Reference management. Clean and simple.

Journal abbreviation: Biomolecular NMR assignments
The abbreviation of the journal title " Biomolecular NMR assignments " is " Biomol. NMR Assign. ". It is the recommended abbreviation to be used for abstracting, indexing and referencing purposes and meets all criteria of the ISO 4 standard for abbreviating names of scientific journals.
Abbreviation rules
The table below outlines in detail the ISO 4 rules and matches to the ISSN maintained list of title word abbreviations ( TWA ) to derive the abbreviation.
Title | Abbreviation | Word/Stem/Rule |
---|---|---|
Biomolecular | Biomol. | matches the stem "biomolecul-" |
NMR | NMR | Rule 4.4: Acronyms shall be retained in the abbreviated title. |
assignments | Assign. | matches the word "assignment" |
Abbreviation systems
Developed by the International Organization for Standardization, the ISO 4 abbreviation system provides a standardized way of abbreviating journal titles. This consistency enables researchers to efficiently identify and cite journals in their work.
Journal title abbreviations come in three primary forms: ISO (International Organization for Standardization) for general disciplines, NLM (National Library of Medicine) for biomedical and life sciences, and CASSI (CAS Source Index) for chemistry and associated fields.
About the journal
Full journal title | Biomolecular NMR assignments |
---|---|
Abbreviation | Biomol. NMR Assign. |
ISSN (print) | |
ISSN (online) | |
NLM catalog |
Other journal abbreviations
- Foundry trade journal
- Experimental mycology
- Ekonomska misao
A Step-By-Step Guide to 1D and 2D NMR Interpretation
Nuclear Magnetic Resonance (NMR) spectroscopy is an incredibly powerful tool for characterizing molecular structures. When submitting to the FDA or other regulatory agencies, full structural characterization by NMR provides crucial evidence of compound identity. A combination of 1-dimensional and 2-dimensional NMR experiments are necessary for complete confidence in chemical structure. This post will walk you through the steps to fully characterize a molecule by 1- and 2-dimensional NMR, including on how to perform NMR interpretation.
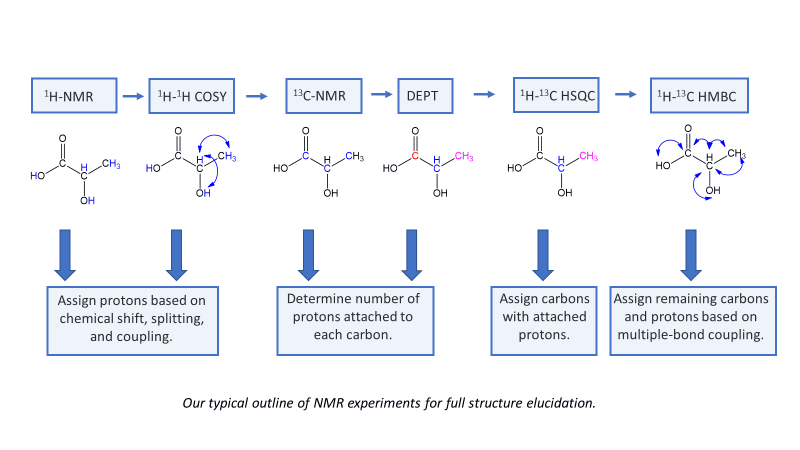
Step 1: ¹H-NMR
The first step in structural characterization is 1-dimensional proton ¹H-NMR. The chemical shift, multiplicity, coupling constants, and integration are all factors to consider when assigning protons. In this example, only three protons can be assigned by the proton spectrum alone: protons 3, 4, and 6.
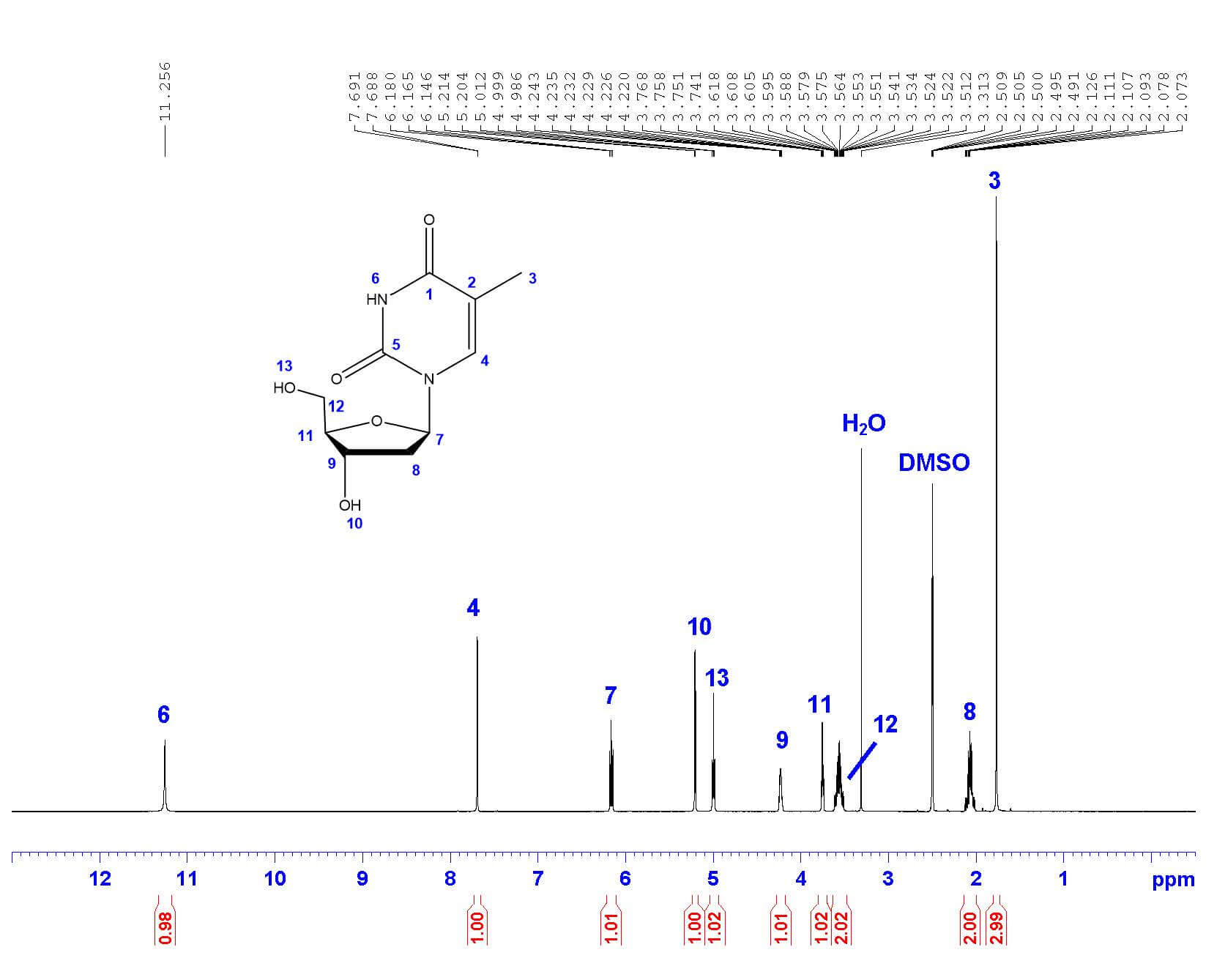
11.256 | s | - | 1H |
7.690 | q | 1.2 | 1H |
6.163 | t | 6.8 | 1H |
5.209 | d | 4.0 | 1H |
4.999 | t | 5.2 | 1H |
4.233 | m | - | 1H |
3.754 | q | 3.7 | 1H |
3.564 | m | - | 2H |
2.068 | m | - | 2H |
1.770 | d | 1.2 | 3H |
To begin, let’s start with proton 3 . Proton 3 is the only methyl group in the structure, and therefore must integrate to 3 protons. The only peak with an integration of 3 is the doublet at 1.770 ppm. The high field chemical shift supports this assignment. The peak is split into a doublet with a coupling constant of 1.2 Hz, reflecting the long-range coupling between protons 3 and 4, which also supports this assignment.
Protons that are coupled to each other should exhibit the same coupling constant. The long-range coupling constant observed for proton 3 (J=1.2 Hz, split into a doublet by proton 4) is reflected in the coupling constant for proton 4 (J=1.2 Hz, split into a quartet by proton 3). Therefore, the peak at 7.690 ppm must represent proton 4 ! The integration and chemical shift support the assignment, as proton 4 is the only aromatic proton in the structure.
There is only one singlet in the ¹H-NMR spectrum. The only proton that should show up as a singlet is proton 6 , as it has no neighboring protons that would split the peak (the nearest proton is 5 bonds away!). The chemical shift of 11.256 ppm supports this assignment, as imide protons often show up far downfield. The peak also integrates to 1 proton, supporting the assignment.
The remaining protons are doublets, triplets, and multiplets that can be assigned by 2-dimensional COSY.
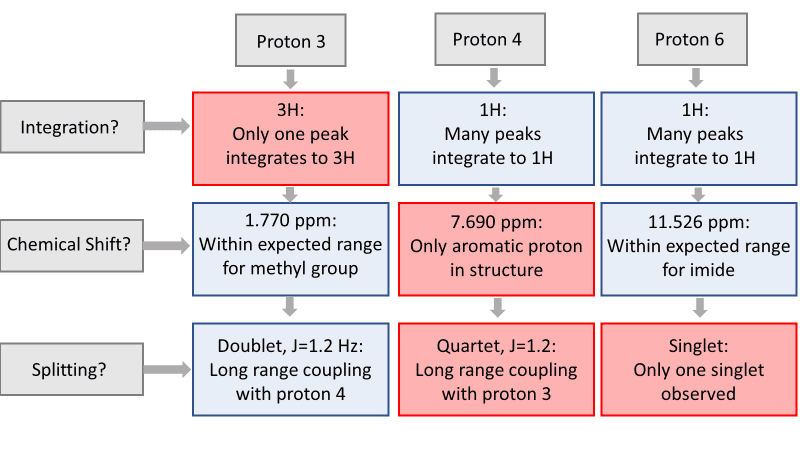
Step 2: ¹H-¹H COSY
¹H-¹H Correlation Spectroscopy (COSY) shows the correlation between hydrogens which are coupled to each other in the ¹H NMR spectrum. The ¹H spectrum is plotted on both 2D axes. While 2-bond and 3-bond ¹H-¹H coupling is easily visible by COSY, long range coupling can also be observed with long acquisition times. The cross-peaks (not on the diagonal) that are symmetric to the diagonal show the COSY correlations. For example, protons 3 and 4 are coupled to each other, since they form a box pattern symmetric to the diagonal. This confirms assignments 3 and 4 made from the proton spectrum alone.
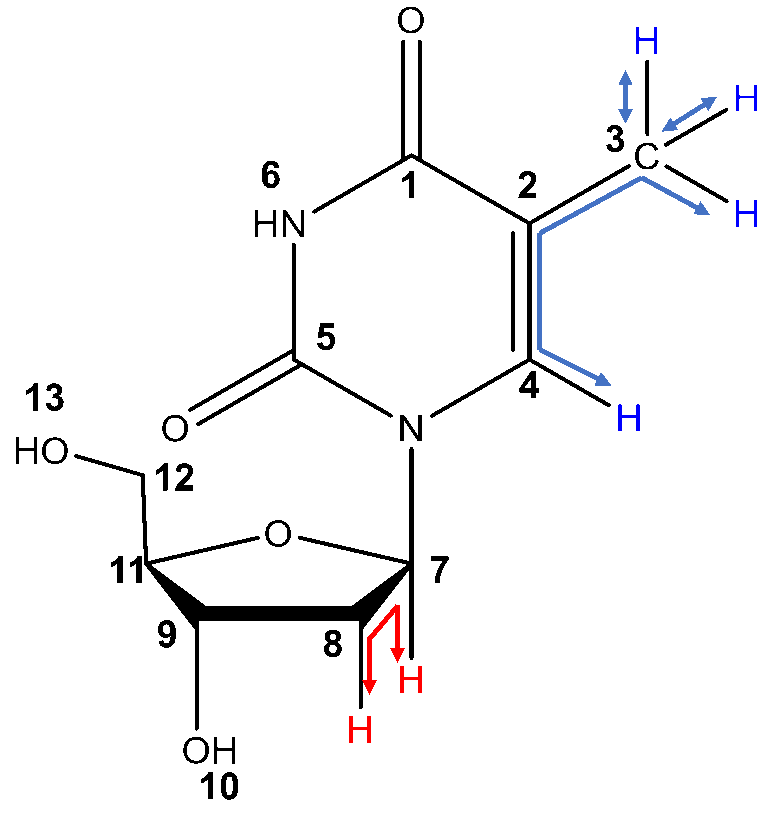
Two types of COSY coupling: 3-bond short range coupling between protons 7 and 8 (red) and 4-bond long range coupling between protons 3 and 4 (blue).
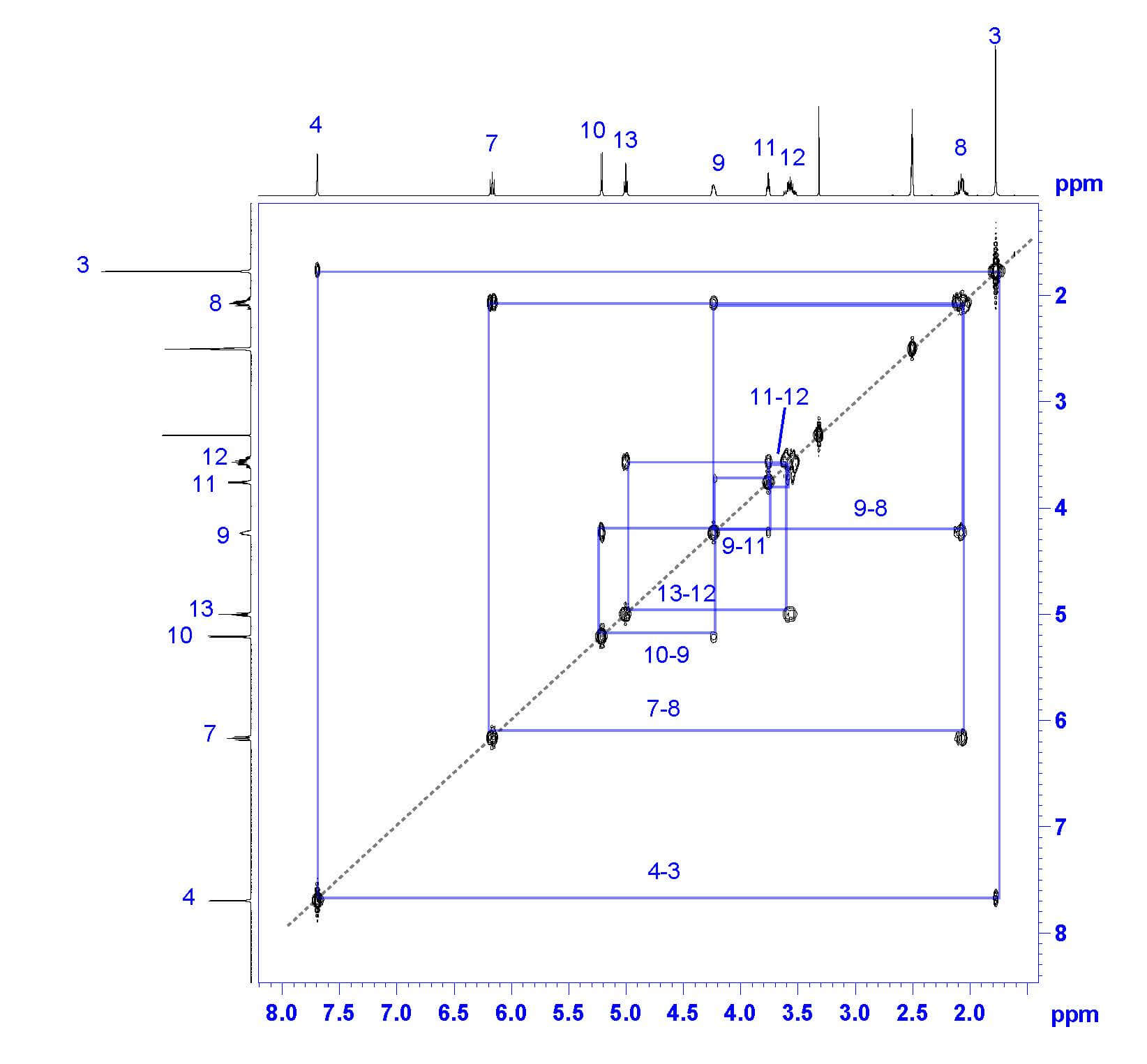
My favorite way to analyze a COSY spectrum with many unassigned protons is to make a table of correlations, like the one seen here. Look at the table for any clear differences in correlation and begin there! In this example, all unassigned protons show one or two COSY correlations-except the proton at 4.233 ppm, which correlates to three other protons by COSY. The only proton expected to correlate with three nonequivalent protons is proton 9 !
11.256 | none | 6 |
7.690 | 4-3 | 4 |
6.163 | one | ? |
5.209 | one | ? |
4.999 | one | ? |
4.233 | three | ? |
3.754 | two | ? |
3.564 | two | ? |
2.068 | two | ? |
1.770 | 3-4 | 3 |
Now that proton 9 has been assigned, the fun really begins. Thymidine’s structure suggests that proton 9 should couple protons 8, 10, and 11. Based on the COSY, proton 9 couples protons at 2.068 ppm (2H), 3.754 ppm (1H), and 5.209 ppm (1H). From this list, we can easily assign proton 8 as the peak at 2.068 ppm based on its integration of 2 protons. To differentiate protons 10 and 11, take a look at our COSY table; 3.754 ppm shows two COSY correlations, while 5.209 ppm only shows one. Therefore, we can assign proton 10 as 5.209 ppm and proton 11 as 3.754 ppm.
Once proton 8 has been assigned, we can easily assign proton 7 based on the remaining COSY correlation for proton 8. Proton 7’s peak at 6.163 ppm is split into a triplet by the two 8 protons, confirming the assignment.
All that remains are protons 12 and 13. We can assign proton 12 (3.564 ppm) based on its integration of 2H and its COSY correlation to proton 11. The last remaining peak at 4.999 ppm must be proton 13 ; this is confirmed by COSY correlation with proton 12, triplet multiplicity based on splitting by proton 12, and integration of one proton.
