- Regular article
- Open access
- Published: 10 February 2023

Novelty and cultural evolution in modern popular music
- Katherine O’Toole ORCID: orcid.org/0000-0001-6653-1486 1 &
- Emőke-Ágnes Horvát 1
EPJ Data Science volume 12 , Article number: 3 ( 2023 ) Cite this article
4174 Accesses
2 Citations
9 Altmetric
Metrics details
The ubiquity of digital music consumption has made it possible to extract information about modern music that allows us to perform large scale analysis of stylistic change over time. In order to uncover underlying patterns in cultural evolution, we examine the relationship between the established characteristics of different genres and styles, and the introduction of novel ideas that fuel this ongoing creative evolution. To understand how this dynamic plays out and shapes the cultural ecosystem, we compare musical artifacts to their contemporaries to identify novel artifacts, study the relationship between novelty and commercial success, and connect this to the changes in musical content that we can observe over time. Using Music Information Retrieval (MIR) data and lyrics from Billboard Hot 100 songs between 1974-2013, we calculate a novelty score for each song’s aural attributes and lyrics. Comparing both scores to the popularity of the song following its release, we uncover key patterns in the relationship between novelty and audience reception. Additionally, we look at the link between novelty and the likelihood that a song was influential given where its MIR and lyrical features fit within the larger trends we observed.
1 Introduction
When NWA dropped their hit song, ‘Straight Outta Compton’, it was one of the hottest new tracks of 1988, but in fact, a key component of the song hearkened all the way back to 1969. By incorporating a sample of the famous ‘Amen Break’ drum solo from The Winstons’ song ‘Amen Brother’, the song is an example of the way that musical traits can persist even as they undergo change and reinvention. This juxtaposition highlights the paradox that make culture so fascinating; it provides us with a foundation of established aesthetics and practices to draw from, even as it continues to change and evolve. This dynamic balance between established norms, and the introduction of novelty provides a rich area of inquiry for cultural analysis that looks not only at the impact of novelty on patterns of commercial production and consumption, but also at how the introduction of novel creative artifacts drives cultural evolution [ 1 – 6 ]. With the rise of digital media, information about the consumption and production of cultural artifacts is available to us at unprecedented scale. In addition, the digitization of artifacts allows us to apply computational analyses to better understand the often nebulous concepts of creativity and novelty, and unlock insights into their effects on cultural change. With music in particular, the availability of digital data, and advances in computational methods of audio analysis have made it possible to investigate these questions at scale. The ubiquity of popular music also means that established markers of success, such as Billboard charts, are based on the opinions of a large population. Additionally, while music styles vary across different genres and cultures, music is a ‘human universal’ found in virtually all societies, and displays organizational properties that we can track over time in order to analyze patterns of cultural change [ 1 , 3 ].
Currently it is possible to extract quantitative metrics from large music data sets using Music Information Retrieval (MIR) software. This data, referred to as audio features, or audio descriptors, is information that can be extracted from audio signals, and can be roughly classified as low-level and high-level features. Low-level features directly describe the audio signal data, for example spectral descriptors, while high-level features typically describe more holistic information about the song such as key, energy level, or danceability [ 7 , 8 ]. Previous work has demonstrated that these high level MIR features provide accurate and robust data for modeling musical preference [ 7 , 9 ], along with comparisons of content similarity that inform automatic genre classification [ 10 , 11 ]. This has enabled researchers to contextualize songs within the larger musical ecosystem they exist in, and to identify long term trends in how genres and styles evolve over time [ 1 – 5 , 12 ]. This data is also used by streaming services such as Spotify to develop music discovery tools and generate recommendations for users. In addition to MIR data, word and document embeddings of lyrics have also been shown to be a rich source of data for music content analysis, including genre and mood classification [ 13 – 17 ]. As with MIR features, these vector embeddings can be used to evaluate the similarity between lyrics of different songs [ 18 – 20 ].
