Click through the PLOS taxonomy to find articles in your field.
For more information about PLOS Subject Areas, click here .
Loading metrics
Open Access
Peer-reviewed
Research Article

The impact of the COVID-19 pandemic on higher education: Assessment of student performance in computer science
Roles Conceptualization, Data curation, Formal analysis, Investigation, Methodology, Software, Supervision, Validation, Writing – original draft, Writing – review & editing
* E-mail: [email protected]
Affiliations Department of Computer Science, Lublin University of Technology, Lublin, Poland, Systems Research Institute, Polish Academy of Sciences, Warsaw, Poland

Roles Conceptualization, Formal analysis, Software, Validation, Visualization, Writing – original draft, Writing – review & editing
Affiliation Department of Computer Science, Lublin University of Technology, Lublin, Poland
Roles Data curation, Software
- Małgorzata Charytanowicz,
- Magdalena Zoła,
- Waldemar Suszyński
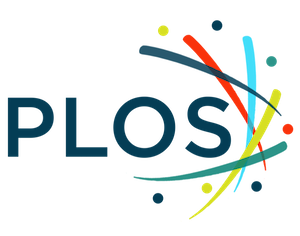
- Published: August 14, 2024
- https://doi.org/10.1371/journal.pone.0305763
- Reader Comments
The COVID-19 pandemic had radically changed higher education. The sudden transition to online teaching and learning exposed, however, some benefits by enhancing educational flexibility and digitization. The long-term effects of these changes are currently unknown, but a key question concerns their effect on student learning outcomes. This study aims to analyze the impact of the emergence of new models and teaching approaches on the academic performance of Computer Science students in the years 2019–2023. The COVID-19 pandemic created a natural experiment for comparisons in performance during in-person versus synchronous online and hybrid learning mode. We tracked changes in student achievements across the first two years of their engineering studies, using both basic (descriptive statistics, t-Student tests, Mann-Whitney test) and advanced statistical methods (Analysis of variance). The inquiry was conducted on 787 students of the Lublin University of Technology (Poland). Our findings indicated that first semester student scores were significantly higher when taught through online (13.77±2.77) and hybrid (13.7±2.86) approaches than through traditional in-person means as practiced before the pandemic (11.37±3.9, p-value < 0.05). Conversely, third semester student scores were significantly lower when taught through online (12.01±3.14) and hybrid (12.04±3.19) approaches than through traditional in-person means, after the pandemic (13.23±3.01, p-value < 0.05). However, the difference did not exceed 10% of a total score of 20 points. With regard to the statistical data, most of the questions were assessed as being difficult or appropriate, with adequate discrimination index, regardless of the learning mode. Based on the results, we conclude that we did not find clear evidence that pandemic disruption and online learning caused knowledge deficiencies. This critical situation increased students’ academic motivation. Moreover, we conclude that we have developed an effective digital platform for teaching and learning, as well as for a secure and fair student learning outcomes assessment.
Citation: Charytanowicz M, Zoła M, Suszyński W (2024) The impact of the COVID-19 pandemic on higher education: Assessment of student performance in computer science. PLoS ONE 19(8): e0305763. https://doi.org/10.1371/journal.pone.0305763
Editor: Prabhat Mittal, Satyawati College (Eve.), University of Delhi, INDIA
Received: October 15, 2023; Accepted: June 4, 2024; Published: August 14, 2024
Copyright: © 2024 Charytanowicz et al. This is an open access article distributed under the terms of the Creative Commons Attribution License , which permits unrestricted use, distribution, and reproduction in any medium, provided the original author and source are credited.
Data Availability: All relevant data are available at the following link: https://zenodo.org/records/11583297 .
Funding: The author(s) received no specific funding for this work.
Competing interests: The authors have declared that no competing interests exist.
1. Introduction
The COVID-19 pandemic brought with it a number of health, economic and social consequences. Indeed, the spread of the SARS-CoV-2 virus turned out to be so dangerous that many countries implemented new regulations in the educational field to limit physical contact. The pandemic-induced school shutdowns and sudden transition to remote teaching and learning at all levels of education. This change-over generated a number of technical and social problems [ 1 – 6 ]. These problems had also affected the academic community, although online or blended learning methods were implemented before the COVID-19 pandemic [ 7 ].
On March 12, 2020, a state of epidemic emergency was declared in Poland, and a week later–a state of pandemic. In consequence, the Minister of Science and Higher Education issued a regulation on the temporary suspension of the functioning of education institutes, lasting from March 12 till 25 2020 [ 8 , 9 ]. On March 25, 2020, the education system, including higher education, was switched to online teaching and learning, as necessitated by the need to maintain social distancing measures. Universities had to adapt to the circumstances almost overnight. However, many universities were not fully prepared with regard to technical capabilities, educational resources and the skills of the teaching staff in organizing distance education [ 10 – 12 ]. Before the COVID-19 pandemic, the applicable regulations of the Ministry of Science and Higher Education did not encourage the authorities of most universities to invest in technologies for conducting fully remote studies. Poland was, however, not an exception in this respect. Many old, prestigious universities in Europe were also reserved about remote learning, and the virtual learning environment was mainly used as a teaching aid.
Fortunately, the information revolution had by this time developed more flexible approaches to learning with the form of Information and Communication Technology (ICT). Indeed, it is one of the leading factors that affect current teaching methodology [ 13 – 18 ]. E-learning systems, their accessibility and functionality, have provided new possibilities to acquire knowledge and to ease the burden of learning. As an outcome, remote teaching and learning are often seen as promising solutions that offer high flexibility and a learner-centered approach that enables students to learn at their own pace [ 19 , 20 ]. Thus, the role of the teacher in the classroom has transformed from that of being the font of knowledge, to an instructional manager identifying relevant resources and creating collaborative learning opportunities. Moreover, online assessments have become increasingly important and now represent one of the most critical aspects of the educational process. Unfortunately, the role of ICT in higher education is still somewhat controversial.
The extreme situation caused by the COVID-19 pandemic provided an opportunity to revise our approach both to traditional and online learning, yet also posing challenges for the future of education systems. The main question of our research was whether the sudden transition to online teaching and learning caused by the COVID-19 pandemic had a negative impact on students academic performance and upon the reliability of the assessment process. We believe that our study can help to reduce the controversies related to remote learning and teaching.
2. Related works
Before the year 2020, the principal recipients of remote education were adults participating in professional development courses [ 21 ]. The COVID-19 pandemic outbreak, however, resulted in increased interest in methods of education that do not require physical meeting between students and teachers. The closure of educational institutions to mitigate the spread of COVID-19 compelled schools and universities to find alternative ways of continuing their operations. This led to the widespread adoption of online learning (e-learning).
The use of e-learning platforms has enabled the transformation of the traditional model of education in which the lecturer transmitted knowledge, into a model of supervised self-education. A separate line of research has been dedicated to the impact of remote education on university students, who are predominantly young adults, and, as such, are less subject to parental supervision. Topics under study include student attitudes towards distance learning [ 22 , 23 ], the technologies and learning platforms utilized [ 24 – 26 ], and the impact of network quality on the smoothness of classes [ 22 , 27 ].
A relatively well researched aspect of e-learning is the analysis of its advantages and disadvantages in comparison to traditional learning [ 28 – 30 ], including its application during the COVID-19 pandemic [ 31 – 34 ]. Undoubtedly, remote education has its benefits, among others, flexibility, speed, time savings [ 35 , 36 ], as well as better use of the infrastructure and organizational savings for the institution [ 37 ]. Distance learning in the form of e-learning also comes with drawbacks, for example, limited interpersonal contacts [ 38 ], lack of immediate feedback [ 39 , 40 ], and problems with self-discipline and adaptability [ 41 – 43 ]. Considering its strengths and weaknesses, e-learning can be viewed as either a replacement or augmentation of traditional approaches to education.
An integral part of remote education is the verification of its results. The topic was covered in literature in the pre-COVID era [ 44 – 46 ], but much less so during the pandemic [ 47 , 48 ]. Our work focuses on the analysis of student performance under the e-learning setup during COVID-19 related confinement and afterwards. The differentiating characteristic of this paper is the fact that it covers a longer period of time, unlike some other research focusing only on a single academic semester [ 49 ].
The COVID-19 pandemic has provided the opportunity to advance usage of online platforms and digital media, as well as to create new education strategies. It should be noted that most students (and instructors) adapted successfully to online teaching and learning [ 50 , 51 ]. However, certain studies [ 52 – 54 ] have indicated negative student feedback. In the year 2023, education has returned to more traditional teaching/learning approaches after more than two years of online learning.
The outbreak of COVID-19 presented a serious challenge to academic education by enforcing a drastic change in the teaching methods. For this reason, we formulated the following research questions:
- How had the COVID-19 pandemic change applied teaching and learning strategies?
- Did the COVID-19 pandemic have a disruptive effect on the academic performance of students resulting in knowledge deficiency?
- How did the change from in-person to online learning affect the reliability of student assessment?
The rest of the paper is structured as follows. Section 3 presents the context of the study, materials and methods. Section 4 explains the results obtained. Sections 5 and 6 conclude our work and describe limitations and future scope.
3. Materials and methods
3.1. design and context.
The research was conducted in the Department of Computer Science of the Lublin University of Technology in Poland, the largest public technical university in the Lublin voivodship. This was a cross-sectional study carried out among students who were enrolled in the first semester of engineering studies in the academic years 2019/2020, 2020/2021 and 2021/2022 (from October to July). Because of the COVID-19 pandemic, the courses of interest in this study were conducted in different delivery formats (in-person, synchronous online and hybrid).
Traditional in-person course delivery format included lectures and laboratories. The former involved, primarily, oral presentations given to a group of students. A teacher-centered approach to learning was applied with discussion and multimedia presentation, as well as whiteboard or chalkboard visual aids to emphasize important points in the lecture. Moreover, a Learning Management System (Moodle LMS) was incorporated within the lectures to develop, organize, deliver and manage didactic materials and assess the effectiveness of education via tests, surveys or assignments. This tool was also employed to provide discussion forums. The faculty used the activity Quiz as a student self-assessment tool, as well as to determine knowledge and skills.
With regard to laboratory work, practical classes were conducted in programming laboratories for the selected courses. In such a teaching/learning format, we found that most students preferred working alone or conducting discussions with their partners or their neighbors.
All students used online manuals or didactic materials delivered by Moodle LMS. Final exams were held at the University via Moodle LMS through in-person proctoring, as this approach allowed the introduction of a live person to monitor the activity of students in a testing environment.
In the synchronous online course format, students obtained theoretical and practical education entirely online via Microsoft Teams by way of video meetings and Moodle LMS. Meetings in Teams include audio, video and screen sharing. All lectures were delivered synchronously using MS Teams. Practical sessions were conducted through online synchronous video meetings in small student groups. Interaction occurred via the discussion board, while MS Teams was also employed to enable scheduled online consultations. Supporting materials (videos, presentations, tasks to do, quizzes, and other didactic materials) were provided to the students through the Moodle LMS. Final exams were conducted under controlled conditions via Moodle LMS through online live proctoring by accepting screen, video and audio sharing.
The hybrid course delivery format combined in-person and online strategies. Students obtained theoretical education entirely online as synchronous sessions by way of MS Teams and Moodle LMS, whilst practical education was obtained through the traditional in-person format, in small student groups. Final exams were held at the University via Moodle LMS through in-person proctoring.
We analyzed exam scores across the first two years of the engineering studies using anonymous data from the Moodle. The Research Ethics Committee of Lublin University of Technology approved the study (Ethical Approval Reference: 3/2023).
3.2. Course selection
The following criteria were used to select the courses:
- the courses covered algorithms and programming,
- the courses had unchanged objectives and learning outcomes during the investigated period,
- the courses were conducted by the same instructors using to the same tools and methods.
Two compulsory courses met these criteria: 1 –Introduction to Computer Science and 2 –Numerical Analysis Algorithms. Both courses were conducted in the Polish language and they provided fundamental knowledge for all areas of Computer Science learning and skills development. Enrolled students were obligated to complete 30 lesson hours of theory and 30 lesson hours of practical experience within a course length of 15 weeks. In the full-time option, four hours of classes were given each course week, and were distributed into two two-hour sessions. Herein, the first consisted of a master class lecture and the second consisted of an interactive problem-based learning laboratory. In the part-time option, the number of in-person teaching hours was reduced to half and classes were held, on average, twice a month, on Saturday and Sunday.
The Introduction to Computer Science course is taught in the first year and is covered in the first semester. Students who successfully completed the course gained five credits, according to the European Credit Transfer and Accumulation System (ECTS). The intention of the offered course is to provide students with knowledge of standard algorithms and data structures, and to provide them with the skills to analyze both the theoretical complexity of algorithms and their practical behaviors. The course covers the following topics:
- Introduction to algorithms and problem-solving techniques.
- Basic programming concepts, types, sequential data structures.
- Programming in Python.
- Searching and sorting algorithms.
- Examples of algorithms, algorithmic strategies.
- Testing and documenting programming code.
- Asymptotic notation and complexity analysis.
- Analyzing program code for correctness, efficiency, and errors.
- Automata theory and formal languages. Turing machine.
- Classes P and NP.
The knowledge and skills to implement and solve algorithmic problems using the mentioned algorithms are developed using Python.
The Numerical Analysis Algorithms course is taught in the second year and is covered in the third semester. Successful completion awards students with five credits, according to ECTS. The primary objective of the course is to develop basic understanding of numerical algorithms, as well as the skills to implement algorithms to solve computer-based mathematical problems. The course covers the following topics:
- Basic numerics, floating-point representation, convergence.
- Horner’s scheme.
- The theory of interpolation: Lagrange polynomial, Hermite interpolation, Neville’s iterative formula.
- Least square approximation.
- Numerical integration: Newton-Cotes formulas, Gaussian quadrature.
- Direct methods for solving systems of linear equations: Gaussian elimination, LU factorization, Cholesky decomposition.
- Householder method.
- Solving nonlinear equations and systems of nonlinear equations: Bisection method, fixed-point iteration, Newton’s method.
- Runge-Kutta methods for ordinary differential equations.
- Characteristic polynomial and eigenvalues.
The knowledge and skills to implement and solve algorithmic problems using the mentioned algorithms were developed using C++ due to its object-oriented programming with high performance, efficient memory management, low-level access to hardware and a rich standard library, including mathematical functions commonly used in numerical algorithms. These allow students to write efficient and customizable numerical algorithms. Objective C++ was one of the courses of the first year of studies.
3.3. The study participants
Study participants were selected from Computer Science students who were enrolled in the two mentioned compulsory courses: Introduction to Computer Science (ICS) (first semester) and Numerical Analysis Algorithms (NAA) (third semester). The first group of students began their studies in the academic year 2019/2020 in a traditional in-person course delivery format that was interrupted because of the confinement. They then continued their studies utilizing the synchronous online format. The second group consisted of students who began their studies in academic year 2020/2021 in the synchronous online format and continued these activities in a hybrid format. The third group of students began their studies in academic year 2021/2022 in a hybrid format that returned to an in-person format in the year 2022/2023. Online learning was supported by Moodle and MS Teams.
Only students enrolled in either the ICS and NAA courses participated in our research. Students who interrupted their studies and did not complete the courses were excluded. Thus, the study group included students who were enrolled in both courses and took both final exams. A total of 787 participants were selected. Table 1 summarizes the study participant groups according to education strategy.
- PPT PowerPoint slide
- PNG larger image
- TIFF original image
https://doi.org/10.1371/journal.pone.0305763.t001
Males constituted 87.5% of the total study participants, while females constituted 12.5%. Regarding nationality, the majority, i.e. 85.5%, came from Poland, while 14.5% came from other countries, mainly Ukraine.
3.4. Online exam quizzes
In this study, the Moodle platform provided by the Computer Science Department from the Lublin University of Technology was applied to conduct the final exam process. Comparative analysis of student academic performance was anchored on the results obtained in their final exams. Final exams were carried through the Moodle platform using Quiz activity . All exams comprised questions of various types, including Multiple Choice , Short Answer , Numerical and Essay as follows:
- Multiple choice questions were employed for evaluating both theoretical and practical contents. For our purpose, the option Multiple answers are allowed was used. Multiple answers questions enable one or more answers to be chosen by providing check boxes next to the answers. We used a negative grade percentage for wrong answers, so that simply ticking all choices did not necessarily generate a full grade. If the sum of partial grades was negative, then the total grade for this question would be zero [ 55 ].
- Short answer or numerical questions were used to evaluate theoretical and practical contents. In a short answer question, the student types in a word or phrase in response to a question. This must exactly match one of the acceptable answers. Numerical questions resembled short-answer questions. Here, the difference was that numerical answers were allowed to have an accepted error for number.
- Essay questions were used to evaluate practical contents, mainly programming and coding skills. We employed essay-type questions to provide the option of answering by entering text online. The option Require the student to enter text was chosen. The Response format option was set to Plain text , monospaced font to improve the readability of code by ensuring consistent and clear alignment. This is particularly helpful for maintaining an organized layout. The essay questions had to be marked manually by the course instructor.
The number of multiple choice questions and short answer / numerical questions was comparable. One question was an essay question. Questions were created and stored separately in a Question bank and were organized into 10 categories according to the implemented curricula and learning outcomes. Each category consisted of at least 50 questions. Quiz settings were as follows:
- Quizzes included 20 questions worth 20 points. There were two categories of questions: theoretical and practical.
- Students were allowed to have one attempt at each quiz. The time limit option was set to 60 minutes.
- Students were not allowed to open other windows or programs while taking these quizzes.
- A password was required. The option Block concurrent connections was checked.
- The Choose Sequential navigation method was employed to compel the student to progress through the questions in order and not return to a previous question or skip to a later one.
- The timeframe when the students were able to see feedback was set to the option After the quiz is closed and the option Whether correct was checked.
- Employed questions were assessed for quality and modified for re-use in the next academic year.
Students were tested using the same evaluation methods and types of questions in in-person, synchronous online and hybrid groups. The Moodle platform collected assessment data and generated report statistics. The data containing students’ exam results (points) were collected and exported from the Moodle platform as.xlsx files.
3.5. Quiz report statistics
Quiz statistics provided test statistics and quiz structure analysis. The test statistics gave information on how students performed on a quiz, and employed descriptive statistics: average grade, median grade, standard deviation of grades, skewness and kurtosis. A detailed analysis of each question was given in quiz structure analysis, and applied the following measures: facility index, discrimination index and discriminative efficiency. Discriminative efficiency is a measure similar to discrimination index [ 55 ].
Facility index.
In this work, facility index of a question was determined by the average score divided by the maximum score and represented as a percentage. A higher value indicated an easier question. The interpretation of its values is given in Table 2 [ 55 ].
https://doi.org/10.1371/journal.pone.0305763.t002
Discrimination index.
Discrimination index is the correlation between the score for this question and the score for the whole quiz represented as a percentage. If the score for the question and the score for the test are well correlated, the question can be categorized as a question with good discrimination. The maximum discrimination requires a facility index in the range 30%–70%, although this is not tantamount to high discrimination index. Discrimination index values should be interpreted according to Table 3 [ 55 ].
https://doi.org/10.1371/journal.pone.0305763.t003
A negative value of a discrimination index would mean that the best students got this question wrong more often than the worst students. A discrimination index of zero would mean it was a poor discriminator between good and bad students. Discrimination index is considered excellent when the value is higher than 40%, and considered good when it ranges from 20% to 40%.
Discriminative efficiency.
The discriminative efficiency estimates how good the discrimination index is relative to the difficulty of the question. This attempts to discriminate between students of different ability, and the higher the value, the better is the question at discriminating between students of different abilities [ 55 ]. Values between 30%–50% provide adequate discrimination, while those above 50% provide very good discrimination.
3.6. Statistical analysis
Data collected was tabulated, and analysis was carried out by applying simple percentage analysis, as well as descriptive analysis, using mean, standard deviation and inferential analysis such as t-Student tests and ANOVA [ 56 , 57 ]. We performed non-parametric alternatives such as a Mann-Whitney U test and the Kruskal-Wallis test to compare samples that cannot be assumed to be normally distributed [ 58 , 59 ]. Statistical significance was set at p<0.05. Data analysis was performed using the Statistica Package, Version 13 (TIBCO Software Inc.).
Participants’ profile
Our study included 787 Computer Science students, aged 18 to 22 years. The participant background characteristics revealed that most students were male (87.5%) and native (Polish; 85.5%). Furthermore, most of the students were enrolled in full-time studies (85.5%) ( Table 4 ).
https://doi.org/10.1371/journal.pone.0305763.t004
The percentages of the students who began their studies in the academic years 2019/2020, 2020/2021 and 2021/2022 were comparable, around 30%. An important aspect of the analysis was the availability of data from the pre-pandemic period that was relevant for our investigations.
Comparison of in-person, synchronous online and hybrid learning
The comparison of in-person, synchronous online, and hybrid teaching methods in student learning outcomes based on background characteristics is presented in Tables 5 and 6 .
https://doi.org/10.1371/journal.pone.0305763.t005
https://doi.org/10.1371/journal.pone.0305763.t006
The findings indicated that for the first semester course Introduction to Computer Science, the relation between learning outcomes and student gender was insignificant (p = 0.427). Moreover, the relation between learning outcomes and study option was also insignificant (p = 0.223). However, there was statistically significant difference between learning outcomes and residency status (p < 0.001). The findings indicated that during in-person and online studies, native students had significantly higher learning outcomes than did non-native students (p < 0.001). In addition, full-time students of online studies had significantly higher learning outcomes (p = 0.002) than did part-time students.
Regarding the learning outcomes of the students as obtained in the third semester course Numerical Analysis Algorithms, gender and study options were also insignificant (p = 0.834; p = 0.157) in relation to learning outcomes. In contrast, residency status was significant (p < 0.001). The findings indicate that native students had significantly higher learning outcomes than did non-native students (p < 0.001). Moreover, full-time students of online studies had significantly higher learning outcomes as compared to part-time students (p = 0.011).
The comparison of teaching methods in participant performance based on different semesters (courses) is presented in Table 7 .
https://doi.org/10.1371/journal.pone.0305763.t007
The differences in mean scores related to the first semester course Introduction to Computer Science, during online and hybrid studies, were significantly higher compared to in-person studies (LSD post-hoc, p < 0.001). However, mean scores related to the third semester course Numerical Analysis Algorithms, during online and hybrid studies, were significantly lower in comparison to in-person studies (LSD post-hoc, p < 0.001). Switching to traditional in-person studies in the academic year 2022/2023 did not degrade student performance.
Quiz quality assessment
Tables 8 and 9 reveal the facility index, discrimination index and discriminative efficiency values from the final exams held from 2019/2020 to 2022/2023.
https://doi.org/10.1371/journal.pone.0305763.t008
https://doi.org/10.1371/journal.pone.0305763.t009
The lowest mean facility index was 47% ± 25%, while the highest mean facility index was 59% ± 20%. Moreover, the mean discrimination index was located within the range between 31% and 37% and the mean discriminative efficiency was found within the range between 43% and 54%. The results indicate, with regard to facility index, that most of the questions were moderately difficult, yet about right for the average student, and demonstrated adequate discrimination—regardless of the course delivery format.
5. Discussion and conclusions
In our study, we compared the learning outcomes of Computer Science students who were taught through synchronous online and hybrid systems, to those who learned in the traditional in-person system, and this revealed significantly higher learning outcomes when taught through online and hybrid systems versus in-person. It is worth noting that student scores showed an increasing trend in the years 2019–2023. Despite this, the significant difference in the results of the students’ final examination was not too large–as it did not exceed 10% of the maximal score.
A comparison between the student groups demonstrates that utilizing synchronous online learning can result in more enhanced educational opportunities for students. However, our findings indicated that native students had significantly higher learning outcomes than did non-native students. The reason could be that the study courses were held in Polish, which is a difficult language for non-native students to learn and utilize.
Several research studies have shown that online learning and the combination of online and in-person learning systems have positive and powerful roles in enhancing the effectiveness of education [ 19 , 29 , 41 , 47 , 60 ]. However, along with enhanced accessibility and flexibility, pure online learning also has several disadvantages, notably, the lack of interpersonal contacts and student satisfaction. In the hybrid form, however, flexibility and accessibility are enhanced, while human connection occurs.
Our results indicated that synchronous online learning could be appreciated as a successful method of conducting Computer Science education and can be used as a tool supporting traditional in-person methods. Although this approach is a little less flexible for teachers and students, and requires reliable technology, in comparison to asynchronous learning, this allows for more real time engagement and feedback [ 61 ].
As the effective measurement of knowledge acquired is an important component of Computer Science education, the use of the Moodle quizzes activity as a continuous assessment of students was analyzed according to statistical data such as the facility index, discrimination index and discriminative efficiency. Out of the exam tests conducted from the academic year 2019/2020 to 2022/2023, the mean facility index scores ranged from 47% to 59% and the mean discrimination index ranged from 31% to 37%. The statistic results indicated that, regarding facility index, most of the questions were moderately difficult and about right for the average student regardless of the course delivery format, and that a consistent and adequate level of discrimination indices was maintained. In addition, the similar results obtained in our study no matter the year, with three different groups of students, also confirmed the validity and reliability of the designed exam tests.
Although online learning requires extensive self-discipline, it allows universities to integrate new technologies into their offer, and hence, effectively facilitate the student learning process. After the COVID-19 pandemic, there has been a quick transition back to in-person teaching, but still there are many proffered activities being in an online format. At present, many students state that they prefer to learn through hybrid learning methods. Furthermore, several studies have shown that e-learning methods are used widely by students outside of their formal curricula for continuing their professional education [ 62 ]. This indicates that students and professionals appreciate and take advantage of self-paced learning environments in which they control their learning pace, information flow, selection of learning activities, as well as their time management. Thus, the digital transformation of the educational process has become a necessity to meet shifting student demands and seems to be one of the leading factors that affect current teaching methodology.
It is worth noting that the extreme situation caused by the COVID-19 pandemic provided an opportunity to revise our approach, both to traditional and online learning, but also posed challenges for the future of education systems. In conclusion, the results of the analysis allow us to answer the questions formulated before in the following way.
- The COVID-19 confinement caused online education, which previously was mainly used as an addition to traditional learning methods, to become the mainstream, in particular, in Computer Science.
- The COVID-19 pandemic did not have a disruptive effect that resulted in knowledge deficiency with regard to the academic performance of Computer Science students. In contrast, this situation increased student academic motivation. Indeed, students demonstrated higher exam scores during subsequent two academic years.
- Despite the change from in-person to online learning, the reliability of student assessment remained at similar levels.
6. Limitations and future works
Our context is algorithms and programming in the first two years of the engineering studies program. While we believe that the long period under study is an advantage of this work, its limitation is the fact that it focuses only on the students of Computer Science. We based our research on the data comprising the performance of students in only two courses. Moreover, only the exam scores from the 1 st and 3 rd semesters were included in the study. The courses of other semesters were not assessed because they did not meet the required assumptions regarding the course selection. Another limitation of our study was that students could share information about the content of the exam. However, we randomly assigned students to subcategory sets to avoid sharing information. In the future it is worth considering extending the analysis to students of other fields, as well as take into account student performance in more courses.
Acknowledgments
The authors thank Mr Jack Dunster for linguistic improvement of the text.
- View Article
- PubMed/NCBI
- Google Scholar
- 4. Salguero A, Alvarado C, Griswold WG, Porter L. Understanding Sources of Student Struggle in Early Computer Science Courses. In: Proceedings of the 17th ACM Conference on International Computing Education Research. 2021;319–333.
- 8. Poland, Ordinance of the Council of Ministers on establishing certain limitations, orders and prohibitions with respect to the occurrence of the state of epidemic, 31 March 2020, § 14–15.
- 9. Poland, Ordinance of the Council of Ministers on establishing certain limitations, orders and prohibitions with respect to the occurrence of the state of epidemic, 7 August 2020, version as of 29 August 2020, § 25.
- 12. European Union Agency for Fundamental Rights, Coronavirus pandemic in the EU–Fundamental rights implications. Bulletin #2, 21 March-30 April 2020, Publications Office, 2020. https://data.europa.eu/doi/10.2811/441998 .
- 13. Charytanowicz M. E-learning technologies in lifelong learning: opportunities and challenges. In ICERI’2019 Proceedings: 12th International Conference of Education, Research and Innovation. 2019;43,9414–9421.
- 14. Charytanowicz M, Miłosz E, Suszyński W, Stęgierski R, Łukasik E. Internet of things as a challenge for higher education. In INTED 2021: 15th International Technology, Education and Development Conference. 2021;5120–5129.
- 16. Miłosz M, Charytanowicz M, Skublewska-Paszkowska M. Analysis of trends in ICT and its implication in higher education curricula. In INTED 2020: 14th International Technology, Education and Development Conference, 2020;500–509.
- 18. Suszyński W, Stęgierski R, Charytanowicz M. Remote access to networking laboratory, secure and scalable solutions. In The 2020 International Conference on Computational Intelligence, Information Technology and Systems Research. 2020;22–23.
- 19. Makarova E. Effectiveness of traditional and online learning: comparative analysis from the student perspective. In: SHS Web of Conferences 2021;99, 01019.
- 31. Amarneh BM, Alshurideh MT, Al Kurdi BH, Obeidat Z. The Impact of COVID-19 on E-learning: Advantages and Challenges. In The international conference on artificial intelligence and computer vision, 2021;75–89. Cham: Springer International Publishing.
- 38. Singh AK, Yusoff MA, Oo N. A comparative study between traditional learning and e-learning. In Proceedings of Teaching and Learning Open Forum 2009. 2009:1–7. CSM, Sarawak.
- 55. docs.moodle.org [Internet]. Moodle Documentation; c2023 [cited 2023 Sep 26]. https://docs.moodle.org/ .
- 56. Boddy R, Smith G. Statistical methods in practice: For scientists and Technologists. Chichester, U.K: Wiley 2009.
- 57. Doncaster CP, Davey AJH. Analysis of variance and covariance: How to choose and construct models for the Life Sciences. Cambridge: Cambridge University Press 2007.
- 58. Freund RJ, Wilson WJ, Mohr DL. Statistical methods. London, United Kingdom: Academic Press, Amsterdam, Elsevier 2022.
- 59. Sprent P, Smeeton NC. Applied Nonparametric Statistical Methods. Boca Raton: Chapman et Hall/CRC 2007.
- 62. Charytanowicz M. E-learning technologies in lifelong learning: opportunities and challenges. ICERI2019 Proceedings. 2019:9414–21.
The Impact of COVID-19 on Student Experiences and Expectations: Evidence from a Survey
In order to understand the impact of the COVID-19 pandemic on higher education, we surveyed approximately 1,500 students at one of the largest public institutions in the United States using an instrument designed to recover the causal impact of the pandemic on students' current and expected outcomes. Results show large negative effects across many dimensions. Due to COVID-19: 13% of students have delayed graduation, 40% lost a job, internship, or a job offer, and 29% expect to earn less at age 35. Moreover, these effects have been highly heterogeneous. One quarter of students increased their study time by more than 4 hours per week due to COVID-19, while another quarter decreased their study time by more than 5 hours per week. This heterogeneity often followed existing socioeconomic divides; lower-income students are 55% more likely to have delayed graduation due to COVID-19 than their higher-income peers. Finally, we show that the economic and health related shocks induced by COVID-19 vary systematically by socioeconomic factors and constitute key mediators in explaining the large (and heterogeneous) effects of the pandemic.
Noah Deitrick and Adam Streff provided excellent research assistance. All errors that remain are ours. The views expressed herein are those of the authors and do not necessarily reflect the views of the National Bureau of Economic Research.
MARC RIS BibTeΧ
Download Citation Data
Published Versions
Mentioned in the news, more from nber.
In addition to working papers , the NBER disseminates affiliates’ latest findings through a range of free periodicals — the NBER Reporter , the NBER Digest , the Bulletin on Retirement and Disability , the Bulletin on Health , and the Bulletin on Entrepreneurship — as well as online conference reports , video lectures , and interviews .
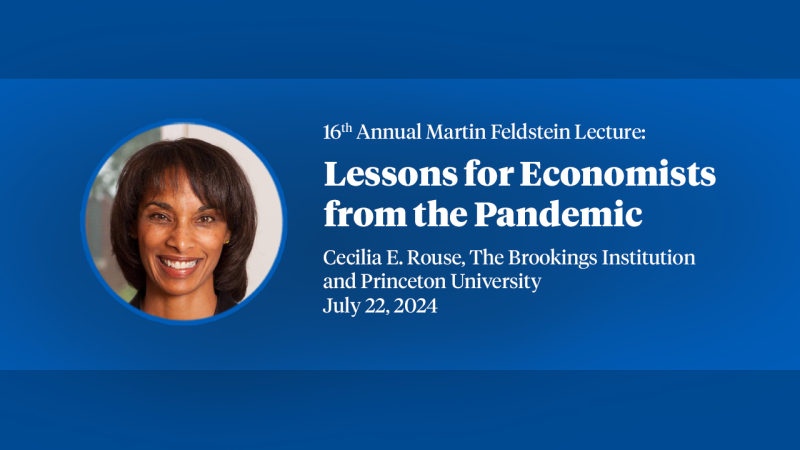
An examination of COVID-19’s impact on student learning: a self-determination theory perspective
- September 2024
- This person is not on ResearchGate, or hasn't claimed this research yet.

