literacies and critical thinking (Stornaiuolo, 2020 ; Weng et al., 2022a ).
Studies that investigated subject-specific learning most often focused on STEM subjects or programming and computational thinking. Based on the findings of these studies, maker-centered learning activities are effective but underused (Mørch et al., 2023 ). Furthermore, in early childhood education, such activities may support children taking on the role of a STEM practitioner (Hachey et al., 2022 ) and, on the other hand, provide them access to learning about STEM subjects beyond their grade level, even in upper secondary education (Tofel-Grehl et al., 2021 ; Winters et al., 2022 ). However, two studies (Falloon et al., 2022 ; Forbes et al., 2021 ) highlighted that it cannot be assumed that students naturally learn science and mathematics conceptual knowledge through making. To achieve learning in STEM subjects, especially science and mathematics, teachers need to specifically identify, design, and focus the making tasks on these areas. One study also looked at the effects of the COVID-19 pandemic on STEM disciplines and found the restrictions on the use of common makerspaces and the changes in the technologies used to have been detrimental to student’s learning in these areas (Dúo-Terrón et al., 2022 ).
Only positive findings emerged from the reviewed studies on how digital making activities promote the development of programming and computational thinking skills and practices (Iwata et al., 2020 ; Liu & Li, 2023 ; Yin et al., 2020 ) and understanding of programming methods used in AI and machine learning (Ng et al., 2023 ). Experiences of fun provided by the making activities were also found to enhance further student learning about programming (Tisza & Markopoulos, 2021 ). One study also reported positive results on student learning of academic writing skills (Stewart et al., 2023 ). There were also three studies (Brownell, 2020 ; Greenberg et al., 2020 ; Wargo, 2021 ) that investigated the potential of maker education to promote equity and learning about social justice and injustice, as well as one study that examined learning opportunities on sustainability (Impedovo & Cederqvist, 2023 ). All these studies found making activities and makerspaces to be fertile ground for learning as well as identity and community building around these topics.
The studies with research interests in the second largest category, facilitation and teaching practices ( n = 13), investigated a multitude of different aspects of this area. The studies on assessment methods highlighted the educational value of process-based portfolios (Fields et al., 2021 ; Riikonen, Kangas et al., 2020 ) and connected portfolios that are digital portfolios aligned with a connected learning framework (Keune et al., 2022 ). On the other hand, Walan and Brink ( 2023 ) concentrated on developing and analyzing the outcomes of a self-assessment tool for maker-centered learning activities designed to promote 21st-century skills. Several research interests emerged from the review related to scaffolding and implementation of maker education in schools. Riikonen, Kangas, and colleagues ( 2020 ) investigated the pedagogical infrastructures of knowledge-creating, maker-centered learning. It emphasized longstanding iterative, socio-material projects, where real-time support and embedded scaffolding are provided to the participants by a multi-disciplinary teacher team and ideally also by peer tutors. Multi-disciplinary collaboration was also emphasized by Pitkänen and colleagues ( 2020 ) in their study on the role of facilitators as educators in Fab Labs. Cross-age peer tutoring was investigated by five studies and found to be highly effective in promoting learning in maker education (Kumpulainen et al., 2020 ; Riikonen, Kangas, et al., 2020 ; Tenhovirta et al., 2022 ; Weng et al., 2022a ; Winters et al., 2022 ). Kajamaa and colleagues ( 2020 ) further highlighted the importance of team teaching and emphasized moving from authoritative interaction with students to collaboration. Sormunen and colleagues’ ( 2020 ) findings on teacher support in an inclusive setting demonstrated how teacher-directed scaffolding and facilitation of student cooperation and reflective discussions are essential in promoting inclusion-related participation, collaboration skills, and student competence building. One study (Andersen et al., 2022 ) took a different approach and investigated the possibilities of automatic scaffolding of making activities through AI. They concluded that automated scaffolding has excellent potential in maker education and went as far as to suggest that a transition should be made to it. One study also recognized the potential of combining making activities with drama education (Walan, 2021 ).
Versatile aspects of different processes, activities, and practices in maker-centered learning projects were studied by 11 qualitative studies included in this review. Two interlinked studies (Davies et al., 2023 ; Riikonen, Seitamaa-Hakkarainen et al., 2020 ) investigated practices and processes related to collaborative invention, making, and knowledge-creation in lower secondary education. Their findings highlighted the multifaceted and iterative nature of such processes as well as the potential of maker education to offer students authentic opportunities for knowledge creation. Sinervo and colleagues ( 2021 ) also investigated the nature of the co-invention processes from the point of view of how children themselves describe and reflect their own processes. Their findings showed how children could recognize different external constraints involved in their design and the importance of iterative ideation processes and testing the ideas through prototyping. Innovation and invention practices were also studied by two other studies in both formal and informal settings with children from the primary level of education (Leskinen et al., 2023 ; Skåland et al., 2020 ). Skåland and colleagues’ ( 2020 ) findings suggest that narrative framing, that is, storytelling with the children, is an especially fruitful approach in a library setting and helps children understand their process of inventing. Similar findings were made in the study on the role of play in early childhood maker education (Fleer, 2022 ), where play enhanced design cognition and related processes and helped young children make sense of design. On the other hand, Leskinen and colleagues ( 2023 ) showed how innovations are jointly practiced in the interaction between students and teachers. They also emphasized the importance of using manifold information sources and material elements in creative innovation processes.
One study (Kajamaa & Kumpulainen, 2020 ) investigated collaborative knowledge practices and how those are mediated in school makerspaces. They identified four types of knowledge practices involved in maker-centered learning activities: orienting, interpreting, concretizing, and expanding knowledge, and how discourse, materials, embodied actions, and the physical space mediate these practices. Their findings also showed that due to the complexity of these practices, students might find maker-centered learning activities difficult. The sophisticated epistemic practices involved in collaborative invention processes were also demonstrated by the findings of Mehto, Riikonen, Hakkarainen, and colleagues ( 2020a ). Other investigators examined how art-based (Lindberg et al., 2020 ), touch-related (Friend & Mills, 2021 ), and information (Li, 2021 ) practices affect and can be incorporated into making. All three studies reported positive findings on the effects of these practices on student learning and, on the other hand, on the further development of the practices themselves.
Research interests related to student motivation, interests, attitudes, engagement, and mindset were studied by eight reviewed articles, all conducted with either mixed (n = 6) or quantitative methods (n = 2). The studies that investigated student motivation and engagement in making activities (Lee, 2021 ; Martin et al., 2020 ; Ng et al., 2023 ; Nikou, 2023 ) highlighted the importance of social interactions and collaboration as highly influential factors in these areas. On the other hand, positive attitudes towards collaboration also developed through these activities (Nguyen et al., 2023 ). Making activities conducted in the context of equity-oriented pedagogy were found to have great potential in sustaining non-dominant youths’, especially girls’, positive attitudes toward science (Hsu et al., 2022 ). On the other hand, a similar potential was not found in the development of interest in STEM subjects with autistic students (Martin et al., 2020 ). Two studies investigated student mindsets in maker-centered learning activities (Doss & Bloom, 2023 ; Vongkulluksn et al., 2021 ). Doss and Bloom ( 2023 ) identified seven different student mindset profiles present in making activities. Over half (56.67%) of the students in their study were found to share the same mindset profile, characterized as: ‘Flexible, Goal-Oriented, Persistent, Optimistic, Humorous, Realistic about Final Product’ (Doss & Bloom, 2023 , p. 4). In turn, Vongkulluksn and colleagues ( 2021 ) investigated the growth mindset trends for students who participated in a makerspace program for two years in an elementary school. Their findings revealed positive results of how makerspace environments can potentially improve students’ growth mindset.