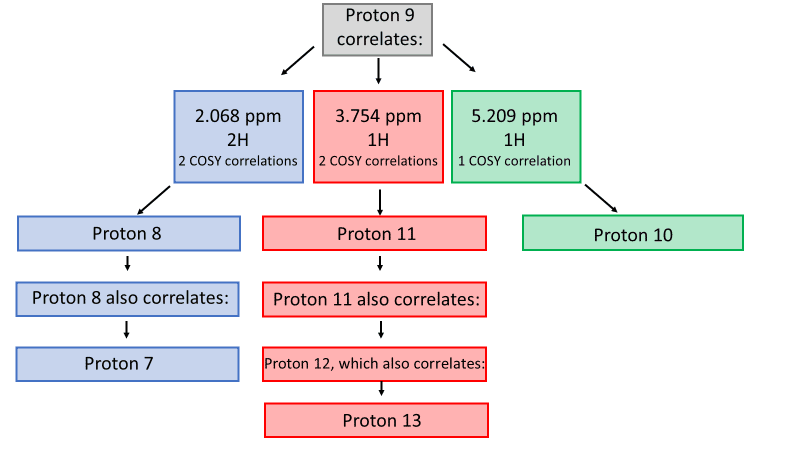
Now we have a fully assigned ¹H-NMR spectrum! This spectrum will help us assign our carbons using HSQC and HMBC NMR spectroscopy.
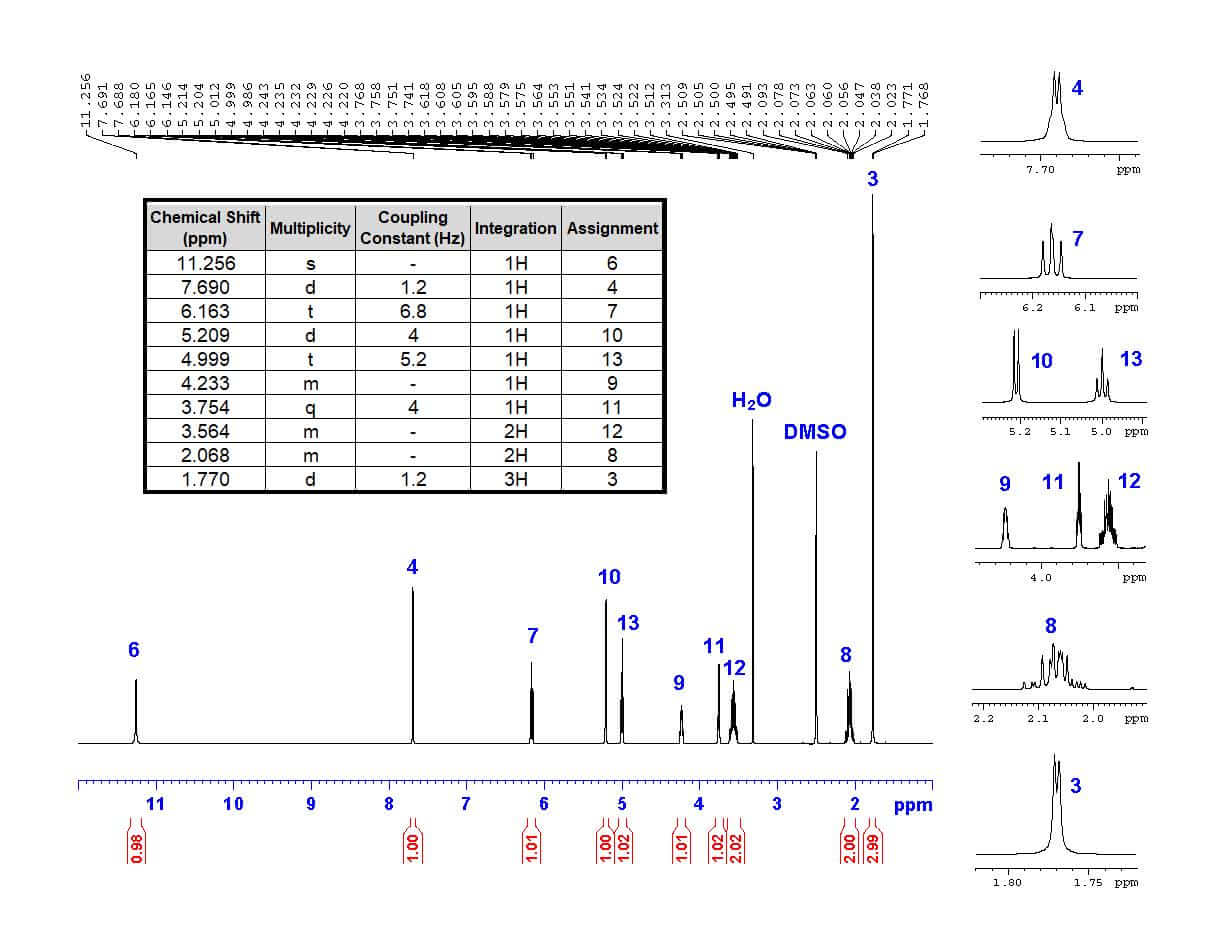
Step 3: ¹³C-NMR
Carbon NMR is a necessary step in full structural characterization. However, ¹³C-NMR alone does not provide enough information to assign the carbons in the molecule. The NMR spectrum below does confirm the number of carbons in the molecule; however, HSQC and HMBC (we will get to these soon!) are necessary to assign the carbons with confidence. Note that one of the carbons is hidden beneath the solvent signal but is clearly visible after zooming into that region.
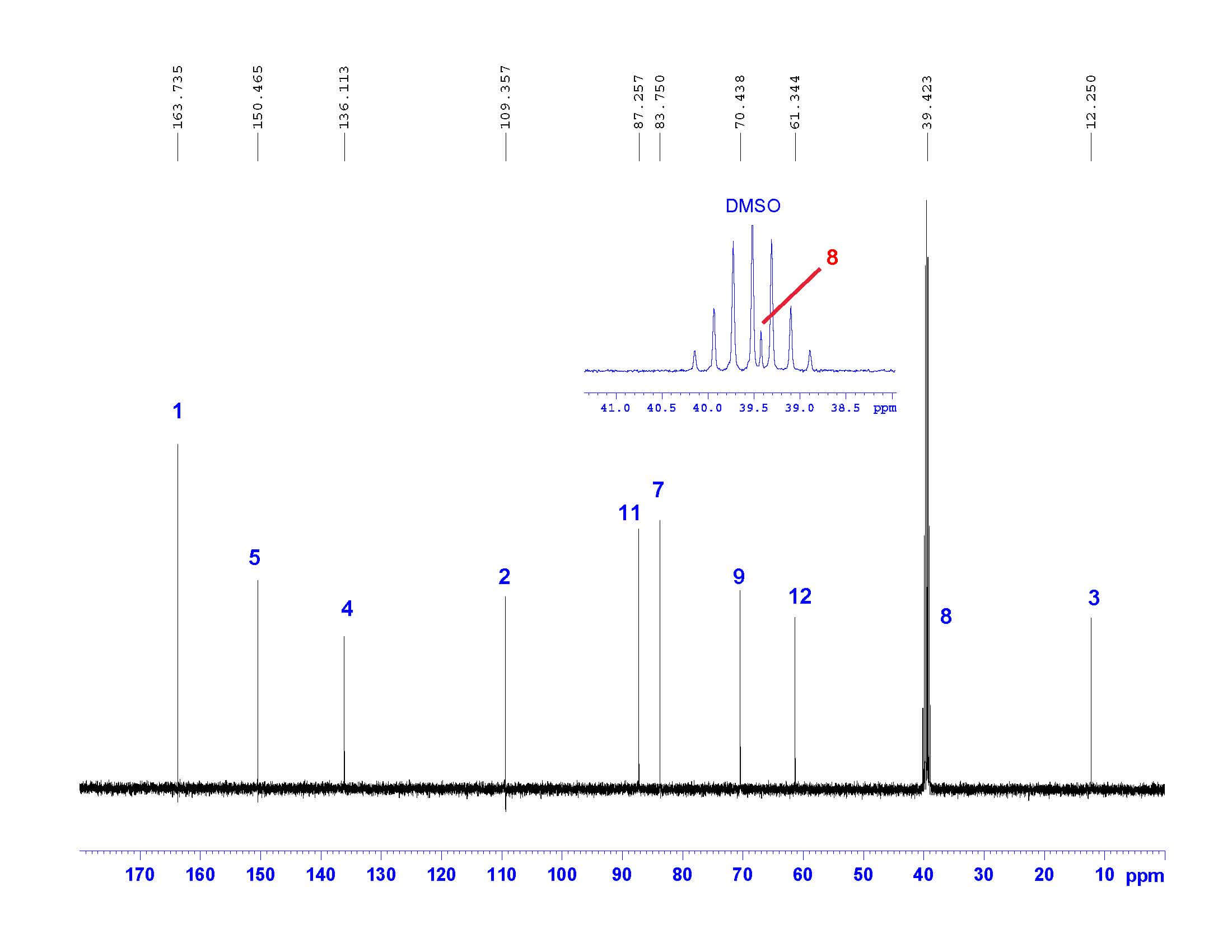
Step 4: DEPT-45, 90, and 135
Distortionless Enhancement of Polarization Transfer (DEPT) experiments help assign carbon peaks by determining the number of protons attached to each carbon. For very simple molecules, DEPT may be enough to partially or fully assign all carbons. In complex molecules, DEPT and HSQC together are useful for confirming both carbon and proton assignments. For example, the DEPT experiments below can only identify carbon 3 -it is the only CH₃ peak. I always go back and use DEPT to confirm the carbons I assigned by HSQC.
- DEPT-45 shows CH, CH₂, and CH₃ carbons as positive peaks. Carbons with no protons are not visible.
- DEPT-90 shows only CH peaks as positive peaks. Carbons with no protons, CH₂, and CH₃ carbons are not visible.
- DEPT-135 shows CH and CH₃ carbons as positive peaks and CH₂ carbons as negative peaks. Carbons with no protons are not visible.
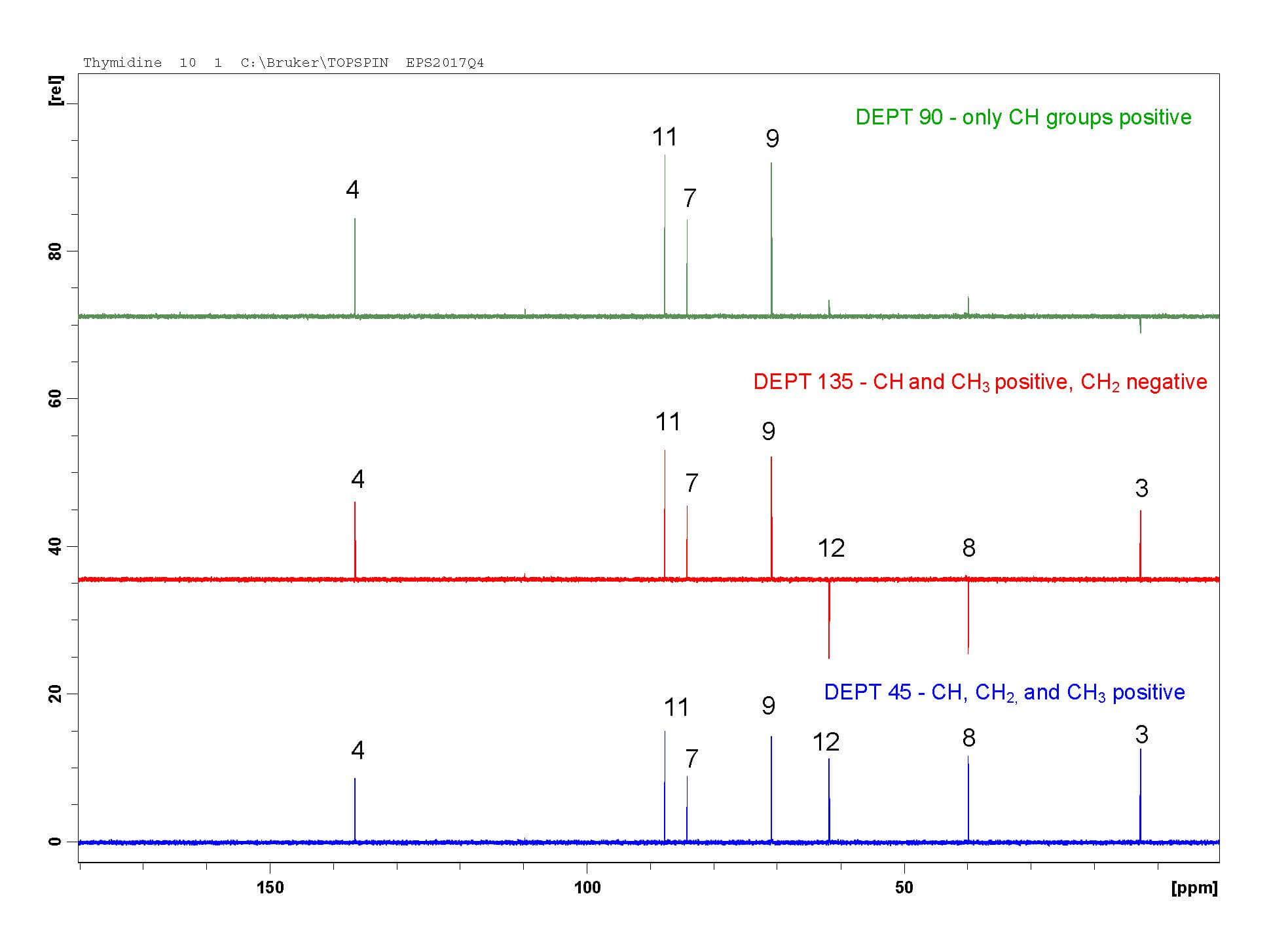
Step 5: ¹H-¹³C HSQC
¹H-¹³C Heteronuclear Single Quantum Coherence Spectroscopy (HSQC) shows which hydrogens are directly attached to which carbon atoms. The ¹H spectrum is shown on the horizontal axis and the ¹³C spectrum is shown on the vertical axis. The HSQC spectrum is most valuable when protons have already been assigned.
For example, HSQC shows a correlation between proton 4 and the carbon at 136.113 ppm; this carbon is now assigned as carbon 4. Carbons 3, 4, 7, 8, 9, 11, and 12 are assigned by HSQC. Only 1-bond correlations are observed, so HSQC assignments are relatively straightforward. The DEPT experiments also confirm these assignments. HSQC is also useful in confirming proton assignments of nitrogen or oxygen-bound protons; they show no signal by HSQC. This further supports the assignments of protons 6, 10, and 13.
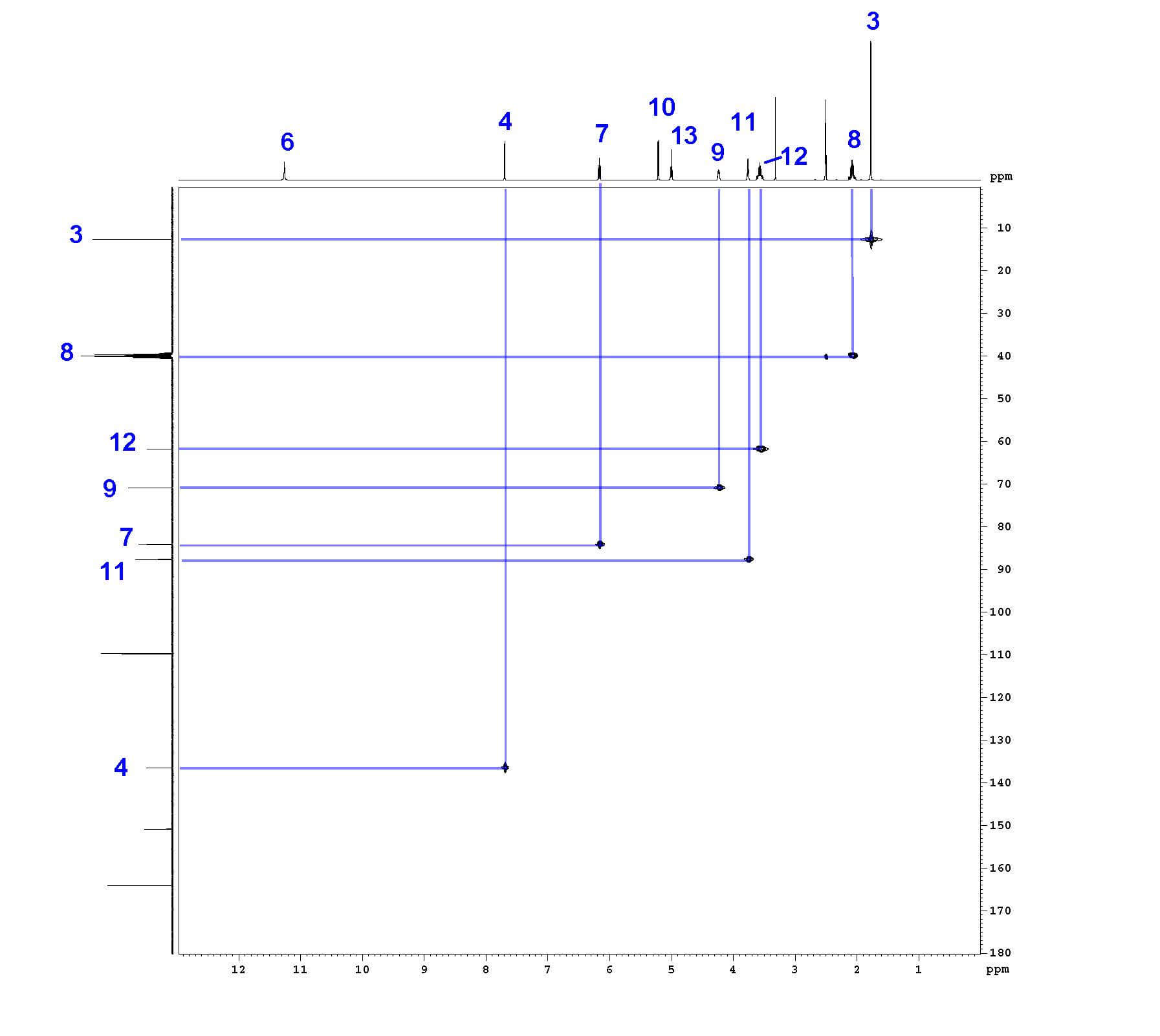
An example correlation between proton and carbon 4 is observed by HSQC.
Step 6: ¹H-¹³C HMBC
¹H-¹³C Heteronuclear Multiple Bond Correlation Spectroscopy (HMBC) shows the correlations between protons and carbons that are separated by multiple bonds. The ¹H spectrum is shown on the horizontal axis and the ¹³C spectrum is shown on the vertical axis. Correlated atoms are shown in blue and the connecting atoms are shown in red. Note that direct hydrogen-carbon bonds (1-bond correlations) are generally not seen. For example, hydrogen 4 shows correlations with carbons 1, 2, 3, 5, and 7, but not carbon 4.