- University of Kentucky

Discover the world's research
- 25+ million members
- 160+ million publication pages
- 2.3+ billion citations
No full-text available

To read the full-text of this research, you can request a copy directly from the authors.

- Saeed Pahlevansharif

- Thomas Babalis
- Konstantina Tsoli
- PSYCHIAT RES

- Kaylene Godfrey

- BEHAV CHANGE

- Charlotte Branchu
- Erwin Flaureau

- Hsin-Te Yeh

- Ying-Chia Hsu

- Matthew LaGrone
- Shenoa Ragavaloo

- Dominic Conroy

- Angela Paladino

- Robert J. Summers

- BMC PSYCHIATRY

- Lars Johan Danbolt

- Martin V. Bonsangue
- Jennifer E. Clinkenbeard

- J AM COLL HEALTH

- Josep Petchamé

- William Talley
- Bridget Irioogbe

- EDUC PSYCHOL REV

- J MED INTERNET RES

- INT J SOC PSYCHIATR

- John P. Bean
- Russell K. Bradley
- David G. Taylor
- Michael Frechette
- Sheryl-Ann K. Stephen
- Marleen McCormick-Pritchard
- James W. Peltier

- Sherry Davis Molock

- Chad E. Kee
- Holly Hapke
- Tereza Dean

- J PUBLIC ECON

- Jacob French

- Basit Zafar

- Jianzhong Zheng

- LEARN INSTR

- James C. Lester

- Petra Brock

- Dean B. McFarlin
- Paul D. Sweeney
- Peter M. Bentler
- Decis Sci J Innovat Educ

- ANNU REV PSYCHOL

- Nathan P. Podsakoff

- Recruit researchers
- Join for free
- Login Email Tip: Most researchers use their institutional email address as their ResearchGate login Password Forgot password? Keep me logged in Log in or Continue with Google Welcome back! Please log in. Email · Hint Tip: Most researchers use their institutional email address as their ResearchGate login Password Forgot password? Keep me logged in Log in or Continue with Google No account? Sign up
The impact of COVID-19 on student achievement and what it may mean for educators
Subscribe to the brown center on education policy newsletter, jim soland , jim soland assistant professor, school of education and human development - university of virginia, affiliated research fellow - nwea megan kuhfeld , megan kuhfeld senior research scientist - nwea beth tarasawa , bt beth tarasawa executive vice president of research - nwea angela johnson , aj angela johnson research scientist - nwea erik ruzek , and er erik ruzek research assistant professor, curry school of education - university of virginia jing liu jing liu assistant professor of education policy - university of maryland-college park.
May 27, 2020
This Chalkboard post from May 2020 draws on historical data and past research to forecast the possible impact of COVID-19 school closures on student achievement. With actual data from the 2020-21 school year now available, please see this December 2020 Chalkboard post for an updated analysis of this trend.
Virtually all K-12 students in the United States are currently missing face-to-face instruction due to COVID-19. Many parents and educators thus share a common worry: When the pandemic subsides, kids will return to school with lower achievement. There are also concerns that the gap between high- and low-achieving students will become larger. Given the need to address these concerns, we decided to use prior test scores from millions of students and leverage research on summer learning patterns to make informed projections of what learning loss due to the pandemic might look like. Ultimately, we wanted to know: What sort of learning losses could we expect from the shortened 2019-20 school year?
Answering this question is complicated by the unique circumstances of COVID-19. Current school closures have added to the time that most students already spend at home during the summer months without explicit face-to-face instruction from teachers. Meanwhile, teachers are scrambling to adapt content for an online platform and parents are juggling work responsibilities (if not joblessness) with caring for and educating their own children. Students themselves are faced with isolation, anxiety about a deadly virus, and uncertainty about the future. In so many ways, the current situation is unprecedented for most people alive today.
Yet there are parallels between the current situation and other reasons students miss school that can give us insight into how COVID-19 may affect achievement. This includes research on the effects of out-of-school time on learning due to absenteeism , weather-related school closures (e.g., Hurricane Katrina in New Orleans), and summer vacation . Existing evidence can provide a rough sense of how time out of school due to COVID-19 will affect achievement.
We relied heavily on past precedent when trying to understand how COVID-19 might impact achievement in the short and medium term. We used a national sample of over 5 million students in grades 3-8 who took MAP Growth assessments in 2017-2018. These assessments enable such estimates because MAP Growth is administered multiple times per year, which means test scores are available in fall, winter, and spring such that changes in achievement during the year can be understood and anticipated. We compared typical growth for students who completed a standard-length school year to projections under multiple scenarios. These scenarios were directly informed by out-of-school-time research.
The results are deeply concerning.
The two figures below show projected math and reading learning patterns from the beginning of the 2019-20 school year (before COVID-19 school closures) through the start of the 2020-21 school year. The solid lines represent average trajectories in a typical year with typical growth (estimated based on a prior year’s data) followed by normal patterns of learning loss over the summer (generally, student achievement/learning tends to decline during the summer, though this varies greatly by student). Next, we assume an extended summer loss would occur during the period since schools closed. We refer to this scenario as the “COVID Slide” (represented by the dotted lines). These projections give a sense of how much learning students could lose, though we hope they will be overestimations of loss, given the online instruction and home schooling occurring.

These preliminary COVID Slide estimates suggest students could begin fall 2020 with roughly 70% of the learning gains in reading from the prior year relative to a typical school year. In mathematics, students may show even smaller learning gains from the previous year, returning with less than 50% of the gains. In lower grades, students may be nearly a full year behind in math compared to what we would observe in normal conditions.
Though not shown in the figures, we produced similar estimates of learning loss based on research showing the effect of being absent on achievement. That is, we simply assumed students’ learning during COVID-19 school closures would be akin to what occurs when students miss school, a large assumption given the online learning and homeschooling now occurring. Results for absenteeism-based projections were often more dire.
We also examined how much more variable achievement might be in the fall—that is, how wide the range in achievement might be between very high and very low-performing students. This range has implications for whether teachers can provide similar content to all students in their classrooms, or if they might need to further differentiate instruction based on a broader range of needs.