Six studies included in this review analyzed collaboration within making activities. Students were found to be supportive and respectful towards each other as well as recognize and draw on each other’s expertise (Giusti & Bombieri, 2020 ; Herro et al., 2021a , b ). The making activities and outcomes were found to act as mediators in promoting mutual recognition between students with varying cognitive capabilities and special needs in inclusive settings (Herro et al., 2021a ). Furthermore, a community of interest that emerges through collaborative making activities was also found to be effective in supporting interest development and sustainability (Tan et al., 2021 ). Students were observed to divide work and share roles during their team projects, usually based on students’ interests, expertise, and skills (Herro et al., 2021a , b ). The findings of Stewart et al.‘s ( 2023 ) study suggested that when roles are preassigned to the team members by teachers, it decreases student stress in maker activities. However, if dominating leadership roles emerged in a team, that was found to lead to less advanced forms of collaboration than shared leadership within the team (Leskinen et al., 2021 ).
Sociomaterial aspects of making activities were in the interest of three reviewed studies (Kumpulainen & Kajamaa, 2020 ; Mehto et al., 2020a ; Mehto et al., 2020b ). Materials were shown to have an active role in knowledge-creation and ideation in open-ended maker-centered learning (Mehto et al., 2020a ), which allows for thinking together with the materials (Mehto et al., 2020b ). The task-related physical materials act as a focal point for team collaboration and invite participation (Mehto et al., 2020b ). Furthermore, a study by Kumpulainen and Kajamaa ( 2020 ) emphasized the sociomaterial dynamics of agency, where agency flows in any combination between students, teachers, and materials. However, the singularity or multiplicity of the materials potentially affects the opportunities for access and control of the process (Mehto et al., 2020b ).
In addition to empirical research interests, five studies focused on developing research methods for measuring and analyzing different aspects of maker education. Biometric measurements were investigated as a potential data source to detect engagement in making activities (Lee, 2021 ). Yin and colleagues ( 2020 ) focused on developing instruments for the quantitative measurement of computational thinking skills. On the other hand, Timotheou and Ioannou ( 2021 ) designed and tested an analytic framework and coding scheme to analyze learning and innovation skills from qualitative interviews and video data. Artificial intelligence as a potential, partially automated tool for analyzing CSCL artifacts was also investigated by one study (Andersen et al., 2022 ). Finally, Riikonen, Seitamaa-Hakkarainen, and colleagues ( 2020 ) developed visual video data analysis methods for investigating collaborative design and making activities.
Slightly over half of the reviewed studies ( n = 33) made clear suggestions for future research. Expectedly, these studies suggested further investigation of their own research interests. However, across the studies, five themes of recommendations for future research interests and designs emerged from the data:
1. Studies conducted with diverse range of participants , pedagogical designs , and contexts (Hartikainen et al., 2023 ; Kumpulainen & Kajamaa, 2020 ; Leskinen et al., 2023 ; Lindberg et al., 2020 ; Liu & Li, 2023 Martin et al., 2020 ; Mehto et al., 2020b ; Nguyen et al., 2023 ; Sormunen et al., 2020 ; Tan et al., 2021 ; Weng et al., 2022a , b ; Yin et al., 2020 ).
2. Longitudinal studies to confirm the existing research findings, further develop pedagogical approaches to making, and to better understand the effects of maker education on students later in their lives (Davies et al., 2023 ; Fields et al., 2021 ; Kumpulainen et al., 2020 ; Kumpulainen & Kajamaa, 2020 ; Stornaiuolo, 2020 ; Tisza & Markopoulos, 2021 ; Walan & Brink, 2023 ; Weng et al., 2022a ).
3. Development of new methods and applying existing methods in different conditions (Doss & Bloom, 2023 ; Kumpulainen et al., 2020 ; Leskinen et al., 2021 ; Mehto et al., 2020b ; Mørch et al., 2023 ; Tan et al., 2021 ; Timotheou & Ioannou, 2021 ; Tisza & Markopoulos, 2021 ).
4. Identifying optimal conditions and practices for learning, skill, and identity development through making (Davies et al., 2023 ; Fields et al., 2021 ; Hartikainen et al., 2023 ; Tofel-Grehl et al., 2021 ).
5. Collaboration from the perspectives of how it affects processes and outcomes of making activities and, on the other hand, how such activities affect collaboration (Pitkänen et al., 2020 ; Tisza & Markopoulos, 2021 ; Weng et al., 2022a ).
Discussion and conclusions
This systematic literature review was conducted to describe the development of research on maker education in the early 2020s. Sixty-two studies from the initial 700 studies identified from the three major educational research databases were included in the review. The qualitative analysis of the reviewed studies revealed some interesting developments in the field. Overall, the research on maker education appears to be active. Maker education seems to be attracting interest from researchers around the globe. However, two epicenters of research, North America and Scandinavia, namely Finland, appear to have an active role in maker research.
Most studies relied on rich qualitative data, often collected using several methods. Video recordings have become a popular way to collect data in maker education research. Although qualitative methods remained the dominant methodological approach in the field (Papavlasopoulou et al., 2017 ; Rouse & Rouse, 2022 ; Schad & Jones, 2020 ), mixed and quantitative methods were used in nearly a third of the reviewed studies. These studies mainly measured learning outcomes or participants’ motivation, interests, attitudes, engagement, and mindsets. There was a great variety in the duration of the maker projects and the number of participants. The projects lasted from less than a day up to five years, and the number of participants varied similarly from one to nearly six hundred. Methodological development was also within the research interests of several studies in this review. Developments were made both in qualitative and quantitative methodologies. Such methodological development was one of the research gaps identified in the previous literature reviews (e.g., Schad & Jones, 2020 ).
The analysis of the reviewed studies revealed an interesting shift in research on maker education from informal settings to formal education. Our review revealed that most studies were conducted exclusively in formal education and often as part of the curricular activity. The need for this development was called for in the previous literature reviews (Papavlasopoulou et al., 2017 ; Rouse & Rouse, 2022 ). However, only a handful of studies were conducted in early childhood education. Winters and colleagues’ ( 2022 ) study adopted a very interesting setting where children from early childhood education worked together and were mentored by students from lower secondary education. This type of research setting could have great potential for future research in maker education.