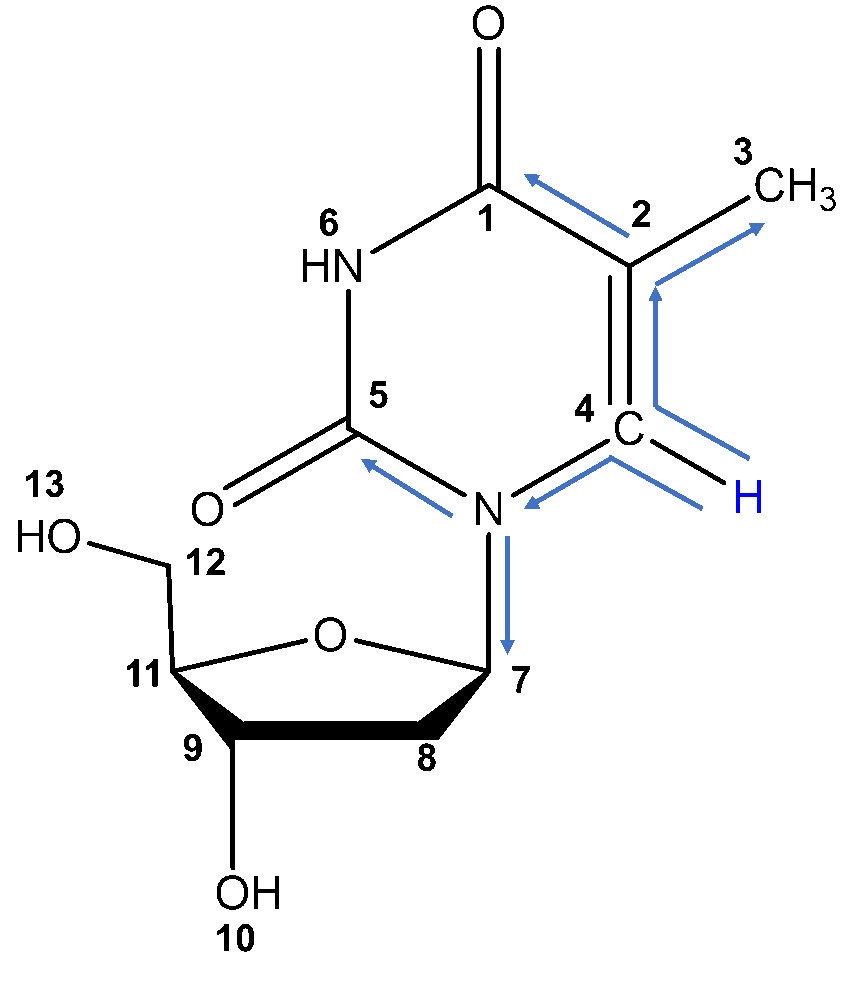
HMBC interactions between proton 4 and carbons 1, 2, 3, 5, and 7.
HMBC is incredibly useful for assigning carbons that have no protons attached. In this example, carbons 1, 2, and 5 have no protons attached. Carbon 1 is assigned by HMBC interactions with protons 3, 4, and 6; carbon 2 by interaction with protons 3, 4, 6, and 7; and carbon 5 by interactions with protons 4 and 7 only. The chemical environment of carbon 5 suggests it would appear more downfield than carbon 1, which confirms these assignments.
HMBC | Proton | |||
Carbon | 3 | 4 | 6 | 7 |
1 | x | x | x | |
2 | x | x | x | x |
5 | x | x |
HMBC also confirms assignments that were based solely on the proton and COSY spectrum. For example, protons 10 and 13 are differentiated by HMBC; proton 10 is confirmed by interactions with carbons 8, 9, and 11 , while proton 13 is confirmed by interactions with 11 and 12 . HMBC supports all proton and all carbon assignments, unambiguously confirming both the structure and analysis of thymidine.
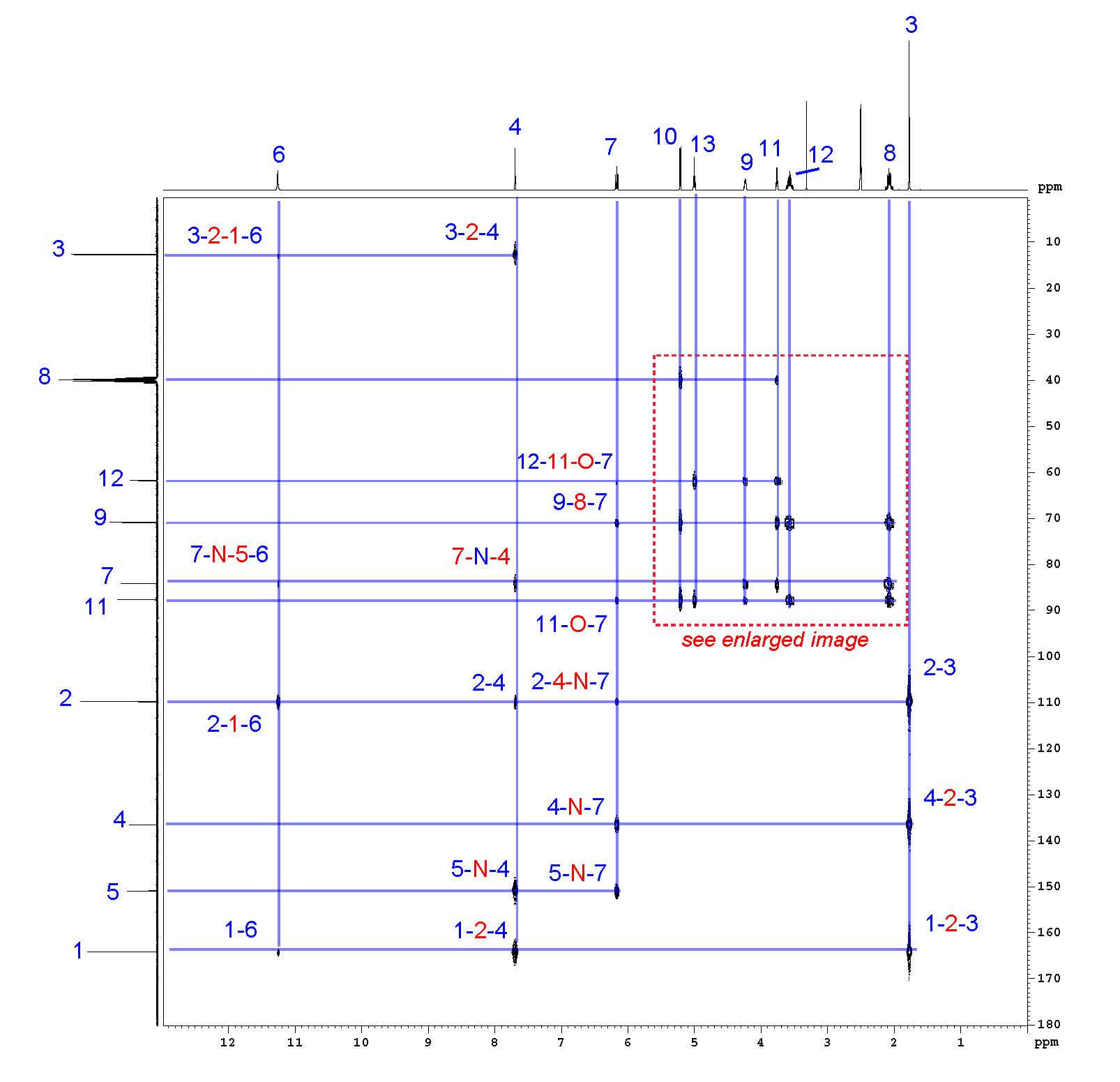
At Emery Pharma, we are experts in 1D and 2D NMR characterization and structure elucidation; in fact, 2D NMR projects are some of our favorites! We have supported numerous pharmaceutical companies in full NMR characterization for API submissions to regulatory agencies, as well as complete structure elucidation of impurities. We provide a fully annotated report with images similar to those seen here and support our results with high resolution mass spectrometry and elemental analysis. For more information on our NMR services, including GLP/cGMP or R&D projects, please visit our NMR Services page , or contact us at [email protected] .
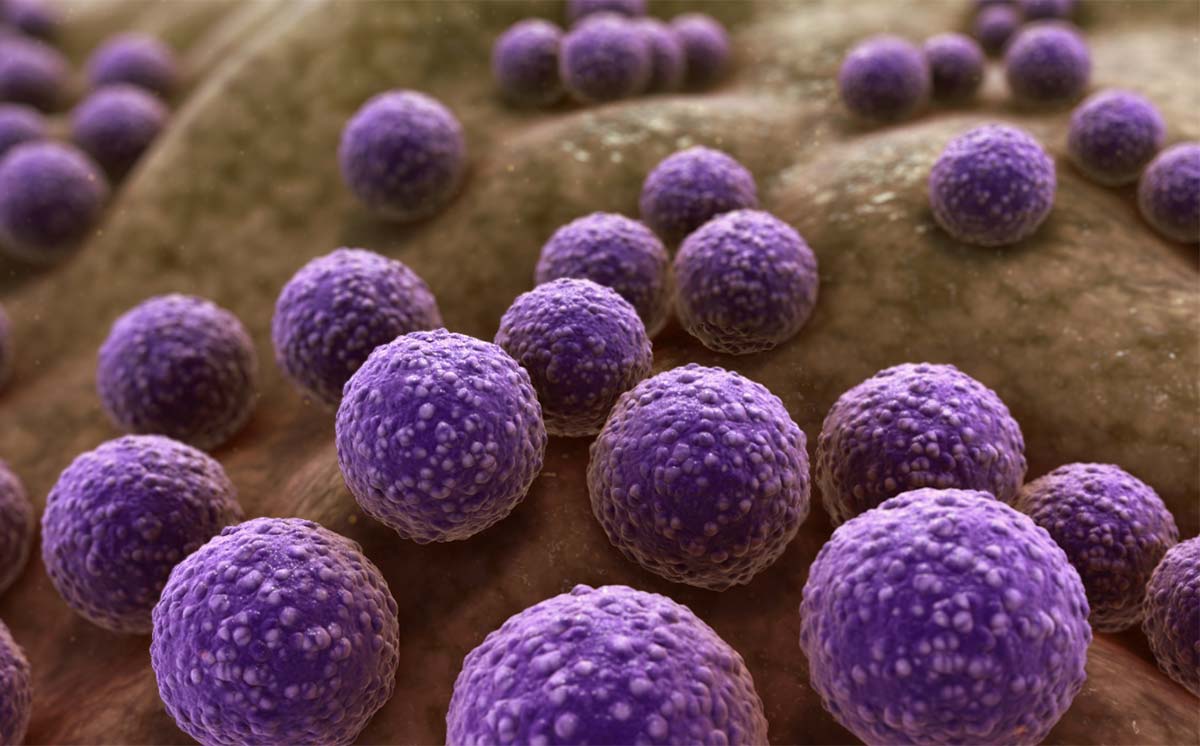
Emery Pharma
Request for proposal, let us be a part of your success story..
Do you have questions regarding a potential project? Or would you like to learn more about our services? Please reach out to a member of the Emery Pharma team via the contact form, and one of our experts will be in touch soon as possible. We look forward to working with you!
Protein NMR
A practical guide, introduction.
Most books on Protein NMR focus on theoretical aspects and pulse sequences with only little space devoted to resonance assignment and structure calculations. At the same time many software manuals provide detailed information on how to use the software, but assume prior knowledge of the concepts of assignment and structure calculation. This has produced a gap in this area which these webpages aim to bridge by describing the concepts of assignment in detail with the help of many illustrations. Much space and discussion is devoted to practical aspects.
The implementation of protein NMR assignment is described using the program CCPNmr Analysis . This program has been developed by CCPN and actively seeks input from the NMR community. CCPNmr Analysis is based on the detailed and well thought-out CCPN Data Model which has the advantage (a) that it feeds directly into the CCPN Format Converter thus simplifying the import from and export to other programs, and (b) that as more and more NMR-related programs adopt the CCPN Data Model it is likely to take on a key role in NMR data management – in a similar way to CCP4 for protein X-ray crystallography. CCPNmr Analysis is already one of the best assignment programs available while still being developed and provides excellent support via the CCPN Mailing List (a manual is also available). (Although I now work for the CCPN group, these webpages and my recommendation to use this program far predate this!)
Webpages include:
- description of several resonance assignment strategies
- simple descriptions and discussions of many multidimensional NMR experiments commonly used in protein NMR
- basic usage of CCPNmr Analysis (versions 1 and 2 )
- how to make publication quality figures using CCPNmr Analysis
- advice for using CCPNmr Analysis with solid-state MAS NMR data
- tutorial on protein assignment using solid-state MAS NMR data
- description of isotopic labelling strategies commonly used in protein NMR
- links to a large number of protein NMR software packages
- suggested literature for further reading
- links to other useful NMR webpages
Thank you for visiting nature.com. You are using a browser version with limited support for CSS. To obtain the best experience, we recommend you use a more up to date browser (or turn off compatibility mode in Internet Explorer). In the meantime, to ensure continued support, we are displaying the site without styles and JavaScript.
- View all journals
- Explore content
- About the journal
- Publish with us
- Sign up for alerts
- Open access
- Published: 18 October 2022
Rapid protein assignments and structures from raw NMR spectra with the deep learning technique ARTINA
- Piotr Klukowski ORCID: orcid.org/0000-0003-1045-3487 1 ,
- Roland Riek ORCID: orcid.org/0000-0002-6333-066X 1 &
- Peter Güntert ORCID: orcid.org/0000-0002-2911-7574 1 , 2 , 3
Nature Communications volume 13 , Article number: 6151 ( 2022 ) Cite this article
13k Accesses
34 Citations
29 Altmetric
Metrics details
- Machine learning
- Solution-state NMR
Nuclear Magnetic Resonance (NMR) spectroscopy is a major technique in structural biology with over 11,800 protein structures deposited in the Protein Data Bank. NMR can elucidate structures and dynamics of small and medium size proteins in solution, living cells, and solids, but has been limited by the tedious data analysis process. It typically requires weeks or months of manual work of a trained expert to turn NMR measurements into a protein structure. Automation of this process is an open problem, formulated in the field over 30 years ago. We present a solution to this challenge that enables the completely automated analysis of protein NMR data within hours after completing the measurements. Using only NMR spectra and the protein sequence as input, our machine learning-based method, ARTINA, delivers signal positions, resonance assignments, and structures strictly without human intervention. Tested on a 100-protein benchmark comprising 1329 multidimensional NMR spectra, ARTINA demonstrated its ability to solve structures with 1.44 Å median RMSD to the PDB reference and to identify 91.36% correct NMR resonance assignments. ARTINA can be used by non-experts, reducing the effort for a protein assignment or structure determination by NMR essentially to the preparation of the sample and the spectra measurements.
Similar content being viewed by others
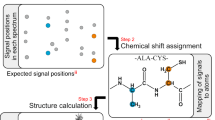
The 100-protein NMR spectra dataset: A resource for biomolecular NMR data analysis
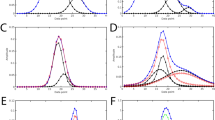
DEEP picker is a deep neural network for accurate deconvolution of complex two-dimensional NMR spectra
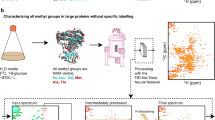
Solution-state methyl NMR spectroscopy of large non-deuterated proteins enabled by deep neural networks
Introduction.
Studying structures of proteins and ligand-protein complexes is one of the most influential endeavors in molecular biology and rational drug design. All key structure determination techniques, X-ray crystallography, electron microscopy, and NMR spectroscopy, have led to remarkable discoveries, but suffer from their respective experimental limitations. NMR can elucidate structures and dynamics of small and medium size proteins in solution 1 and even in living cells 2 . However, the analysis of NMR spectra and the resonance assignment, which are indispensable for NMR studies, remain time-consuming even for a skilled and experienced spectroscopist. Attributed to this, the percentage of NMR protein structures in the Protein Data Bank (PDB) has decreased from a maximum of 14.6% in 2007 to 7.3% in 2021 ( https://www.rcsb.org/stats ). The problem has sparked research towards automating different tasks in NMR structure determination 3 , 4 , including peak picking 5 , 6 , 7 , 8 , 9 , resonance assignment 10 , 11 , 12 , and the identification of distance restraints 13 , 14 . Several of these methods are available as webservers 15 , 16 . This enabled semi-automatic 17 , 18 but not yet unsupervised automation of the entire NMR structure determination process, except for a very small number of favorable proteins 7 , 19 .
The advance of machine learning techniques 20 now offers unprecedented possibilities for reliably replacing decisions of human experts by efficient computational tools. Here, we present a method that achieves this goal for NMR assignment and structure determination. We show for a diverse set of 100 proteins that NMR resonance assignments and protein structures can be determined within hours after completing the NMR measurements. Our method, Art ificial I ntelligence for N MR A pplications, ARTINA (Fig. 1 ), combines machine learning for tasks that are difficult to model otherwise with existing algorithms—evolutionary optimization for resonance assignment with FLYA 12 , chemical shift database searches for torsion angle restraint generation with TALOS-N 21 , ambiguous distance restraints, network-anchoring and constraint combination for NOESY assignment 14 , 22 and simulated annealing by torsion angle dynamics for structure calculation with CYANA 23 . Machine learning is used in multiple flavors—deep residual neural networks 24 for visual spectrum analysis to identify peak positions (pp-ResNet) and to deconvolve overlapping signals (deconv-ResNet) in 25 different types of spectra (Supplementary Table 1 ), kernel density estimation (KDE) to reconstruct original peak positions in folded spectra, a deep graph neural network 25 , 26 (GNN) for chemical shift estimation within the refinement of chemical shift assignments, and a gradient boosted trees 27 (GBT) model for the selection of structure proposals.
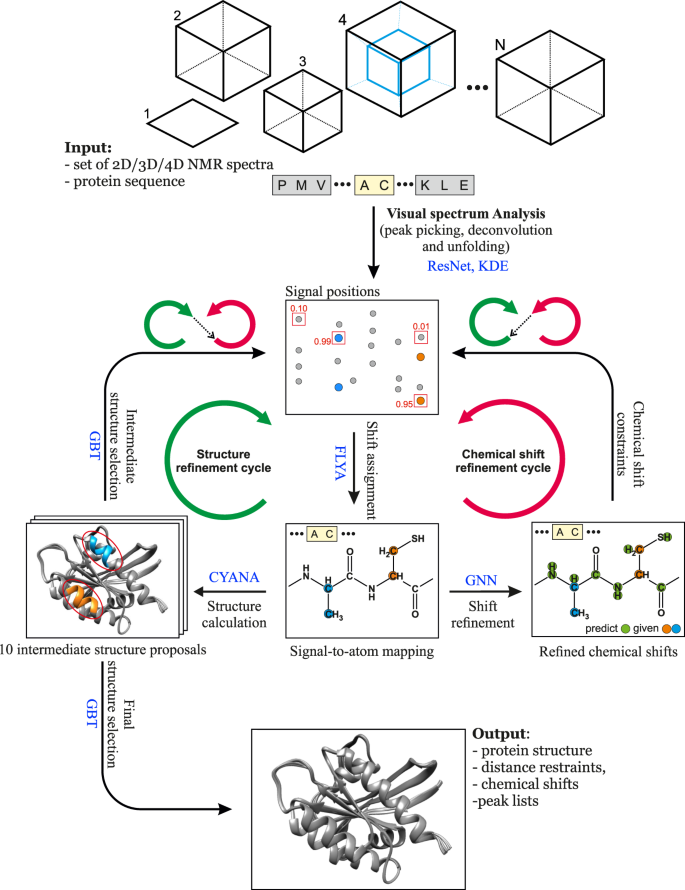
The flowchart presents the interplay between the main components of the automated protein structure determination workflow: Residual Neural Network (ResNet), FLYA automated chemical shift assignment, Graph Neural Network (GNN), Gradient Boosted Trees (GBT), and CYANA structure calculation.