The above figures show our estimate of that variability by subject for 4 th and 6 th grade. The shaded areas display the spread in potential outcomes between students who were in the 25 th percentile of summer learning loss (who showed steep declines) and those in the 75 th percentile (who showed flat lines or even small gains during the summer). In mathematics, we see a fair amount of variability in learning rates, though the majority of students show losses over the extended closure and summer period. However, in reading, there is an even wider spread of potential outcomes, with students who are in the 75 th percentile and above showing sizable learning gains during the summer. Further, the figure below shows that extended time out of school may lead to more variability in achievement when students return in the fall relative to a typical year. A wider range of learning needs like the ones suggested by the figure could create greater challenges for teachers.

The New York Times warns that today’s students could be the “COVID generation.” As we think through our road to recovery, we hope education leaders consider our projections among many data points when preparing to support students returning in the fall. Specifically, our results indicate that:
- Students may be substantially behind, especially in mathematics . Thus, teachers of different grade levels may wish to coordinate in order to determine where to start instruction. Educators will also need to find ways to assess students early, either formally or informally, to understand exactly where students are academically.
- Students are likely to enter school with more variability in their academic skills than under normal circumstances. Therefore, educators may need to consider ways to further differentiate instruction or provide opportunities for individualized learning.
- Students who lose the most during the summer tend to gain the most when back in school, but this may not hold for COVID-19 . Regardless, the ground that students have to make up during the 2020-21 academic year will probably be greater due to COVID-19. Therefore, educators may want to work with students to determine growth rates needed to catch up and set learning goals for the year that are ambitious but obtainable.
Finally, the effects of COVID-19 our study cannot examine may be the ones most worthy of addressing. Prior research on students displaced by Hurricane Katrina indicated that they had difficulty concentrating and often manifested symptoms of depression in the months following the hurricane. Understanding these impacts and how best to support students’ social and emotional needs after the huge disruption of COVID-19 will be essential. Many students may face greater food insecurity, loss of family income, loss of family members to the coronavirus, and fear of catching the virus themselves.
While the scale of the COVID-19 school closures is novel, the inequalities in our school systems are unfortunately anything but new. Our models cannot account for the reality that the crisis is having an unequal impact on our most underserved communities. Nonetheless, we hope these analyses, which synthesize what we know from existing bodies of research, will inform tomorrow’s decision-making.
Related Content
Online only
2:00 pm - 3:00 pm EDT
A. Brooks Bowden, Rebecca Davis
May 26, 2020
Douglas N. Harris
April 24, 2020
Education Policy K-12 Education
Governance Studies
Brown Center on Education Policy
Magdalena Rodríguez Romero
September 10, 2024
Tom Swiderski, Sarah Crittenden Fuller, Kevin C. Bastian
September 9, 2024
Julien Lafortune, Barbara Biasi, David Schönholzer
September 6, 2024
COVID Hurt Student Learning: Key Findings From a Year of Research

- Share article
Dozens of studies have come out over the past months concluding that the pandemic had a negative—and uneven—effect on student learning.
National analyses have shown that students who were already struggling fell further behind than their peers, and that Black and Latino students experienced greater declines in test scores than their peers.
But taken together, what implications do they have for school and district leaders looking for a path forward?
Here are four questions and answers, based on what we’ve learned from the most salient studies, that dig into the evidence.
Did students who stayed in remote learning longer fare worse than those who learned in person?
Generally, yes—but not in every single instance.
School buildings shut down in spring 2020 . By fall 2021, most students were back learning in person. But schools took a variety of different approaches in the middle, during the 2020-21 school year.
Several studies have attempted to examine the effects of the choices that districts made during that time period. And they found that students who were mostly in-person fared better than students who were mostly remote.
An analysis of 2021 spring state test data across 12 states found that districts that offered more access to in-person options saw smaller declines in math and reading scores than districts that offered less access. In reading, the effect was much larger in districts with a higher share of Black and Hispanic students.
Assessment experts, as well as the researchers, have urged caution about these results, noting that it’s hard to draw conclusions from results on spring 2021 state tests, given low rates of participation and other factors that affected how the tests were administered.
But it wasn’t just state test scores that were affected. Interim test scores—the more-frequent assessments that schools give throughout the year—saw declines too.
Another study examined scores on the Measures of Academic Progress assessment, or MAP , an interim test developed by NWEA, a nonprofit assessment provider. Researchers at NWEA, the American Institutes for Research, and Harvard examined data from 2.1 million students during the 2020-21 school year.
Students in districts that were remote during this period had lower achievement growth than students in districts that offered in-person learning. The effects were most substantial for high-poverty schools in remote learning districts.
Still, other research introduces some caveats.
The Education Recovery Scorecard, a collaboration between researchers at Stanford and Harvard, analyzed states’ scores on the 2022 National Assessment of Educational Progress. They compared these scores to the average amount of time that a district in the state spent in remote learning.
For the most part, this analysis confirmed the findings of previous research: In states where districts were remote longer, student achievement was worse. But there were also some outliers, like California. There, students saw smaller declines in math than average, even though the state had the highest closure rates on average. The researchers also noted that even among districts that spent the same amount of time in 2020-21 in remote learning, there were differences in achievement declines.
Are there other factors that could have contributed to these declines?
It’s probable. Remote learning didn’t take place in a vacuum, as educators and experts have repeatedly pointed out. But there’s not a lot of empirical evidence on this question just yet.
Children switched to virtual instruction as the pandemic unfolded around them—parents lost jobs, family members fell sick and died. In many cases, the school districts that chose remote learning served communities that also suffered some of the highest mortality rates from COVID.
The NWEA, AIR, and Harvard researchers—the group that looked at interim test data—note this. “It is possible that the relationships we have observed are not entirely causal, that family stress in the districts that remained remote both caused the decline in achievement and drove school officials to keep school buildings closed,” they wrote.
The Education Recovery Scorecard team plans to investigate the effects of other factors in future research, “such as COVID death rates, broadband connectivity, the predominant industries of employment and occupations for parents in the school district.”
Most of this data is from the 2020-21 school year. What’s happening now? Are students making progress?
They are—but it’s unevenly distributed.
NWEA, the interim assessment provider, recently analyzed test data from spring 2022 . They found that student academic progress during the 2021-22 school did start to rebound.
But even though students at both ends of the distribution are making academic progress, lower-scoring students are making gains at a slower rate than higher-scoring students.
“It’s kind of a double whammy. Lower-achieving students were harder hit in that initial phase of the pandemic, and they’re not achieving as steadily,” Karyn Lewis, the lead author of the brief, said earlier in November .
What should schools do in response? How can they know where to focus their efforts?
That depends on what your own data show—though it’s a good bet that focusing on math, especially for kids who were already struggling, is a good place to start.
Test results across the board, from the NAEP to interim assessment data, show that declines have been larger in math than in reading . And kids who were already struggling fell further behind than their peers, widening gaps with higher-achieving students.
But these sweeping analyses don’t tell individual teachers, or even districts, what their specific students need. That may look different from school to school.
“One of the things we found is that even within a district, there is variability,” Sean Reardon, a professor of poverty and inequality in education at Stanford University and a researcher on the Education Recovery Scorecard, said in a statement.
“School districts are the first line of action to help children catch up. The better they know about the patterns of learning loss, the more they’re going to be able to target their resources effectively to reduce educational inequality of opportunity and help children and communities thrive,” he said.
Experts have emphasized two main suggestions in interviews with Education Week.
- Figure out where students are. Teachers and school leaders can examine interim test data from classrooms or, for a more real-time analysis, samples of student work. These classroom-level data are more useful for targeting instruction than top-line state test results or NAEP scores, experts say.
- Districts should make sure that the students who have been disproportionately affected by pandemic disruptions are prioritized for support.
“The implication for district leaders isn’t just, ‘am I offering the right kinds of opportunities [for academic recovery]?’” Lewis said earlier this month. “But also, ‘am I offering them to the students who have been harmed most?’”
A version of this article appeared in the December 14, 2022 edition of Education Week as COVID Hurt Student Learning: Four Key Findings from A Year of Research
Sign Up for The Savvy Principal
Edweek top school jobs.