Another research gap identified in the previous literature reviews was the need to study and measure a wide variety of potential learning opportunities and outcomes of maker education (Lin et al., 2020 ; Rouse & Rouse, 2022 ; Schad & Jones, 2020 ). The analysis revealed that new research in the field is actively filling this gap. Skills that go beyond subject-specific content and the development of participants’ identities through making activities were especially actively studied from various perspectives. The findings of these studies were distinctively positive, corresponding with the conclusions of the previous literature reviews (e.g., Papavlasopoulou et al., 2017 ; Schad & Jones, 2020 ; Vossoughi & Bevan, 2014 ). This potential of maker education should be recognized by educators and policymakers, especially when the advancements in AI technologies will forefront the need for the humane skills of working creatively with knowledge and different ways of knowing, empathic engagement, and collaboration (e.g., Liu et al., 2024 ; Markauskaite et al., 2022 ; Qadir, 2023 ; World Economic Forum, 2023 ). Some of these studies also addressed the issue of promoting equity through maker education, which was called for in the previous literature review (Rouse & Rouse, 2022 ; Vossoughi & Bevan, 2014 ). However, considering the small number of these studies, more research will still be needed.
The two other popular research interest categories that emerged from the analysis were facilitation and teaching practices as well as processes, activities, and practices involved in making – both identified as research gaps in the previous literature reviews (Iivari et al., 2016 ; Papavlasopoulou et al., 2017 ; Rouse & Rouse, 2022 ; Schad & Jones, 2020 ; Vossoughi & Bevan, 2014 ). The teaching practices and scaffolding of making activities were investigated from different aspects, such as assessment methods, implementation of maker education in schools, and cross-age peer tutoring. The results of these studies highlighted the positive effects of multi-disciplinary collaboration and peer tutoring. Such pedagogical approaches should be more widely promoted as integral parts of the pedagogical infrastructure in schools. However, this calls for measures from policymakers and school authorities to enable such collaborative ways of teaching that extend beyond the traditional structures of school organizations. Furthermore, although research on this area has been active and multi-faceted, the facilitation of maker education in inclusive settings especially calls for further investigation. In terms of processes, practices, and activities involved in making, the reviewed studies investigated a variety of aspects that revealed the sophisticated epistemic practices involved and the importance of concrete making, prototyping, and iterative ideation in maker-centered learning activities. These studies further highlighted the potential of maker education to offer students authentic opportunities for knowledge creation. Studies also examined collaboration and sociomateriality involved in maker education. Especially sociomateriality is a relatively new, emerging area of research in maker education.
The reviewed studies identified five research gaps that require further investigation: (1) conducting studies with a diverse range of participants, pedagogical designs, and contexts; (2) carrying out longitudinal studies; (3) developing new methods and applying existing methods in different settings; (4) identifying the most effective conditions and practices for learning, skill development and identity formation in maker education, and (5) understanding how collaboration affects the processes and outcomes of making activities and vice versa. In addition to the research gaps identified by reviewed studies, the analysis revealed additional gaps. Studies conducted in early childhood education and inclusive settings remain especially under-represented, although maker pedagogies have been found to have great potential in these areas. Similarly, many researchers have recognized the potential of maker education to promote equality between children from different backgrounds and genders. Still, only a handful of studies investigated these issues. Thus, more research is needed, especially on best practices and pedagogical approaches in this area. Furthermore, the processes involved in and affecting maker-centered learning call for further investigations.
The field has matured based on the analysis of the reviewed studies. It is moving from striving to understand what can be achieved to investigating the underlying conditions behind learning through making, how desired outcomes can be best achieved, as well as how the processes involved in making unfold, what the effects are in the long run, and how to understand best and measure different phenomena related to making. Furthermore, researchers are looking into more and more opportunities to expand the learning opportunities of maker education by combining them with other creative pedagogies and applying them to projects that seek to introduce subject-specific content beyond STEM to students.
This systematic literature review has several limitations. The typical limitations of most review studies, the potential loss of search results due to limited search terms and databases used, apply to this review. For example, more culturally diverse search results might have been reached with the addition of other databases and further search terms. However, the search string was carefully designed and tested to include as many common terms used in maker education research as possible, including possible variations. Furthermore, the three databases used in the search, Scopus, ERIC, and EBSCO, are regarded as the most comprehensive databases of educational research available. Thus, although some studies might not have been identified because of these limitations, it can be assumed that this review gives a comprehensive enough snapshot of research on maker education in the early years of the 2020s.
Andersen, R., Mørch, A. I., & Litherland, K. T. (2022). Collaborative learning with block-based programming: Investigating human-centered artificial intelligence in education. Behaviour & Information Technology , 41 (9), 1830–1847. https://doi.org/10.1080/0144929X.2022.2083981
Article Google Scholar
Blikstein, P. (2013). Digital fabrication and ‘making’ in education: The democratization of invention. In C. Büching & J. Walter-Herrmann (Eds.), FabLabs: Of machines, makers and inventors (pp. 203–222). Transcript Publishers. https://doi.org/10.1515/transcript.9783839423820.203
Brownell, C. J. (2020). Keep walls down instead of up: Interrogating writing/making as a vehicle for black girls’ literacies. Education Sciences , 10 (6), 159. https://doi.org/10.3390/educsci10060159
Chawla, L., & Heft, H. (2002). Children’s competence and the ecology of communities: A functional approach to the evaluation of participation. Journal of Environmental Psychology , 22 (1–2), 201–216. https://doi.org/10.1006/jevp.2002.0244
Critical Appraisal Skills Programme (2023). CASP Qualitative Studies Checklist . https://casp-uk.net/checklists/casp-qualitative-studies-checklist-fillable.pdf
Davies, S., Seitamaa-Hakkarainen, P., & Hakkarainen, K. (2023). Idea generation and knowledge creation through maker practices in an artifact-mediated collaborative invention project. Learning, Culture and Social Interaction, 39 , 100692. https://doi.org/10.1016/j.lcsi.2023.100692
Doss, K., & Bloom, L. (2023). Mindset and the desire for feedback during creative tasks. Journal of Creativity , 33 (1), 100047. https://doi.org/10.1016/j.yjoc.2023.100047
Dúo-Terrón, P., Hinojo-Lucena, F. J., Moreno-Guerrero, A. J., & López-Belmonte, J. (2022). Impact of the pandemic on STEAM disciplines in the sixth grade of primary education. European Journal of Investigation in Health Psychology and Education , 12 (8), 989–1005. https://doi.org/10.3390/ejihpe12080071
Falloon, G., Forbes, A., Stevenson, M., Bower, M., & Hatzigianni, M. (2022). STEM in the making? Investigating STEM learning in junior school makerspaces. Research in Science Education , 52 (2), 511–537. https://doi.org/10.1007/s11165-020-09949-3
Fields, D. A., Lui, D., Kafai, Y. B., Jayathirtha, G., Walker, J., & Shaw, M. (2021). Communicating about computational thinking: Understanding affordances of portfolios for assessing high school students’ computational thinking and participation practices. Computer Science Education , 31 (2), 224–258. https://doi.org/10.1080/08993408.2020.1866933
Fleer, M. (2022). The genesis of design: Learning about design, learning through design to learning design in play. International Journal of Technology and Design Education , 32 (3), 1441–1468. https://doi.org/10.1007/s10798-021-09670-w
Forbes, A., Falloon, G., Stevenson, M., Hatzigianni, M., & Bower, M. (2021). An analysis of the nature of young students’ STEM learning in 3D technology-enhanced makerspaces. Early Education and Development , 32 (1), 172–187. https://doi.org/10.1080/10409289.2020.1781325
Friend, L., & Mills, K. A. (2021). Towards a typology of touch in multisensory makerspaces. Learning Media and Technology , 46 (4), 465–482. https://doi.org/10.1080/17439884.2021.1928695