A major challenge in developing ARTINA was the collection and preparation of a large training data set that is required for machine learning, because, in contrast to assignments and structures, NMR spectra are generally not archived in public data repositories. Instead, we were obliged to collect from different sources and standardize complete sets of multidimensional NMR spectra for the assignment and structure determination of 100 proteins.
In the following work, we describe the algorithm, training and test data, and results of ARTINA automated structure determination, which are on par with those achieved in weeks or months of human experts’ labor.
Benchmark dataset
One of the major obstacles for developing deep learning solutions for protein NMR spectroscopy is the lack of a large-scale standardized benchmark dataset of protein NMR spectra. To date, published manuscripts presenting the most notable methods for computational NMR, typically refer to less than 50 2D/3D/4D NMR spectra in their experimental sections. Even the well-recognized CASD-NMR competition cannot serve as a major source of training data for deep learning, since only the NOESY spectra of 10 proteins were used in the last round of the event 28 .
To make our study possible, we established a standardized benchmark of 1329 2D/3D/4D NMR spectra, which allows 100 proteins to be recalculated using their original spectral data (Fig. 2 and Supplementary Table 2 ). Each protein record in our dataset contains 5–20 spectra together with manually identified chemical shifts (usually depositions at the Biological Magnetic Resonance Data Bank, BMRB) and the previously determined (“ground truth”) protein structure (PDB record; Supplementary Table 3 ). The benchmark covers protein sizes typically studied by NMR spectroscopy with sequence lengths between 35 and 175 residues (molecular mass 4–20 kDa).
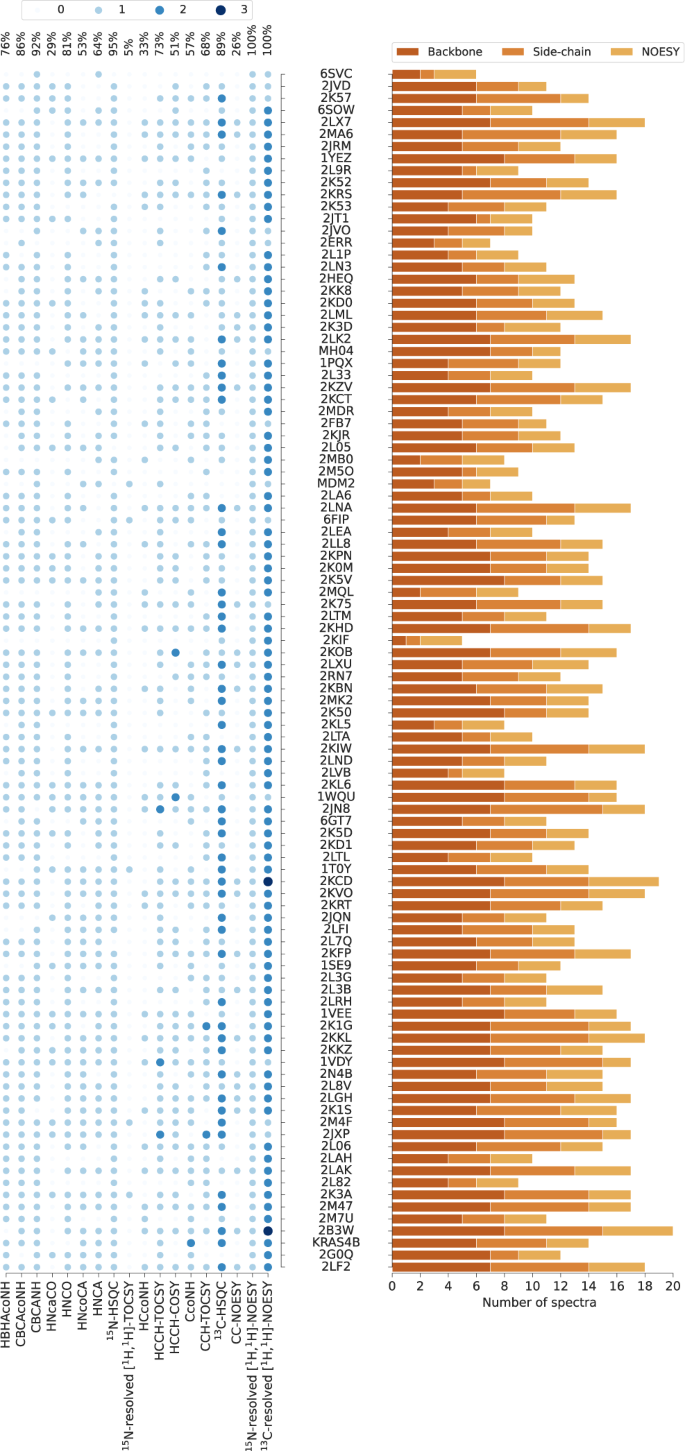
PDB codes (or names, MH04, MDM2, KRAS4B, if PDB code unavailable) of the 100 benchmark proteins are ordered by the number of residues. The histogram shows the number of spectra for backbone assignment, side-chain assignment, and NOE measurement. Spectrum types in each data set are shown by light to dark blue circles indicating the number of individual spectra of the given type. The percentages of benchmark records that contain a given spectrum type are given at the top. Spectrum types present in less than 5% of the data sets have been omitted.
Automated protein structure determination
The accuracy of protein structure determination with ARTINA was evaluated in a 5-fold cross-validation experiment with the aforementioned benchmark dataset. Five instances of pp-ResNet and GBT were trained, each one using data from about 80% of the proteins for training and the remaining ones for testing. Since each protein was present exactly once in the test set, reported quality metrics were obtained directly in the cross-validation experiment, and no averaging between data splits was required. To deploy pp-ResNet and GBT models in our online system, we constructed an ensemble by averaging predictions of all 5 cross-validation models. The other models were trained only once using either generated data (deconv-ResNet, Supplementary Fig. 1 ) or BMRB depositions excluding all benchmark proteins (GNN, KDE).
In this experiment, we reproduced 100 structures in fully automated manner using only NMR spectra and the protein sequences as input. Since ARTINA has no tunable parameters and does not require any manual curation of data, each structure was calculated by a single execution of the ARTINA workflow. All benchmark datasets were analyzed by ARTINA in parallel with execution times of 4–20 h per protein.
All automatically determined structures, overlaid with the corresponding reference structures from the PDB, are visualized in Fig. 3 , Supplementary Fig. 2 , and Supplementary Movie 1 . ARTINA was able to reproduce the reference structures with a median backbone root-mean-square deviation (RMSD) of 1.44 Å between the mean coordinates of the ARTINA structure bundle and the mean coordinates of the corresponding reference PDB structure bundle for the backbone atoms N, C α , C’ in the residue ranges determined by CYRANGE 29 (Fig. 4a and Supplementary Table 4 ). ARTINA automatically identified between 459 and 4678 distance restraints (2198 on average over 100 proteins), which corresponds to 4.25–33.20 restraints per residue (Fig. 4b ). This number is mainly influenced by the extent of unstructured regions and the quality of the NOESY spectra. In agreement with earlier findings 30 , it correlates only weakly with the backbone RMSD to reference (linear correlation coefficient −0.38). As a more expressive validation measure for the structures from ARTINA, we computed a predicted RMSD to the PDB reference structure on the basis of the RMSDs between the 10 candidate structure bundles calculated in ARTINA (see “Methods”, Fig. 5 , and Supplementary Table 5 ). The average deviation between actual and predicted RMSDs for the 100 proteins in this study is 0.35 Å, and their linear correlation coefficient is 0.77 (Fig. 5 ). In no case, the true RMSD exceeds the predicted one by more than 1 Å.
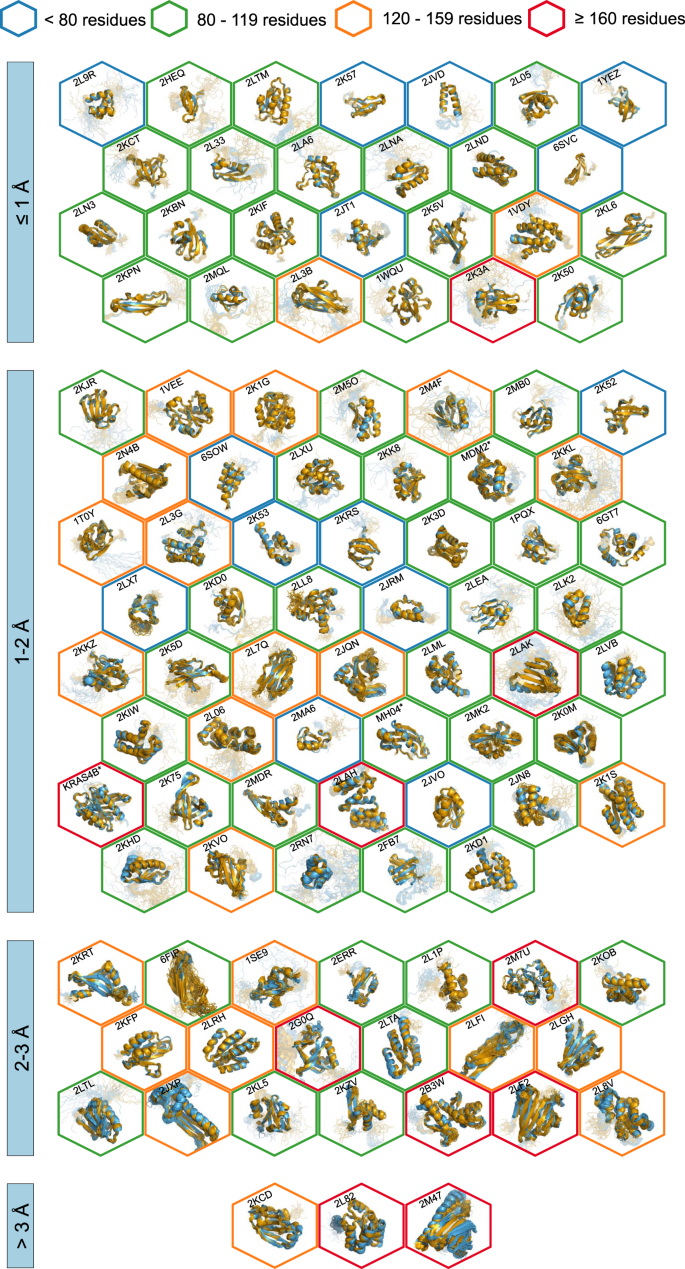
The structures are aligned with the RMSD to reference range as indicated on the left and hexagonal frames color-coded by their size as indicated above. Structures with no corresponding PDB depositions are marked by an asterisk.
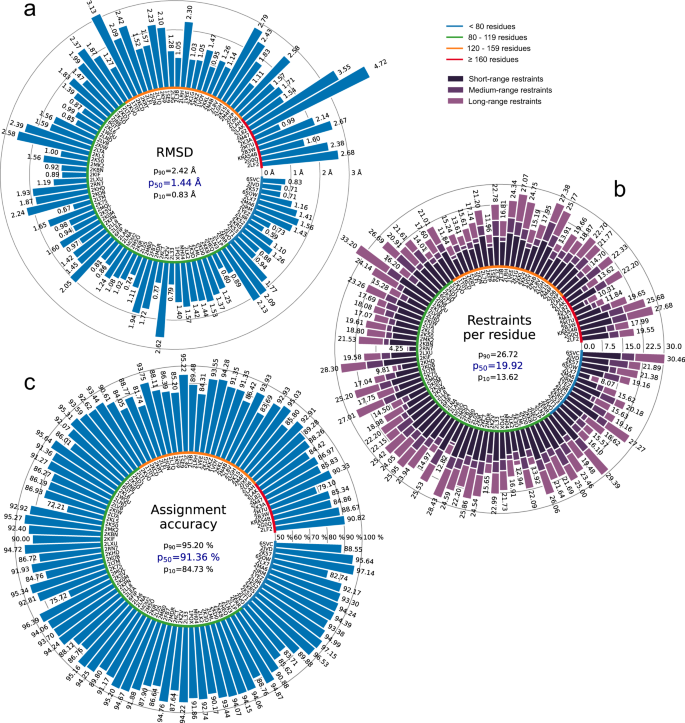
a Backbone RMSD to reference. b Number of distance restraints per residue. c Chemical shift assignment accuracy. Bars represent quantity values for benchmark proteins, identified by PDB codes (or protein names). Proteins are ordered by size, which is indicated by a color-coded circle. Values in the center of each panel are 10th, 50th, and 90th percentiles of values presented in the bar plot. Short/medium/long-range restraints are between residues i and j with | i – j | ≤ 1, 2 ≤ | i – j | ≤ 4, and | i – j | ≥ 5, respectively.
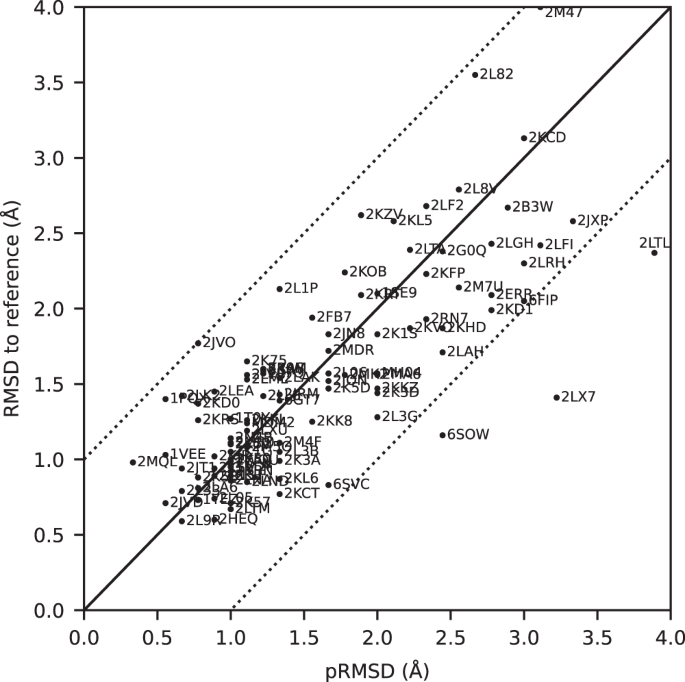
The predicted RMSD to reference (pRMSD) is calculated from the ARTINA results without knowledge of the reference PDB structure (see “Methods”) and, by definition, always in the range of 0–4 Å. For comparability, actual RMSD values to reference are also truncated at 4 Å (protein 2M47 with RMSD 4.47 Å). The dotted lines represent deviations of ±1 Å between the two RMSD quantities.
Additional structure validation scores obtained from ANSSUR 31 (Supplementary Table 6 ), RPF 32 (Supplementary Table 7 ), and consensus structure bundles 33 (Supplementary Table 8 ) confirm that overall the ARTINA structures and the corresponding reference PDB structures are of equivalent quality. Energy refinement of the ARTINA structures in explicit water using OPALp 34 (not part of the standard ARTINA workflow) does not significantly alter the agreement with the PDB reference structures (Supplementary Table 9 ). The benchmark data set comprises 78 protein structures determined by the Northeast Structural Genomics Consortium (NESG). On average, ARTINA yielded structures of the same accuracy for NESG targets (median RMSD to reference 1.44 Å) as for proteins from other sources (1.42 Å).
On average, ARTINA correctly assigned 90.39% of the chemical shifts (Fig. 4c ), as compared to the manually prepared assignments, including both “strong” (high-reliability) and “weak” (tentative) FLYA assignments 12 . Backbone chemical shifts were assigned more accurately (96.03%) than side-chain ones (86.50%), which is mainly due to difficulties in assigning lysine/arginine (79.97%) and aromatic (76.87%) side-chains. Further details on the assignment accuracy for individual amino acid types in the protein cores (residues with less than 20% solvent accessibility) are given in Supplementary Table 10 . Assignments for core residues, which are important for the protein structure, are generally more accurate than for the entire protein, in particular for core Ala, Cys, and Asp residues, which show a median assignment accuracy of 100% over the 100 proteins. The lowest accuracies are observed for core His (83.3%), Phe (83.3%), and Arg (87.5%) residues. The three proteins with highest RMSD to reference, 2KCD, 2L82, and 2M47 (see below), show 68.2, 83.8, and 75.7% correct aromatic assignments, respectively, well below the corresponding median of 85.5%. On the other hand, the assignment accuracies for the methyl-containing residues Ala, Ile, Val are above average and reach a median of 100, 97.6, and 98.6%, respectively.
The quality of automated structure determination and chemical shift assignment reflects the performance of deep learning-based visual spectrum analysis, presented qualitatively in Figs. 6 – 7 , Supplementary Fig. 3 , and Supplementary Movies 2 – 4 . In this experiment, our models (pp-ResNet, deconv-ResNet) automatically identified 1,168,739 cross-peaks with high confidence (≥0.50) in the benchmark spectra. All 1329 peak lists, together with automatically determined protein structures and chemical shift lists, are available for download.
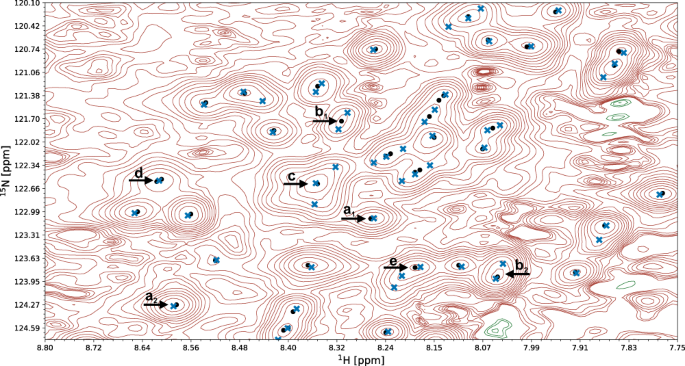
A fragment of a 15 N-HSQC spectrum of the protein 1T0Y is shown. Initial signal positions identified by the peak picking model pp-ResNet (black dots) are deconvolved by deconv-ResNet, yielding the final coordinates used for automated assignment and structure determination (blue crosses). a 1 , a 2 Initial peak picking marker position is refined by the deconvolution model. b 1 , b 2 pp-ResNet output is deconvolved into two components. c The deconvolution model supports maximally 3 components per initial signal. d Two peak picking markers are merged by the deconvolution model. e Peak picking output deconvolved into three components.
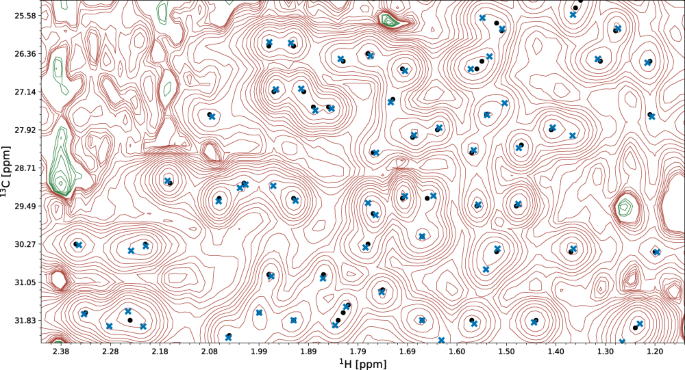
A fragment of the 13 C-HSQC spectrum of protein 2K0M is shown. Initial signal positions identified by the peak picking model pp-ResNet (black dots) are deconvolved by deconv-ResNet, yielding the final coordinates used for automated assignment and structure determination (blue crosses).