In combination with data on commercial success and popularity, MIR and lyric data has enabled researchers to examine how the novelty of songs correlates with their success. For both MIR features and lyrics, the novelty of a song relative to its peers has been found to play a role in determining its cultural success, with the most popular songs demonstrating an optimal level of differentiation that allows them to stand out without being perceived as too dissimilar [ 21 – 23 ]. Identifying these patterns of optimal differentiation in consumer preferences is important for both the music industry at large, and for development of recommender systems [ 24 ]. However, previous work has still looked at MIR and lyrical novelty separately, and there is a lack of understanding as to how the relationship between these two dimensions of novelty might affect listeners perception of overall song novelty, and the success of the song. Work in genre and mood classification has shown MIR and lyric data to be complementary, with the inclusion of both sets of features having a positive impact on classification accuracy [ 13 , 16 , 25 , 26 ]. Since both of these components contribute to the overall perception of the song’s mood and genre, we propose to study whether there is also a relationship between a song’s MIR novelty and its lyrical novelty, and if that relationship influences its performance on the Billboard Hot 100 chart. Additionally, we consider an alternative definition of success in terms of how likely it was that the song exerted some degree of stylistic influence on the cultural ecosystem. This has been done in previous studies with classical music by tracking the reappearance of specific motifs or harmonic patterns, however the granularity required in this type of analysis makes it difficult to scale [ 4 ]. By using MIR and lyric features though, it is possible to perform this type of analysis at scale by using the similarity measures between a song and later releases to determine the likelihood that the song in question was influential [ 27 ]. In doing so, we can examine whether a song’s novelty and initial success affect its likelihood of being influential in the long term, and gain insight into how the introduction of novel attributes fuels ongoing creative evolution in modern popular music.
In this paper, using MIR and lyric feature data from Billboard Hot 100 songs between 1974-2013, we calculated novelty scores for each song relative to its genre and release year, and compared these to the total number of weeks the song spent on the Hot 100 chart. We found that the novelty scores at which optimal differentiation occurred were quite similar for both MIR and lyrics, and the most successful songs where those that were optimally differentiated for both. When looking at the probability of a song being influential, we also observed optimal differentiation occurring with respect to the novelty scores. Additionally, we found that there was no correlation between the time the song spent on the chart, and its probability of being influential. Rather, we found that for different novelty scores, the amount of time the song spent on the chart affected its likelihood of being influential. By utilizing computational data and methodology to extract high level patterns of change within the musical ecosystem, this research highlights the importance of considering alternate metrics for evaluating success when studying cultural artifacts by providing insight into how novelty affects both short and long term performance of cultural artifacts.
2 Related literature
2.1 novelty metrics.
The word novelty is used to describe ideas or artifacts which are new, original, and in some way dissimilar and different to what came before [ 28 , 29 ]. The production of novelty is important for innovation. Thus, understanding how novelty occurs is a salient question across many domains[ 30 ], including the sciences [ 29 , 31 , 32 ], and creative industries such as music[ 33 ], film[ 34 ], fashion [ 6 ], and literature [ 35 ]. Novelty can be evaluated in terms of how similar or dissimilar an artifact is when compared to other artifacts within the larger cultural context, which allows examining its relationship to the cultural space it is embedded in[ 36 ].
One way this can be achieved is by constructing feature representations that capture information about the key attributes of the artifacts. By representing each artifact based on set of features, this allows us to map individual artifacts to a shared multidimensional feature space and compare them to one another based on their relative positioning. It is then possible to use a distance metric as a way of measuring how similar or dissimilar artifacts are from one another[ 18 – 23 , 35 , 37 ].
Previous work with music similarity has utilized MIR features to model individual songs as feature vectors due to the ability of MIR features to capture perceptually relevant audio information that has been validated against human perceptions of audio similarity [ 21 , 22 , 38 ].
This feature representation approach can also be applied to textual data. Previous research into lyrical novelty used Latent Dirichlet Allocation (LDA) to identify latent topics based on word co-occurence, and represent individual songs based on their topic composition [ 23 ]. A similar approach using LDA has also been applied to research into fan fiction, with the Jensen-Shannon Distance between the topic representations of artifact being used to calculate their relative similarity [ 35 ]. The development of word embedding models means that text can also be represented as a feature vector. These models use textual training data to analyze word usage and map each word to a position in multidimensional feature space based on the context in which they are used, allowing us to compare the similarity of words by compare the relative positions of their vectors to one another [ 19 ].
For example, the vectors for the words ‘sad’ and ‘morose’ would be closer to one another in the vector space than the vectors for the words ‘sad’ and ‘happy’. This can also be extended to map longer text inputs such as paragraphs or entire documents to single vectors. This approach has been leveraged in previous work on the analysis of patent novelty, where document embedding models have been used to generate feature vector representations for patents, allowing the cosine similarity between them to be calculated [ 20 ]. There are also domain specific models that can be used for document embeddings, such as BioBERT, which as has similarly been used to assess the relative novelty of PubMed Articles by generating document feature vectors [ 37 ].