Giusti, T., & Bombieri, L. (2020). Learning inclusion through makerspace: A curriculum approach in Italy to share powerful ideas in a meaningful context. The International Journal of Information and Learning Technology , 37 (3), 73–86. https://doi.org/10.1108/IJILT-10-2019-0095
Greenberg, D., Calabrese Barton, A., Tan, E., & Archer, L. (2020). Redefining entrepreneurialism in the maker movement: A critical youth approach. Journal of the Learning Sciences , 29 (4–5), 471–510. https://doi.org/10.1080/10508406.2020.1749633
Hachey, A. C., An, S. A., & Golding, D. E. (2022). Nurturing kindergarteners’ early STEM academic identity through makerspace pedagogy. Early Childhood Education Journal , 50 (3), 469–479. https://doi.org/10.1007/s10643-021-01154-9
Haddaway, N. R., Page, M. J., Pritchard, C. C., & McGuinness, L. A. (2022). PRISMA2020: An R package and Shiny app for producing PRISMA 2020-compliant flow diagrams, with interactivity for optimised digital transparency and open synthesis. Campbell Systematic Reviews , 18 (2). https://doi.org/10.1002/cl2.1230
Hagerman, M. S., Cotnam-Kappel, M., Turner, J. A., & Hughes, J. M. (2022). Literacies in the making: Exploring elementary students’ digital-physical meaning-making practices while crafting musical instruments from recycled materials. Technology Pedagogy and Education , 31 (1), 63–84. https://doi.org/10.1080/1475939X.2021.1997794
Hartikainen, H., Ventä-Olkkonen, L., Kinnula, M., & Iivari, N. (2023). We were proud of our idea: How teens and teachers gained value in an entrepreneurship and making project. International Journal of Child-Computer Interaction , 35 , 100552. https://doi.org/10.1016/j.ijcci.2022.100552
Herro, D., Quigley, C., & Abimbade, O. (2021a). Assessing elementary students’ collaborative problem-solving in makerspace activities. Information and Learning Sciences , 122 (11/12), 774–794. https://doi.org/10.1108/ILS-08-2020-0176
Herro, D., Quigley, C., Plank, H., & Abimbade, O. (2021b). Understanding students’ social interactions during making activities designed to promote computational thinking. The Journal of Educational Research , 114 (2), 183–195. https://doi.org/10.1080/00220671.2021.1884824
Hsu, P. S., Lee, E. M., & Smith, T. J. (2022). Exploring the influence of equity-oriented pedagogy on non-dominant youths’ attitudes toward science through making. RMLE Online , 45 (8), 1–16. https://doi.org/10.1080/19404476.2022.2116668
Iivari, N., Molin-Juustila, T., & Kinnula, M. (2016). The future digital innovators: Empowering the young generation with digital fabrication and making completed research paper. Proceedings of the 37th International Conference on Information Systems, ICIS 2016. 2 .
Impedovo, M., & Cederqvist, A. M. (2023). Socio-(im)material-making activities in minecraft: Retracing digital literacy applied to ESD. Research in Science & Technological Education , 1–21. https://doi.org/10.1080/02635143.2023.2245355
International Organization for Standardization (2020). ISO 3166-2:2020 - Codes for the representation of names of countries and their subdivisions — Part 2: Country subdivision code . https://www.iso.org/standard/72483.html
Iwata, M., Pitkänen, K., Laru, J., & Mäkitalo, K. (2020). Exploring potentials and challenges to develop twenty-first century skills and computational thinking in K-12 maker education. Frontiers in Education , 5 . https://doi.org/10.3389/feduc.2020.00087
Kafai, Y. B. (1996). Learning through artifacts: Communities of practice in classrooms. AI and Society , 10 (1), 89–100. https://doi.org/10.1007/BF02716758
Kafai, Y. B., & Peppler, K. A. (2011). Youth, technology, and DIY: Developing participatory competencies in creative media production. Review of Research in Education , 35 (1), 89–119. https://doi.org/10.3102/0091732X10383211
Kafai, Y., Fields, D. A., & Searle, K. (2014). Electronic textiles as disruptive designs: Supporting and challenging maker activities in schools. Harvard Educational Review , 84 (4), 532–556. https://doi.org/10.17763/haer.84.4.46m7372370214783
Kajamaa, A., & Kumpulainen, K. (2020). Students’ multimodal knowledge practices in a makerspace learning environment. International Journal of Computer-Supported Collaborative Learning , 15 (4), 411–444. https://doi.org/10.1007/s11412-020-09337-z
Kajamaa, A., Kumpulainen, K., & Olkinuora, H. (2020). Teacher interventions in students’ collaborative work in a technology-rich educational makerspace. British Journal of Educational Technology , 51 (2), 371–386. https://doi.org/10.1111/bjet.12837
Kangas, K., Seitamaa-Hakkarainen, P., & Hakkarainen, K. (2013). Figuring the world of designing: Expert participation in elementary classroom. International Journal of Technology and Design Education, 23 (2), 425–442. https://doi.org/10.1007/s10798-011-9187-z
Keune, A., Peppler, K., & Dahn, M. (2022). Connected portfolios: Open assessment practices for maker communities. Information and Learning Sciences , 123 (7/8), 462–481. https://doi.org/10.1108/ILS-03-2022-0029
Koh, J. H. L., Chai, C. S., Wong, B., & Hong, H. Y. (2015). Design thinking for education: Conceptions and applications in teaching and learning . Springer. https://doi.org/10.1007/978-981-287-444-3
Kumpulainen, K., & Kajamaa, A. (2020). Sociomaterial movements of students’ engagement in a school’s makerspace. British Journal of Educational Technology , 51 (4), 1292–1307. https://doi.org/10.1111/bjet.12932
Kumpulainen, K., Kajamaa, A., Leskinen, J., Byman, J., & Renlund, J. (2020). Mapping digital competence: Students’ maker literacies in a school’s makerspace. Frontiers in Education , 5 . https://doi.org/10.3389/feduc.2020.00069
Lee, V. R. (2021). Youth engagement during making: Using electrodermal activity data and first-person video to generate evidence-based conjectures. Information and Learning Sciences , 122 (3/4), 270–291. https://doi.org/10.1108/ILS-08-2020-0178
Leskinen, J., Kajamaa, A., & Kumpulainen, K. (2023). Learning to innovate: Students and teachers constructing collective innovation practices in a primary school’s makerspace. Frontiers in Education , 7 . https://doi.org/10.3389/feduc.2022.936724
Leskinen, J., Kumpulainen, K., Kajamaa, A., & Rajala, A. (2021). The emergence of leadership in students’ group interaction in a school-based makerspace. European Journal of Psychology of Education , 36 (4), 1033–1053. https://doi.org/10.1007/s10212-020-00509-x
Lindberg, L., Fields, D. A., & Kafai, Y. B. (2020). STEAM maker education: Conceal/reveal of personal, artistic and computational dimensions in high school student projects. Frontiers in Education , 5 . https://doi.org/10.3389/feduc.2020.00051
Lin, Q., Yin, Y., Tang, X., Hadad, R., & Zhai, X. (2020). Assessing learning in technology-rich maker activities: A systematic review of empirical research. Computers and Education , 157 . https://doi.org/10.1016/j.compedu.2020.103944
Liu, S., & Li, C. (2023). Promoting design thinking and creativity by making: A quasi-experiment in the information technology course. Thinking Skills and Creativity , 49 , 101335. https://doi.org/10.1016/j.tsc.2023.101335
Liu, W., Fu, Z., Zhu, Y., Li, Y., Sun, Y., Hong, X., Li, Y., & Liu, M. (2024). Co-making the future: Crafting tomorrow with insights and perspectives from the China-U.S. young maker competition. International Journal of Technology and Design Education . https://doi.org/10.1007/s10798-024-09887-5
Li, X. (2021). Young people’s information practices in library makerspaces. Journal of the Association for Information Science and Technology , 72 (6), 744–758. https://doi.org/10.1002/asi.24442
Markauskaite, L., Marrone, R., Poquet, O., Knight, S., Martinez-Maldonado, R., Howard, S., Tondeur, J., De Laat, M., Buckingham Shum, S., Gašević, D., & Siemens, G. (2022). Rethinking the entwinement between artificial intelligence and human learning: What capabilities do learners need for a world with AI? Computers and Education: Artificial Intelligence , 3 . https://doi.org/10.1016/j.caeai.2022.100056
Marsh, J., Arnseth, H., & Kumpulainen, K. (2018). Maker literacies and maker citizenship in the MakEY (makerspaces in the early years) project. Multimodal Technologies and Interaction , 2 (3), 50. https://doi.org/10.3390/mti2030050
Martin, L. (2015). The promise of the maker movement for education. Journal of Pre-College Engineering Education Research , 5 (1), 30–39. https://doi.org/10.7771/2157-9288.1099
Martin, W. B., Yu, J., Wei, X., Vidiksis, R., Patten, K. K., & Riccio, A. (2020). Promoting science, technology, and engineering self-efficacy and knowledge for all with an autism inclusion maker program. Frontiers in Education , 5 . https://doi.org/10.3389/feduc.2020.00075