Sign Up & Sign In

Thank you for visiting nature.com. You are using a browser version with limited support for CSS. To obtain the best experience, we recommend you use a more up to date browser (or turn off compatibility mode in Internet Explorer). In the meantime, to ensure continued support, we are displaying the site without styles and JavaScript.
- View all journals
- Explore content
- About the journal
- Publish with us
- Sign up for alerts
- Open access
- Published: 13 September 2024
Grit and academic resilience during the COVID-19 pandemic
- Daniel L. Chen 1 ,
- Seda Ertac 2 ,
- Theodoros Evgeniou ORCID: orcid.org/0000-0001-9525-6110 3 ,
- Xin Miao 4 ,
- Ali Nadaf 4 &
- Emrah Yilmaz 2 , 5
npj Science of Learning volume 9 , Article number: 57 ( 2024 ) Cite this article
Metrics details
Grit, a non-cognitive skill that indicates perseverance and passion for long-term goals, has been shown to predict academic achievement. This paper provides evidence that grit also predicts student outcomes during the challenging period of the Covid-19 pandemic. We use a unique dataset from a digital learning platform in the United Arab Emirates to construct a behavioral measure of grit. We find that controlling for baseline achievement, students who were grittier according to this measure before the pandemic, register lower declines in math and science scores during the coronavirus period. Using machine learning, behavioral data obtained from the platform prior to the pandemic can explain 77% of the variance in academic resilience. A survey measure of grit coming from the same students, on the other hand, does not have significant predictive power over performance changes. Our findings have implications for interventions on non-cognitive skills, as well as how data from digital learning platforms can be used to predict student behavior and outcomes, which we expect will be increasingly relevant as AI-based learning technologies become more common.
Introduction
Grit is an important non-cognitive skill that has been shown to predict achievement outcomes including educational attainment, academic performance, and retention 1 , 2 . In the school context, grit has a significant role on outcomes over and above cognitive skills, and can have large multiplier effects, given that students who give up early have fewer chances to recover. Recognizing this, in many countries, educational policymakers and NGOs implement programs to foster grit in the school environment, especially with “growth mindset” interventions that teach students the malleability of skills through effort and perseverance 3 , 4 . In addition to its association with performance at school, the non-cognitive skill of grit can also be important in predicting resilience in the distance learning context during the Covid-19 pandemic. The challenges brought about by reduced access to teachers and peers in remote learning likely impaired the support system for students, changed the interaction of teachers, students and learning content 5 , and made self-regulated learning more central 6 . Motivating oneself when tackling potentially difficult learning tasks can be more challenging with remote learning, in the absence of direct interactions with teachers and peers. Indeed, recent evidence shows that even in “best-case scenario” countries with favorable conditions, students have experienced learning losses with remote instruction during the pandemic 7 .
In this paper, we use unique data from an online educational platform in the United Arab Emirates to explore whether the non-cognitive skill of “grit” predicts performance changes in math and science subjects during the coronavirus period, among a sample of 5th-9th graders. The platform was used for blended learning in K-12 public school classrooms to deliver core curriculum before the pandemic. With the switch to remote education due to Covid-19, it became the sole remote learning environment in the country for public K-12 schools. In this context, we utilize two alternative pre-pandemic measures of grit, one coming from a survey and one coming from actual behavior in the form of students’ response to setbacks experienced within the digital learning platform, to predict performance changes during the pandemic.
How to measure non-cognitive skills accurately is a major issue that has interdisciplinary relevance. In much of the literature, grit has been measured by the Duckworth-Quinn grit scale 8 . Measured this way, it has been shown to correlate with a wide range of outcomes such as retention and school performance, although there are also studies that have found null effects 9 . However, survey measures tend to be prone to demand effects or social desirability effects 10 , which may be particularly concerning in an adolescent student sample. Experimental economists have recently sought to construct behavioral measures of grit, using external, dynamic tasks that require real effort 11 . While such measures have been shown to have strong predictive power over success, implementing and using them at a large scale may be difficult in the regular classroom environment, as they tend to require the use of material rewards and implementation by external researchers. In this respect, using naturally occurring data from a digital learning platform enables us to obtain a unique measure of “behavioral grit” that would be difficult to obtain in the traditional learning environment and possible to use more widely, with the growing integration of digital learning into education. Specifically, we are able to observe “after-hours” studying and practice by students, capturing sustained voluntary effort, which allows us to define a measure of “revealed” grit in terms of the response to performance setbacks experienced on the platform. Using this behavioral measure along with a well-known survey measure in the same sample, we are thus able to make the contribution of comparing the predictive power of survey measures of grit and the behavioral measure of grit from platform data, both coming from the pre-Covid period, on student performance and behavior during Covid-19.
Our data confirms that the pandemic has led to significant declines in performance in math and science subjects, in line with studies showing that learning has been disrupted during the Covid-19 period. We find that the survey measure of grit 8 has limited power in predicting performance in our sample, especially during the coronavirus period, and importantly, it does not predict the declines in performance. We hypothesize that “revealed grit”, measured using behavioral data coming from the digital platform, may be a better predictor of the performance response. Specifically, we hypothesize that students whose behavior before the pandemic period was grittier, register lower declines in academic performance during Covid-19, controlling for baseline diagnostic scores. We indeed find that revealed grit, unlike or stronger than survey-based measures: 1) predicts both /pre-Covid and after-Covid performance on the digital platform in math, 2) predicts the change in performance, with grittier students registering lower declines in performance in math and science. Finally, we show that pre-Covid behavioral data in the digital platform contains significant information to explain academic resilience: up to 77% of the variance in declines in performance in math and science during the Covid-19 period can be explained using machine learning.
The rest of the paper is organized as follows. In the next section (Results), we present the findings. The following section puts forward a discussion, and in a Methods section, we put forward details about the data and empirical methodology, as well as the construction of the behavioral grit measure.
Performance changes and Covid-19
As expected, the Covid-19 period has led to an overall decline in performance, in both math and science subjects. Table 1 shows that in math, there is an about 11 points decline in the average score on the platform, and a similar decline of 10 points in science, for both genders. These declines are significant at the 1% level ( p < 0.0001 for math and science in t tests).
Grit measures and school performance: pre- and post-Covid
The “behavioral grit” measure we construct is based on the student’s response to a performance that deviates in a negative way from her earlier average performance (please see the Methods section for details). We interpret this fall as a “setback”, and the student’s positive response to this setback (higher effort exerted out of school hours following the setback) as “grit”. Table 2 shows that there is a strong correlation between behavioral grit and student performance measured in overall platform scores in math, controlling for baseline diagnostic test scores which can be considered as an objective baseline performance measure. One standard deviation of behavioral grit is associated with 4.6 points higher math scores prior to covid relative to an average of roughly 50, which is equivalent to 50% relative to the mean, and the association is significant ( p < 0.05). For science, grit is not strongly associated with test scores prior to Covid. Note that grit’s stronger association with math is expected, given that students tend to find math subjects particularly difficult, and consistently with this, grit has been shown to have the most relevance in math performance 11 . Girls in this sample perform 42 points higher on math tests, and 37 percent higher on science tests ( p < 0.05). The diagnostic score for each subject, as expected, is also positively associated with platform performance ( p < 0.01 for both subjects). After Covid commenced, grit is associated with both math and science test scores ( p < 0.01) at a substantial 15 points in both subjects. Of note, the gender gap shrinks for the math field but remains the same for the science field.
In comparison, Table 3 shows that survey grit is only associated with one of these four measures of student performance—0.8 points in science prior to Covid ( p < 0.10). Notably, the association with gender here is more statistically significant in comparison to Table 1 . Moreover, science performance may be picking up certain characteristics of students that are associated with self-reported grit, however this association is small and disappears as a significant factor in the post-Covid period.
To visualize this comparison, we present a figure that includes the coefficients for behavioral grit and survey grit side-by-side in Fig. 1 .
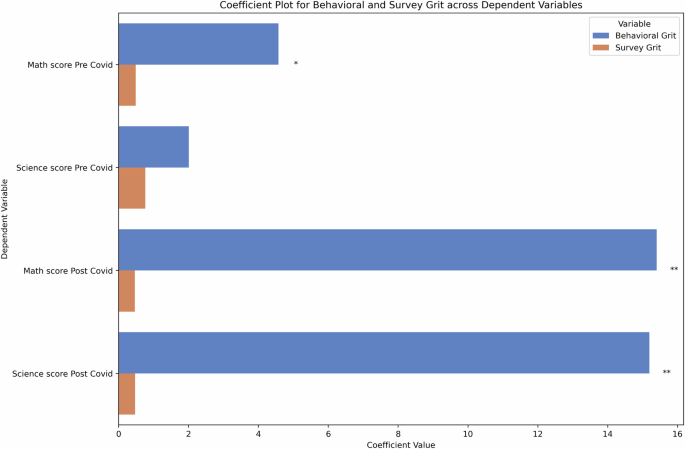
This figure presents coefficient plots comparing the effects of behavioral grit and survey grit on academic performance in math and science, both before and after the onset of the Covid-19 pandemic. Derived from regression analyses controlling for baseline diagnostic test scores and gender, these plots illustrate the magnitude and statistical significance of associations between grit measures and academic resilience. Behavioral grit is measured by students’ positive responses (increased effort) to performance setbacks, while survey grit is self-reported. The figure allows for direct comparison of how these different grit measures relate to academic performance across subjects and in response to the pandemic’s disruption.
Grit measures and change in performance post-Covid
Next, we explore non-parametrically the relationship between behavioral grit and academic resilience (change in overall test scores, as used above, during Covid-19). A significant predictive association for behavioral grit can be seen in binned scatterplots in Fig. 2 (math) and Fig. 3 (science), which condense the information from a scatterplot by partitioning the x‐axis into bins and calculating the mean of y within each bin.
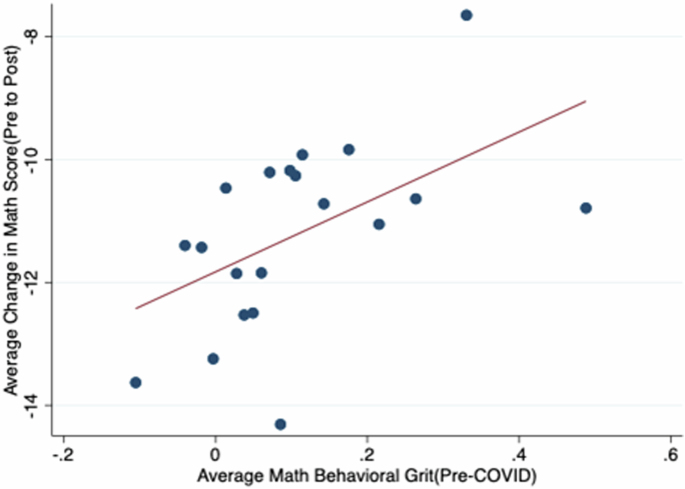
This figure presents binned scatterplots illustrating the correlation between pre-Covid behavioral grit and changes in math scores, demonstrating academic resilience in mathematics. This visualization technique divides the independent variable (pre-Covid behavioral grit) into equal-sized intervals or bins, calculates the average change in math scores within each bin, and plots these averages against the bin midpoints. This method reduces visual noise and helps identify overall trends that might be obscured in a standard scatterplot, potentially revealing nonlinear relationships. The plot’s pattern indicates the correlation between pre-pandemic grit and math score changes, with the slope showing the direction and strength of this relationship. The figure helps demonstrate whether students’ grit, measured by behavioral indicators before the Covid-19 pandemic, correlates with their resilience in math performance. The number of bins used balances granularity with noise reduction, affecting the plot’s detail and clarity. This visualization is generated using the statistical software Stata.
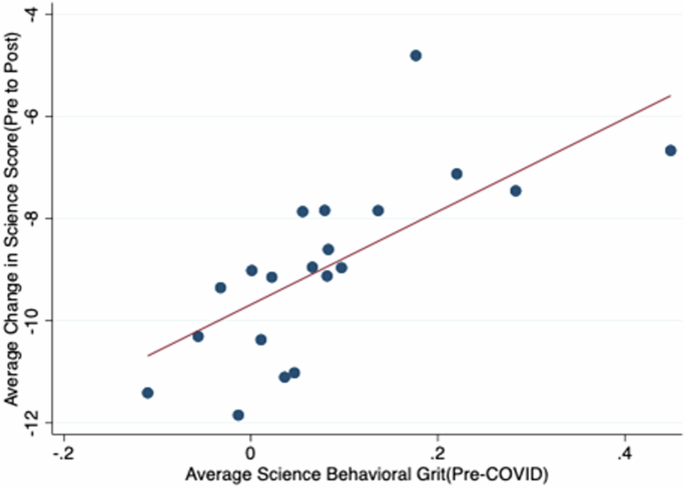
This figure presents binned scatterplots illustrating the correlation between pre-Covid behavioral grit and changes in science scores, demonstrating academic resilience in science. This visualization technique divides the independent variable (pre-Covid behavioral grit) into equal-sized intervals or bins, calculates the average change in science scores within each bin, and plots these averages against the bin midpoints. This method reduces visual noise and helps identify overall trends that might be obscured in a standard scatterplot, potentially revealing nonlinear relationships. The plot’s pattern indicates the correlation between pre-pandemic grit and science score changes, with the slope showing the direction and strength of this relationship. The figure helps demonstrate whether students’ grit, measured by behavioral indicators before the Covid-19 pandemic, correlates with their resilience in science performance. The number of bins used balances granularity with noise reduction, affecting the plot’s detail and clarity. This visualization is generated using the statistical software Stata.
Table 4 shows, in regression form, that survey grit is essentially not correlated or slightly negatively correlated with resilience during Covid-19, where resilience is measured as the performance change, defined as overall test scores (as above) after Covid minus overall test scores pre-Covid. In contrast, behavioral grit is associated with 0.1 points greater resilience for math and science, respectively. The lack of similar predictive associations for survey grit can also be seen in Figs. 4 and 5 , which present the non- parametric relationship between survey grit and academic resilience in math and science test scores, respectively. To further visualize this comparison, we present a figure that includes the coefficients for behavioral grit and survey grit side-by-side in Fig. 6 .
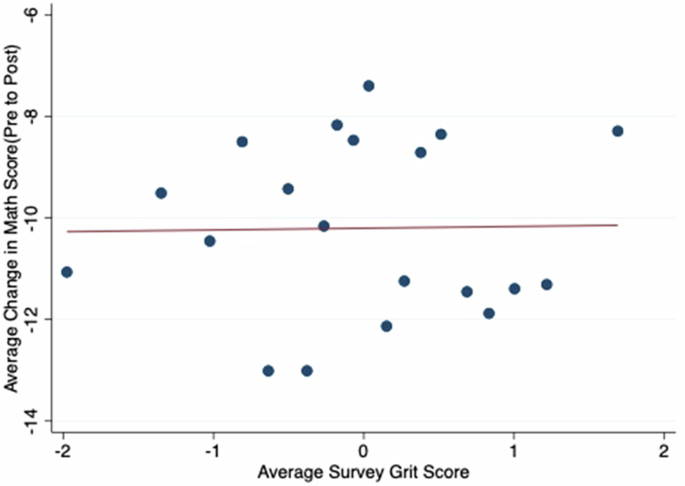
This figure presents binned scatterplots illustrating the correlation between behavioral grit and changes in math scores, our measure for academic resilience in mathematics. This visualization technique divides the independent variable (behavioral grit) into equal-sized intervals or bins, calculates the average change in math scores within each bin, and plots these averages against the bin midpoints. This method reduces visual noise and helps identify overall trends that might be obscured in a standard scatterplot, potentially revealing nonlinear relationships. The plot’s pattern indicates the correlation between grit and math score changes, with the slope showing the direction and strength of this relationship. The figure helps demonstrate whether students’ grit, measured by behavioral indicators, correlates with their resilience in math performance. The number of bins used balances granularity with noise reduction, affecting the plot’s detail and clarity. This visualization is generated using the statistical software Stata.
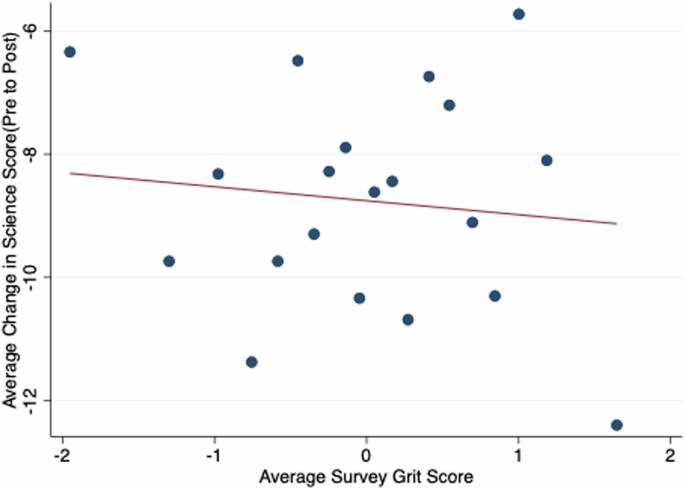
This figure presents binned scatterplots illustrating the correlation between survey grit and changes in science scores, our measure for academic resilience in science. This visualization technique divides the independent variable (survey grit) into equal-sized intervals or bins, calculates the average change in science scores within each bin, and plots these averages against the bin midpoints. This method reduces visual noise and helps identify overall trends that might be obscured in a standard scatterplot, potentially revealing nonlinear relationships. The plot’s pattern indicates the correlation between grit and science score changes, with the slope showing the direction and strength of this relationship. The figure helps demonstrate whether students’ grit, measured by survey responses, correlates with their resilience in science performance. The number of bins used balances granularity with noise reduction, affecting the plot’s detail and clarity. This visualization is generated using the statistical software Stata.
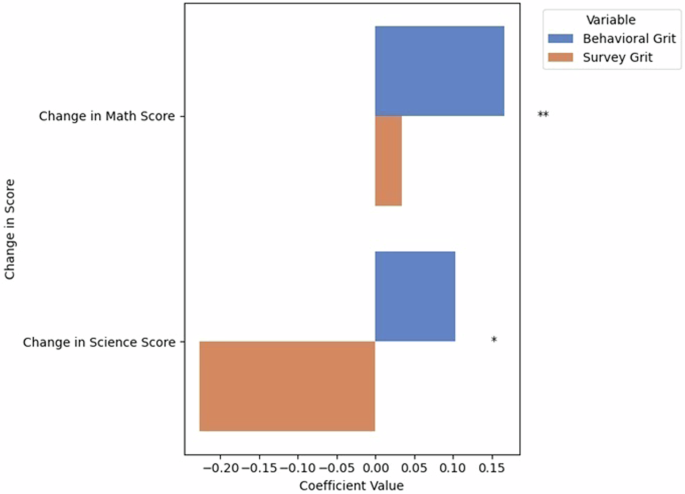
This figure presents coefficient plots comparing the effects of behavioral grit and survey grit on changes in academic performance in math and science after the onset of the Covid-19 pandemic. This visualization is derived from regression analyses measuring resilience as the change in overall test scores from the pre-Covid to the Covid periods. The four bars display the coefficients for behavioral grit and changes in math and science scores, and survey grit and changes in math and science scores. The magnitude of each bar indicates the strength of the association between the respective grit measure and academic resilience. The figure illustrates the contrasting associations between behavioral versus self-reported grit with students’ academic performance changes during the pandemic, and allows for a direct comparison of how the two different grit measures predict the performance response.
To examine total variance explained by behavioral grit measured using the digital platform, we use an elastic regression model in which we include all clicks recorded by the platform, pre-processed into 400 categories. The detailed set of observables we have for this test include students accessing different types of instructional content on the platform for each subject (e.g. watching a video, accessing course-related reading material, doing exercises, starting/ending tests to check understanding), number of times each content is accessed, and the time spent on the platform. To aid interpretability, we first use principal components analysis on these other variables. Note that before the elastic regression model, we orthogonalize each explanatory variable to the behavioral grit measure by projecting each successive variable on the behavioral grit variable and taking residuals. Then the elastic regression model is run by using the uncorrelated variables (i.e., residuals).
In Table 5 , we report the multivariate regression including only the vari ables selected by elastic regression. The results indicate that 77% of variance in resilience is explained by behavioral data measured in the platform. The data, while inadequate to definitively infer causality, thus show the potential for high-dimensional revealed preference measurement of non-cognitive skills.
The coronavirus pandemic has brought a unique set of challenges for education. The learning loss incurred due to the pandemic by students in grades 1 to 12 is estimated to translate to about 3 percent lower income over the entire lifetime 12 . In this paper, we show that an individual non-cognitive skill, grit, can predict how large of a performance loss the student would experience due to the pandemic.
Research on the effects of Covid-19 has shown that personality traits can predict behavioral responses such as hoarding or compliance with guidelines 13 , as well as with coping responses 14 . Johnston et al. 15 show that Covid-19 led to declines in mental health, and this decline is not predicted by financial resources but by the non-cognitive skill of self-efficacy 15 . We show that in the educational setting, the non-cognitive skill of grit predicts a less negative performance response to the challenges faced during the pandemic. In this sense, we provide new evidence for why fostering grit in student samples is important for achieving better learning outcomes. The finding is in line with the recent literature showing associations between grit/resilience and the response to the pandemic. For instance, studies have found better mental health and satisfaction outcomes 16 and a lower level of academic/career concerns 17 among students during the pandemic, with the former result also extending to samples other than students, e.g. health personnel 18 . Similarly, a link between growth mindset (a precursor for grit) and lower perceived stress and higher academic engagement/satisfaction outcomes among students in the Covid-19 period has been documented 19 . Finally, two studies with smaller samples show a positive link between a survey measure of grit and exam performance during e-learning 20 , 21 , indicating that the expected positive link between grit and achievement also extends to the pandemic period.
Our behavioral measure of grit is based on identifying performance declines, and utilizing the unique advantage offered by the data recorded in voluntary after-school hours activity on the platform to measure the revealed effort response. In this sense, our measure captures the “perseverance of effort” aspect of the construct of grit. Our results are therefore consistent with Crede et al. and Ponnock et al. 22 , 23 , who show that it is the perseverance facet of grit (in contrast to consistency of interest) that has explanatory power over academic performance.
Our findings suggest that programs that aim to build grit early on in the educational environment (e.g. through promoting growth mindset), can also have positive effects on resilience and the academic performance response in times of crisis 3 , 24 . To the extent that such crises also exacerbate educational inequalities 25 , teaching grit could reduce the widening gap between students coming from different social strata. The results also underscore the potential for digital learning platforms not only as facilitators of learning but also as a valuable tool for both assessing and fostering non-cognitive skills, which can indirectly lead to better learning outcomes. That is, such platforms, by design can be used, to not only deliver learning content but also to collect valuable behavioral information that can provide a foundation for customized learning for students with heterogeneous levels of non-cognitive skills.
The finding that behavioral data can explain a significant portion of the variance in academic resilience suggests that data collected from digital learning platforms can be valuable for measuring non-cognitive skills, as well as predicting students’ future performance or response to shocks. Using digital data on behavior and performance, AI-based remote learning technologies can therefore be used to identify not only students who are struggling in terms of comprehension but also those who lack resilience or motivation, and to actively respond by customizing the learning experience for different students by adapting levels/sequences of difficulty, presenting content that requires sustained effort, or providing personalized motivational feedback tailored to individual performance patterns. We expect that such strategies will be especially relevant with the growing use of AI-based online learning technologies, and may create significant benefit in improving student engagement and achievement. Moreover, features that promote gritty behavior (e.g. messages about growth mindset, which has been shown to be effective in several interventions) can be directly incorporated into digital learning environments to support self-regulated virtual learning. Overall, digital learning platforms can be used to collect valuable behavioral information that can equip educators with insights into their students’ engagement and perseverance and can support more targeted interventions to cultivate grit, both within and beyond the classroom setting. Finally, our results underscore the importance of constructing revealed preference measures of non-cognitive skills, and show how self-reported survey data can be complemented with high-dimensional revealed preference data for predicting educational outcomes.