Error analysis
The largest deviations from the PDB reference structure were observed for the proteins 2KCD, 2L82, and 2M47, for which the pRMSD consistently indicated low accuracy (Fig. 5 ). Significant deviations are mainly due to displacements of terminal secondary structure elements (e.g., a tilted α-helix near a chain terminus), or inaccurate loop conformations (e.g., more flexible than in the PDB deposition). We investigated the origin of these discrepancies.
2KCD is a 120-residue (14.4 kDa) protein from Staphylococcus saprophyticus with an α-β roll architecture. Its dataset comprises 19 spectra (8 backbone, 6 side-chain, and 5 NOESY). The ARTINA structure has a backbone RMSD to PDB reference of 3.13 Å, which is caused by the displacement of the C-terminal α-helix (residues 105–109; Supplementary Fig. 4a ). Excluding this 5-residue fragment decreases the RMSD to 2.40 Å (Supplementary Table 11 ). The positioning of this helix appears to be uncertain, since an ARTINA calculation without the 4D CC-NOESY spectrum yields a significantly lower RMSD of 1.77 Å (Supplementary Table 12 ).
2L82 is a de novo designed protein of 162 residues (19.7 kDa) with an αβ 3-layer (αβα) sandwich architecture. Although only 9 spectra (4 backbone, 2 side-chain and 3 NOESY) are available, ARTINA correctly assigned 97.87% backbone and 81.05% side-chain chemical shifts. The primary reason for the high RMSD value of 3.55 Å is again a displacement of the C-terminal α-helix (residues 138–153). The remainder of the protein matches closely the PDB deposition (1.04 Å RMSD, Supplementary Fig. 4b ).
The protein with highest RMSD to reference (4.72 Å) in our benchmark dataset is 2M47, a 163-residue (18.8 kDa) protein from Corynebacterium glutamicum with an α-β 2-layer sandwich architecture, for which 17 spectra (7 backbone, 7 side chain and 3 NOESY) are available. The main source of discrepancy are two α-helices spanning residues 111–157 near the C-terminus. Nevertheless, the residues contributing to the high RMSD value are distributed more extensively than in 2L82 and 2KCD just discussed. Interestingly, 2 of the 10 structure proposals calculated by ARTINA have an RMSD to reference below 2 Å (1.66 Å and 1.97 Å). In the final structure selection step, our GBT model selected the 4.72 Å RMSD structure as the first choice and 1.66 Å as the second one (Supplementary Fig. 4c ). Such results imply that the automated structure determination of this protein is unstable. Since ARTINA returns the two structures selected by GBT with the highest confidence, the user can, in principle, choose the better structure based on contextual information.
In addition to these three case studies, we performed a quantitative analysis of all regular secondary structure elements and flexible loops present in our 100-protein benchmark in order to assess their impact on the backbone RMSD to reference (Supplementary Table 11 ). All residues in the structurally well-defined regions determined by CYRANGE 29 were assigned to 6 partially overlapping sets: (a) first secondary structure element, (b) last secondary structure element, (c) α-helices, (d) β-sheets, (e) α-helices and β-sheets, and (f) loops. Then, the RMSD to reference was calculated 6 times, each time with one set excluded. In total, for 66 of the 100 proteins the lowest RMSD was obtained if set (f) was excluded from RMSD calculation, and 13% benefited most from removal of the first or last secondary structure element (a or b). Moreover, for 18 out of the 19 proteins with more than 0.5 Å RMSD decrease compared to the RMSD for all well-defined residues, (a), (b), or (f) was the primary source of discrepancy. These results are consistent with our earlier statement that deviations in automatically determined protein structures are mainly caused by terminal secondary structure elements or inaccurate loop conformations.
Ablation studies
During the experiment, we captured the state of each structure determination at 9 time-points, 3 per structure determination cycle: (a) after the initial FLYA shift assignment, (b) after GNN shift refinement, and (c) after structure calculation (Fig. 1 ). Comparative analysis of these states allowed us to quantify the contribution of different ARTINA components to the structure determination process (Table 1 ).
The results show a strong benefit of the refinement cycles, as quantities reported in Table 1 consistently improve from cycle 1 to 3. The majority of benchmark proteins converge to the correct fold after the first cycle (1.56 Å median backbone RMSD to reference), which is further refined to 1.52 Å in cycle 2 and 1.44 Å in cycle 3. Additionally, within each chemical shift refinement cycle, improvements in assignment accuracy resulting from the GNN predictions are observed. This quantity also increases consistently across all refinement cycles, in particular for side-chains. Refinement cycles are particularly advantageous for large and challenging systems, such as 2LF2, 2M7U, or 2B3W, which benefit substantially in cycles 2 and 3 from the presence of the approximate protein fold in the chemical shift assignment step.
Impact of 4D NOESY experiments
As presented in Fig. 2 , 26 out of 100 benchmark datasets contain 4D CC-NOESY spectra, which require long measurement times and were used in the manual structure determination. To quantify their impact, we performed automated structure determinations of these 26 proteins with and without the 4D CC-NOESY spectra (Supplementary Table 12 ).
On average, the presence of 4D CC-NOESY improves the backbone RMSD to reference by 0.15 Å (decrease from 1.88 to 1.73 Å) and has less than 1% impact on chemical shift assignment accuracy. However, the impact is non-uniform. For three proteins, 2KIW, 2L8V, and 2LF2, use of the 4D CC-NOESY decreased the RMSD by more than 1 Å. On the other hand, there is also one protein, 2KCD, for which the RMSD decreased by more than 1 Å by excluding the 4D CC-NOESY.
These results suggest that overall the amount of information stored in 2D/3D experiments is sufficient for ARTINA to reach close to optimal performance, and only modest improvement can be achieved by introducing additional information redundancy from 4D CC-NOESY spectra.
Automated chemical shift assignment
Apart from structure determination, our data analysis pipeline for protein NMR spectroscopy can address an array of problems that are nowadays approached manually or semi-manually. For instance, ARTINA can be stopped after visual spectrum analysis, returning positions and intensities of cross-peaks that can be utilized for any downstream task, not necessarily related to protein structure determination.
Alternatively, a single chemical shift refinement cycle can be performed to get automatically assigned cross-peaks from spectra and sequence. We evaluated this approach with three sets of spectra: (i) Exclusively backbone assignment spectra were used to assign N, C α , C β , C’, and H N shifts. With this input, ARTINA assigned 92.40% (median value) of the backbone shifts correctly. (ii) All through-bond but no NOESY spectra were used to assign the backbone and side-chain shifts. This raised the percentage of correct backbone assignments to 94.20%. (iii) The full data set including NOESY yielded 96.60% correct assignments of the backbone shifts. These three experiments were performed for the 45 benchmark proteins, for which CBCANH and CBCAcoNH, as well as either HNCA and HNcoCA or HNCO and HNcaCO experiments were available. The availability of NOESY spectra had a large impact on the side-chain assignments: 86.00% were correct for the full spectra set iii, compared to 73.70% in the absence of NOESY spectra (spectra set ii). The presence of NOESY spectra consistently improved the chemical shift assignment accuracy of all amino acid types (Supplementary Tables 13 and 14 ). The improvement is particularly strong for aromatic residues (Phe, 61.6 to 76.5%, Trp 52.5 to 80%, and Tyr 71.4 to 89.7%), but not limited to this group.
The results obtained with ARTINA differ in several aspects substantially from previous approaches towards automating protein NMR analysis 3 , 4 , 7 , 12 , 17 , 18 , 19 , 35 . First, ARTINA comprehends the entire workflow from spectra to structures rather than individual steps in it, and there are strictly no manual interventions or protein-specific parameters to be adapted. Second, the quality of the results regarding peak identification, resonance assignments, and structures have been assessed on a large and diverse set of 100 proteins; for the vast majority of which they are on par with what can be achieved by human experts. Third, the method provides a two-orders-of-magnitude leap in efficiency by providing assignments and a structure within hours of computation time rather than weeks or months of human work. This reduces the effort for a protein structure determination by NMR essentially to the preparation of the sample and the measurement of the spectra. Its implementation in the https://nmrtist.org webserver (Supplementary Movie 5 ) encapsulates its complexity, eliminates any intermediate data and format conversions by the user, and enables the use of different types of high-performance hardware as appropriate for each of the subtasks. ARTINA is not limited to structure determination but can be used equally well for peak picking and resonance assignment in NMR studies that do not aim at a structure, such as investigations of ligand binding or dynamics.
Although ARTINA has no parameters to be optimized by the user, care should be given to the preparation of the input data, i.e., the choice, measurement, processing, and specification of the spectra. Spectrum type, axes, and isotope labeling declarations must be correct, and chemical shift referencing consistent over the entire set of spectra. Slight variations of corresponding chemical shifts within the tolerances of 0.03 ppm for 1 H and 0.4 ppm for 13 C/ 15 N can be accommodated, but larger deviations, resulting, for instance, from the use of multiple samples, pH changes, protein degradation, or inaccurate referencing, can be detrimental. Where appropriate, ARTINA proposes corrections of chemical shift referencing 36 . Furthermore, based on the large training data set, which comprises a large variety of spectral artifacts, ARTINA largely avoids misinterpreting artifacts as signals. However, with decreasing spectral quality, ARTINA, like a human expert, will progressively miss real signals.
Regarding protein size and spectrum quality, limitations of ARTINA are similar to those encountered by a trained spectroscopist. Machine-learning-based visual analysis of spectra requires signals to be present and distinguishable in the spectra. ARTINA does not suffer from accidental oversight that may affect human spectra analysis. On the other hand, human experts may exploit contextual information to which the automated system currently has no access because it identifies individual signals by looking at relatively small, local excerpts of spectra.
In this paper, we used all spectra that are available from the earlier manual structure determination. For most of the 100 proteins, the spectra data set has significant redundancy regarding information for the resonance assignment. Our results indicate that one can expect to obtain good assignments and structures also from smaller sets of spectra 37 , with concomitant savings of NMR measurement time. We plan to investigate this in a future study.
The present version of ARTINA can be enhanced in several directions. Besides improving individual models and algorithms, it is conceivable to integrate the so far independently trained collection of machine learning models, plus additional models that replace conventional algorithms, into a coherent system that is trained as a whole. Furthermore, the reliability of machine learning approaches depends strongly on the quantity and quality of training data available. While the collection of the present training data set for ARTINA was cumbersome, from now on it can be expected to expand continuously through the use of the https://nmrtist.org website, both quantitatively and qualitatively with regard to greater variability in terms of protein types. spectral quality, source laboratory, data processing (including non-linear sampling), etc., which can be exploited in retraining the models. ARTINA can also be extended to use additional experimental input data, e.g., known partial assignments, stereospecific assignments, 3 J couplings, residual dipolar couplings, paramagnetic data, and H-bonds. Structural information, e.g., from AlphaFold 38 , can be used in combination with reduced sets of NMR spectra for rapid structure-based assignment. Finally, the range of application of ARTINA can be generalized to small molecule-protein complexes relevant for structure-activity relationship studies in drug research, protein-protein complexes, RNA, solid state, and in-cell NMR.
Overall, ARTINA stands for a paradigm change in biomolecular NMR from a time-consuming technique for specialists to a fast method open to researchers in molecular biology and medicinal chemistry. At the same time, in a larger perspective, the appearance of generally highly accurate structure predictions by AlphaFold 38 is revolutionizing structural biology. Nevertheless, there remains space for the experimental methods, for instance, to elucidate various states of proteins under different conditions or in dynamic exchange, or for studying protein-ligand interaction. Regarding ARTINA, one should keep in mind that its applications extend far beyond structure determination. It will accelerate virtually any biological NMR studies that require the analysis of multidimensional NMR spectra and chemical shift assignments. Protein structure determination is just one possible ARTINA application, which is both demanding in terms of the amount and quality of required experimental data and amenable to quantitative evaluation.
Spectrum benchmark collection
To collect the benchmark of NMR spectra (Fig. 2 and Supplementary Table 2 ), we implemented a crawler software, which systematically scanned the FTP server of the BMRB data bank 39 , identifying data files relevant to our study. Additional datasets were obtained by setting up a website for the deposition of published data ( https://nmrdb.ethz.ch ), from our collaboration network, or had been acquired internally in our laboratory. NMR data was collected from these channels either in the form of processed spectra (Sparky 40 , NMRpipe 41 , XEASY 42 , Bruker formats), or in the form of time-domain data accompanied by depositor-supplied NMRpipe processing scripts. No additional spectra processing (e.g., baseline correction) was performed as part of this study.
The most challenging aspects of the benchmark collection process were: scarcity of data—only a small fraction of all BMRB depositions are accompanied by uploaded spectra (or time-domain data), lack of standards for NMR data depositions—each protein data set had to be prepared manually, as the original data was stored in different formats (spectra name conventions, axis label standards, spectra data format), and difficulties in correlating data files deposited in the BMRB FTP site with contextual information about the spectrum and the sample (e.g., sample characteristics, measurement conditions, instrument used). Manually prepared (mostly NOESY) peak lists, which are available from the BMRB for some of the proteins in the benchmark, were not used for this study.
Different approaches to 3D 13 C-NOESY spectra measurement had to be taken into account: (i) Two separate 13 C NOESY for aliphatic and aromatic signals. These were analyzed by ARTINA without any special treatment. We used ALI , ARO tags (Supplementary Movie S5 ) to provide the information that only either aliphatic or aromatics shifts are expected in a given spectrum. (ii) Simultaneous NC-NOESY. These spectra were processed twice to have proper scaling of the 13 C and 15 N axes in ppm units, and cropped to extract 15 N-NOESY and 13 C-NOESY spectra. If nitrogen and carbon cross-peak amplitudes have different signs, we used POS , NEG tags to provide the information that only either positive or negative signals should be analyzed. (iii) Aliphatic and aromatic signals in a single 13 C-NOESY spectrum. These measurements do not require any special treatment, but proper cross-peak unfolding plays a vital role in aromatic signals analysis.
Overview of the ARTINA algorithm
ARTINA uses as input only the protein sequence and a set of NMR spectra, which may contain any combination of 25 experiments currently supported by the method (Supplementary Table 1 ). Within 4–20 h of computation time (depending on protein size, number of spectra, and computing hardware load), ARTINA determines: (a) cross-peak positions for each spectrum, (b) chemical shift assignments, (c) distance restraints from NOESY spectra, and (d) the protein structure. The whole process does not require any human involvement, allowing rapid protein NMR assignment and structure determination by non-experts.
The ARTINA workflow starts with visual spectrum analysis (Fig. 1 ), wherein cross-peak positions are identified in frequency-domain NMR spectra using deep residual neural networks (ResNet) 24 . Coordinates of signals in the spectra are passed as input to the FLYA automated assignment algorithm 12 , yielding initial chemical shift assignments . In the subsequent chemical shift refinement step, we bring to the workflow contextual information about thousands of protein structures solved by NMR in the past using a deep GNN 25 that was trained on BMRB/PDB depositions. Its goal is to predict expected values of yet missing chemical shifts, given the shifts that have already been confidently and unambiguously assigned by FLYA. With these GNN predictions as additional input, the cross-peak positions are reassessed in a second FLYA call, which completes the chemical shift refinement cycle (Fig. 1 ).
In the structure refinement cycle , 10 variants of NOESY peak lists are generated, which differ in the number of cross-peaks selected from the output of the visual spectrum analysis by varying the confidence threshold of a signal selected by ResNet between 0.05 and 0.5. Each set of NOESY peak lists is used in an independent CYANA structure calculation 22 , 23 , yielding 10 intermediate structure proposals (Fig. 1 ). The structure proposals are ranked in the intermediate structure selection step based on 96 features with a dedicated GBT model. The selected best structure proposal is used as contextual information in a consecutive FLYA run, which closes the structure refinement cycle .
After the two initial steps of visual spectrum analysis and initial chemical shift assignment, ARTINA interchangeably executes refinement cycles. The chemical shift refinement cycle provides FLYA with tighter restraints on expected chemical shifts, which helps to assign ambiguous cross-peaks. The structure refinement cycle provides information about possible through-space contacts, allowing identified cross-peaks (especially in NOESY) to be reassigned. The high-level concept behind the interchangeable execution of refinement cycles is to iteratively update the protein structure given fixed chemical shifts, and update chemical shifts given the fixed protein structure. Both refinement cycles are executed three times.
Automated visual analysis of the spectrum
We established two machine learning models for the visual analysis of multidimensional NMR spectra (see downloads in the Code availability section). In their design, we made no assumptions about the downstream task and the 2D/3D/4D experiment type. Therefore, the proposed models can be used as the starting point of our automated structure determination procedure, as well as for any other task that requires cross-peak coordinates.
The automated visual analysis starts by selecting all extrema \({{{{{\boldsymbol{x}}}}}}=\left\{{{{{{{\boldsymbol{x}}}}}}}_{1},{{{{{{\boldsymbol{x}}}}}}}_{2},\ldots,{{{{{{\boldsymbol{x}}}}}}}_{N}\right\}\) , \({{{{{{\boldsymbol{x}}}}}}}_{n}\in {{\mathbb{N}}}^{D}\) in the NMR spectrum, which is represented as a D -dimensional regular grid storing signal intensities at discrete frequencies. We formulated the peak picking task as an object detection problem, where possible object positions are confined to \({{{{{\boldsymbol{x}}}}}}\) . This task was addressed by training a deep residual neural network 24 , in the following denoted as peak picking ResNet (pp-ResNet), which learns a mapping \({{{{{{\boldsymbol{x}}}}}}}_{n}\to[0,\,1]\) that assigns to each signal extremum a real-valued score, which resembles its probability of being a true signal rather than an artefact.
Our network architecture is strongly linked to ResNet-18 24 . It contains 8 residual blocks, followed by a single fully connected layer with sigmoidal activation. After weight initialization with Glorot Uniform 43 , the architecture was trained by optimizing a binary cross-entropy loss using Adam 44 with learning rate 10 –4 and gradient clipping of 0.5.
To establish an experimental training dataset for pp-ResNet, we normalized the 1329 spectra in our benchmark with respect to resolution (adjusting the number of data grid points per unit chemical shift (ppm) using linear interpolation) and signal amplitude (scaling the spectrum by a constant). Subsequently, 675,423 diverse 2D fragments of size 256 × 32 × 1 were extracted from the normalized spectra and manually annotated, yielding 98,730 positive and 576,693 negative class training examples. During the training process, we additionally augmented this dataset by flipping spectrum fragments along the second dimension (32 pixels), stretching them by 0–30% in the first and second dimensions, and perturbing signal intensities with Gaussian noise addition.