Mehto, V., Riikonen, S., Hakkarainen, K., Kangas, K., & Seitamaa‐Hakkarainen, P. (2020a). Epistemic roles of materiality within a collaborative invention project at a secondary school. British Journal of Educational Technology, 51 (4), 1246–1261. https://doi.org/10.1111/bjet.12942
Mehto, V., Riikonen, S., Kangas, K., & Seitamaa-Hakkarainen, P. (2020b). Sociomateriality of collaboration within a small team in secondary school maker-centered learning project. International Journal of Child-Computer Interaction , 26. https://doi.org/10.1016/j.ijcci.2020.100209
Morado, M. F., Melo, A. E., & Jarman, A. (2021). Learning by making: A framework to revisit practices in a constructionist learning environment. British Journal of Educational Technology , 52 (3), 1093–1115. https://doi.org/10.1111/bjet.13083
Mørch, A. I., Flø, E. E., Litherland, K. T., & Andersen, R. (2023). Makerspace activities in a school setting: Top-down and bottom-up approaches for teachers to leverage pupils’ making in science education. Learning Culture and Social Interaction , 39 , 100697. https://doi.org/10.1016/j.lcsi.2023.100697
Ng, D. T. K., Su, J., & Chu, S. K. W. (2023). Fostering secondary school students’ AI literacy through making AI-driven recycling bins. Education and Information Technologies , 1–32. https://doi.org/10.1007/s10639-023-12183-9
Nguyen, H. B. N., Hong, J. C., Chen, M. L., Ye, J. N., & Tsai, C. R. (2023). Relationship between students’ hands-on making self-efficacy, perceived value, cooperative attitude and competition preparedness in joining an iSTEAM contest. Research in Science & Technological Education , 41 (1), 251–270. https://doi.org/10.1080/02635143.2021.1895100
Nikou, S. A. (2023). Student motivation and engagement in maker activities under the lens of the activity theory: A case study in a primary school. Journal of Computers in Education . https://doi.org/10.1007/s40692-023-00258-y
Ouzzani, M., Hammady, H., Fedorowicz, Z., & Elmagarmid, A. (2016). Rayyan – a web and mobile app for systematic reviews. Systematic Reviews , 5 (1), 210. https://doi.org/10.1186/s13643-016-0384-4
Page, M. J., McKenzie, J. E., Bossuyt, P. M., Boutron, I., Hoffmann, T. C., Mulrow, C. D., Shamseer, L., Tetzlaff, J. M., Akl, E. A., Brennan, S. E., Chou, R., Glanville, J., Grimshaw, J. M., Hróbjartsson, A., Lalu, M. M., Li, T., Loder, E. W., Mayo-Wilson, E., McDonald, S., & Moher, D. (2021). The PRISMA 2020 statement: An updated guideline for reporting systematic reviews. Systematic Reviews , 10 (1), 89. https://doi.org/10.1186/s13643-021-01626-4
Papavlasopoulou, S., Giannakos, M. N., & Jaccheri, L. (2017). Empirical studies on the Maker Movement, a promising approach to learning: A literature review. Entertainment Computing , 18 , 57–78. https://doi.org/10.1016/j.entcom.2016.09.002
Papert, S. (1980). Mindstroms: Children, computers, and powerful ideas . Basic Books.
Pitkänen, K., Iwata, M., & Laru, J. (2020). Exploring technology-oriented fab lab facilitators’ role as educators in K-12 education: Focus on scaffolding novice students’ learning in digital fabrication activities. International Journal of Child-Computer Interaction , 26 , 100207. https://doi.org/10.1016/j.ijcci.2020.100207
Qadir, J. (2023). Engineering education in the era of ChatGPT: Promise and pitfalls of generative AI for education. IEEE Global Engineering Education Conference, EDUCON , 2023-May . https://doi.org/10.1109/EDUCON54358.2023.10125121
Resnick, M. (2017). Lifelong kindergarten: Cultivating creativity through projects, passions, peers, and play . MIT Press.
Riikonen, S., Kangas, K., Kokko, S., Korhonen, T., Hakkarainen, K., & Seitamaa-Hakkarainen, P. (2020). The development of pedagogical infrastructures in three cycles of maker-centered learning projects. Design and Technology Education: An International Journal, 25 (2), 29–49.
Riikonen, S., Seitamaa-Hakkarainen, P., & Hakkarainen, K. (2020). Bringing maker practices to school: Tracing discursive and materially mediated aspects of student teams’ collaborative making processes. International Journal of Computer-Supported Collaborative Learning, 15 (3), 319–349. https://doi.org/10.1007/s11412-020-09330-6
Rouse, R., & Rouse, A. G. (2022). Taking the maker movement to school: A systematic review of preK-12 school-based makerspace research. Educational Research Review , 35 . Elsevier Ltd. https://doi.org/10.1016/j.edurev.2021.100413
Schad, M., & Jones, W. M. (2020). The maker movement and education: A systematic review of the literature. Journal of Research on Technology in Education , 52 (1), 65–78. https://doi.org/10.1080/15391523.2019.1688739
Sinervo, S., Sormunen, K., Kangas, K., Hakkarainen, K., Lavonen, J., Juuti, K., Korhonen, T., & Seitamaa-Hakkarainen, P. (2021). Elementary school pupils’ co-inventions: Products and pupils’ reflections on processes. International Journal of Technology and Design Education, 31 (4), 653–676. https://doi.org/10.1007/s10798-020-09577-y
Skåland, G., Arnseth, H. C., & Pierroux, P. (2020). Doing inventing in the library. Analyzing the narrative framing of making in a public library context. Education Sciences , 10 (6), 158. https://doi.org/10.3390/educsci10060158
Sormunen, K., Juuti, K., & Lavonen, J. (2020). Maker-centered project-based learning in inclusive classes: Supporting students’ active participation with teacher-directed reflective discussions. International Journal of Science and Mathematics Education , 18 (4), 691–712. https://doi.org/10.1007/s10763-019-09998-9
Stewart, A., Yuan, J., Kale, U., Valentine, K., & McCartney, M. (2023). Maker activities and academic writing in a middle school science classroom. International Journal of Instruction , 16 (2), 125–144. https://doi.org/10.29333/iji.2023.1628a
Stornaiuolo, A. (2020). Authoring data stories in a media makerspace: Adolescents developing critical data literacies. Journal of the Learning Sciences , 29 (1), 81–103. https://doi.org/10.1080/10508406.2019.1689365
Tan, A. L., Jamaludin, A., & Hung, D. (2021). In pursuit of learning in an informal space: A case study in the Singapore context. International Journal of Technology and Design Education , 31 (2), 281–303. https://doi.org/10.1007/s10798-019-09553-1
Tenhovirta, S., Korhonen, T., Seitamaa-Hakkarainen, P., & Hakkarainen, K. (2022). Cross-age peer tutoring in a technology-enhanced STEAM project at a lower secondary school. International Journal of Technology and Design Education, 32 (3), 1701–1723. https://doi.org/10.1007/s10798-021-09674-6
Timotheou, S., & Ioannou, A. (2021). Learning and innovation skills in making contexts: A comprehensive analytical framework and coding scheme. Educational Technology Research and Development , 69 (6), 3179–3207. https://doi.org/10.1007/s11423-021-10067-8
Tisza, G., & Markopoulos, P. (2021). Understanding the role of fun in learning to code. International Journal of Child-Computer Interaction , 28 , 100270. https://doi.org/10.1016/j.ijcci.2021.100270
Tofel-Grehl, C., Ball, D., & Searle, K. (2021). Making progress: Engaging maker education in science classrooms to develop a novel instructional metaphor for teaching electric potential. The Journal of Educational Research , 114 (2), 119–129. https://doi.org/10.1080/00220671.2020.1838410
Tong, A., Flemming, K., McInnes, E., Oliver, S., & Craig, J. (2012). Enhancing transparency in reporting the synthesis of qualitative research: ENTREQ. BMC Medical Research Methodology , 12 (1), 181. https://doi.org/10.1186/1471-2288-12-181
UNESCO Institute for Statistics (2012). International standard classification of education: ISCED 2011 . https://uis.unesco.org/sites/default/files/documents/international-standard-classification-of-education-isced-2011-en.pdf
UNESCO Institute for Statistics (2021). Using ISCED Diagrams to Compare Education Systems . https://neqmap.bangkok.unesco.org/wp-content/uploads/2021/06/UIS-ISCED-DiagramsCompare-web.pdf
Vongkulluksn, V. W., Matewos, A. M., & Sinatra, G. M. (2021). Growth mindset development in design-based makerspace: A longitudinal study. The Journal of Educational Research , 114 (2), 139–154. https://doi.org/10.1080/00220671.2021.1872473
Vossoughi, S., & Bevan, B. (2014). Making and tinkering: A review of the literature. National Research Council Committee on Out of School Time STEM , 67 , 1–55.
Google Scholar
Vuopala, E., Guzmán Medrano, D., Aljabaly, M., Hietavirta, D., Malacara, L., & Pan, C. (2020). Implementing a maker culture in elementary school – students’ perspectives. Technology Pedagogy and Education , 29 (5), 649–664. https://doi.org/10.1080/1475939X.2020.1796776
Walan, S. (2021). The dream performance – a case study of young girls’ development of interest in STEM and 21st century skills, when activities in a makerspace were combined with drama. Research in Science & Technological Education , 39 (1), 23–43. https://doi.org/10.1080/02635143.2019.1647157