The benefit of this approach to calculating novelty is that mapping artifacts to a shared feature space allows us to contextualize our measurement of novelty within the larger domain context. A challenge with measuring novelty is that what is considered novel is always changing, and to assess whether or not an artifact is novel, we must contextualize it by comparing it to its contemporaries [ 12 , 39 , 40 ]. By mapping artifacts to a shared feature space and using distance metrics to evaluate similarity, we are able to identify novel artifacts that are informed by this context, without first needing to identify specific markers of novelty.
2.2 Novelty and success
Previous research from psychological studies of culture has suggested that the novelty of cultural artifacts impacts how favorably they will be perceived by audiences [ 23 ]. At the individual level, the relationship between subjective novelty and enjoyment has been modeled as an inverse U-curve [ 41 , 42 ]. In this model, objects that are too familiar or too novel will be less successful, with there being a ‘comfort zone’ that describes the desired amount of novelty. When considering large scale consumption, we also see that competition for audience attention means that artifacts needs some degree of novelty to stand out, however audiences are also shown to be averse to very high levels of novelty as well [ 43 ]. In studies of scientific research, a bias against highly novel work has been observed, with very novel work being less likely to be initially recognized and successful, even in cases where high levels of success are achieved in the long term [ 44 ]. Multiple sociological studies exploring this idea across other domains have also found that there appears to be a certain degree of novelty that allows individual artifacts to stand out from their peers, referred to as ‘optimal differentiation’ [ 21 , 22 ]. The idea behind optimal differentiation in the music industry is that although songs must be similar enough to previous work to maintain cohesion in the cultural schema, there must be the introduction of new elements that innovates on the established genre norms and sets them apart, without straying too far out of that comfort zone.
Within the music industry, there are of course many factors that influence the likelihood of a song’s success. While it is impossible to control for all of these, previous work has demonstrated that the relationship between an artifact’s novelty and their likelihood of success is still significant. Previous research on lyric differentiation found that the degree of differentiation had a significant effect on the ranking of a song on the Billboard digital downloads list even when controlling for amount of radio airplay, artist, and specific lyrical topics [ 23 ]. Additionally, research on MIR feature differentiation also found that the effect of differentiation on the amount of time a song spent on the Billboard Hot 100 chart remained significant when controlling for artist popularity in terms of how many times the artist had previously charted, genre preferences, and variations in amount of institutional support that artist received based on whether there were with a major or independent music label[ 21 , 22 ]. In this paper, we build on this previous research to examine whether we can observe a relationship between lyric novelty and MIR novelty at the individual song level, as well as whether the relationship between these different types of novelty also correlates with patterns of commercial success.
2.3 Cultural evolution
We can also think of success from the perspective of impacting cultural evolution. We know that over time, musical styles and genres evolve, and their defining characteristics change. As novel artifacts are introduced into the wider cultural ecosystem, they bring new ideas and creative perspectives, which may or may not be incorporated into the existing stylistic norms[ 36 ]. Studies have shown that we can quantitatively track this evolution over time by analyzing changes to the presence and frequency of musical features over time [ 1 – 4 ]. We can therefore see whether the features of a given artifact become more or less prominent in the style as a whole over time. If later artifacts are very similar to the artifact in question, this tells us that many of the artifact’s features have been incorporated into the stylistic norms, and therefore the artifact is more likely to have been stylistically influential. Although it is not possible to prove a causal relationship, measuring the degree of similarity between a cultural artifact and other artifacts produced at a later time is a standard approach for inferring potential influence [ 27 ]. Although novelty plays an important role in fueling stylistic evolution, we are lacking empirical evidence about the correlation between the degree of novelty in an artifact, and how likely it is that the artifact will be influential.
3 Research questions
Based on the above gaps in the literature, we aimed to answer the following research questions:
RQ 1: What is the relationship between a song’s MIR novelty and its lyric novelty?
RQ 2: Does the relationship between a song’s MIR novelty and lyric novelty impact its likelihood of success?
RQ 3: Does the MIR novelty and/or lyric novelty of a song impact the probability of the song being influential?
RQ 4: How does the amount of change over time to the average MIR features compare to the amount of change over time to the average lyric features?