Our study has several limitations: we should note that the survey measure of grit may have drawbacks in the sense that students may not have proper incentives to respond truthfully, and factors such as social desirability or boredom may affect answers and introduce bias or noise. From an external validity perspective, our data come from middle-schoolers in a set of schools in the United Arab Emirates, and we cannot exclude the possibility that the results are country-dependent or age-dependent. That said, we believe that external validity may be more of a concern in the results regarding the survey grit measure rather than behavioral grit. If the students in our sample, for example, are more likely to report socially desirable answers in self-reports than those in other countries or in other age groups, this may prevent a direct generalization on the relative costs from using survey vs. behavioral data in other contexts. Still, since the measure from the online educational platform reflects real actions, it is not prone to social desirability effects or reporting confounds, and the negative association between the behavioral measure of grit and performance declines are likely to be more generally relevant. Finally, we should note that the results may also depend on how the pandemic was experienced in the country and the level of integration of digital learning into the normal school curriculum. On these fronts, we believe that our estimates may be conservative, and results may have been even more pronounced in other settings. This is because the digital platform we study was already partly integrated into the school curriculum and may have facilitated a level of familiarity with remote learning. If this were not the case, the switch to online education may have even higher costs and adaptability issues, possibly leading to higher performance declines with the pandemic. Similarly, United Arab Emirates had a strict testing and vaccination policy, which kept case counts under control, which may have limited the traumatic experience of the pandemic in the country. It would be an interesting avenue for further research to study the relationship of behavioral measures of non-cognitive skills such as grit and educational engagement and outcomes as well as responses to different shocks using data from different settings, to explore the boundaries of the results we find. Future research should also consider the measurement of other non-cognitive skills and aspects of motivation from behavioral digital learning data, and explore the potential of using such data for both assessment/evaluation of individual non-cognitive skills and designing related targeted interventions. Along the latter dimension, it is possible to envision many different types of practical applications, ranging from informational interventions for students, parents and schools, to motivational interventions based on embedding adaptive content into learning platforms. We expect that such applications would also provide fruitful ground for collaborations between academia and private/public institutions that work in the domain of digital education, as the conclusions of research on these platforms using data provided by these stakeholders may provide valuable insight into the optimal design and efficient use of these platforms to maximize student learning.
Our data come from a widely used digital learning platform in the United Arab Emirates. The data are provided by Alef Education, a global K-12 education technology company that provides K12 students and teachers with core curriculum and learning analytics through blended learning settings. The platform also provides experiential learning that enables students to apply and transfer their newly acquired skills, and practice lessons for further skill mastery, with the aim of creating an effective instructional and classroom model. In the pre- pandemic period, the platform was used for blended learning in K-12 public school classrooms, delivering core curriculum. After the pandemic, with the switch to complete distance learning, the platform became the sole environment for teaching and learning in the country, in conjunction with communication tools such as Zoom. The educational technology organization that has developed the platform conducts diagnostic performance tests and surveys at schools in the United Arab Emirates as part of their periodic research and evaluation activities, with approvals from the Department of Education and Knowledge (ADEK) and relevant school principals prior to data collection. Students and teachers receive a thorough explanation of the purpose of any questionnaires or surveys. All data collected comply with government student privacy protocols and exclude personally identifying information. The dataset we use in the paper comes from a subset of students, for whom survey measures of grit and performance data in out-of-platform diagnostic tests before the pandemic period are available, in addition to data coming from the digital platform. All data used in this paper were provided to the authors as secondary, anonymized data.
Construction of the behavioral grit measure
There are several types of activities for students to engage in on the platform. For each subject (math and science), students can watch instructional videos, study digital course materials, and take online assessments to check their understanding of the material. The platform records every activity, from when the student started the activity to when it ended, how many assessments the student attempted, as well as all other login/logout attempts etc. Here, we define two important concepts that will be used in the current paper for analysis. We define a student’s “platform performance” as the average score of all assessments taken by the student within a specific period of time. These scores, and hence their averages, range from 0 to 100. We define performance metrics as above for each student and subject (math and science) in the pre/post-Covid periods separately. We also calculate the differences in score from pre- to post-Covid per subject, in order to see what might have impacted the change in performance.
The second variable we use is the number of attempts for a test made by each student. The intensity of engagement with the digital platform during school hours is usually determined by the teacher. For this reason, we focus on out-of-school hours, where it is completely up to the student to use the platform for learning or practice. Given that out-of-school hours activity is voluntary, students differ in how many times they attempt an assessment task. The number of times a student approaches an assessment task is defined as the “attempts”, which we use to define gritty behavior.
There are many ways to conceptualize how digital learning data can be used to measure grit. Our grit measure is based on the student’s response to a performance that deviates from her earlier average performance. We interpret this fall as a “setback”, and the student’s positive response to this setback (highereffort exerted out of school hours following the setback) as “grit”. Specifically, we define our main grit variable as follows. For each student and for every day in our sample (“Day t”), we calculate the difference between the student’s average performance in the past week (during the days t-7 to t-1) and performance on Day t. If today’s score is sizably lower than last week’s average score, that is, if there is an at least 5- point score decline from the previous week, we define this incidence as a “setback” where a behavioral grit response may be observed. We define the gritty response as an increase in effort, which we capture by the number of attempts the student made in their formative tests on the platform during out-of-school hours following the setback. Specifically, for each setback event, we calculate the difference between the number of out-of-school attempts on the following day (t + 1), and compare this with the previous week’s average attempt (t-7 to t-1). Note that we do not include weekends in the calculations, as the number of attempts is significantly lower. When Thursday is day t, then Sunday becomes t + 1.The behavioral grit variable takes the value of 1 if there is an increase in attempts, and 0 otherwise. Once we have the grit measure for a given day t, we take an average over all days in our sample, for both pre- and during-covid periods. Thus, we have, for each student, what percentage of the time out-of-school attempts increased after a sizable score decline. We interpret this as a measure of behavioral grit, which we calculate separately for math and science subjects. We then compare our behavioral grit measure with the well-known survey grit measure, based on Duckworth and Quinn’s “short grit scale” 8 , with questions reproduced in the Methods section.
We summarize the calculation of our behavioral grit measure below, and provide some numerical examples of the calculation in Fig. 7 :
Determine if there is a significant setback: The average performance of the subject from day t-7 to t-1 is compared to the performance on day t. If there is a significant drop in performance on day t, it is considered a “setback”.
Assess gritty behavior, defined as a positive response to the setback in terms of attempts made: If a setback is observed on day t, the number of attempts made by the subject in out-of-school hours after day t is examined. If the subject increases their attempts following the setback as compared to his/her average attempts in the period before the setback, this is counted as gritty behavior. Note that grit is not defined when there is no setback.
Behavioral grit is then calculated as the percentage of times when gritty behavior is shown in response to setbacks–for example, if there are setbacks on 6 days in the window taken and gritty behavior is shown twice, the behavioral grit score for that period is 2/6 = 0.33. Doing this calculation for each day for 8 weeks before Covid-19 and for 12 weeks during Covid-19, we calculate the average behavioral grit scores before and after the COVID-19 pandemic.
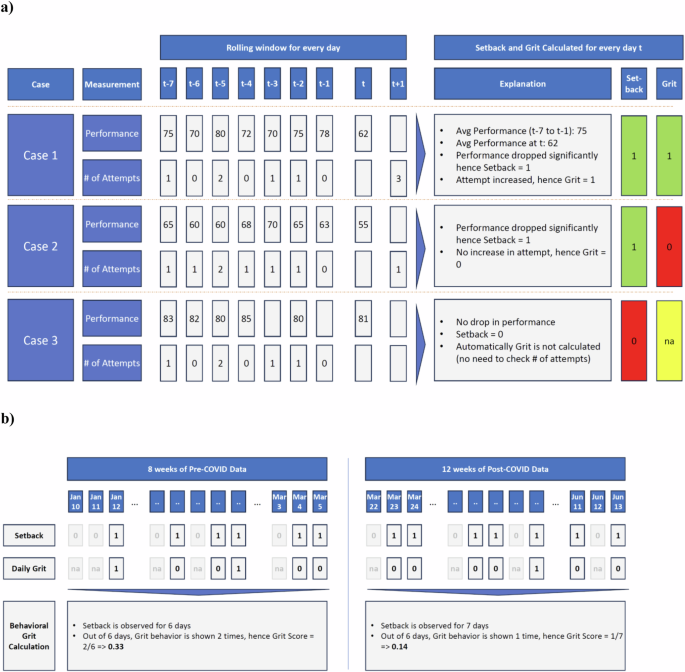
The figure gives concrete examples of the calculation of the behavioral grit measure. Panel a shows how setbacks are determined, and consequently, daily grit. Note that daily grit is not calculated when there is no setback. Panel b shows the calculation of the average behavioral grit for the pre- and post-Covid periods.
In our sample, we have 56 days of observations for the pre-covid and 84 days of observations for the during-covid period. Since we compare with an average from the previous week, we omit first-week observations both from pre and during-covid periods. Note that before the start of the “covid period” in our data, there was a 16-day break, where average activity on the platform is 90% less than both the pre- and post-covid periods. Therefore, we have 49 effective days for pre-Covid and 77 days for during-Covid. If performance does not decline sizably or increases on a given day, the grit variable for that day is not defined. Also, since there is little room for performance improvement for very high-performing students (whose daily score average is consistently higher than 90), these students are omitted from the analysis.
We should note that there may be other ways to define “behavioral grit” using such data. Our results are largely robust to a set of variations in the assumptions we make in the definition; for example, if we look at effort not only the day after, but two days after the setback, or if we define “setback” to be only when the student registers a “bottom 20%” performance based on his/her historic performance distribution.
The survey questions in the Duckworth-Quinn short grit scale are reproduced below:
New ideas and projects sometimes distract me from previous ones
Setbacks don’t discourage me. I bounce back from disappointments faster than most people
I have been obsessed with an idea or project for a short time but later lost interest
I am a hard worker
I often set a goal but later choose to follow a different one
I have difficulty keeping my focus on projects that take more than a few months to complete
I finish whatever I begin
I am diligent
We use data coming from 1920 students, in 17 schools and 229 classes, out of which 1279 are female and 641 are male. The students are middle- and high schoolers, and the data include five grades: grades 5 to 9. To be specific, we have 391, 262, 488, 600 and 179 students in grade 5 to 9, respectively. Note that during our data period, there were only two students changing school, and these have been omitted from the analysis. In case of class changes, we take the last class that the student was enrolled in in the academic year as the relevant class. We have access to objective scores from a diagnostic test on math and science as well as a survey, which were all run before the pandemic, in Fall 2019. In addition, we have platform data from the period January 10 to March 5, 2020 (8 weeks), which we define as the pre-Covid period. The post-Covid period goes from 22 March, 2020 (the day when anti-Covid measures were taken in the UAE and fully remote schooling started), to June 13, 2020 (12 weeks). We omit the intermediate period March 5 to 22, 2020, as the schools were closed and there were very limited attempts on the platform. Note that the sample we use in this paper reflects the set of students, out of a representative sample of all Abu Dhabi public school students, who completed both the diagnostic test (mandatory) and the grit survey (optional). There may be selection in the sample in the sense that the lower tail in terms of motivation have likely been left out, but our results on the effect of grit may be considered as conservative in this sample.
In addition to the above variables, in additional analysis of revealed preference data on non-cognitive skills, we implement an elastic regression using 400 variables from the digital platform. These variables come from “click data” recorded on the platform, and include a detailed set of observables about students’ platform activity, such as accessing different types of instructional content for each subject (e.g. videos, reading material, exercises, tests) as well as the timing and duration of these activities. The machine-learning model reduces the size of coefficients to zero when they do not explain much variance, allowing the consideration of a wide range of potential explanatory variables. To reduce risk of confusion, we use principal components analysis (PCA), a technique for reducing dimensionality of datasets by creating new uncorrelated variables that successively maximize variance and minimize information loss. We use PCA on all of the features from the digital platform. We then report the elastic regression including only the variables selected by elastic regression.
Elastic regression is a statistical method used to analyze the relationship between a dependent variable and one or more independent variables. It combines two popular regression techniques: ridge regression and lasso regression. In traditional linear regression, the goal is to find a line that best fits the data points by minimizing the sum of squared differences between the predicted values and the actual values. However, when dealing with a large number of variables or when the variables are highly correlated, linear regression can lead to overfitting or unstable estimates. Elastic regression addresses these issues by introducing two penalty terms to the regression equation. The first term, borrowed from ridge regression, shrinks the coefficients of the variables towards zero, reducing their impact on the model. The second term, borrowed from Lasso regression, can set some coefficients exactly to zero, effectively performing variable selection. By combining these two penalties, elastic regression strikes a balance between reducing the complexity of the model and selecting relevant variables. It is particularly useful when dealing with high-dimensional data, where the number of variables is larger than the number of observations. This machine-learning model reduces the size of coefficients to zero when they do not explain much variance, allowing the consideration of a wide range of potential confounding variables so as to address concerns of possible omitted variable bias 26 , 27 .
We also use principal components analysis (PCA), a technique for reducing the dimensionality of datasets by creating uncorrelated variables that successively maximize variance and minimize information loss. Principal Component Analysis (PCA) is a statistical technique used to reduce the dimensionality of a dataset while retaining most of its variation. It is commonly used for feature extraction and data compression. PCA works by identifying the directions (principal components) in which the data varies the most. These principal components are linear combinations of the original variables and are orthogonal to each other, meaning they are uncorrelated. The first principal component captures the largest amount of variation in the data, followed by the second principal component, which captures the second largest amount of variation, and so on. By selecting a subset of the top principal components, we can effectively reduce the dimensionality of the data while preserving its essential structure. PCA has numerous applications in various fields, including biology, genetics, neuroscience, and image processing. We use PCA on all of the variables coming from click data from the digital learning platform, and then run a multivariate regression that includes the principal components selected by elastic regression along with our measure of behavioral grit.
Data availability
The data that used in the paper comes from students in public schools in the United Arab Emirates. The authors have received the de-identified data from ALEF Education. The students are minors and therefore the data is sensitive. Due to the legal agreement between ALEF Education and the Ministry of Education of the United Arab Emirates, the public sharing of data is restricted. Requests for data underlying the results in the study can be made to the data owner, United Arab Emirates Ministry of Education ( https://www.moe.gov.ae/ ).
Code availability
The code is available upon request from the authors.
Duckworth, A. L., Kirby, T. A., Tsukayama, E., Berstein, H. & Ericsson, K. A. Deliberate practice spells success: why grittier competitors triumph at the national spelling bee. Soc. Psychol. Pers. Sci. 2 , 174–181 (2011).
Article Google Scholar
Eskreis-Winkler, L., Duckworth, A. L., Shulman, E. P. & Beal, S. The grit effect: predicting retention in the military, the workplace, school and marriage. Front. Psychol. 5 , 36 (2014).
Article PubMed PubMed Central Google Scholar
Dweck, C. Mindset: The New Psychology of Success (Random House, 2006).
Paunesku, D. et al. Mind-set interventions are a scalable treatment for academic underachievement. Psychol. Sci. 26 , 784–793 (2015).
Article PubMed Google Scholar
City, E. A., Elmore, R. F., Fiarman, S. E., & Teitel, L. Instructional Rounds in Education (Harvard Education Press) 30 (2009).
McCombs, B. L. The role of the self-system in self-regulated learning. Contemp. Educ. Psychol. 11 , 314–332 (1986).
Engzell, P., Frey, A. & Verhagen, M. D. Learning loss due to school closures during the COVID- 19 pandemic. Proc. Natl. Acad. Sci . 118 (2021).
Duckworth, A. L. & Quinn, P. D. Development and validation of the Short Grit Scale (GRIT–S). J. Pers. Assess. 91 , 166–174 (2009).
Zisman, C. & Ganzach, Y. In a representative sample grit has a negligible effect on educational and economic success compared to intelligence. Soc. Psychol. Pers. Sci. 12 , 296–303 (2021).
Bertrand, M. & Mullainathan, S. Do people mean what they say? Implications for subjective survey data. Am. Econ. Rev. 91 , 67–72 (2001).
Alan, S., Boneva, T. & Ertac, S. Ever failed, try again, succeed better: results from a randomized educational intervention on grit. Q. J. Econ. 134 , 1121–1162 (2019).
Google Scholar
Hanushek, E. A. & Woessmann, L. The Economic Impacts of Learning Losses (OECD Education Working Papers, 2020).
Zettler, I. et al. The role of personality in COVID-19-related perceptions, evaluations, and behaviors: findings across five samples, nine traits, and 17 criteria. Soc. Psychol. Pers. Sci . 13 , 299–310 (2022).
Volk, A. A., Brazil, K. J., Franklin-Luther, P., Dane, A. V. & Vaillancourt, T. The influence of demographics and personality on COVID-19 coping in young adults. Pers. Individ Differ. 168 , 110398 (2021).
Johnston, D. W., Kung, C. S. J., & Shields, M. A. Who is resilient in a time of crisis? The importance of financial and non-financial resources. IZA Discussion Paper No. 13720 (2020).
Casali, N., Feraco, T. & Meneghetti, C. Keep going, keep growing: a longitudinal analysis of grit, posttraumatic growth, and life satisfaction in school students under COVID-19. Learn. Individ. Differ. 105 , 102320 (2023).
Lytle, A. & Shin, J. L. Resilience and grit predict fewer academic and career concerns among first-year undergraduate students during COVID-19. Soc. Psychol. Educ. 26 , 227–240 (2023).
Luceño-Moreno, L., Talavera-Velasco, B., García-Albuerne, Y. & Martín-García, J. Symptoms of posttraumatic stress, anxiety, depression, levels of resilience and burnout in spanish health personnel during the COVID-19 pandemic. Int. J. Environ. Res. Public Health 17 , 5514 (2020).
Zhao, H., Xiong, J., Zhang, Z. & Qi, C. Growth mindset and college students’ learning engagement during the COVID-19 pandemic: a serial mediation model. Front. Psychol. 12 , 621094 (2021).
Ghanizadeh, A. Higher education amid COVID-19 pandemic: Contributions from resilience, positive orientation and grit. J. Appl. Res. High. Educ. 14 , 1670–1685 (2022).
Sulla, F., Aquino, A. & Rollo, D. University students’ online learning during COVID-19: the role of grit in academic performance. Front. Psychol. 13 , 825047 (2022).
Credé, M., Tynan, M. C. & Harms, P. D. Much ado about grit: a meta-analytic synthesis of the grit literature. J. Pers. Soc. Psychol. 113 , 492 (2017).
Ponnock, A. et al. Grit and conscientiousness: another jangle fallacy. J. Res. Pers . 89 (2020).
Yeager, D. S. et al. A national experiment reveals where a growth mindset improves achievement. Nature 573 , 364–369 (2019).
Article CAS PubMed PubMed Central Google Scholar
Marina, M. & Bonacini, L. Coronavirus pandemic, remote learning and education inequalities. GLO Discussion Paper. No. 679, Global Labor Organization (GLO), Essen (2020).
Zou, H. & Hastie, T. Regularization and variable selection via the elastic net. J. R. Stat. Soc. B 67 , 301–320 (2005).
Friedman, J., Hastie, T. & Tibshirani, R. Regularization paths for generalized linear models via coordinate descent. J. Stat. Softw. 33 , 1–22 (2010).
Download references
Author information
Authors and affiliations.
Toulouse School of Economics, Institute for Advanced Studies, 21 allée de Brienne, 31015 Toulouse cedex 6, Toulouse, France
Daniel L. Chen
Koc University Department of Economics, Sariyer, Istanbul, 34450, Turkey
Seda Ertac & Emrah Yilmaz
Technology Management and Decision Sciences, INSEAD, 77300, Fontainebleau, France
Theodoros Evgeniou
Alef Education Building 2, TwoFour54, P.O. Box 769719, Abu Dhabi, UAE
Xin Miao & Ali Nadaf
FTI Delta Analytics Center of Excellence, The Buildings by Daman - Office 1501 - Za’abeel-DIFC, Dubai, UAE
Emrah Yilmaz
You can also search for this author in PubMed Google Scholar
Contributions
Idea and Research Conceptualization: S.E., D.L.C., T.E. and the ALEF team (A.N. and X.M.) contributed significantly to the conceptualization and design of the research. Write-up: The manuscript was primarily written by SE and DLC, with substantial input and review from T.E., A.N., X.M., and E.Y. Data Analysis: E.Y. took the lead in data analysis, with significant contributions from DLC, ensuring the integrity and accuracy of the data analysis process. All authors (S.E, D.L.C., T.E., A.N., X.M., and E.Y.) read and approved the completed version, and accept accountability for all aspects of the work.
Corresponding author
Correspondence to Daniel L. Chen .
Ethics declarations
Competing interests.
The authors declare no competing interests.
Additional information
Publisher’s note Springer Nature remains neutral with regard to jurisdictional claims in published maps and institutional affiliations.
Rights and permissions
Open Access This article is licensed under a Creative Commons Attribution-NonCommercial-NoDerivatives 4.0 International License, which permits any non-commercial use, sharing, distribution and reproduction in any medium or format, as long as you give appropriate credit to the original author(s) and the source, provide a link to the Creative Commons licence, and indicate if you modified the licensed material. You do not have permission under this licence to share adapted material derived from this article or parts of it. The images or other third party material in this article are included in the article’s Creative Commons licence, unless indicated otherwise in a credit line to the material. If material is not included in the article’s Creative Commons licence and your intended use is not permitted by statutory regulation or exceeds the permitted use, you will need to obtain permission directly from the copyright holder. To view a copy of this licence, visit http://creativecommons.org/licenses/by-nc-nd/4.0/ .
Reprints and permissions