Walan, S., & Brink, H. (2023). Students’ and teachers’ responses to use of a digital self-assessment tool to understand and identify development of twenty-first century skills when working with makerspace activities. International Journal of Technology and Design Education . https://doi.org/10.1007/s10798-023-09845-7
Wargo, J. M. (2021). Sound civics, heard histories: A critical case of young children mobilizing digital media to write (right) injustice. Theory & Research in Social Education , 49 (3), 360–389. https://doi.org/10.1080/00933104.2021.1874582
Weng, X., Chiu, T. K. F., & Jong, M. S. Y. (2022a). Applying relatedness to explain learning outcomes of STEM maker activities. Frontiers in Psychology , 12 . https://doi.org/10.3389/fpsyg.2021.800569
Weng, X., Chiu, T. K. F., & Tsang, C. C. (2022b). Promoting student creativity and entrepreneurship through real-world problem-based maker education. Thinking Skills and Creativity , 45 , 101046. https://doi.org/10.1016/j.tsc.2022.101046
Winters, K. L., Gallagher, T. L., & Potts, D. (2022). Creativity, collaboration, and cross-age mentorships using STEM-infused texts. Elementary STEM Journal , 27 (2), 7–14.
World Economic Forum (2023). The future of jobs report 2023 . https://www.weforum.org/reports/the-future-of-jobs-report-2023/
Xiang, S., Yang, W., & Yeter, I. H. (2023). Making a makerspace for children: A mixed-methods study in Chinese kindergartens. International Journal of Child-Computer Interaction , 36 , 100583. https://doi.org/10.1016/j.ijcci.2023.100583
Yin, Y., Hadad, R., Tang, X., & Lin, Q. (2020). Improving and assessing computational thinking in maker activities: The integration with physics and engineering learning. Journal of Science Education and Technology , 29 (2), 189–214. https://doi.org/10.1007/s10956-019-09794-8
Yulis San Juan, A., & Murai, Y. (2022). Turning frustration into learning opportunities during maker activities: A review of literature. International Journal of Child-Computer Interaction , 33 , 100519. https://doi.org/10.1016/j.ijcci.2022.100519
Download references
Open Access funding provided by University of Helsinki (including Helsinki University Central Hospital). This work has been funded by the Strategic Research Council (SRC) established within the Research Council of Finland, grants #312527, #352859, and # 352971.
Open Access funding provided by University of Helsinki (including Helsinki University Central Hospital).
Author information
Authors and affiliations.
Faculty of Educational Sciences, University of Helsinki, Helsinki, Finland
Sini Davies & Pirita Seitamaa-Hakkarainen
You can also search for this author in PubMed Google Scholar
Corresponding author
Correspondence to Sini Davies .
Ethics declarations
Conflict of interest.
The authors have no relevant financial or non-financial interests to disclose.
Additional information
Publisher’s note.
Springer Nature remains neutral with regard to jurisdictional claims in published maps and institutional affiliations.
Rights and permissions
Open Access This article is licensed under a Creative Commons Attribution 4.0 International License, which permits use, sharing, adaptation, distribution and reproduction in any medium or format, as long as you give appropriate credit to the original author(s) and the source, provide a link to the Creative Commons licence, and indicate if changes were made. The images or other third party material in this article are included in the article’s Creative Commons licence, unless indicated otherwise in a credit line to the material. If material is not included in the article’s Creative Commons licence and your intended use is not permitted by statutory regulation or exceeds the permitted use, you will need to obtain permission directly from the copyright holder. To view a copy of this licence, visit http://creativecommons.org/licenses/by/4.0/ .
Reprints and permissions
About this article
Davies, S., Seitamaa-Hakkarainen, P. Research on K-12 maker education in the early 2020s – a systematic literature review. Int J Technol Des Educ (2024). https://doi.org/10.1007/s10798-024-09921-6
Download citation
Accepted : 02 July 2024
Published : 27 August 2024
DOI : https://doi.org/10.1007/s10798-024-09921-6
Share this article
Anyone you share the following link with will be able to read this content:
Sorry, a shareable link is not currently available for this article.
Provided by the Springer Nature SharedIt content-sharing initiative
- Maker education
- K-12 education
- Systematic literature review
- Maker-centered learning
- Maker culture
- Design and making
- Find a journal
- Publish with us
- Track your research
Information
- Author Services
Initiatives
You are accessing a machine-readable page. In order to be human-readable, please install an RSS reader.
All articles published by MDPI are made immediately available worldwide under an open access license. No special permission is required to reuse all or part of the article published by MDPI, including figures and tables. For articles published under an open access Creative Common CC BY license, any part of the article may be reused without permission provided that the original article is clearly cited. For more information, please refer to https://www.mdpi.com/openaccess .
Feature papers represent the most advanced research with significant potential for high impact in the field. A Feature Paper should be a substantial original Article that involves several techniques or approaches, provides an outlook for future research directions and describes possible research applications.
Feature papers are submitted upon individual invitation or recommendation by the scientific editors and must receive positive feedback from the reviewers.
Editor’s Choice articles are based on recommendations by the scientific editors of MDPI journals from around the world. Editors select a small number of articles recently published in the journal that they believe will be particularly interesting to readers, or important in the respective research area. The aim is to provide a snapshot of some of the most exciting work published in the various research areas of the journal.
Original Submission Date Received: .
- Active Journals
- Find a Journal
- Proceedings Series
- For Authors
- For Reviewers
- For Editors
- For Librarians
- For Publishers
- For Societies
- For Conference Organizers
- Open Access Policy
- Institutional Open Access Program
- Special Issues Guidelines
- Editorial Process
- Research and Publication Ethics
- Article Processing Charges
- Testimonials
- Preprints.org
- SciProfiles
- Encyclopedia
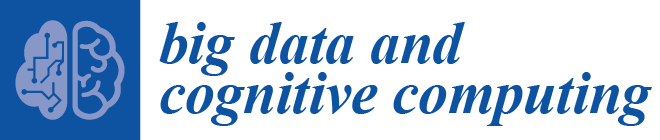
Article Menu
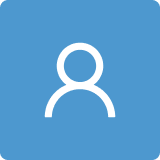
- Subscribe SciFeed
- Recommended Articles
- Google Scholar
- on Google Scholar
- Table of Contents
Find support for a specific problem in the support section of our website.
Please let us know what you think of our products and services.
Visit our dedicated information section to learn more about MDPI.
JSmol Viewer
Review of federated learning and machine learning-based methods for medical image analysis.
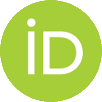
Share and Cite
Hernandez-Cruz, N.; Saha, P.; Sarker, M.M.K.; Noble, J.A. Review of Federated Learning and Machine Learning-Based Methods for Medical Image Analysis. Big Data Cogn. Comput. 2024 , 8 , 99. https://doi.org/10.3390/bdcc8090099
Hernandez-Cruz N, Saha P, Sarker MMK, Noble JA. Review of Federated Learning and Machine Learning-Based Methods for Medical Image Analysis. Big Data and Cognitive Computing . 2024; 8(9):99. https://doi.org/10.3390/bdcc8090099
Hernandez-Cruz, Netzahualcoyotl, Pramit Saha, Md Mostafa Kamal Sarker, and J. Alison Noble. 2024. "Review of Federated Learning and Machine Learning-Based Methods for Medical Image Analysis" Big Data and Cognitive Computing 8, no. 9: 99. https://doi.org/10.3390/bdcc8090099
Article Metrics
Further information, mdpi initiatives, follow mdpi.
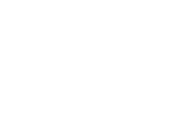
Subscribe to receive issue release notifications and newsletters from MDPI journals
- Computer Science and Engineering
- Computer Security and Reliability
- Cybersecurity
Machine Learning in Cybersecurity: Systematic Literature Review
- January 2024
- Conference: 22nd LACCEI International Multi-Conference for Engineering, Education and Technology (LACCEI 2024): “Sustainable Engineering for a Diverse, Equitable, and Inclusive Future at the Service of Education, Research, and Industry for a Society 5.0.”
- This person is not on ResearchGate, or hasn't claimed this research yet.
Discover the world's research
- 25+ million members
- 160+ million publication pages
- 2.3+ billion citations
No full-text available