Our data comprises songs from the Billboard Hot 100 chart, which tracks the 100 most popular songs in the United States for each week based on Nielsen radio play scores, physical and digital music sales, along with streaming figures. It therefore serves to track the commercial success of individual songs. For the purposes of our analysis, we measured the success of each song at the time of their initial release based on how many weeks it had been included on the Hot 100 Chart. More time spent on the chart was therefore indicative of higher degrees of success. The Billboard Hot 100 chart is an industry standard for measuring song popularity, and has been used in numerous studies on popular music due to their reliable insight into the most popular American music at a given time [ 22 ]. This data set allowed us to limit our analysis to only popular music that was most representative of the prevailing cultural space at each point in time. The data set included song genre from Discogs.com, the total number of weeks each song spent on the chart, and MIR feature data. We acquired text of the lyrics for each song from a variety of online sources with our custom scraping tools. The subset of the data used in our analysis consisted of 14,248 songs that were on the Billboard Hot 100 chart between 1974-2013, encompassing 3,973 unique artists and bands across 643 different record labels and 17 genres.
5 Methodology
In Figure 1 we have included an illustration of the data processing pipeline used to generate the metrics used for our analyses.
Extract MIR and Lyric Features: MIR and lyric features represent dimensions that define an MIR feature space and a lyric feature space, respectively. The feature values we derived for each song provide us with a vector that maps that song to these multi-dimensional feature spaces. This allows us to compare the aural and lyrical similarity of sets of songs based on the relative positions of their MIR feature vectors, and the relative positions of their lyric feature vectors.
Calculate Song Novelty: Since the novelty of a song is assessed relative to the other songs released in the same time period, we can group the songs based on the year they were released. We also choose to only generate within-genre novelty comparisons, as the stylistic variation between genres means that cross-genre comparisons would not give us a good measure of novelty. We can then group the songs by genre and release year and calculate an MIR novelty score and a lyric novelty score for each individual song, based on the average distance between the song’s vector and the other song vectors that were released in the same year and genre. For example, when calculating the novelty of a rock song released in 1985, we would compare it to the other rock songs that were also released in 1985. The farther away an individual song’s vector is from the average position of the song vectors in that subset, the more novel that song is.
Calculate Song Relative Novelty: Once we have computed the initial novelty score of a song, relative to the year and genre it was released in, we can calculate how novel it is compared to songs that are in the same genre, but were released in a later year. This gives us a score which shows the relative novelty of the song when compared to a given year. For example, if a Rock song was released in 1982, we could calculate its relative novelty with respect to 1985 by finding the average distance between its feature vectors, and the feature vectors of all the Rock songs that were released in 1985.
Calculate Influence Probability: We can calculate the change in relative novelty by subtracting the song’s relative novelty score from its initial novelty score. If there is an increase in relative novelty, it is not likely that the song was influential. If there is a decrease in relative novelty, it is more likely the song was influential.
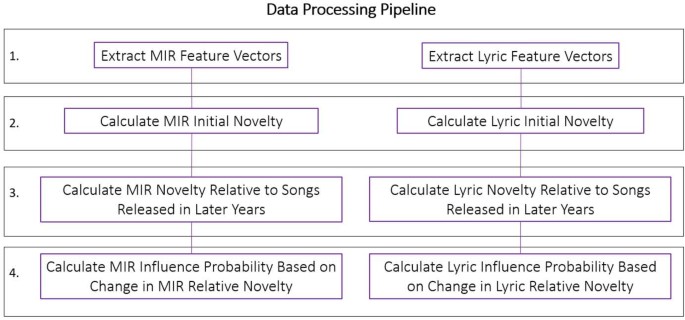
Each of the steps in our data processing pipeline used to generate the MIR and lyric song novelty metrics used in our analyses
5.1 Feature extraction
The MIR features used in our analysis consisted of quantitative data for 13 high level MIR features which were derived from The Echo Nest using their Music Information Retrieval (MIR) system. A description of the MIR features can be found in Table 5 in the Appendix .
The lyrics features were generated using a document embedding system. We cleaned, preprocessed, and tokenized the lyrics using the Gensim simple preprocessing utility [ 45 ]. We then trained a Doc2Vec model which had a vector of 100 dimensions and iterated over the training corpus 40 times [ 19 ]. The minimum word count was set to 2 in order to discard words with a single occurrence. This model was then used to generate a 100 length feature vector for the lyrics of each individual song. Unlike the MIR features, the lyric features do not map to concrete concepts, however all together they define a feature space where we can compare how relatively similar the contents of two documents are by looking at how close their vectors are.