The role of the pp-ResNet is to quickly iterate over signal extrema in the spectrum, filtering out artefacts and selecting approximate cross-peak positions for the downstream task. The relatively small network architecture (8 residual blocks) and input size of 2D 256 × 32 image patches make it possible to analyze large 3D 13 C-resolved NOESY spectra in less than 5 min on a high-end desktop computer. Simultaneously, the first dimension of the image patch (256 pixels) provides long-range contextual information on the possible presence of signals aligned with the current extremum (e.g., C α , C β cross-peaks in an HNCACB spectrum).
Extrema classified with high confidence as true signals by pp-ResNet undergo subsequent analysis with a second deep residual neural network (deconv-ResNet). Its objective is to perform signal deconvolution, based on a 3D spectrum fragment (64 × 32 × 5 voxels) that is cropped around a signal extremum selected by pp-ResNet. This task is defined as a regression problem, where deconv-ResNet outputs a 3 × 3 matrix storing 3D coordinates of up to 3 deconvolved peak components, relative to the center of the input image. To ensure permutation invariance with respect to the ordering of components in the output coordinate matrix, and to allow for a variable number of 1–3 peak components, the architecture was trained with a Chamfer distance loss 45 .
Since deconv-ResNet deals only with true signals and their local neighborhood, its training dataset can be conveniently generated. We established a spectrum fragment generator, based on rules reflecting the physics of NMR, which produced 110,000 synthetic training examples (Supplementary Fig. 1 ) having variable (a) numbers of components to deconvolve (1–3), (b) signal-to-noise ratio, (c) component shapes (Gaussian, Lorentzian, and mixed), (d) component amplitude ratios, (e) component separation, and (f) component neighborhood type (i.e., NOESY-like signal strips or HSQC-like 2D signal clusters). The deconv-ResNet model was thus trained on fully synthetic data.
Signal unaliasing
To use ResNet predictions in automated chemical shift assignment and structure calculation, detected cross-peak coordinates must be transformed from the spectrum coordinate system to their true resonance frequencies. We addressed the problem of automated signal unfolding with the classical machine learning approach to density estimation.
At first, we generated 10 5 cross-peaks associated with each experiment type supported by ARTINA (Supplementary Table 1 ). In this process, we used randomly selected chemical shift lists deposited in the BMRB database, excluding depositions associated with our benchmark proteins. Subsequently, we trained a Kernel Density Estimator (KDE):
which captures the distribution \({p}_{e}\left({{{{{\boldsymbol{x}}}}}}\right)\) of true peaks being present at position \({{{{{\boldsymbol{x}}}}}}\) in spectrum type \(e\) , based on N e = 10 5 cross-peaks coordinates \({{{{{{\boldsymbol{x}}}}}}}_{i}^{(e)}\) generated with BMRB data, and \(\kappa\) being the Gaussian kernel.
Unfolding a k -dimensional spectrum is defined as a discrete optimization problem, solved independently for each cross-peak \({{{{{{\boldsymbol{x}}}}}}}_{j}^{\left(e\right)}\) observed in a spectrum of type \(e\) :
where \({{{{{\boldsymbol{w}}}}}}\in{{\mathbb{R}}}^{k}\) is a vector storing the spectral widths in each dimension (ppm units), \({{\circ }}\) is element-wise multiplication, \({{{{{\boldsymbol{s}}}}}}\in \,{{\mathbb{Z}}}^{k}\) is a vector indicating how many times the cross-peak is unfolded in each dimension, and \({{{{{{\boldsymbol{s}}}}}}}^{{{{{{\boldsymbol{*}}}}}}}\in {{\mathbb{Z}}}^{k}\) is the optimal cross-peak unfolding.
As long as regular and folded signals do not overlap or have different signs in the spectrum, KDE can unfold the peak list regardless of spectrum dimensionality. The spectrum must not be cropped in the folded dimension, i.e., the folding sweep width must equal the width of the spectrum in the corresponding dimension.
All 2D/3D spectra in our benchmark were folded in at most one dimension and satisfy the aforementioned requirements. However, the 4D CC-NOESY spectra satisfy neither, as regular and folded peaks both overlap and have the same signal amplitude sign. This introduces ambiguity in the spectrum unfolding that prevents direct use of the KDE technique. To retrieve original signal positions, 4D CC-NOESY cross-peaks were unfolded to overlap with signals detected in 3D 13 C-NOESY. In consequence, 4D CC-NOESY unfolding depended on other experiments, and individual 4D cross-peaks were retained only if they were confirmed in a 3D experiment.
Chemical shift assignment
Chemical shift assignment is performed with the existing FLYA algorithm 12 that uses a genetic algorithm combined with local optimization to find an optimal matching between expected and observed peaks. FLYA uses as input the protein sequence, lists of peak positions from the available spectra, chemical shift statistics, either from the BMRB 39 or the GNN described in the next section, and, if available, the structure from the previous refinement cycle. The tolerance for the matching of peak positions and chemical shifts was set to 0.03 ppm for 1 H, and 0.4 ppm for 13 C/ 15 N shifts. Each FLYA execution comprises 20 independent runs with identical input data that differ in the random numbers used in the optimization algorithm. Nuclei for which at least 80% of the 20 runs yield, within tolerance, the same chemical shift value are classified as reliably assigned 12 and used as input for the following chemical shift refinement step.
Chemical shift refinement
We used a graph data structure to combine FLYA-assigned shifts with information from previously assigned proteins (BMRB records) and possible spatial interactions. Each node corresponds to an atom in the protein sequence, and is represented by a feature vector composed of (a) a one-hot encoded atom type code (e.g., C α , H β ), (b) a one-hot encoded amino acid type, (c) the value of the chemical shift assigned by FLYA (only if a confident assignment is available, zero otherwise), (d) atom-specific BMRB shift statistics (mean and standard deviation), and (e) 30 chemical shift values obtained from BMRB database fragments. The latter feature is obtained by searching BMRB records for assigned 2–3-residue fragments that match the local protein sequence and have minimal mean-squared-error (MSE) to shifts confidently assigned by FLYA (non-zero values of feature (c) in the local neighborhood of the atom). The edges of the graph correspond to chemical bonds or skip connections. The latter connect the C β atom of a given residue with C β atoms 2, 3, and 5 residues apart in the amino acid sequence, and have the purpose to capture possible through-space influence on the chemical shift that is typically observed in secondary structure elements.
The chemical shift refinement task is defined as a node regression problem, where an expected value of the chemical shift is predicted for each atom that lacks a confident FLYA assignment. This task is addressed with a DeepGCN model 25 , 26 that was trained on 28,400 graphs extracted from 2840 referenced BMRB records 39 . Each training example was created by building a fully assigned graph out of a single BMRB record, and dropping chemical shift values (feature (c) above) for randomly chosen atoms that FLYA typically assigns either with low confidence or inaccurately.
Our DeepGCN model is designed specifically for de novo structure determination, as it uses only the protein sequence and partial shift assignments to estimate values of missing chemical shifts. Its predictions are used to guide the FLYA genetic algorithm optimization 12 by reducing its search range for assignments. The precise final chemical shift value is always determined by the position of a signal in the spectrum, rather than the model prediction alone.
Torsion angle restraints
Before each structure calculation step, torsion angle restraints for the ϕ and ψ angles of the polypeptide backbone were obtained from the current backbone chemical shifts using the program TALOS-N 21 . Restraints were only generated if TALOS-N classified the prediction as ‘Good’, ‘Strong’, or ‘Generous’. Given a TALOS-N torsion angle prediction of ϕ ± Δ ϕ , the allowed range of the torsion angle was set to ϕ ± max(Δ ϕ , 10°) for ‘Good’ and ‘Strong’ predictions, and ϕ ± 1.5 max(Δ ϕ , 10°) for ‘Generous’ predictions, and likewise for ψ .
Structure calculation and selection
Given the chemical shift assignments and NOESY cross-peak positions and intensities, the structure is calculated with CYANA 23 using the established method 22 that comprises 7 cycles of NOESY cross-peak assignment and structure calculation, followed by a final structure calculation. In total, 8 × 100 conformers are calculated for a given input data set using 30,000 torsion angle dynamics steps per conformer. The 20 conformers with the lowest final target function value are chosen to represent the solution structure proposal. The entire combined NOESY assignment and structure calculation procedure is executed independently 10 times based on 10 variants of NOESY peak lists, which differ in the number of cross-peaks selected from the output of the visual spectrum analysis. The first set generously includes all signals selected by ResNet with confidence ≥0.05. The other variants of NOESY peak lists follow the same principle with increasingly restrictive confidence thresholds of 0.1, 0.15, …, 0.5.
The CYANA structures calculations are followed by a structure selection step, wherein the 10 intermediate structure proposals are compared pairwise by a Gradient Boosted Tree (GBT) model that uses 96 features from each structure proposal (including the CYANA target function value 23 , number of long-range distance restraints, etc.; for details, see downloads in the Code availability section) to rank the structures by their expected accuracy. The best structure from the ranking is subsequently used as contextual information for the chemical shift refinement cycle (Fig. 1 ), or returned as the final outcome of ARTINA. The second-best final structure is also returned for comparison.
To train GBT, we collected a set of successful and unsuccessful structure calculations with CYANA. Each training example was a tuple ( s i , r i ), where s i is the vector of features extracted from the CYANA structure calculation output, and r i is the RMSD of the output structure to the PDB reference. The GBT was trained to take the features s i and s j of two structure calculations with CYANA as input, and to predict a binary order variable o ij , such that o ij = 1 if r i < r j , and 0 otherwise. Importantly, the deposited PDB reference structures were not used directly in the GBT model training (they are used only to calculate the RMSDs). Consequently, the GBT model is unaffected by methodology and technicalities related to PDB deposition (e.g., the structure calculation software used to calculate the deposited reference structure).
Structure accuracy estimate
As an accuracy estimate for the final ARTINA structure, a predicted RMSD to reference (pRMSD) is calculated from the ARTINA results (without knowledge of the reference PDB structure). It aims at reproducing the actual RMSD to reference, which is the RMSD between the mean coordinates of the ARTINA structure bundle and the mean coordinates of the corresponding reference PDB structure bundle for the backbone atoms N, C α , C’ in the residue ranges as given in Supplementary Table 4 . The predicted RMSD is given by pRMSD = (1 – t ) × 4 Å, where, in analogy to the GDT_HA value 46 , t is the average fraction of the RMSDs ≤ 0.5, 1, 2, 4 Å between the mean coordinates of the best ARTINA candidate structure bundle and the mean coordinates of the structure bundles of the 9 other structure proposals. Since t ∈ [0, 1], the pRMSD is always in the range of 0–4 Å, grouping all “bad” structures with expected RMSD to reference ≥ 4 Å at pRMSD = 4 Å.
Reporting summary
Further information on research design is available in the Nature Research Reporting Summary linked to this article.
Data availability
References structures: PDB Protein Data Bank ( https://www.rcsb.org/ ; accession codes in Fig. 2 and Supplementary Table 3 ).
Spectra and reference assignments: BMRB Biological Magnetic Resonance Data Bank ( https://bmrb.io/ ; entry IDs in Supplementary Table 3 ).
Peak lists, assignments, and structures: https://nmrtist.org/static/public/publications/artina/ARTINA_results.zip and in the ETH Research Collection under DOI 10.3929/ethz-b-000568621.
Source data for Figs. 2 , 4 , and 5 is available in Supplementary Tables 2 , 4 , and 5, respectively.
Code availability
The ARTINA algorithm is available as a webserver at https://nmrtist.org . pp-ResNet, deconv-ResNet, GNN, and GBT are available for download in binary form, together with architecture schemes, example input data, model input description, and source code that allows to read model files and make predictions ( https://github.com/PiotrKlukowski/ARTINA , https://nmrtist.org/static/public/publications/artina/models/ {ARTINA_peak_picking.zip, ARTINA_peak_deconvolution.zip, ARTINA_shift_prediction.zip, ARTINA_structure_ranking.zip}). These files provide a full technical specification of the components developed within ARTINA, and allow for their independent use in Python.
Existing software used: Python ( https://www.python.org/ ), CYANA ( https://www.las.jp/ ), TALOS-N ( https://spin.niddk.nih.gov/bax/software/TALOS-N ).
Wüthrich, K. NMR studies of structure and function of biological macromolecules (Nobel Lecture). Angew. Chem. Int. Ed. 42 , 3340–3363 (2003).
Article CAS Google Scholar
Sakakibara, D. et al. Protein structure determination in living cells by in-cell NMR spectroscopy. Nature 458 , 102–105 (2009).
Article ADS CAS Google Scholar
Guerry, P. & Herrmann, T. Advances in automated NMR protein structure determination. Q. Rev. Biophys. 44 , 257–309 (2011).
Güntert, P. Automated structure determination from NMR spectra. Eur. Biophys. J. 38 , 129–143 (2009).
Garrett, D. S., Powers, R., Gronenborn, A. M. & Clore, G. M. A common sense approach to peak picking two-, three- and four-dimensional spectra using automatic computer analysis of contour diagrams. J. Magn. Reson. 95 , 214–220 (1991).
ADS CAS Google Scholar
Koradi, R., Billeter, M., Engeli, M., Güntert, P. & Wüthrich, K. Automated peak picking and peak integration in macromolecular NMR spectra using AUTOPSY. J. Magn. Reson. 135 , 288–297 (1998).
Würz, J. M. & Güntert, P. Peak picking multidimensional NMR spectra with the contour geometry based algorithm CYPICK. J. Biomol. NMR 67 , 63–76 (2017).
Klukowski, P. et al. NMRNet: A deep learning approach to automated peak picking of protein NMR spectra. Bioinformatics 34 , 2590–2597 (2018).
Li, D. W., Hansen, A. L., Yuan, C. H., Bruschweiler-Li, L. & Brüschweiler, R. DEEP picker is a deep neural network for accurate deconvolution of complex two-dimensional NMR spectra. Nat. Commun. 12 , 5229 (2021).
Bartels, C., Güntert, P., Billeter, M. & Wüthrich, K. GARANT—A general algorithm for resonance assignment of multidimensional nuclear magnetic resonance spectra. J. Comput. Chem. 18 , 139–149 (1997).
Zimmerman, D. E. et al. Automated analysis of protein NMR assignments using methods from artificial intelligence. J. Mol. Biol. 269 , 592–610 (1997).
Schmidt, E. & Güntert, P. A new algorithm for reliable and general NMR resonance assignment. J. Am. Chem. Soc. 134 , 12817–12829 (2012).
Linge, J. P., O’Donoghue, S. I. & Nilges, M. Automated assignment of ambiguous nuclear overhauser effects with ARIA. Methods Enzymol. 339 , 71–90 (2001).
Herrmann, T., Güntert, P. & Wüthrich, K. Protein NMR structure determination with automated NOE assignment using the new software CANDID and the torsion angle dynamics algorithm DYANA. J. Mol. Biol. 319 , 209–227 (2002).
Allain, F., Mareuil, F., Ménager, H., Nilges, M. & Bardiaux, B. ARIAweb: a server for automated NMR structure calculation. Nucleic Acids Res. 48 , W41–W47 (2020).
Lee, W. et al. I-PINE web server: Aan integrative probabilistic NMR assignment system for proteins. J. Biomol. NMR 73 , 213–222 (2019).
Huang, Y. P. J. et al. An integrated platform for automated analysis of protein NMR structures. Methods Enzymol. 394 , 111–141 (2005).
Kobayashi, N. et al. KUJIRA, a package of integrated modules for systematic and interactive analysis of NMR data directed to high-throughput NMR structure studies. J. Biomol. NMR 39 , 31–52 (2007).
López-Méndez, B. & Güntert, P. Automated protein structure determination from NMR spectra. J. Am. Chem. Soc. 128 , 13112–13122 (2006).
Murphy, K. P. Probabilistic Machine Learning: An Introduction (MIT Press, 2022).
Shen, Y. & Bax, A. Protein backbone and sidechain torsion angles predicted from NMR chemical shifts using artificial neural networks. J. Biomol. NMR 56 , 227–241 (2013).
Güntert, P. & Buchner, L. Combined automated NOE assignment and structure calculation with CYANA. J. Biomol. NMR 62 , 453–471 (2015).
Güntert, P., Mumenthaler, C. & Wüthrich, K. Torsion angle dynamics for NMR structure calculation with the new program DYANA. J. Mol. Biol. 273 , 283–298 (1997).
Article Google Scholar
Kaiming, H., Xiangyu, Z., Shaoqing, R. & Jian, S. Deep residual learning for image recognition. In Proc. 2016 IEEE Conference on Computer Vision and Pattern Recognition (CVPR) 770–778 (2016).
Kipf, T. N. & Welling, M. Semi-supervised classification with graph convolutional networks. Preprint at https://arxiv.org/abs/1609.02907 (2016).
Chiang, W. L. et al. Cluster-GCN: An efficient algorithm for training deep and large graph convolutional networks. In Proc. 25th ACM SIGKDD International Conference on Knowledge Discovery & Data Mining (KDD) 257–266 (2019).
Prokhorenkova, L., Gusev, G., Vorobev, A., Dorogush, A. V. & Gulin, A. CatBoost: Unbiased boosting with categorical features. In Proc. 32nd Conference on Neural Information Processing Systems (NIPS) (2018).
Rosato, A. et al. The second round of Critical Assessment of Automated Structure Determination of Proteins by NMR: CASD-NMR-2013. J. Biomol. NMR 62 , 413–424 (2015).
Kirchner, D. K. & Güntert, P. Objective identification of residue ranges for the superposition of protein structures. BMC Bioinform. 12 , 170 (2011).
Buchner, L. & Güntert, P. Systematic evaluation of combined automated NOE assignment and structure calculation with CYANA. J. Biomol. NMR 62 , 81–95 (2015).
Fowler, N. J., Sljoka, A. & Williamson, M. P. A method for validating the accuracy of NMR protein structures. Nat. Commun . 11 , 6321 (2020).
Huang, Y. J., Powers, R. & Montelione, G. T. Protein NMR recall, precision, and F-measure scores (RPF scores): Structure quality assessment measures based on information retrieval statistics. J. Am. Chem. Soc. 127 , 1665–1674 (2005).
Buchner, L. & Güntert, P. Increased reliability of nuclear magnetic resonance protein structures by consensus structure bundles. Structure 23 , 425–434 (2015).
Koradi, R., Billeter, M. & Güntert, P. Point-centered domain decomposition for parallel molecular dynamics simulation. Comput. Phys. Commun. 124 , 139–147 (2000).
Herrmann, T., Güntert, P. & Wüthrich, K. Protein NMR structure determination with automated NOE-identification in the NOESY spectra using the new software ATNOS. J. Biomol. NMR 24 , 171–189 (2002).
Buchner, L., Schmidt, E. & Güntert, P. Peakmatch: A simple and robust method for peak list matching. J. Biomol. NMR 55 , 267–277 (2013).
Scott, A., López-Méndez, B. & Güntert, P. Fully automated structure determinations of the Fes SH2 domain using different sets of NMR spectra. Magn. Reson. Chem. 44 , S83–S88 (2006).
Jumper, J. et al. Highly accurate protein structure prediction with AlphaFold. Nature 596 , 583–589 (2021).