To read the full-text of this research, you can request a copy directly from the authors.
- NEURAL NETWORKS

- Lord Anertei Tetteh

- INFORM FUSION

- Dušan Gabrijelčič

- Amit Kumar Mishra
- COMPUT SYST SCI ENG
- Abdullah Alshehri

- Ali Alowayr
- Mohammed Yahya Alghamdi

- COMPUT NETW

- Pablo Fernández Saura

- NEURAL COMPUT APPL

- Shareeful Islam

- Maruf Pasha

- NEUROCOMPUTING
- Guangxia Li
- Yulong Shen
- Peilin Zhao

- Carla Iglesias Comesaña

- Lingshuang Kong
- Chunhua Wang

- Nagarajan Ranganathan
- COMPUT ELECTR ENG
- Qinghui Liu
- Tianping Zhang
- Deepak Kumar Sharma
- Jahanavi Mishra
- Aeshit Singh
- Jerry Chun-Wei Lin

- Yunsheng Fu
- Brij B. Gupta

- INT J ELEC POWER
- Recruit researchers
- Join for free
- Login Email Tip: Most researchers use their institutional email address as their ResearchGate login Password Forgot password? Keep me logged in Log in or Continue with Google Welcome back! Please log in. Email · Hint Tip: Most researchers use their institutional email address as their ResearchGate login Password Forgot password? Keep me logged in Log in or Continue with Google No account? Sign up