About this article
Cite this article.
Chen, D.L., Ertac, S., Evgeniou, T. et al. Grit and academic resilience during the COVID-19 pandemic. npj Sci. Learn. 9 , 57 (2024). https://doi.org/10.1038/s41539-024-00265-3
Download citation
Received : 08 May 2023
Accepted : 07 August 2024
Published : 13 September 2024
DOI : https://doi.org/10.1038/s41539-024-00265-3
Share this article
Anyone you share the following link with will be able to read this content:
Sorry, a shareable link is not currently available for this article.
Provided by the Springer Nature SharedIt content-sharing initiative
Quick links
- Explore articles by subject
- Guide to authors
- Editorial policies
Sign up for the Nature Briefing newsletter — what matters in science, free to your inbox daily.

- Our Mission
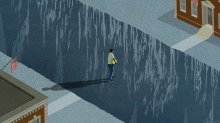
Covid-19’s Impact on Students’ Academic and Mental Well-Being
The pandemic has revealed—and exacerbated—inequities that hold many students back. Here’s how teachers can help.
The pandemic has shone a spotlight on inequality in America: School closures and social isolation have affected all students, but particularly those living in poverty. Adding to the damage to their learning, a mental health crisis is emerging as many students have lost access to services that were offered by schools.
No matter what form school takes when the new year begins—whether students and teachers are back in the school building together or still at home—teachers will face a pressing issue: How can they help students recover and stay on track throughout the year even as their lives are likely to continue to be disrupted by the pandemic?
New research provides insights about the scope of the problem—as well as potential solutions.
The Achievement Gap Is Likely to Widen
A new study suggests that the coronavirus will undo months of academic gains, leaving many students behind. The study authors project that students will start the new school year with an average of 66 percent of the learning gains in reading and 44 percent of the learning gains in math, relative to the gains for a typical school year. But the situation is worse on the reading front, as the researchers also predict that the top third of students will make gains, possibly because they’re likely to continue reading with their families while schools are closed, thus widening the achievement gap.
To make matters worse, “few school systems provide plans to support students who need accommodations or other special populations,” the researchers point out in the study, potentially impacting students with special needs and English language learners.
Of course, the idea that over the summer students forget some of what they learned in school isn’t new. But there’s a big difference between summer learning loss and pandemic-related learning loss: During the summer, formal schooling stops, and learning loss happens at roughly the same rate for all students, the researchers point out. But instruction has been uneven during the pandemic, as some students have been able to participate fully in online learning while others have faced obstacles—such as lack of internet access—that have hindered their progress.
In the study, researchers analyzed a national sample of 5 million students in grades 3–8 who took the MAP Growth test, a tool schools use to assess students’ reading and math growth throughout the school year. The researchers compared typical growth in a standard-length school year to projections based on students being out of school from mid-March on. To make those projections, they looked at research on the summer slide, weather- and disaster-related closures (such as New Orleans after Hurricane Katrina), and absenteeism.
The researchers predict that, on average, students will experience substantial drops in reading and math, losing roughly three months’ worth of gains in reading and five months’ worth of gains in math. For Megan Kuhfeld, the lead author of the study, the biggest takeaway isn’t that learning loss will happen—that’s a given by this point—but that students will come back to school having declined at vastly different rates.
“We might be facing unprecedented levels of variability come fall,” Kuhfeld told me. “Especially in school districts that serve families with lots of different needs and resources. Instead of having students reading at a grade level above or below in their classroom, teachers might have kids who slipped back a lot versus kids who have moved forward.”
Disproportionate Impact on Students Living in Poverty and Students of Color
Horace Mann once referred to schools as the “great equalizers,” yet the pandemic threatens to expose the underlying inequities of remote learning. According to a 2015 Pew Research Center analysis , 17 percent of teenagers have difficulty completing homework assignments because they do not have reliable access to a computer or internet connection. For Black students, the number spikes to 25 percent.
“There are many reasons to believe the Covid-19 impacts might be larger for children in poverty and children of color,” Kuhfeld wrote in the study. Their families suffer higher rates of infection, and the economic burden disproportionately falls on Black and Hispanic parents, who are less likely to be able to work from home during the pandemic.
Although children are less likely to become infected with Covid-19, the adult mortality rates, coupled with the devastating economic consequences of the pandemic, will likely have an indelible impact on their well-being.
Impacts on Students’ Mental Health
That impact on well-being may be magnified by another effect of school closures: Schools are “the de facto mental health system for many children and adolescents,” providing mental health services to 57 percent of adolescents who need care, according to the authors of a recent study published in JAMA Pediatrics . School closures may be especially disruptive for children from lower-income families, who are disproportionately likely to receive mental health services exclusively from schools.
“The Covid-19 pandemic may worsen existing mental health problems and lead to more cases among children and adolescents because of the unique combination of the public health crisis, social isolation, and economic recession,” write the authors of that study.
A major concern the researchers point to: Since most mental health disorders begin in childhood, it is essential that any mental health issues be identified early and treated. Left untreated, they can lead to serious health and emotional problems. In the short term, video conferencing may be an effective way to deliver mental health services to children.
Mental health and academic achievement are linked, research shows. Chronic stress changes the chemical and physical structure of the brain, impairing cognitive skills like attention, concentration, memory, and creativity. “You see deficits in your ability to regulate emotions in adaptive ways as a result of stress,” said Cara Wellman, a professor of neuroscience and psychology at Indiana University in a 2014 interview . In her research, Wellman discovered that chronic stress causes the connections between brain cells to shrink in mice, leading to cognitive deficiencies in the prefrontal cortex.
While trauma-informed practices were widely used before the pandemic, they’re likely to be even more integral as students experience economic hardships and grieve the loss of family and friends. Teachers can look to schools like Fall-Hamilton Elementary in Nashville, Tennessee, as a model for trauma-informed practices .
3 Ways Teachers Can Prepare
When schools reopen, many students may be behind, compared to a typical school year, so teachers will need to be very methodical about checking in on their students—not just academically but also emotionally. Some may feel prepared to tackle the new school year head-on, but others will still be recovering from the pandemic and may still be reeling from trauma, grief, and anxiety.
Here are a few strategies teachers can prioritize when the new school year begins:
- Focus on relationships first. Fear and anxiety about the pandemic—coupled with uncertainty about the future—can be disruptive to a student’s ability to come to school ready to learn. Teachers can act as a powerful buffer against the adverse effects of trauma by helping to establish a safe and supportive environment for learning. From morning meetings to regular check-ins with students, strategies that center around relationship-building will be needed in the fall.
- Strengthen diagnostic testing. Educators should prepare for a greater range of variability in student learning than they would expect in a typical school year. Low-stakes assessments such as exit tickets and quizzes can help teachers gauge how much extra support students will need, how much time should be spent reviewing last year’s material, and what new topics can be covered.
- Differentiate instruction—particularly for vulnerable students. For the vast majority of schools, the abrupt transition to online learning left little time to plan a strategy that could adequately meet every student’s needs—in a recent survey by the Education Trust, only 24 percent of parents said that their child’s school was providing materials and other resources to support students with disabilities, and a quarter of non-English-speaking students were unable to obtain materials in their own language. Teachers can work to ensure that the students on the margins get the support they need by taking stock of students’ knowledge and skills, and differentiating instruction by giving them choices, connecting the curriculum to their interests, and providing them multiple opportunities to demonstrate their learning.