Ulrich, E. L. et al. BioMagResBank. Nucleic Acids Res. 36 , D402–D408 (2008).
Goddard, T. D. & Kneller, D. G. Sparky 3. (University of California, San Francisco, 2001).
Delaglio, F. et al. NMRPipe—A multidimensional spectral processing system based on Unix pipes. J. Biomol. NMR 6 , 277–293 (1995).
Bartels, C., Xia, T. H., Billeter, M., Güntert, P. & Wüthrich, K. The program XEASY for computer-supported NMR spectral analysis of biological macromolecules. J. Biomol. NMR 6 , 1–10 (1995).
Glorot, X. & Bengio, Y. Understanding the difficulty of training deep feedforward neural networks. Proc. Mach. Learn. Res. 9 , 249–256 (2010).
Google Scholar
Kingma, D. P. & Ba, J. Adam: A method for stochastic optimization. Preprint at https://arxiv.org/abs/1412.6980 (2015).
Davies, E. R. Computer Vision (Academic Press, 2018).
Kryshtafovych, A. et al. New tools and expanded data analysis capabilities at the protein structure prediction center. Proteins 69 , 19–26 (2007).
Download references
Acknowledgements
We thank Drs. Frédéric Allain, Fred Damberger, Hideo Iwai, Harindranath Kadavath, Julien Orts, and Dean Strotz for providing unpublished spectra. This project has received funding from the European Union’s Horizon 2020 research and innovation program under the Marie Sklodowska-Curie grant agreement No 891690 (P.K.), and a Grant-in-Aid for Scientific Research of the Japan Society for the Promotion of Science (P.G., 20 K06508).
Author information
Authors and affiliations.
Laboratory of Physical Chemistry, ETH Zurich, Vladimir-Prelog-Weg 2, 8093, Zurich, Switzerland
Piotr Klukowski, Roland Riek & Peter Güntert
Institute of Biophysical Chemistry, Goethe University Frankfurt, Max-von-Laue-Str. 9, 60438, Frankfurt am Main, Germany
- Peter Güntert
Department of Chemistry, Tokyo Metropolitan University, 1-1 Minami-Osawa, Hachioji, 192-0397, Tokyo, Japan
You can also search for this author in PubMed Google Scholar
Contributions
P.K. prepared training and test data sets, designed and trained machine learning models, performed experiments described in the manuscript, and implemented ARTINA within the nmrtist.org web platform. P.K. and P.G. wrote the software. P.K., R.R., and P.G. conceived the project, analyzed the results, and wrote the manuscript.
Corresponding authors
Correspondence to Piotr Klukowski , Roland Riek or Peter Güntert .
Ethics declarations
Competing interests.
The authors declare no competing interests.
Peer review
Peer review information.
Nature Communications thanks Benjamin Bardiaux, Gaetano Montelione, Theresa Ramelot, and the other, anonymous, reviewer(s) for their contribution to the peer review of this work. Peer reviewer reports are available.
Additional information
Publisher’s note Springer Nature remains neutral with regard to jurisdictional claims in published maps and institutional affiliations.
Supplementary information
Supplementary info file #1, description of additional supplementary files, supplementary movie 1, supplementary movie 2, supplementary movie 3, supplementary movie 4, supplementary movie 5, reporting summary, peer review file, rights and permissions.
Open Access This article is licensed under a Creative Commons Attribution 4.0 International License, which permits use, sharing, adaptation, distribution and reproduction in any medium or format, as long as you give appropriate credit to the original author(s) and the source, provide a link to the Creative Commons license, and indicate if changes were made. The images or other third party material in this article are included in the article’s Creative Commons license, unless indicated otherwise in a credit line to the material. If material is not included in the article’s Creative Commons license and your intended use is not permitted by statutory regulation or exceeds the permitted use, you will need to obtain permission directly from the copyright holder. To view a copy of this license, visit http://creativecommons.org/licenses/by/4.0/ .
Reprints and permissions
About this article
Cite this article.
Klukowski, P., Riek, R. & Güntert, P. Rapid protein assignments and structures from raw NMR spectra with the deep learning technique ARTINA. Nat Commun 13 , 6151 (2022). https://doi.org/10.1038/s41467-022-33879-5
Download citation
Received : 28 March 2022
Accepted : 30 September 2022
Published : 18 October 2022
DOI : https://doi.org/10.1038/s41467-022-33879-5
Share this article
Anyone you share the following link with will be able to read this content:
Sorry, a shareable link is not currently available for this article.
Provided by the Springer Nature SharedIt content-sharing initiative
This article is cited by
- Gogulan Karunanithy
- Vaibhav Kumar Shukla
- D. Flemming Hansen
Nature Communications (2024)
- Piotr Klukowski
- Fred F. Damberger
Scientific Data (2024)
Manual and automatic assignment of two different Aβ40 amyloid fibril polymorphs using MAS solid-state NMR spectroscopy
- Natalia Rodina
- Riddhiman Sarkar
Biomolecular NMR Assignments (2024)
Overlay databank unlocks data-driven analyses of biomolecules for all
- Anne M. Kiirikki
- Hanne S. Antila
- O. H. Samuli Ollila
5D solid-state NMR spectroscopy for facilitated resonance assignment
- Alexander Klein
- Suresh K. Vasa
- Rasmus Linser
Journal of Biomolecular NMR (2023)
By submitting a comment you agree to abide by our Terms and Community Guidelines . If you find something abusive or that does not comply with our terms or guidelines please flag it as inappropriate.
Quick links
- Explore articles by subject
- Guide to authors
- Editorial policies
Sign up for the Nature Briefing newsletter — what matters in science, free to your inbox daily.


An official website of the United States government
The .gov means it’s official. Federal government websites often end in .gov or .mil. Before sharing sensitive information, make sure you’re on a federal government site.
The site is secure. The https:// ensures that you are connecting to the official website and that any information you provide is encrypted and transmitted securely.
- Publications
- Account settings
Preview improvements coming to the PMC website in October 2024. Learn More or Try it out now .
- Advanced Search
- Journal List
- v.13(1); 2019 Feb
Nuclear Magnetic Resonance Spectroscopy for Medical and Dental Applications: A Comprehensive Review
1 Department of Oral Biology, Riyadh College of Dentistry and Pharmacy, Riyadh, Saudi Arabia
Talal Siddiqui
2 General Dental Practitioner, Karachi, Pakistan
3 Department of Biomedical Dental Sciences, College of Dentistry, Imam Abdulrahman Bin Faisal University, Dammam, Saudi Arabia
Imran Farooq
Muhammad sohail zafar.
4 Department of Restorative Dentistry, College of Dentistry, Taibah University, Madinah Munawwarah, Saudi Arabia
5 Department of Dental Materials, Islamic International Dental College, Riphah International University, Islamabad, Pakistan
Zohaib Khurshid
6 Department of Prosthodontics and Implantology, College of Dentistry, King Faisal University, Al-Ahsa, Saudi Arabia
Nuclear magnetic resonance (NMR) spectroscopy is one of the most significant analytical techniques that has been developed in the past few decades. A broad range of biological and nonbiological applications ranging from an individual cell to organs and tissues has been investigated through NMR. Various aspects of this technique are still under research, and many functions of the NMR are still pending a better understanding and acknowledgment. Therefore, this review is aimed at providing a general overview of the main principles, types of this technique, and the advantages and disadvantages of NMR spectroscopy. In addition, an insight into the current uses of NMR in the field of medicine and dentistry and ongoing developments of NMR spectroscopy for future applications has been discussed.
Introduction
The electromagnetic spectra have been routinely used in the field of medicine and dentistry to detect abnormalities and fractures and to observe healing tissues, but this worthy detection tool comes with a risk of exposing the patients to excessive radiations. Although X-rays are swift and painless, long-term exposure to their radiations could cause harmful effects including cellular damage. Many new powerful analytical tools have been developed in recent years which can deliver precise results with minimal potential damage to the body tissues. 1 Nuclear magnetic resonance (NMR) was first discovered in the 1940s. 2 The NMR uses the magnetic properties of assured atomic nuclei and is widely being used in physics and chemistry. In dentistry, this technique is predominantly beneficial to explore the structure of amorphous glasses and dental cements, bioactive glasses interaction with oral tissues, identification of salivary metabolites for disease detection, 3 4 and understanding the periodontal diseases by gingival crevicular fluid biomarkers analysis. 5 6 It is also commonly used to review the fluoridation of apatite surfaces in the tooth structure. 7 Therefore, this review is aimed at providing a general overview of the main principles of NMR, types of this technique, and the advantages and disadvantages of NMR spectroscopy. In addition, an insight into the current uses of NMR in the field of medicine and dentistry and ongoing developments of NMR spectroscopy for future applications is discussed.
Basic Mechanism of Action of Nuclear Magnetic Resonance
The basic principles of NMR are that the structural and chemical composition of different substances can be determined by their nuclei, which have their distinctive magnetic field. The basic NMR spectrometer analyzes using a magnetic field and a special detector to assess the changes ( Fig. 1 ). The strength of the external magnetic field causes electrically charged nucleus to move from a lower energy level (E1) to a higher energy level (E2) and the difference between E2 and E1 is symbolized as ΔE which is dependent on the power of the magnetic field and size of the nuclear field moment. 8

Schematic presentation of a typical nuclear magnetic resonance spectrometer showing the relationship of various components (magnet, magnetic field, and detector).
The electromagnetic radiation rhythm attains the NMR signal with a frequency ( v ) causing the nuclei to move to a higher energy level (E1/E2). When this electromagnetic radiation is stopped, it causes the nuclei to relax and accomplish thermal equilibrium. This release of energy from the nuclei is recorded in the form of spectra on the computer, and these spectra are exclusive for every nucleus and are equivalent to the energy levels between the two states (E2/E1) 8 ( Fig. 2 ).

A line in the spectrum is related to a transition between two energy levels (E2 and E1). 9
Nuclear Spin
The protons and neutrons of an atom exhibit spin. In some materials, the protons and neutrons exhibit paired spin such as carbon 12C, oxygen 16O, and sulfur 32S, and in these cases, they cancel each other causing the nucleus not to spin. In some materials, the number of protons and neutrons in an atom are unpaired such as in proton 1 H, phosphorus 31 P, and fluoride 19 F.
Applied Magnetic Field and the Nuclear Moment
The spin of the protons and neutrons creates a magnetic moment in the nucleus of an atom. This magnetic moment could be toward the externally applied magnetic field or away from it. B0 denotes this applied magnetic field. The more desired direction of the nuclear moment is toward the magnetic field which is at lower energy level denoted as α compared with the path opposite to the magnetic field denoted as β 5 . The magnetic field is accountable for retaining the distinction in the energy levels. If it does not happen, all the orientations of the spin of 21 + 1 would be of equal energy. 2
Magnetic Field Strength
NMR requires a magnetic field that is both strong and uniform. The magnetic field strength is measured in Tesla or MHz. The NMR requires a reference nucleus to represent the strength of the magnetic field.
Chemical Shift
The movement of the electrons creates a magnetic field in and around the nucleus. This magnetic field created is different in the direction as compared with the outer magnetic field. Any change in the magnetic field causes a similar change in the spectrum of the NMR. This sum of the shift is controlled by nature of the nucleus and nature of the motion of electrons in its surrounding atoms and molecules. This phenomenon is called “chemical shift (CS).” A reference compound is needed to measure CS 9 and to determine and differentiate magnetically inequivalent nuclei present in a molecule.
Spin–Spin Coupling
The nuclei close to each other induce an incident called spin–spin coupling (SS) due to the difference in nuclei's magnetic field direction. This direction could be either toward or opposing the magnetic field, causing the splitting of NMR signals. This magnetic field direction could either strengthen or fade the signals of NMR signals that can split into two or more components depending upon the specific nuclei having characteristic distance and relative potency. 10
Spin Relaxation (SR)
Spin relaxation is the comeback of energy levels to stability. This occurs due to the loss of resonance signals with the passage of time after releasing the resonance frequency. There are two relaxation processes allowing the nuclear spin to return to stability, which are spin–lattice relaxation and spin–spin relaxation. 11 T 1 represents the time for a specific nucleus to come back to its thermal stability. Several the elements such as structure, molecular mass, temperature, and the solution could influence this procedure. 12 SS relaxation happens when energy is lost from the nuclei/loss of signals. The energy lost is conveyed to nearby spin-active nuclei. SS relaxation time ( T 2 ) is the half-life of this procedure.
Types of Nuclear Magnetic Resonance Spectroscopy
Solid-state NMRs are used for chemical analysis to recognize any changes in the structure during phase transitions and different transformations in a solid state. The main technique frequently used in a solid-state NMR is magic angle spinning (MAS). This magic angle makes the resolution of the sample more precise by making the broader lines of the NMR narrower, 13 resulting in narrower signals giving isotropic values and spinning sidebands to identify the CS of the nuclei for structural determination of solid materials.

Phosphorus Nuclear Magnetic Resonance
In the solid-state NMR, phosphorus is one of the isotopes used to study the molecules and structures of different samples. Compound classes of phosphorus were identified which included orthophosphate diesters, polyphosphate, phosphonates, orthophosphate monoesters, and orthophosphates. 14
Proton Nuclear Magnetic Resonance
Proton is the initial and the most frequent atom to be used in NMR spectroscopy. It is also called hydrogen-NMR ( 1 H-NMR) that provides information about the different varieties of hydrogen present in the molecule and also gives information about its adjacent surroundings. 15 1 H-NMR spectrum of main materials shows small CS range for usual compound is being studied. This CS ranges from +14 to –14 ppm and a broad difference in extent of coupling constant was observed. 16
27 Silicon Magic Angle Spinning Nuclear Magnetic Resonance
Silicon is an essential element, and its 29 Si isotope, which is used in 29 Si-NMR, has 4.70% natural occurrence with the half spin nucleus. It is another spectroscopic technique used to investigate the structures of organic compounds. Its value of the magnetic moment is a little low causing a low resonance frequency. The predominance of 29 Si-NMR shifts is present in a range from +50 to –200 ppm. 17
19 Fluorine Magic angle Spinning Nuclear Magnetic Resonance
Isotopes of fluoride are naturally present in very less quantities except for the 19 F isotope. F-19 is the only constant isotope of fluorine found in large quantities. Due to its good nuclear qualities and a great quantity, it is used in 19 F MAS NMR technique. 19 F-NMR technique is very rapid when compared with 1 H-NMR technique, and without a doubt, 19 F nucleus is one of the most amenable NMR nuclei. Fluorine has a spin of half-nucleus. Its nucleus in molecules is usually encircled by nine electrons, and its binding energy is 147,801 keV. The sensitivity of 19 F-NMR spectroscopy to its CS (to study the fine details of the local surrounding) is much high for fluorine, thus making it very reactive to NMR measurements with an extremely broad CS range. 18 Yesinowski and Mobley 19 verified the capacity of this NMR to differentiate between fluorapatite, fluorohydroxyapatite, and calcium fluoride in massive phase and also on hydroxyapatite surfaces. 19 F-NMR can identify fluoride even in minimal concentrations starting from 0.1%. 19 F-NMR technique has also been used to study the metabolism of drugs containing fluoride. 20
13 Carbon Nuclear Magnetic Resonance Spectroscopy
This technique is a significant tool to recognize carbon atoms in any organic material. It also gives detailed information regarding the chemical structure of the organic compound being studied. 13 C is an isotope of carbon which has a spin quantum number of ½ and is only 1.1% naturally present, and this isotope can be detected by 13 C-NMR. 13 C-NMR is less responsive to carbon in view of the fact that the main isotope of carbon is 12 C, which is not magnetically active; therefore, it cannot be detected through this technique. The intensities of the signals in carbon-NMR are not usually comparative to the number of corresponding 13 C atoms. They are strongly reliant on the numerals of adjacent spins. 21 Magnets utilized in C-NMR have a usual diameter of 10 mm and its usual range of CS is much larger compared with proton NMR. 13 C-NMR can be used to find out the composition of different molecules and is also used in the drug industry to verify drug purity.
27 Aluminum Magic Angle Spinning Nuclear Magnetic Resonance
27 Aluminum MAS-NMR has a natural abundance of 100%, and it has a 5/2 nuclear spin. The nucleus of aluminum is very responsive giving wide lines over the broad range of CS. The main application of this NMR is to identify the existence of aluminum and to observe the probable structural changes of the different varieties of aluminum. In a previous study, 27 Al-NMR has been used to observe alteration of Al (IV) into Al (VI) in the setting glass carbomer cement. 22
Advantages of Nuclear Magnetic Resonance Spectroscopy
Noninvasiveness.
The fundamental quality of NMR is its noninvasiveness. Due to NMR, the studies of biological cells and tissues are now possible without damaging the sample. The fact that both spectrum and imaging can be obtained without destroying the sample is noticeably the greatest advantage of NMR as in in vivo studies. 23
Lack of Ionizing Radiation
Another major advantage of NMR is its lack of ionizing radiation. Many techniques are being used in vivo studies involving ionizing radiations. NMR has made it possible to avoid the exposure from radiation, which could be harmful to both the researcher and the subject. NMR utilizes isotopes which are stable such as carbon-13 to measure metabolic fluxes instead of radioactive compounds. NMR not only minimizes the exposure to observer and subject from the harmful rays but also eliminates the need to dispose of the radioactive tissues and other materials that might be contaminated during the study. 1 Thus, the application of NMR can ensure the safety of the employees as well as reduce experimental cost due to the removal of discarded radioactive substances.
Adjustability
Extensive variety of processes can be investigated through NMR due to the flexibility of the particular technique that can be applied. This technique not only gives information about the physiology of the tissues, but it also gives great images of those tissues. With NMR, a single study can be performed with the same basic technique in both humans and animals, which is important to increase the translation of information. The computed tomography (CT) scan technique can only provide the imaging but not the metabolic or anatomic details, 24 whereas NMR spectroscopy has the capability of acquiring a wide variety of information.
Detailed Structural Analysis
Over the period, NMR has played a major responsibility in determining the mechanisms and chemical connections at a molecular level. This technique has helped to obtain information regarding the minute details about the physical and chemical characteristics of structures. 25 NMR can also analyze the parameters of CS, and it can give details on the local bonding environment around a particular atom, which could be calculated for the extended period of times with NMR. 26 It utilizes the pseudo wave function to get information about large compound structures. 27 NMR has the capability to assist studies of biochemical processes conducted in vivo, which is not efficiently achieved with other imaging techniques. Lee et al 28 proposed that NMR is a better-quality technique as compared with X-ray diffraction in determining the archaeological bone structure.
Disadvantages of Nuclear Magnetic Resonance Spectroscopy
There are a few limitations.
Presence of High Magnetic Field Surroundings Is Essential
An unavoidable outcome when performing the NMR technique is the requirement to perform in a surrounding which has a high magnetic field. The presence of the magnetic field can affect the proper functioning of monitors and computer-controlled devices. If any sharp objects such as a scalpel, scissors, or stapler are present in the NMR magnetic field area, it can get attracted to the magnet field which can cause severe injuries to the workers. Nowadays, monitoring devices used in the magnetic field area are being designed to function properly under the magnetic field. 29 Furthermore, instruments being used in NMR studies are made nonferromagnetic to reduce the problems encountered with high magnetic field surroundings. 30 NMR system cannot be purchased by a single investigator or for single research because of its high cost.