IMAGES
COMMENTS
The literature review shows several studies on a variety of le. arning styles-interactive, social, innovative, experiential, game-based, self-regulated, integrated, and expeditionary le. arning ...
In this review of the literature on e-learning, we present and discuss definitions of e-learning, hybrid learning and blended learning, and we review the literature comparing different online teaching formats with traditional on-campus/face-to-face teaching. With this point of departure, we explore which factors affect students' learning ...
Example literature review #4: "Learners' Listening Comprehension Difficulties in English Language Learning: A Literature Review" (Chronological literature review about how the concept of listening skills has changed over time.) You can also check out our templates with literature review examples and sample outlines at the links below.
This systematic literature review aimed to provide updated information on lifelong learning in educational research by examining theoretical documents and empirical papers from 2000 to 2022. This review sought to identify concepts, theories, and research trends and methods linked to lifelong learning in educational research in different ...
This study is a literature review using meta-analysis. Meta-analysis is a review of research results systematic, especially on the results of research empirically related to online learning efficacy for designing and developing instructional materials that can provide wider access to quality higher education.
E-learning systems are receiving ever increasing attention in academia, business and public administration. Major crises, like the pandemic, highlight the tremendous importance of the appropriate development of e-learning systems and its adoption and processes in organizations. Managers and employees who need efficient forms of training and learning flow within organizations do not have to ...
A literature review is a critical analysis and synthesis of existing research on a particular topic. It provides an overview of the current state of knowledge, identifies gaps, and highlights key findings in the literature. 1 The purpose of a literature review is to situate your own research within the context of existing scholarship, demonstrating your understanding of the topic and showing ...
This review enabled us to identify the online learning research themes examined from 2009 to 2018. In the section below, we review the most studied research themes, engagement and learner characteristics along with implications, limitations, and directions for future research. 5.1. Most studied research themes.
1. Definition. Not to be confused with a book review, a literature review surveys scholarly articles, books and other sources (e.g. dissertations, conference proceedings) relevant to a particular issue, area of research, or theory, providing a description, summary, and critical evaluation of each work. The purpose is to offer an overview of significant literature published on a topic.
A literature review is a review and synthesis of existing research on a topic or research question. A literature review is meant to analyze the scholarly literature, make connections across writings and identify strengths, weaknesses, trends, and missing conversations. A literature review should address different aspects of a topic as it ...
The checklist represents the learning outcomes of the LR. First category: Coverage. 1. Justified criteria exist for the inclusion and exclusion of literature in the review . ... The broader scholarly literature should be related to the chosen main topic for the LR (how to develop the literature review section). The LR can cover the literature ...
There have been several literature review projects on active learning. However, all of them are narrative reviews, and this type of review does typically not aim to examine the internal validity of the studies in focus (Toronto, 2020).We argue that research quality appraisal should form an essential part of a literature review as this helps to mitigate bias in research.
What is a literature review? A literature review is a piece of academic writing demonstrating knowledge and understanding of the academic literature on a specific topic placed in context. A literature review also includes a critical evaluation of the material; this is why it is called a literature review rather than a literature report. It is a ...
Specifically, the research agenda includes the recommendation that future research considers (1) multiple motivation constructs, (2) behavioral mediators, (3) a network approach, (4) alignment of intervals of measurement and the short vs. long time scales of motivation constructs, (5) designs that meet the criteria for making causal, reciprocal ...
21. Sommerauer P, Müller O. Augmented reality for teaching and learning - A literature review on theoretical and empirical foundations. 26th European Conference on Information Systems: Beyond Digitization - Facets of Socio-Technical Change, ECIS 2018. 2018. 22. Diegmann Manuel Schmidt-Kraepelin Sven Eynden Dirk Basten P. Augmented Reality in ...
To address this limitation of the literature, this paper presents a systematic review and critique of literature in this field. Our review of 105 studies suggests that there are statistically significant relationships between different types of leadership and learning at the individual, group, and organizational levels.
Approaches to learning: Literature review 2 Some of the sources were obtained through the snowballing method by checking the references lists of the existing sources. Overview of this literature review In section 1, common educational objectives across national and international educational systems are reviewed. A balanced emphasis on knowledge ...
Bhutan first declared closing of schools and institutions and reduction of business hours during the second week of March 2020 (Kuensel, 2020, 6 March).The complete nationwide lockdown was implemented from 1 August 2020 (Palden, 2020).In between, movements were allowed, offices began functioning, schools and college reopened for selected levels and continued with online class for others.
A literature review with scientific mapping. ... Review of Educational Research 80(1): 71-107. Crossref. Web of Science. ... Hargreaves A (2007) Sustainable learning communities. In: Stoll L, Louis KS (eds) Professional Learning Communities: Divergence, Depth and Dilemmas. New York, NY: McGraw Hill (Open University Press, 181-195. ...
Improve teaching and learning quality. As Lowther et al. (2008) have stated that there are three important characteristics are needed to develop good quality teaching and learning with ICT: autonomy, capability, and creativity. Autonomy means that students take control of their learning through their use of ICT.
Abstract. Organizational learning (OL) enables organizations to transform individual knowledge into organizational knowledge. Organizations struggle to implement practical approaches due to the lack of concrete prescriptions. We performed a literature review to identify OL approaches and linked these approaches to OL theories.
setting. This systematic literature review examined the relationship between social-. emotional learning programs in schools and academic outcomes, such as grades, test. scores, or grade point averages. Secondly, it explored the relationship between students'. social-emotional skills and these academic outcomes.
Machine learning is a broad term encompassing a number of methods that allow the investigator to learn from the data. These methods may permit large real-world databases to be more rapidly translated to applications to inform patient-provider decision making. This systematic literature review was conducted to identify published observational research of employed machine learning to inform ...
Machine learning can enhance the analysis of these data, improving the comprehension of health and well-being. Therefore, this paper presents a systematic literature review on machine learning and digital phenotyping, examining the research field by filtering 2,860 articles from eleven databases published up to November 2023.
K, P. P., Mittal, M., Aggarwal, A. (2023) Literature Review: Learning Through Ga me-Based Technology Enhances Cognitive Skills. may be taught in a way that is very different from how it is taught ...
Recent technological advancements have enhanced our ability to collect and analyze rich multimodal data (e.g., speech, video, and eye gaze) to better inform learning and training experiences. While previous reviews have focused on parts of the multimodal pipeline (e.g., conceptual models and data fusion), a comprehensive literature review on the methods informing multimodal learning and ...
Background: Low levels of physical activity (PA) in adolescents highlight the necessity for effective intervention. During adolescence, peer relationships can be a fundamental aspect of adopting and maintaining positive health behaviors. Aim: This review aims to determine peer-led strategies that showed promise to improve PA levels of adolescents. It will also identify patterns across these ...
This systematic literature review focuses on the research published on K-12 maker education in the early 2020s, providing a current picture of the field. Maker education is a hands-on approach to learning that encourages students to engage in collaborative and innovative activities, using a combination of traditional design and fabrication tools and digital technologies to explore real-life ...
Federated learning is an emerging technology that enables the decentralised training of machine learning-based methods for medical image analysis across multiple sites while ensuring privacy. This review paper thoroughly examines federated learning research applied to medical image analysis, outlining technical contributions. We followed the guidelines of Okali and Schabram, a review ...
This article presents a systematic literature review and a detailed analysis of AI use cases for cybersecurity provisioning. The review resulted in 2395 studies, of which 236 were identified as ...