The Impact of COVID-19 on the Careers of Women in Academic Sciences, Engineering, and Medicine (2021)
Chapter: 8 major findings and research questions, 8 major findings and research questions, introduction.
The COVID-19 pandemic, which began in late 2019, created unprecedented global disruption and infused a significant level of uncertainty into the lives of individuals, both personally and professionally, around the world throughout 2020. The significant effect on vulnerable populations, such as essential workers and the elderly, is well documented, as is the devastating effect the COVID-19 pandemic had on the economy, particularly brick-and-mortar retail and hospitality and food services. Concurrently, the deaths of unarmed Black people at the hands of law enforcement officers created a heightened awareness of the persistence of structural injustices in U.S. society.
Against the backdrop of this public health crisis, economic upheaval, and amplified social consciousness, an ad hoc committee was appointed to review the potential effects of the COVID-19 pandemic on women in academic science, technology, engineering, mathematics, and medicine (STEMM) during 2020. The committee’s work built on the National Academies of Sciences, Engineering, and Medicine report Promising Practices for Addressing the Underrepresentation of Women in Science, Engineering, and Medicine: Opening Doors (the Promising Practices report), which presents evidence-based recommendations to address the well-established structural barriers that impede the advancement of women in STEMM. However, the committee recognized that none of the actions identified in the Promising Practices report were conceived within the context of a pandemic, an economic downturn, or the emergence of national protests against structural racism. The representation and vitality of academic women in STEMM had already warranted national attention prior to these events, and the COVID-19
pandemic appeared to represent an additional risk to the fragile progress that women had made in some STEMM disciplines. Furthermore, the future will almost certainly hold additional, unforeseen disruptions, which underscores the importance of the committee’s work.
In times of stress, there is a risk that the divide will deepen between those who already have advantages and those who do not. In academia, senior and tenured academics are more likely to have an established reputation, a stable salary commitment, and power within the academic system. They are more likely, before the COVID-19 pandemic began, to have established professional networks, generated data that can be used to write papers, and achieved financial and job security. While those who have these advantages may benefit from a level of stability relative to others during stressful times, those who were previously systemically disadvantaged are more likely to experience additional strain and instability.
As this report has documented, during 2020 the COVID-19 pandemic had overall negative effects on women in academic STEMM in areas such productivity, boundary setting and boundary control, networking and community building, burnout rates, and mental well-being. The excessive expectations of caregiving that often fall on the shoulders of women cut across career timeline and rank (e.g., graduate student, postdoctoral scholar, non-tenure-track and other contingent faculty, tenure-track faculty), institution type, and scientific discipline. Although there have been opportunities for innovation and some potential shifts in expectations, increased caregiving demands associated with the COVID-19 pandemic in 2020, such as remote working, school closures, and childcare and eldercare, had disproportionately negative outcomes for women.
The effects of the COVID-19 pandemic on women in STEMM during 2020 are understood better through an intentionally intersectional lens. Productivity, career, boundary setting, mental well-being, and health are all influenced by the ways in which social identities are defined and cultivated within social and power structures. Race and ethnicity, sexual orientation, gender identity, academic career stage, appointment type, institution type, age, and disability status, among many other factors, can amplify or diminish the effects of the COVID-19 pandemic for a given person. For example, non-cisgender women may be forced to return to home environments where their gender identity is not accepted, increasing their stress and isolation, and decreasing their well-being. Women of Color had a higher likelihood of facing a COVID-19–related death in their family compared with their white, non-Hispanic colleagues. The full extent of the effects of the COVID-19 pandemic for women of various social identities was not fully understood at the end of 2020.
Considering the relative paucity of women in many STEMM fields prior to the COVID-19 pandemic, women are more likely to experience academic isolation, including limited access to mentors, sponsors, and role models that share gender, racial, or ethnic identities. Combining this reality with the physical isolation stipulated by public health responses to the COVID-19 pandemic,
women in STEMM were subject to increasing isolation within their fields, networks, and communities. Explicit attention to the early indicators of how the COVID-19 pandemic affected women in academic STEMM careers during 2020, as well as attention to crisis responses throughout history, may provide opportunities to mitigate some of the long-term effects and potentially develop a more resilient and equitable academic STEMM system.
MAJOR FINDINGS
Given the ongoing nature of the COVID-19 pandemic, it was not possible to fully understand the entirety of the short- or long-term implications of this global disruption on the careers of women in academic STEMM. Having gathered preliminary data and evidence available in 2020, the committee found that significant changes to women’s work-life boundaries and divisions of labor, careers, productivity, advancement, mentoring and networking relationships, and mental health and well-being have been observed. The following findings represent those aspects that the committee agreed have been substantiated by the preliminary data, evidence, and information gathered by the end of 2020. They are presented either as Established Research and Experiences from Previous Events or Impacts of the COVID-19 Pandemic during 2020 that parallel the topics as presented in the report.
Established Research and Experiences from Previous Events
Leading up to the COVID-19 pandemic, the representation of women has slowly increased in STEMM fields, from acquiring Ph.D.s to holding leadership positions, but with caveats to these limited steps of progress; for example, women representation in leadership positions tends to be at institutions with less prestige and fewer resources. While promising and encouraging, such progress is fragile and prone to setbacks especially in times of crisis (see ). | |
Social crises (e.g., terrorist attacks, natural disasters, racialized violence, and infectious diseases) and COVID-19 pandemic-related disruptions to workload and schedules, added to formerly routine job functions and health risks, have the potential to exacerbate mental health conditions such as insomnia, depression, anxiety, and posttraumatic stress. All of these conditions occur more frequently among women than men. As multiple crises coincided during 2020, there is a greater chance that women will be affected mentally and physically (see and ). |
___________________
1 This finding is primarily based on research on cisgender women and men.
Structural racism is an omnipresent stressor for Women of Color, who already feel particularly isolated in many fields and disciplines. Attempts to ensure equity for all women may not necessarily create equity for women across various identities if targeted interventions designed to promote gender equity do not account for the racial and ethnic heterogeneity of women in STEMM (see , , and ). |
Impacts of the COVID-19 Pandemic during 2020
While some research indicates consistency in publications authored by women in specific STEMM disciplines, like Earth and space sciences, during 2020, several other preliminary measures of productivity suggest that COVID-19 disruptions have disproportionately affected women compared with men. Reduced productivity may be compounded by differences in the ways research is conducted, such as whether field research or face-to-face engagement with human subjects is required (see ). | |
Many administrative decisions regarding institutional supports made during 2020, such as work-from-home provisions and extensions on evaluations or deliverables, are likely to exacerbate underlying gender-based inequalities in academic advancement rather than being gender neutral as assumed. For example, while colleges and universities have offered extensions for those on the tenure track and federal and private funders have offered extensions on funding and grants, these changes do not necessarily align with the needs expressed by women, such as the need for flexibility to contend with limited availability of caregiving and requests for a reduced workload, nor do they generally benefit women faculty who are not on the tenure track. Furthermore, provision of institutional support may be insufficient if it does not account for the challenges faced by those with multiple marginalized identities (see and ). | |
Organizational-level approaches may be needed to address challenges that have emerged as a result of the COVID-19 pandemic in 2020, as well as those challenges that may have existed before the pandemic but are now more visible and amplified. Reliance on individual coping strategies may be insufficient (see and ). | |
The COVID-19 pandemic has intensified complications related to worklife boundaries that largely affect women. Preliminary evidence |
from 2020 suggests women in academic STEMM are experiencing increased workload, decreased productivity, changes in interactions, and difficulties from remote work caused by the COVID-19 pandemic and associated disruptions. Combined with the gendered division of nonemployment labor that affected women before the pandemic, these challenges have been amplified, as demonstrated by a lack of access to childcare, children’s heightened behavioral and academic needs, increased eldercare demands, and personal physical and mental health concerns. These are particularly salient for women who are parents or caregivers (see ). | |
During the COVID-19 pandemic, technology has allowed for the continuation of information exchange and many collaborations. In some cases technology has facilitated the increased participation of women and underrepresented groups. However, preliminary indicators also show gendered impacts on science and scientific collaborations during 2020. These arise because some collaborations cannot be facilitated online and some collaborations face challenges including finding time in the day to engage synchronously, which presents a larger burden for women who manage the larger share of caregiving and other household duties, especially during the first several months of the COVID-19 pandemic (see ). | |
During the COVID-19 pandemic in 2020, some professional societies adapted to the needs of members as well as to broader interests of individuals engaged in the disciplines they serve. Transitioning conferences to virtual platforms has produced both positive outcomes, such as lower attendance costs and more open access to content, and negative outcomes, including over-flexibility (e.g., scheduling meetings at non-traditional work hours; last-minute changes) and opportunities for bias in virtual environments (see ). | |
During the COVID-19 pandemic in 2020, many of the decision-making processes, including financial decisions like lay-offs and furloughs, that were quickly implemented contributed to unilateral decisions that frequently deviated from effective practices in academic governance, such as those in crisis and equity-minded leadership. Fast decisions greatly affected contingent and nontenured faculty members—positions that are more often occupied by women and People of Color. In 2020, these financial decisions already had negative, short-term effects and may portend long-term consequences (see ). | |
Social support, which is particularly important during stressful situations, is jeopardized by the physical isolation and restricted social interactions that have |
been imposed during the COVID-19 pandemic. For women who are already isolated within their specific fields or disciplines, additional social isolation may be an important contributor to added stress (see ). | |
For women in the health professions, major risk factors during the COVID-19 pandemic in 2020 included unpredictability in clinical work, evolving clinical and leadership roles, the psychological demands of unremitting and stressful work, and heightened health risks to family and self (see ). |
RESEARCH QUESTIONS
While this report compiled much of the research, data, and evidence available in 2020 on the effects of the COVID-19 pandemic, future research is still needed to understand all the potential effects, especially any long-term implications. The research questions represent areas the committee identified for future research, rather than specific recommendations. They are presented in six categories that parallel the chapters of the report: Cross-Cutting Themes; Academic Productivity and Institutional Responses; Work-Life Boundaries and Gendered Divisions of Labor; Collaboration, Networking, and Professional Societies; Academic Leadership and Decision-Making; and Mental Health and Well-being. The committee hopes the report will be used as a basis for continued understanding of the impact of the COVID-19 pandemic in its entirety and as a reference for mitigating impacts of future disruptions that affect women in academic STEMM. The committee also hopes that these research questions may enable academic STEMM to emerge from the pandemic era a stronger, more equitable place for women. Therefore, the committee identifies two types of research questions in each category; listed first are those questions aimed at understanding the impacts of the disruptions from the COVID-19 pandemic, followed by those questions exploring the opportunities to help support the full participation of women in the future.
Cross-Cutting Themes
- What are the short- and long-term effects of the COVID-19 pandemic on the career trajectories, job stability, and leadership roles of women, particularly of Black women and other Women of Color? How do these effects vary across institutional characteristics, 2 discipline, and career stage?
2 Institutional characteristics include different institutional types (e.g., research university, liberal arts college, community college), locales (e.g., urban, rural), missions (e.g., Historically Black Colleges and Universities, Hispanic-Serving Institutions, Asian American/Native American/Pacific Islander-Serving Institutions, Tribal Colleges and Universities), and levels of resources.
- How did the confluence of structural racism, economic hardships, and environmental disruptions affect Women of Color during the COVID-19 pandemic? Specifically, how did the murder of George Floyd, Breonna Taylor, and other Black citizens impact Black women academics’ safety, ability to be productive, and mental health?
- How has the inclusion of women in leadership and other roles in the academy influenced the ability of institutions to respond to the confluence of major social crises during the COVID-19 pandemic?
- How can institutions build on the involvement women had across STEMM disciplines during the COVID-19 pandemic to increase the participation of women in STEMM and/or elevate and support women in their current STEMM-related positions?
- How can institutions adapt, leverage, and learn from approaches developed during 2020 to attend to challenges experienced by Women of Color in STEMM in the future?
Academic Productivity and Institutional Responses
- How did the institutional responses (e.g., policies, practices) that were outlined in the Major Findings impact women faculty across institutional characteristics and disciplines?
- What are the short- and long-term effects of faculty evaluation practices and extension policies implemented during the COVID-19 pandemic on the productivity and career trajectories of members of the academic STEMM workforce by gender?
- What adaptations did women use during the transition to online and hybrid teaching modes? How did these techniques and adaptations vary as a function of career stage and institutional characteristics?
- What are examples of institutional changes implemented in response to the COVID-19 pandemic that have the potential to reduce systemic barriers to participation and advancement that have historically been faced by academic women in STEMM, specifically Women of Color and other marginalized women in STEMM? How might positive institutional responses be leveraged to create a more resilient and responsive higher education ecosystem?
- How can or should funding arrangements be altered (e.g., changes in funding for research and/or mentorship programs) to support new ways of interaction for women in STEMM during times of disruption, such as the COVID-19 pandemic?
Work-Life Boundaries and Gendered Divisions of Labor
- How do different social identities (e.g., racial; socioeconomic status; culturally, ethnically, sexually, or gender diverse; immigration status; parents of young children and other caregivers; women without partners) influence the management of work-nonwork boundaries? How did this change during the COVID-19 pandemic?
- How have COVID-19 pandemic-related disruptions affected progress toward reducing the gender gap in academic STEMM labor-force participation? How does this differ for Women of Color or women with caregiving responsibilities?
- How can institutions account for the unique challenges of women faculty with parenthood and caregiving responsibilities when developing effective and equitable policies, practices, or programs?
- How might insights gained about work-life boundaries during the COVID-19 pandemic inform how institutions develop and implement supportive resources (e.g., reductions in workload, on-site childcare, flexible working options)?
Collaboration, Networking, and Professional Societies
- What were the short- and long-term effects of the COVID-19 pandemic-prompted switch from in-person conferences to virtual conferences on conference culture and climate, especially for women in STEMM?
- How will the increase in virtual conferences specifically affect women’s advancement and career trajectories? How will it affect women’s collaborations?
- How has the shift away from attending conferences and in-person networking changed longer-term mentoring and sponsoring relationships, particularly in terms of gender dynamics?
- How can institutions maximize the benefits of digitization and the increased use of technology observed during the COVID-19 pandemic to continue supporting women, especially marginalized women, by increasing accessibility, collaborations, mentorship, and learning?
- How can organizations that support, host, or facilitate online and virtual conferences and networking events (1) ensure open and fair access to participants who face different funding and time constraints; (2) foster virtual connections among peers, mentors, and sponsors; and (3) maintain an inclusive environment to scientists of all backgrounds?
- What policies, practices, or programs can be developed to help women in STEMM maintain a sense of support, structure, and stability during and after periods of disruption?
Academic Leadership and Decision-Making
- What specific interventions did colleges and universities initiate or prioritize to ensure that women were included in decision-making processes during responses to the COVID-19 pandemic?
- How effective were colleges and universities that prioritized equity-minded leadership, shared leadership, and crisis leadership styles at mitigating emerging and potential negative effects of the COVID-19 pandemic on women in their communities?
- What specific aspects of different leadership models translated to more effective strategies to advance women in STEMM, particularly during the COVID-19 pandemic?
- How can examples of intentional inclusion of women in decision-making processes during the COVID-19 pandemic be leveraged to develop the engagement of women as leaders at all levels of academic institutions?
- What are potential “top-down” structural changes in academia that can be implemented to mitigate the adverse effects of the COVID-19 pandemic or other disruptions?
- How can academic leadership, at all levels, more effectively support the mental health needs of women in STEMM?
Mental Health and Well-being
- What is the impact of the COVID-19 pandemic and institutional responses on the mental health and well-being of members of the academic STEMM workforce as a function of gender, race, and career stage?
- How are tools and diagnostic tests to measure aspects of wellbeing, including burnout and insomnia, used in academic settings? How does this change during times of increased stress, such as the COVID-19 pandemic?
- How might insights gained about mental health during the COVID-19 pandemic be used to inform preparedness for future disruptions?
- How can programs that focus on changes in biomarkers of stress and mood dysregulation, such as levels of sleep, activity, and texting patterns, be developed and implemented to better engage women in addressing their mental health?
- What are effective interventions to address the health of women academics in STEMM that specifically account for the effects of stress on women? What are effective interventions to mitigate the excessive levels of stress for Women of Color?
This page intentionally left blank.
The spring of 2020 marked a change in how almost everyone conducted their personal and professional lives, both within science, technology, engineering, mathematics, and medicine (STEMM) and beyond. The COVID-19 pandemic disrupted global scientific conferences and individual laboratories and required people to find space in their homes from which to work. It blurred the boundaries between work and non-work, infusing ambiguity into everyday activities. While adaptations that allowed people to connect became more common, the evidence available at the end of 2020 suggests that the disruptions caused by the COVID-19 pandemic endangered the engagement, experience, and retention of women in academic STEMM, and may roll back some of the achievement gains made by women in the academy to date.
The Impact of COVID-19 on the Careers of Women in Academic Sciences, Engineering, and Medicine identifies, names, and documents how the COVID-19 pandemic disrupted the careers of women in academic STEMM during the initial 9-month period since March 2020 and considers how these disruptions - both positive and negative - might shape future progress for women. This publication builds on the 2020 report Promising Practices for Addressing the Underrepresentation of Women in Science, Engineering, and Medicine to develop a comprehensive understanding of the nuanced ways these disruptions have manifested. The Impact of COVID-19 on the Careers of Women in Academic Sciences, Engineering, and Medicine will inform the academic community as it emerges from the pandemic to mitigate any long-term negative consequences for the continued advancement of women in the academic STEMM workforce and build on the adaptations and opportunities that have emerged.
READ FREE ONLINE
Welcome to OpenBook!
You're looking at OpenBook, NAP.edu's online reading room since 1999. Based on feedback from you, our users, we've made some improvements that make it easier than ever to read thousands of publications on our website.
Do you want to take a quick tour of the OpenBook's features?
Show this book's table of contents , where you can jump to any chapter by name.
...or use these buttons to go back to the previous chapter or skip to the next one.
Jump up to the previous page or down to the next one. Also, you can type in a page number and press Enter to go directly to that page in the book.
Switch between the Original Pages , where you can read the report as it appeared in print, and Text Pages for the web version, where you can highlight and search the text.
To search the entire text of this book, type in your search term here and press Enter .
Share a link to this book page on your preferred social network or via email.
View our suggested citation for this chapter.
Ready to take your reading offline? Click here to buy this book in print or download it as a free PDF, if available.
Get Email Updates
Do you enjoy reading reports from the Academies online for free ? Sign up for email notifications and we'll let you know about new publications in your areas of interest when they're released.
Advertisement
Pakistani students’ perceptions about knowledge, use and impact of artificial intelligence (AI) on academic writing: a case study
- Published: 11 September 2024
Cite this article
- Shaista Rashid 1 ,
- Sadia Malik ORCID: orcid.org/0000-0002-4989-2359 2 ,
- Faheem Abbas 2 &
- Javaria Ahmad Khan 3
12 Accesses
Explore all metrics
Integrating artificial intelligence (AI) in language pedagogy can help learn and develop many skills. In this context, this study explores Pakistani students' perceptions and trends regarding the knowledge, use, and impact of AI on their academic writing. The data was collected using a quantitative method, using a questionnaire through cluster sampling of four faculties and random sampling of 229 students from Bahuddin Zakariya University, Multan, Pakistan. Data is subjected to frequency analysis, Kruskal–Wallis hypothesis test, and chi-square association test using SPSS. The findings reveal that most students agree regarding the knowledge, use, and impact of AI on their academic writing. For the Kruskal–Wallis test, significant variations are seen in semesters and age groups for all three variables; however, only the knowledge variable shows significant variation across faculties. Moreover, chi-square test results indicate an association among components of knowledge, use, and impact of AI. The research suggests that academia should introduce AI as a pedagogical tool to improve students' comprehension, productivity, and writing quality. Furthermore, trends indicate that comprehensive policy formulation should be implemented to equip students of all faculties, semesters, and age groups to use technology equally.
This is a preview of subscription content, log in via an institution to check access.
Access this article
Subscribe and save.
- Get 10 units per month
- Download Article/Chapter or eBook
- 1 Unit = 1 Article or 1 Chapter
- Cancel anytime
Price includes VAT (Russian Federation)
Instant access to the full article PDF.
Rent this article via DeepDyve
Institutional subscriptions
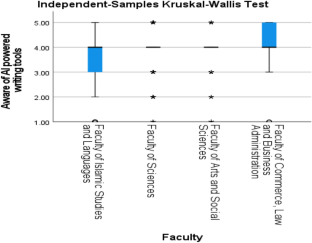
Similar content being viewed by others
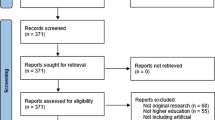
Artificial intelligence in higher education: the state of the field
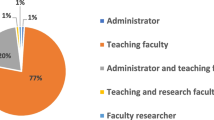
Algorithmically-driven writing and academic integrity: exploring educators' practices, perceptions, and policies in AI era
Learning What Works in Improving Writing: A Meta-Analysis of Technology—Oriented Studies Across Saudi Universities
Explore related subjects.
- Artificial Intelligence
- Digital Education and Educational Technology
Data availability
The data was collected through questionnaire using Google Forms; SPSS file can be provided if requested.
Ahmed, M., Siddiqui, M., & Usman, T. (2024). Impact of artificial intelligence-based writing assistant on the academic writing skills of university faculty in Pakistan. International Journal of Human and Society, 4 (1), 539–545.
Google Scholar
Aladini, A. (2023). AI applications impact on improving EFL university academic writing skills and their logical thinking. Al-’ulūm Al-Tarbawiyyaẗ, 31 (2), 25–44. https://doi.org/10.21608/ssj.2023.320166
Article Google Scholar
Ali, Z. (2020). Artificial intelligence (AI): A review of its uses in language teaching and learning. IOP Conference Series: Materials Science and Engineering, 769 (1), 012043. https://doi.org/10.1088/1757-899x/769/1/012043
Almusharraf, N., & Bailey, D. (2021). A regression analysis approach to measuring the influence of student characteristics on language learning strategies. International Journal of Instruction, 14 (4), 463–482. https://doi.org/10.29333/iji.2021.14428a
Beiki, M. (2022). Review of writing-related theories. Cultural Arts Research and Development, 2 (1), 27–33. https://doi.org/10.55121/card.v2i1.20
Burkhard. (2022). Student perceptions of AI-powered writing tools: Towards individualized teaching strategies. 19th International Conference on Cognition and Exploratory Learning in Digital Age (CELDA 2022) . https://doi.org/10.33965/celda2022_202207l010
Chaudhry, I. S., Sarwary, S. A. M., Refae, G. A. E., & Chabchoub, H. (2023). Time to revisit existing student’s performance evaluation approach in higher education sector in a new era of Chatgpt — a case study. Cogent Education, 10 (1), 2210461. https://doi.org/10.1080/2331186x.2023.2210461
Chen, L., Chen, P., & Lin, Z. (2020). Artificial intelligence in education: A review. IEEE Access, 8 , 75264–75278. https://doi.org/10.1109/access.2020.2988510
Cunningham, U., Rashid, S., & Van Le, T. (2019). The effect of learner training on the use of digital tools to support English writing skills. Asian EFL Journal , 21 , 27–49. http://uu.diva-portal.org/smash/record.jsf?pid=diva2:1300583
Dhara, S., Chatterjee, S., Chaudhuri, R., Goswami, A., & Ghosh, S. K. (2022). Artificial intelligence in assessment of students’ performance. In CRC Press eBooks (pp. 153–167). https://doi.org/10.1201/9781003184157-8
Dizon, G., & Gayed, J. M. (2021). Examining the impact of Grammarly on the quality of mobile L2 writing. The JALT CALL Journal, 17 (2), 74–92. https://doi.org/10.29140/jaltcall.v17n2.336
Feise, R. J. (2002). Do multiple outcome measures require p-value adjustment? BMC Medical Research Methodology, 2 (1), 8. https://doi.org/10.1186/1471-2288-2-8
Fitria, T. N. (2021). Grammarly as AI-powered English writing assistant: students’ alternative for writing English. Metathesis, 5 (1), 65–78. https://doi.org/10.31002/metathesis.v5i1.3519
Flavell, J. H. (1979). Metacognition and cognitive monitoring: A new area of cognitive-developmental inquiry. American Psychologist, 34 (10), 906–911. https://doi.org/10.1037/0003-066x.34.10.906
Ghafar, Z. N., Salh, H. F., Abdulrahim, M. A., Farxha, S. S., Arf, S. F., & Rahim, R. I. (2023). The role of artificial intelligence technology on English language learning: a literature review. Canadian Journal of Language and Literature Studies, 3 (2), 17–31. https://doi.org/10.53103/cjlls.v3i2.87
Ginting, P., Batubara, H. M., & Hasnah, Y. (2023). Artificial intelligence powered writing tools as adaptable aids for academic writing: Insight from EFL college learners in writing final project. Zenodo, 6 (10), 4640–4650. https://doi.org/10.5281/zenodo.8407887
Grájeda, A., Burgos, J., Olivera, P. C., & Sanjinés, A. (2023). Assessing student-perceived impact of using artificial intelligence tools: Construction of a synthetic index of application in higher education. Cogent Education, 11 (1), 2287917. https://doi.org/10.1080/2331186x.2023.2287917
Imran, M., & Almusharraf, N. (2023). Analyzing the role of ChatGPT as a writing assistant at higher education level: A systematic review of the literature. Contemporary Educational Technology, 15 (4), ep464. https://doi.org/10.30935/cedtech/13605
Kaur, P., Stoltzfus, J., & Yellapu, V. (2018). Descriptive statistics. Biostatistics, 4 (1), 60–67. https://doi.org/10.4103/ijam.ijam_7_18
Keleş, P. U., & Aydın, S. (2021). University students’ perceptions about artificial intelligence. Shanlax International Journal of Education, 9 , 212–220. https://doi.org/10.34293/education.v9is1-may.4014
Knowles, E. (2005). The Oxford dictionary of phrase and fable. In Oxford University Press eBooks . https://doi.org/10.1093/acref/9780198609810.001.0001
Kothari, C. R. (2004). Research methodology: Methods and techniques . New Age International.
LeCompte, M. D., & Schensul, J. J. (2015). Ethics in ethnography: A mixed methods approach . AltaMira Press.
Lei, H. (2022). High school students’ foreign language vocabulary acquisition in the era of artificial intelligence. Advances in Social Science, Education and Humanities Research, 637 , 669–662. https://doi.org/10.2991/assehr.k.220131.121
Li, A. W. (2023). Using Perceptive to support AI-based online writing assessment across the disciplines. Assessing Writing, 57 , 100746. https://doi.org/10.1016/j.asw.2023.100746
Mahmud, F. A. (2023). Investigating EFL students’ writing skills through artificial intelligence: Wordtune application as a tool. Journal of Language Teaching and Research, 14 (5), 1395–1404. https://doi.org/10.17507/jltr.1405.28
Malik, A. R., Pratiwi, Y., Andajani, K., Numertayasa, I. W., Suharti, S., Darwis, A., & Marzuki, M. (2023). Exploring artificial intelligence in academic essay: Higher education student’s perspective. International Journal of Educational Research Open, 5 , 100296. https://doi.org/10.1016/j.ijedro.2023.100296
Malik, S., Sadiq, U., & Khan, J. A. (2021). Belief, practices, and challenges of Pakistani primary grade government school teachers: Variable analysis affecting pronunciation and phonics teaching. Humanities & Social Sciences Reviews, 9 (1), 206–217. https://doi.org/10.18510/hssr.2021.9122
Marzuki, Widiati, U., Rusdin, D., Darwin, D., & Indrawati, I. (2023). The impact of AI writing tools on the content and organization of students’ writing: EFL teachers’ perspective. Cogent Education, 10 (2), 1–17. https://doi.org/10.1080/2331186x.2023.2236469
Mayo, D. G., & Cox, D. R. (2006). Frequentist statistics as a theory of inductive inference. In Institute of Mathematical Statistics eBooks (pp. 77–97). https://doi.org/10.1214/074921706000000400
McHugh, M. L. (2013). The chi-square test of independence. Biochemia Medica, 23 (2), 143–149. https://doi.org/10.11613/bm.2013.018
Nazari, N., Shabbir, M. S., & Setiawan, R. (2021). Application of artificial intelligence powered digital writing assistant in higher education: Randomized controlled trial. Heliyon, 7 (5), e07014. https://doi.org/10.1016/j.heliyon.2021.e07014
Ostertagová, E., Ostertag, O., & Kováč, J. (2014). Methodology and application of the Kruskal-Wallis test. Applied Mechanics and Materials, 611 , 115–120. https://doi.org/10.4028/www.scientific.net/amm.611.115
Pacheco-Mendoza, S., Guevara, C., Samaniego, J., & Fernandez, J. (2023). Artificial intelligence in higher education: A predictive model for academic performance. Education Sciences, 13 (10), 990. https://doi.org/10.3390/educsci13100990
Park, J. (2019). An AI-based English grammar checker vs. human raters in evaluating EFL learners’ writing. Multimedia-Assisted Language Learning , 22 (1), 112–131. https://www.kci.go.kr/kciportal/ci/sereArticleSearch/ciSereArtiView.kci?sereArticleSearchBean.artiId=ART002449524
Rashid, S., Cunningham, U., Watson, K., & Howard, J. (2018). Revisiting the digital divide(s): Technology-enhanced English language practices at a university in Pakistan. Australian Journal of Applied Linguistics, 1 (2), 64–87. https://doi.org/10.29140/ajal.v1n2.7
Ribeiro, R. (2022). AI in English Language Learning | Cambridge English . World of Better Learning | Cambridge University Press. https://www.cambridge.org/elt/blog/2020/03/09/artificial-intelligence-english-language-learning/
Rothman, K. J. (1990). No adjustments are needed for multiple comparisons. Epidemiology, 1 (1), 43–46. https://doi.org/10.1097/00001648-199001000-00010
Rusmiyanto, R., Huriati, N., Fitriani, N., Tyas, N. K., Rofi’i, A., & Sari, M. N. (2023). The role of artificial intelligence (AI) in developing English language learner’s communication skills. Journal on Education, 6 (1), 750–757. https://doi.org/10.31004/joe.v6i1.2990
Saini, N. (2023). Research paper on artificial intelligence and its applications. International Journal for Research Trends and Innovation, 8 (4), 356–360.
Sharifi, A., Ahmadi, M., & Ala, A. (2021). The impact of artificial intelligence and digital style on industry and energy post-COVID-19 pandemic. Environmental Science and Pollution Research, 28 (34), 46964–46984. https://doi.org/10.1007/s11356-021-15292-5
Slimi, Z. (2023). The impact of artificial intelligence on higher education: An empirical study. European Journal of Educational Sciences . https://doi.org/10.19044/ejes.v10no1a17
Sumakul, D. T. Y. G., Hamied, F. A., & Sukyadi, D. (2022). Students’ perceptions of the use of AI in a writing class. Advances in Social Science, Education and Humanities Research, 624 , 52–57. https://doi.org/10.2991/assehr.k.220201.009
Toncic, J. (2020). Teachers, AI grammar checkers, and the newest literacies: Emending writing pedagogy and assessment. Digital Culture & Education, 12 (1), 26–51.
Turner, E. (2021). Causes for leaving jobs: A comparative analysis. 2nd International Conference on Research in Management . https://doi.org/10.33422/2nd.icrmanagement.2021.02.43
Utami, S. P. T., Andayani, A., Winarni, R., & Sumarwati, S. (2023). Utilization of artificial intelligence technology in an academic writing class: How do Indonesian students perceive? Contemporary Educational Technology, 15 (4), ep450. https://doi.org/10.30935/cedtech/13419
Verma, M. (2018). Artificial intelligence and its scope in different areas with special reference to the field of education. International Journal of Advanced Educational Research , 3 (1), 5–10. http://files.eric.ed.gov/fulltext/ED604401.pdf
Victorivna, K. L., Oleksandrovych, V. A., Oleksandrivna, K. I., & Oleksandrivna, K. N. (2022). Artificial intelligence in language learning: What are we afraid of? Arab World English Journal, 8 , 262–273. https://doi.org/10.24093/awej/call8.18
Wang, T., Lund, B., Marengo, A., Pagano, A., Mannuru, N. R., Teel, Z. A., & Pange, J. (2023). Exploring the potential impact of artificial intelligence (AI) on international students in higher education: Generative AI, chatbots, analytics, and international student success. Applied Sciences, 13 (11), 6716. https://doi.org/10.3390/app13116716
Zhao, X. (2022). Leveraging artificial intelligence (AI) technology for English writing: Introducing Wordtune as a digital writing assistant for EFL writers. RELC Journal, 54 (3), 890–894. https://doi.org/10.1177/00336882221094089
Download references
Acknowledgements
The authors would like to thank Prince Sultan University for its support.
No funding was sought from any external source for this research.
Author information
Authors and affiliations.
Prince Sultan University, Riyadh, Saudi Arabia
Shaista Rashid
Department of English, Bahauddin Zakariya University, Multan, Pakistan
Sadia Malik & Faheem Abbas
Department of Statistics, Bahauddin Zakariya University, Multan, Pakistan
Javaria Ahmad Khan
You can also search for this author in PubMed Google Scholar
Corresponding author
Correspondence to Sadia Malik .
Ethics declarations
Conflict of interest.
There is no conflict of interest of any sort.
Ethical statement
All participants gave their informed consent for inclusion before they participated in the study. Their responses were evaluated anonymously as the data was presented in aggregate form.
Additional information
Publisher's note.
Springer Nature remains neutral with regard to jurisdictional claims in published maps and institutional affiliations.
Rights and permissions
Springer Nature or its licensor (e.g. a society or other partner) holds exclusive rights to this article under a publishing agreement with the author(s) or other rightsholder(s); author self-archiving of the accepted manuscript version of this article is solely governed by the terms of such publishing agreement and applicable law.
Reprints and permissions
About this article
Rashid, S., Malik, S., Abbas, F. et al. Pakistani students’ perceptions about knowledge, use and impact of artificial intelligence (AI) on academic writing: a case study. J. Comput. Educ. (2024). https://doi.org/10.1007/s40692-024-00338-7
Download citation
Received : 15 May 2024
Revised : 08 August 2024
Accepted : 14 August 2024
Published : 11 September 2024
DOI : https://doi.org/10.1007/s40692-024-00338-7
Share this article
Anyone you share the following link with will be able to read this content:
Sorry, a shareable link is not currently available for this article.
Provided by the Springer Nature SharedIt content-sharing initiative
- Artificial intelligence
- Academic writing
- Higher education
- Language pedagogy
- Find a journal
- Publish with us
- Track your research