Uses in Medicine and Dentistry
CT scan images of the cranium are restricted by artifacts, but this limitation does not occur with NMR. In the field of medicine, NMR gives the benefit of identifying pediatric tumors, hematomas, and other pathologies. 31 Since multiple sclerosis is a very tricky disease to identify, NMR has become the prime diagnostic device for multiple sclerosis. 17 NMR has particular use for certain body areas such as brain where it produces very detailed and definite images showing delineation between gray and white matter, 32 whereas some tissues such as bone, having low water percentage cannot emit strong signals to create images for NMR. 33 Moreover, NMR is apparently victorious in identifying breast cancer at an early stage. According to a radiologist at Cleveland, a mammogram cannot differentiate between small cancer and a spot, when there are multiple cysts in the breast; however, with NMR, this distinction is possible. 34 NMR technique also gives good images of fatty tissues, and a large quantity of fat creates wonderful images. In addition, the diagnosis of vascular diseases is promising with the NMR 35 as it enables the detailed structural analysis of the surfaces of blood vessels and their abnormalities.
In dentistry, the aim of treatment is to preserve natural tissue and reconstruct the loss tissue with the help of biomaterials. These dental biomaterials are studied by many characterizing machines such as mechanical tester, physical testing, rheological testing, and biocompatibility testing. For that, NMR spectroscopy is a miracle machine to understand in-depth chemical reaction of materials ingredients and their effect with natural tissues. Extensive research on gas ionomer cement (GIC), resin composites, dental bone cements, and periodontal membranes materials has been conducted using the NMR spectroscopy. Prosser et al used NMR spectroscopy and reported the role of tartaric acid in the setting reaction of GIC, was “The fluid cement pastes have shown that tartaric acid reacts more readily than the polyacid with the glass, and hence suppresses the premature gelation of the cement.” 36
The cross-linking of Al in the setting of GIC is very crucial, and upon cement formation, Al ions in the glass are leached out from the surface layer of the glass, which is revealed by solid-state NMR spectroscopy. 37 A novel antimicrobial polymeric dental restorative material was experimentally synthesized to see the biocompatibility, strength, and remineralization property by NMR ( 1 H- and 13 C-NMR) spectroscopy. 38 The advancements of proteomics in dentistry have brought a revolution in the management of oral diseases and analysis of molecular changes during the reconstruction or rehabilitation of oral tissues (soft and hard) with dental materials. 39 To observe the orthodontically induced external apical root resorption biomarkers, Zhou et al studied the 1 H-NMR-based metabolomics and detected the inflammatory metabolites from saliva samples. 40 This study brings an importance of NMR spectroscopy in the field of clinical dentistry and dental early diagnosis. Another study on salivary metabolomics has reported the identification of several metabolic signatures from the control and sarcoidosis patients. 41 The noninvasive, easy, and low-cost sampling of the human saliva attracted it as a diagnostic oral fluid, and by these omic devices such as NMR, more biomarkers can be explored. 42
Conclusions
Considering the potential advantages of the NMR technique, it can be concluded with authority that it has become a preferred choice of technique for any diagnosis, treatment planning, maintenance of treatment and also to see the behavior of foreign materials interaction with the human body. NMR is still a growing technology, and it is being anticipated that few discoveries are now just around the corner.
Financial Support and Sponsorship
Conflicts of Interest None declared.
Backbone assignment of CcdB_G100T toxin from E.coli in complex with the toxin binding C-terminal domain of its cognate antitoxin CcdA
- Bahnikana Nanda
- Jayantika Bhowmick
- Siddhartha P. Sarma
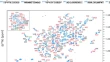
The backbone NMR resonance assignments of the stabilized E. coli β clamp
- Socheata Lim
- Dmitry M. Korzhnev
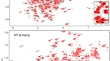
The 1 H, 15 N, and 13 C resonance assignments of a single-domain antibody against immunoglobulin G
- Vanessa Bezerra de Oliveira Leite
- Rafael Alves de Andrade
- Marcius da Silva Almeida
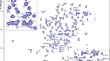
NMR 1 H, 13 C, 15 N backbone resonance assignments of 14-3-3ζ binding region of human FOXO3a (residues 1-284)
- Shota Enomoto
- Shoichi Nakatsuka
- Masanori Osawa
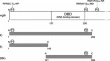
1 H, 13 C and 15 N backbone resonance assignment of the calcium-activated EndoU endoribonuclease
- Florian Malard
- Fedor V. Karginov
- Sébastien Campagne
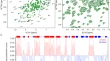
Solution NMR backbone assignment of the N-terminal tandem Zα1-Zα2 domains of Z-DNA binding protein 1
- Lily G. Beck
- Jeffrey B. Krall
- Beat Vögeli
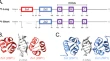
NMR-based solution structure of the Caulobacter crescentus ProXp-ala trans -editing enzyme
- Antonia D. Duran
- Eric M. Danhart
- Mark P. Foster
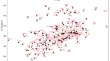
Solution NMR backbone resonance assignment of the full-length resistance-related calcium-binding protein Sorcin
- Kathleen Joyce Carillo
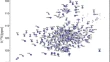
Chemical shift assignments of the α-actinin C-terminal EF-hand domain bound to a cytosolic C0 domain of GluN1 (residues 841–865) from the NMDA receptor
- Johannes W. Hell
- James B. Ames
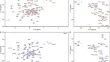
1 H, 15 N and 13 C resonance assignments of eggcase silk protein 3
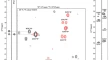
1 H, 15 N, and 13 C resonance assignments of the N-terminal domain and ser-arg-rich intrinsically disordered region of the nucleocapsid protein of the SARS-CoV-2
- Peter R. Bezerra
- Ariana A. Vasconcelos
- Fabio C. L. Almeida
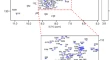
1 H, 13 C and 15 N resonance assignments of a shark variable new antigen receptor against hyaluronan synthase
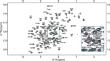
Manual and automatic assignment of two different Aβ40 amyloid fibril polymorphs using MAS solid-state NMR spectroscopy
- Natalia Rodina
- Riddhiman Sarkar
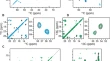
1 H, 13 C and 15 N assignment of self-complemented MrkA protein antigen from Klebsiella pneumoniae
- Valentina Monaci
- Gianmarco Gasperini
- Francesca Cantini
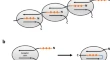
Solid-state NMR assignment of α-synuclein polymorph prepared from helical intermediate
- Sahil Ahlawat
- Surabhi Mehra
- Vipin Agarwal
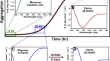
Solid-state NMR backbone chemical shift assignments of α-synuclein amyloid fibrils at fast MAS regime
- Zigmantas Toleikis
- Piotr Paluch
- Alons Lends
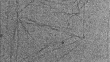
Backbone and methyl side-chain resonance assignments of the Fab fragment of adalimumab
- Muzaddid Sarker
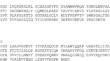
Assignment of the Lassa virus transmembrane domain in the prefusion and postfusion states in detergent micelles
- Patrick M. Keating
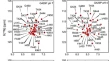
NMR resonance assignment of the cell death execution domain BELL2 from multicellular bacterial signalosomes
- Loic Delcourte
- Corinne Sanchez
- Antoine Loquet
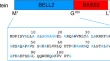
Backbone NMR resonance assignments for the VP1u N-terminal receptor-binding domain of the human parvovirus pathogen B19
- Maria Luiza Caldas Nogueira
- Renuk Lakshmanan
- Joanna R. Long
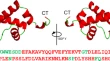
1 H, 13 C and 15 N backbone resonance assignment of Cel45A from Phanerochaete chrysosporium
- Laura Okmane
- Mats Sandgren
- Gustav Nestor
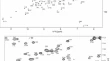
Backbone triple resonance assignments of the dimerization domain of NF-kappaB p52 subunit
- Sunirmala Sahoo
- Nitin Dhaka
- Sulakshana P. Mukherjee
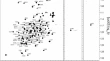
Resonance assignments of cytochrome MtoD from the extracellular electron uptake pathway of sideroxydans lithotrophicus ES-1
- Anaísa Coelho
- José M. Silva
- Catarina M. Paquete
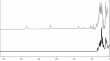
NMR chemical shift assignment of Drosophila odorant binding protein 44a in complex with 8(Z)-eicosenoic acid
- Myriam L. Cotten
- Mary R. Starich
- Nico Tjandra
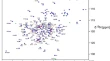
Backbone and methyl side-chain resonance assignments of the single chain Fab fragment of trastuzumab
- Donald Gagné
- James M. Aramini
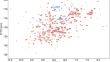
1 H, 13 C, and 15 N resonance assignments of the La Motif of the human La-related protein 1
- Benjamin C. Smith
- Robert Silvers
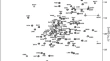
1 H, 15 N and 13 C resonance backbone and side-chain assignments and secondary structure determination of the BRCT domain of Mtb LigA
- Jayanti Vaishnav
- Ravi Sankar Ampapathi
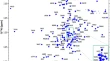
Chemical shift assignment of dsRBD1 and dsRBD2 of Arabidopsis thaliana DRB3, an essential protein involved in RNAi-mediated antiviral defense
- Jaydeep Paul
- Mandar V. Deshmukh
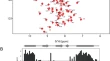
Solution NMR chemical shift assignment of apo and molybdate-bound ModA at two pHs
- Hiep LD Nguyen
- Karin A. Crowhurst
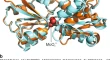
1 H, 13 C, and 15 N backbone and methyl group resonance assignments of ricin toxin A subunit
- Shibani Bhattacharya
- Tassadite Dahmane
- Nilgun E. Tumer
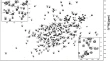
Backbone chemical shift and secondary structure assignments for mouse siderocalin
- Johanna Moeller
- Nina G. Bozhanova
- Clara T. Schoeder
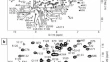
1 H, 13 C, and 15 N resonance assignments and solution structure of the N-terminal divergent calponin homology (NN-CH) domain of human intraflagellar transport protein 54
- Kanako Kuwasako
- Weirong Dang
- Yutaka Muto
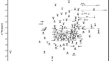
1 H, 13 C and 15 N backbone and side-chain resonance assignments of the human oncogenic protein NCYM
- Assia Mouhand
- Kazuma Nakatani
- Christian Roumestand
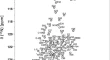
1 H, 15 N and 13 C resonance assignments of S2A mutant of human carbonic anhydrase II
- Himanshu Singh
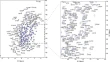
Backbone 1 H, 13 C and 15 N resonance assignment of the ubiquitin specific protease 7 catalytic domain (residues 208–554) in complex with a small molecule ligand
- Maya J. Pandya
- Wojciech Augustyniak
- Martin J. Watson
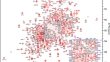
Backbone 1H, 13C, and 15N chemical shift assignments for human SERF2
- Bikash R. Sahoo
- Vivekanandan Subramanian
- James C.A. Bardwell
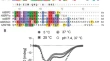
1 H, 13 C and 15 N backbone resonance assignments of hepatocyte nuclear factor-1-beta (HNF1β) POU S and POU HD
- Sayaka Hokazono
- Eri Imagawa
- Tsuyoshi Konuma
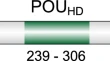
NMR study of the structure and dynamics of the BRCT domain from the kinetochore protein KKT4
- Patryk Ludzia
- Hanako Hayashi
- Christina Redfield
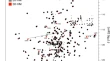
Chemical shift assignments of the ACID domain of MED25, a subunit of the mediator complex in Arabidopsis thaliana
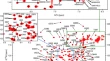
NMR 1 H, 13 C, 15 N backbone resonance assignments of wild-type human K-Ras and its oncogenic mutants G12D and G12C bound to GTP
- Chunhua Yuan
- Alexandar L. Hansen
- Rafael Brüschweiler
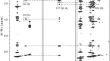
13 C and 15 N resonance assignments of alpha synuclein fibrils amplified from Lewy Body Dementia tissue
- Alexander M. Barclay
- Dhruva D. Dhavale
- Chad M. Rienstra
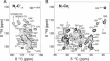
Chemical shift assignments of the catalytic domain of Staphylococcus aureus LytM
- Helena Tossavainen
- Ilona Pitkänen
- Perttu Permi
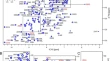
Correction to: NMR resonance assignments of 18.5 kDa complex of Arabidopsis thaliana DRB7.2:DRB4 interaction domains
- Sneha Paturi
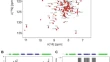
Backbone NMR resonance assignments for the C terminal domain of the Streptococcus mutans adhesin P1
- Emily-Qingqing Peng
- M. Luiza Caldas Nogueira
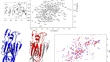
Assignment of the disordered, proline-rich N-terminal domain of the tumour suppressor p53 protein using 1 H N and 1 H α -detected NMR measurements
- Fanni Sebák
- Péter Ecsédi
- Andrea Bodor
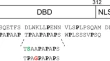
Solution NMR assignments and structure for the dimeric kinesin neck domain
- Richard A. Kammerer
- Andrei T. Alexandrescu
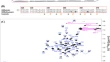
1 H, 15 N, 13 C resonance assignments for proteasome shuttle factor hHR23a
- Kylie J. Walters
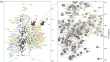
Solution-state NMR assignment and secondary structure analysis of the monomeric Pseudomonas biofilm-forming functional amyloid accessory protein FapA
- Chang-Hyeock Byeon
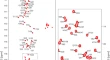
Chemical shift assignments of wildtype human leptin
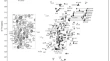
Backbone 1 H, 15 N and 13 C resonance assignments for an E2 ubiquitin conjugating enzyme-UBE2T
- Qiwei Huang
- CongBao Kang
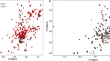
- Find a journal
- Publish with us
- Track your research

IMAGES
VIDEO
COMMENTS
H NMR spectrum should be integrated. Chemical shift values should be included. 2.5 The solvent peak should be clearly labeled on the spectrum. 2.6 All peaks should be visible on the spectrum. Insets are encouraged to show expanded regions. At minimum, the spectral window should be 1 ppm to 9 ppm - for 1 H NMR and -10 ppm to 180 ppm for 13 C NMR.
Journal abbreviation: Biomolecular NMR assignments. The abbreviation of the journal title "Biomolecular NMR assignments" is "Biomol.NMR Assign.". It is the recommended abbreviation to be used for abstracting, indexing and referencing purposes and meets all criteria of the ISO 4 standard for abbreviating names of scientific journals.
ISO4 Abbreviation of Biomolecular NMR Assignments. ISO 4 (Information and documentation - Rules for the abbreviation of title words and titles of publications) is an international standard, defining a uniform system for the abbreviation of serial publication titles. One major use of ISO 4 is to abbreviate the names of scientific journals.
Overview. Biomolecular NMR Assignments is a dedicated forum for publishing sequence-specific resonance assignments for proteins and nucleic acids. Provides an avenue for depositing these data into a public database at BioMagResBank. Assignment Notes are published in biannual editions in June and December. No page charges or fees for online ...
Nuclear magnetic resonance (NMR) is a physical phenomenon in which nuclei in a strong constant magnetic field are disturbed by a weak oscillating magnetic field (in the near field [1]) and respond by producing an electromagnetic signal with a frequency characteristic of the magnetic field at the nucleus. This process occurs near resonance, when ...
Keep lettering consistently sized throughout your final-sized artwork, usually about 2-3 mm (8-12 pt). Variance of type size within an illustration should be minimal, e.g., do not use 8-pt type on an axis and 20-pt type for the axis label. Avoid effects such as shading, outline letters, etc.
Step 1: ¹H-NMR. The first step in structural characterization is 1-dimensional proton ¹H-NMR. The chemical shift, multiplicity, coupling constants, and integration are all factors to consider when assigning protons. In this example, only three protons can be assigned by the proton spectrum alone: protons 3, 4, and 6. Chemical Shift (ppm)
Assignment Notes documenting assignments of proteins by solid-state NMR spectroscopy are encouraged: use of solid-state NMR is an acceptable justification for assignments of cooperatively folded proteins of less than 100 residues. Complete backbone assignments and complete or nearly complete side chain assignments are required.
Explore popular shortcuts to use Biomolecular NMR Assignments abbreviation and the short forms with our easy guide. Review the list of 1 top ways to abbreviate Biomolecular NMR Assignments. Updated in 2017 to ensure the latest compliance and practices
Solution NMR. Assignment Theory. Visualising 3D Spectra; Triple Resonance Backbone Assignment ... Glossary / Abbreviations. 2D two-dimensional. 3D three-dimensional. Double Labelled Shorthand for protein which has been uniformly 15 N- and 13 C-labelled. Double Resonance NMR spectroscopy using two NMR-active nuclei. For proteins this usually ...
Although automated assignment methods are desirable and work toward this goal is ongoing, interactive methods remain the standard for much NMR assignment. Although interactive analysis is aided by the display of peak-boxes associated with each measured peak, which can display known assignments or other annotations, the tracking of thousands of ...
Much space and discussion is devoted to practical aspects. The implementation of protein NMR assignment is described using the program CCPNmr Analysis. This program has been developed by CCPN and actively seeks input from the NMR community. CCPNmr Analysis is based on the detailed and well thought-out CCPN Data Model which has the advantage (a ...
A novel approach for sequential assignment of proton, carbon-13, and nitrogen-15 spectra of larger proteins: heteronuclear triple-resonance three-dimensional NMR spectroscopy. Application to ...
1. Background. Over the past fifty years nuclear magnetic resonance spectroscopy, commonly referred to as nmr, has become the preeminent technique for determining the structure of organic compounds. Of all the spectroscopic methods, it is the only one for which a complete analysis and interpretation of the entire spectrum is normally expected.
Nuclear Magnetic Resonance (NMR) spectroscopy is a major technique in structural biology with over 11,800 protein structures deposited in the Protein Data Bank. NMR can elucidate structures and ...
It is also called hydrogen-NMR ( 1 H-NMR) that provides information about the different varieties of hydrogen present in the molecule and also gives information about its adjacent surroundings. 15 1 H-NMR spectrum of main materials shows small CS range for usual compound is being studied. This CS ranges from +14 to -14 ppm and a broad ...
Article 31 August 2024. Chemical shift assignments of the α-actinin C-terminal EF-hand domain bound to a cytosolic C0 domain of GluN1 (residues 841-865) from the NMDA receptor. Aritra Bej. Johannes W. Hell. James B. Ames. Article Open access 29 August 2024. 1 H, 15 N and 13 C resonance assignments of eggcase silk protein 3.
A further recent innovation is to make NMR signal shapes differ between amino acids by supplying isotopically patterned pyruvate during protein expression in genetically engineered bacteria ().Because biosynthesis paths differ for the 20 natural amino acids (), so do the isotope patterns in their NMR spectra.This radically simplifies signal assignment but still requires a human to look at the ...