An official website of the United States government
The .gov means it’s official. Federal government websites often end in .gov or .mil. Before sharing sensitive information, make sure you’re on a federal government site.
The site is secure. The https:// ensures that you are connecting to the official website and that any information you provide is encrypted and transmitted securely.
- Publications
- Account settings
Preview improvements coming to the PMC website in October 2024. Learn More or Try it out now .
- Advanced Search
- Journal List
- Elsevier - PMC COVID-19 Collection

The Effect of COVID-19 on Education
Jacob hoofman.
a Wayne State University School of Medicine, 540 East Canfield, Detroit, MI 48201, USA
Elizabeth Secord
b Department of Pediatrics, Wayne Pediatrics, School of Medicine, Pediatrics Wayne State University, 400 Mack Avenue, Detroit, MI 48201, USA
COVID-19 has changed education for learners of all ages. Preliminary data project educational losses at many levels and verify the increased anxiety and depression associated with the changes, but there are not yet data on long-term outcomes. Guidance from oversight organizations regarding the safety and efficacy of new delivery modalities for education have been quickly forged. It is no surprise that the socioeconomic gaps and gaps for special learners have widened. The medical profession and other professions that teach by incrementally graduated internships are also severely affected and have had to make drastic changes.
- • Virtual learning has become a norm during COVID-19.
- • Children requiring special learning services, those living in poverty, and those speaking English as a second language have lost more from the pandemic educational changes.
- • For children with attention deficit disorder and no comorbidities, virtual learning has sometimes been advantageous.
- • Math learning scores are more likely to be affected than language arts scores by pandemic changes.
- • School meals, access to friends, and organized activities have also been lost with the closing of in-person school.
The transition to an online education during the coronavirus disease 2019 (COVID-19) pandemic may bring about adverse educational changes and adverse health consequences for children and young adult learners in grade school, middle school, high school, college, and professional schools. The effects may differ by age, maturity, and socioeconomic class. At this time, we have few data on outcomes, but many oversight organizations have tried to establish guidelines, expressed concerns, and extrapolated from previous experiences.
General educational losses and disparities
Many researchers are examining how the new environment affects learners’ mental, physical, and social health to help compensate for any losses incurred by this pandemic and to better prepare for future pandemics. There is a paucity of data at this juncture, but some investigators have extrapolated from earlier school shutdowns owing to hurricanes and other natural disasters. 1
Inclement weather closures are estimated in some studies to lower middle school math grades by 0.013 to 0.039 standard deviations and natural disaster closures by up to 0.10 standard deviation decreases in overall achievement scores. 2 The data from inclement weather closures did show a more significant decrease for children dependent on school meals, but generally the data were not stratified by socioeconomic differences. 3 , 4 Math scores are impacted overall more negatively by school absences than English language scores for all school closures. 4 , 5
The Northwest Evaluation Association is a global nonprofit organization that provides research-based assessments and professional development for educators. A team of researchers at Stanford University evaluated Northwest Evaluation Association test scores for students in 17 states and the District of Columbia in the Fall of 2020 and estimated that the average student had lost one-third of a year to a full year's worth of learning in reading, and about three-quarters of a year to more than 1 year in math since schools closed in March 2020. 5
With school shifted from traditional attendance at a school building to attendance via the Internet, families have come under new stressors. It is increasingly clear that families depended on schools for much more than math and reading. Shelter, food, health care, and social well-being are all part of what children and adolescents, as well as their parents or guardians, depend on schools to provide. 5 , 6
Many families have been impacted negatively by the loss of wages, leading to food insecurity and housing insecurity; some of loss this is a consequence of the need for parents to be at home with young children who cannot attend in-person school. 6 There is evidence that this economic instability is leading to an increase in depression and anxiety. 7 In 1 survey, 34.71% of parents reported behavioral problems in their children that they attributed to the pandemic and virtual schooling. 8
Children have been infected with and affected by coronavirus. In the United States, 93,605 students tested positive for COVID-19, and it was reported that 42% were Hispanic/Latino, 32% were non-Hispanic White, and 17% were non-Hispanic Black, emphasizing a disproportionate effect for children of color. 9 COVID infection itself is not the only issue that affects children’s health during the pandemic. School-based health care and school-based meals are lost when school goes virtual and children of lower socioeconomic class are more severely affected by these losses. Although some districts were able to deliver school meals, school-based health care is a primary source of health care for many children and has left some chronic conditions unchecked during the pandemic. 10
Many families report that the stress of the pandemic has led to a poorer diet in children with an increase in the consumption of sweet and fried foods. 11 , 12 Shelter at home orders and online education have led to fewer exercise opportunities. Research carried out by Ammar and colleagues 12 found that daily sitting had increased from 5 to 8 hours a day and binge eating, snacking, and the number of meals were all significantly increased owing to lockdown conditions and stay-at-home initiatives. There is growing evidence in both animal and human models that diets high in sugar and fat can play a detrimental role in cognition and should be of increased concern in light of the pandemic. 13
The family stress elicited by the COVID-19 shutdown is a particular concern because of compiled evidence that adverse life experiences at an early age are associated with an increased likelihood of mental health issues as an adult. 14 There is early evidence that children ages 6 to 18 years of age experienced a significant increase in their expression of “clinginess, irritability, and fear” during the early pandemic school shutdowns. 15 These emotions associated with anxiety may have a negative impact on the family unit, which was already stressed owing to the pandemic.
Another major concern is the length of isolation many children have had to endure since the pandemic began and what effects it might have on their ability to socialize. The school, for many children, is the agent for forming their social connections as well as where early social development occurs. 16 Noting that academic performance is also declining the pandemic may be creating a snowball effect, setting back children without access to resources from which they may never recover, even into adulthood.
Predictions from data analysis of school absenteeism, summer breaks, and natural disaster occurrences are imperfect for the current situation, but all indications are that we should not expect all children and adolescents to be affected equally. 4 , 5 Although some children and adolescents will likely suffer no long-term consequences, COVID-19 is expected to widen the already existing educational gap from socioeconomic differences, and children with learning differences are expected to suffer more losses than neurotypical children. 4 , 5
Special education and the COVID-19 pandemic
Although COVID-19 has affected all levels of education reception and delivery, children with special needs have been more profoundly impacted. Children in the United States who have special needs have legal protection for appropriate education by the Individuals with Disabilities Education Act and Section 504 of the Rehabilitation Act of 1973. 17 , 18 Collectively, this legislation is meant to allow for appropriate accommodations, services, modifications, and specialized academic instruction to ensure that “every child receives a free appropriate public education . . . in the least restrictive environment.” 17
Children with autism usually have applied behavioral analysis (ABA) as part of their individualized educational plan. ABA therapists for autism use a technique of discrete trial training that shapes and rewards incremental changes toward new behaviors. 19 Discrete trial training involves breaking behaviors into small steps and repetition of rewards for small advances in the steps toward those behaviors. It is an intensive one-on-one therapy that puts a child and therapist in close contact for many hours at a time, often 20 to 40 hours a week. This therapy works best when initiated at a young age in children with autism and is often initiated in the home. 19
Because ABA workers were considered essential workers from the early days of the pandemic, organizations providing this service had the responsibility and the freedom to develop safety protocols for delivery of this necessary service and did so in conjunction with certifying boards. 20
Early in the pandemic, there were interruptions in ABA followed by virtual visits, and finally by in-home therapy with COVID-19 isolation precautions. 21 Although the efficacy of virtual visits for ABA therapy would empirically seem to be inferior, there are few outcomes data available. The balance of safety versus efficacy quite early turned to in-home services with interruptions owing to illness and decreased therapist availability owing to the pandemic. 21 An overarching concern for children with autism is the possible loss of a window of opportunity to intervene early. Families of children and adolescents with autism spectrum disorder report increased stress compared with families of children with other disabilities before the pandemic, and during the pandemic this burden has increased with the added responsibility of monitoring in-home schooling. 20
Early data on virtual schooling children with attention deficit disorder (ADD) and attention deficit with hyperactivity (ADHD) shows that adolescents with ADD/ADHD found the switch to virtual learning more anxiety producing and more challenging than their peers. 22 However, according to a study in Ireland, younger children with ADD/ADHD and no other neurologic or psychiatric diagnoses who were stable on medication tended to report less anxiety with at-home schooling and their parents and caregivers reported improved behavior during the pandemic. 23 An unexpected benefit of shelter in home versus shelter in place may be to identify these stressors in face-to-face school for children with ADD/ADHD. If children with ADD/ADHD had an additional diagnosis of autism or depression, they reported increased anxiety with the school shutdown. 23 , 24
Much of the available literature is anticipatory guidance for in-home schooling of children with disabilities rather than data about schooling during the pandemic. The American Academy of Pediatrics published guidance advising that, because 70% of students with ADHD have other conditions, such as learning differences, oppositional defiant disorder, or depression, they may have very different responses to in home schooling which are a result of the non-ADHD diagnosis, for example, refusal to attempt work for children with oppositional defiant disorder, severe anxiety for those with depression and or anxiety disorders, and anxiety and perseveration for children with autism. 25 Children and families already stressed with learning differences have had substantial challenges during the COVID-19 school closures.
High school, depression, and COVID-19
High schoolers have lost a great deal during this pandemic. What should have been a time of establishing more independence has been hampered by shelter-in-place recommendations. Graduations, proms, athletic events, college visits, and many other social and educational events have been altered or lost and cannot be recaptured.
Adolescents reported higher rates of depression and anxiety associated with the pandemic, and in 1 study 14.4% of teenagers report post-traumatic stress disorder, whereas 40.4% report having depression and anxiety. 26 In another survey adolescent boys reported a significant decrease in life satisfaction from 92% before COVID to 72% during lockdown conditions. For adolescent girls, the decrease in life satisfaction was from 81% before COVID to 62% during the pandemic, with the oldest teenage girls reporting the lowest life satisfaction values during COVID-19 restrictions. 27 During the school shutdown for COVID-19, 21% of boys and 27% of girls reported an increase in family arguments. 26 Combine all of these reports with decreasing access to mental health services owing to pandemic restrictions and it becomes a complicated matter for parents to address their children's mental health needs as well as their educational needs. 28
A study conducted in Norway measured aspects of socialization and mood changes in adolescents during the pandemic. The opportunity for prosocial action was rated on a scale of 1 (not at all) to 6 (very much) based on how well certain phrases applied to them, for example, “I comforted a friend yesterday,” “Yesterday I did my best to care for a friend,” and “Yesterday I sent a message to a friend.” They also ranked mood by rating items on a scale of 1 (not at all) to 5 (very well) as items reflected their mood. 29 They found that adolescents showed an overall decrease in empathic concern and opportunity for prosocial actions, as well as a decrease in mood ratings during the pandemic. 29
A survey of 24,155 residents of Michigan projected an escalation of suicide risk for lesbian, gay, bisexual, transgender youth as well as those youth questioning their sexual orientation (LGBTQ) associated with increased social isolation. There was also a 66% increase in domestic violence for LGBTQ youth during shelter in place. 30 LGBTQ youth are yet another example of those already at increased risk having disproportionate effects of the pandemic.
Increased social media use during COVID-19, along with traditional forms of education moving to digital platforms, has led to the majority of adolescents spending significantly more time in front of screens. Excessive screen time is well-known to be associated with poor sleep, sedentary habits, mental health problems, and physical health issues. 31 With decreased access to physical activity, especially in crowded inner-city areas, and increased dependence on screen time for schooling, it is more difficult to craft easy solutions to the screen time issue.
During these times, it is more important than ever for pediatricians to check in on the mental health of patients with queries about how school is going, how patients are keeping contact with peers, and how are they processing social issues related to violence. Queries to families about the need for assistance with food insecurity, housing insecurity, and access to mental health services are necessary during this time of public emergency.
Medical school and COVID-19
Although medical school is an adult schooling experience, it affects not only the medical profession and our junior colleagues, but, by extrapolation, all education that requires hands-on experience or interning, and has been included for those reasons.
In the new COVID-19 era, medical schools have been forced to make drastic and quick changes to multiple levels of their curriculum to ensure both student and patient safety during the pandemic. Students entering their clinical rotations have had the most drastic alteration to their experience.
COVID-19 has led to some of the same changes high schools and colleges have adopted, specifically, replacement of large in-person lectures with small group activities small group discussion and virtual lectures. 32 The transition to an online format for medical education has been rapid and impacted both students and faculty. 33 , 34 In a survey by Singh and colleagues, 33 of the 192 students reporting 43.9% found online lectures to be poorer than physical classrooms during the pandemic. In another report by Shahrvini and colleagues, 35 of 104 students surveyed, 74.5% students felt disconnected from their medical school and their peers and 43.3% felt that they were unprepared for their clerkships. Although there are no pre-COVID-19 data for comparison, it is expected that the COVID-19 changes will lead to increased insecurity and feelings of poor preparation for clinical work.
Gross anatomy is a well-established tradition within the medical school curriculum and one that is conducted almost entirely in person and in close quarters around a cadaver. Harmon and colleagues 36 surveyed 67 gross anatomy educators and found that 8% were still holding in-person sessions and 34 ± 43% transitioned to using cadaver images and dissecting videos that could be accessed through the Internet.
Many third- and fourth-year medical students have seen periods of cancellation for clinical rotations and supplementation with online learning, telemedicine, or virtual rounds owing to the COVID-19 pandemic. 37 A study from Shahrvini and colleagues 38 found that an unofficial document from Reddit (a widely used social network platform with a subgroup for medical students and residents) reported that 75% of medical schools had canceled clinical activities for third- and fourth-year students for some part of 2020. In another survey by Harries and colleagues, 39 of the 741 students who responded, 93.7% were not involved in clinical rotations with in-person patient contact. The reactions of students varied, with 75.8% admitting to agreeing with the decision, 34.7% feeling guilty, and 27.0% feeling relieved. 39 In the same survey, 74.7% of students felt that their medical education had been disrupted, 84.1% said they felt increased anxiety, and 83.4% would accept the risk of COVID-19 infection if they were able to return to the clinical setting. 39
Since the start of the pandemic, medical schools have had to find new and innovative ways to continue teaching and exposing students to clinical settings. The use of electronic conferencing services has been critical to continuing education. One approach has been to turn to online applications like Google Hangouts, which come at no cost and offer a wide variety of tools to form an integrative learning environment. 32 , 37 , 40 Schools have also adopted a hybrid model of teaching where lectures can be prerecorded then viewed by the student asynchronously on their own time followed by live virtual lectures where faculty can offer question-and-answer sessions related to the material. By offering this new format, students have been given more flexibility in terms of creating a schedule that suits their needs and may decrease stress. 37
Although these changes can be a hurdle to students and faculty, it might prove to be beneficial for the future of medical training in some ways. Telemedicine is a growing field, and the American Medical Association and other programs have endorsed its value. 41 Telemedicine visits can still be used to take a history, conduct a basic visual physical examination, and build rapport, as well as performing other aspects of the clinical examination during a pandemic, and will continue to be useful for patients unable to attend regular visits at remote locations. Learning effectively now how to communicate professionally and carry out telemedicine visits may better prepare students for a future where telemedicine is an expectation and allow students to learn the limitations as well as the advantages of this modality. 41
Pandemic changes have strongly impacted the process of college applications, medical school applications, and residency applications. 32 For US medical residencies, 72% of applicants will, if the pattern from 2016 to 2019 continues, move between states or countries. 42 This level of movement is increasingly dangerous given the spread of COVID-19 and the lack of currently accepted procedures to carry out such a mass migration safely. The same follows for medical schools and universities.
We need to accept and prepare for the fact that medial students as well as other learners who require in-person training may lack some skills when they enter their profession. These skills will have to be acquired during a later phase of training. We may have less skilled entry-level resident physicians and nurses in our hospitals and in other clinical professions as well.
The COVID-19 pandemic has affected and will continue to affect the delivery of knowledge and skills at all levels of education. Although many children and adult learners will likely compensate for this interruption of traditional educational services and adapt to new modalities, some will struggle. The widening of the gap for those whose families cannot absorb the teaching and supervision of education required for in-home education because they lack the time and skills necessary are not addressed currently. The gap for those already at a disadvantage because of socioeconomic class, language, and special needs are most severely affected by the COVID-19 pandemic school closures and will have the hardest time compensating. As pediatricians, it is critical that we continue to check in with our young patients about how they are coping and what assistance we can guide them toward in our communities.
Clinics care points
- • Learners and educators at all levels of education have been affected by COVID-19 restrictions with rapid adaptations to virtual learning platforms.
- • The impact of COVID-19 on learners is not evenly distributed and children of racial minorities, those who live in poverty, those requiring special education, and children who speak English as a second language are more negatively affected by the need for remote learning.
- • Math scores are more impacted than language arts scores by previous school closures and thus far by these shutdowns for COVID-19.
- • Anxiety and depression have increased in children and particularly in adolescents as a result of COVID-19 itself and as a consequence of school changes.
- • Pediatricians should regularly screen for unmet needs in their patients during the pandemic, such as food insecurity with the loss of school meals, an inability to adapt to remote learning and increased computer time, and heightened anxiety and depression as results of school changes.
The authors have nothing to disclose.

IMAGES
COMMENTS
Regarding the impact of the pandemic on major choice, students who report that COVID-19 impacted their major choice were more likely to be in lower-paying majors before the pandemic; mean pre-COVID major-specific annual earnings were $43,053 ($46,943) for students whose major choice was (not) impacted by COVID-19. 9 Impacted students were also ...
This work attempts to synthetize existing research about the impact of Covid-19 school closure on student achievement. It extends previous systematic reviews and meta-analyses by (a) using a more balanced sample in terms of country composition, (b) considering new moderators (type of data and research design), and (c) including studies on tertiary education students in addition to primary and ...
variation in the e ects of COVID-19 across students. In terms of labor market expectations, on average, students foresee a 13 percentage points decrease in. the probability of. on, a reduction of 2 percent in their reservation wages, a. d a2.3 percent decrease in their expected earn. ID-19 demonstrate that stude.
This study aims to investigate the perceived impact of COVID-19 on student learning and academic performance through a qualitative approach. ... The themes were deductively derived from the key research questions but inductively from the qualitative data organized and analyzed thematically using data from interviews and secondary sources ...
The use of a quantitative methodology was advocated to answer the research questions posed, and to satisfy the ... This could be due to the notorious economic impact that students claim that COVID-19 has triggered ... (Spain), in the competitive call for research projects on SARS-CoV-2 and COVID-19 disease, co-financed with European FEDER ...
This approach allowed participants to provide substantial insights by responding to open-ended questions on the research topic. The interviews explored the impact of COVID-19 on students ...
This study incorporated comprehensive literature reviews to explore COVID-19's impact on students' academic performance and mental health. To find relevant articles, we used the Scopus database to search the pieces through the online libraries of the North East University Bangladesh, Utah State University, USA, and Shahjalal University of Science and Technology, Bangladesh.
The COVID-19 pandemic had radically changed higher education. The sudden transition to online teaching and learning exposed, however, some benefits by enhancing educational flexibility and digitization. The long-term effects of these changes are currently unknown, but a key question concerns their effect on student learning outcomes. This study aims to analyze the impact of the emergence of ...
An Analysis of COVID-19 Student Learning Loss (World Bank, 2022). Zierer, K. Effects of pandemic-related school closures on pupils' performance and learning in selected countries: a rapid review ...
Due to COVID-19: 13% of students have delayed graduation, 40% lost a job, internship, or a job offer, and 29% expect to earn less at age 35. Moreover, these effects have been highly heterogeneous. One quarter of students increased their study time by more than 4 hours per week due to COVID-19, while another quarter decreased their study time by ...
The present research aimed to analyse students' attainment during the COVID-19 pandemic by using student performance data. We focused on the relationship between participation in online courses ...
The rapid and unexpected onset of the COVID-19 global pandemic has generated a great degree of uncertainty about the future of education and has required teachers and students alike to adapt to a new normal to survive in the new educational ecology. Through this experience of the new educational ecology, educators have learned many lessons, including how to navigate through uncertainty by ...
The survey included both close-ended and open-ended questions assessing the impact of COVID-19. Results: The students of color reported disruptive changes in finances (54%), living situation (35% ...
As we outline in our new research study released in January, the cumulative impact of the COVID-19 pandemic on students' academic achievement has been large. We tracked changes in math and ...
Understanding these impacts and how best to support students' social and emotional needs after the huge disruption of COVID-19 will be essential. Many students may face greater food insecurity ...
The research team reviewed data from 8,000 communities in 40 states and Washington, D.C., including 2022 NAEP scores and Spring 2022 assessments, COVID death rates, voting rates, and trust in government, patterns of social activity, and survey data from Facebook/Meta on family activities and mental health during the pandemic.
Sarah Schwartz is a reporter for Education Week who covers curriculum and instruction. A version of this article appeared in the December 14, 2022 edition of Education Week as COVID Hurt Student ...
Canadian research on COVID-19 and its impact on mental health and other outcomes is only now starting to be released. For example, Hawke et al. (2020) ... Students were asked to respond to questions related to their education following March 2020 school closures. This included asking about which support services they had received (e.g., family ...
Grit measures and school performance: pre- and post-Covid. The "behavioral grit" measure we construct is based on the student's response to a performance that deviates in a negative way from ...
For Black students, the number spikes to 25 percent. "There are many reasons to believe the Covid-19 impacts might be larger for children in poverty and children of color," Kuhfeld wrote in the study. Their families suffer higher rates of infection, and the economic burden disproportionately falls on Black and Hispanic parents, who are less ...
The COVID-19 pandemic has provided us with an opportunity to pave the way for introducing digital learning. This article aims to provide a comprehensive report on the impact of the COVID-19 pandemic on online teaching and learning of various papers and indicate the way forward.
University students have been one of the most affected groups worldwide during the COVID-19 pandemic, having experienced increased stress, anxiety, and depression. Resilience is reported as the most predictive factor in decreasing the impact of the threats of the COVID-19 pandemic. However, university students' resilience during the COVID-19 pandemic has not received much attention; this is ...
The Impact of COVID-19 on the Careers of Women in Academic Sciences, Engineering, and Medicine will inform the academic community as it emerges from the pandemic to mitigate any long-term negative consequences for the continued advancement of women in the academic STEMM workforce and build on the adaptations and opportunities that have emerged.
Students were also asked about the impact of COVID-19 on depressive and suicidal thoughts. These constructs were derived from existing literature identifying prominent factors affecting college students' mental health [20,21]. Feedback on the severity of COVID-19's impact on these aspects were elicited using a 4-point scale: 0 (none), 1 ...
Integrating artificial intelligence (AI) in language pedagogy can help learn and develop many skills. In this context, this study explores Pakistani students' perceptions and trends regarding the knowledge, use, and impact of AI on their academic writing. The data was collected using a quantitative method, using a questionnaire through cluster sampling of four faculties and random sampling of ...
The reactions of students varied, with 75.8% admitting to agreeing with the decision, 34.7% feeling guilty, and 27.0% feeling relieved. 39 In the same survey, 74.7% of students felt that their medical education had been disrupted, 84.1% said they felt increased anxiety, and 83.4% would accept the risk of COVID-19 infection if they were able to ...