5.2 Novelty scores
To generate our novelty scores, we calculated the distance between each individual song vector, and the other song vectors within the same genre and release year. We opted to use a distance metric when quantifying the change in average genre positioning, and the individual song novelty scores, as opposed to cosine similarity which was used in previous studies. This allows us to track how much the average position of a genre’s feature vectors is changing over time for both MIR features and lyric features, in addition to measuring the individual song novelty scores.
For the lyric vector distances, we used Euclidean distance, as there was no significant covariance between any of the individual features that comprised the feature vectors. For each song, the lyric feature vector can be written as:
For each genre-year group of songs, we can then take the component-wise average across all the individual song vectors to calculate the average feature vector for that genre-year group, which can be written as:
We then calculate the Euclidean distance between this average vector, and the individual song vectors for each song within the genre-year group:
For the MIR vector distances, we followed a similar approach. With MIR data however, some feature values are used as input for determining the values of other features, which means that there are covariances between the features. For example when calculating the danceability of a song, tempo and valence values are included. As a result, we cannot use the Euclidean distance, and instead need to use the Mahalanobis distance. The Mahalanobis distance is similar to Euclidean distance, but is calculated using the covariance matrix of all of the feature vectors, so that it can account for any dependencies between the features, and scale the distance accordingly.
For each song, the MIR feature vector can be written as:
We again take the average of all the song vectors within the genre-year group in order to yield the average MIR feature vector:
We then calculate the covariance matrix, C for the MIR feature vectors, which gives us the covariance between each pair of MIR features included in our feature vectors. In order to be consistent when calculating the within-genre distances for different years, a covariance matrix was generated for each genre using data from all years, and used in the distance calculations, rather than generating a covariance matrix for each genre-year subset. Because covariances between MIR features mainly vary across genres, but are fairly consistent within genre over time, this allowed us to make sure that the normalization applied by the Mahalanobis distance calculation was consistent across all the subsets of a genre, allowing us to compare different time periods.
The Mahalanobis distance \(D_{M}\) between an individual song song m⃗ and the rest of the song vectors in the same genre-year group can then be calculated as follows:
Calculating the individual song distances yields a distribution of MIR vector distances and a distribution of lyric vector distances for each of the genre-year subsets. In Table 1 we have included the total number of songs for each year, as well as the average MIR and lyric vector distances across all genres for each year.
In order to compare song novelty between songs from different years and genres, we then normalize each song’s vector distance relative to the mean distance and standard deviation of the distances for all the songs within the same genre-year subset. This allows us account for variations in the distributions of distances within each genre-year subset. We do this by calculating the z-score for each individual vector distance. The z-score tells us the relative positioning of a vector distance within a distribution by subtracting the mean distance of the distribution, and then dividing the difference by the standard deviation of the distribution. In doing so, the z-score tells us how many standard deviations from the mean that particular value is, which indicates how novel the vector distance is, and allows us to compare it to novelty scores drawn from different distributions.
5.3 Relative novelty scores
In order to evaluate the likelihood that a song was influential, we have to compare it to the cultural ecosystem at later points in time. To compare a song’s similarity to songs released in later years, we can use the same approach that we took for calculating the novelty scores, but instead of comparing the song to songs in the same genre-year subset, we compare it to songs in the same genre, but released in a later year.
Not all of the genres in our dataset had songs included on the Hot100 chart for every year within the time period we looked at, meaning there were a large number of relative novelty scores that could not be calculated. Because of this, we limited our analysis to the 6 genres with the greatest number of songs; Rock, R&B, Rap, Country, Pop, and Electronica. For each song within these genres, we compared it to the genre-year subsets of the subsequent ten years following the song’s release. For example, if a Rock song was released in 1982, we would compare its feature vector to the average feature vector of Rock songs that were released in 1983, 1984, 1985 and so on. Using the same process as we used for calculating the initial novelty scores, we calculated the Euclidean distance for the lyric vector distances, and the Mahalanobis distance for the MIR vector distances. Again, in order to account for variations in the mean distance and total range of distances within different genre-year distributions, we calculated the z-score for each of the 10 relative vector distances that had been calculated for each song. For each relative vector distance, this was done using the mean and standard deviation of the distances in the genre-year subset that had been used for that specific relative comparison. This yielded relative novelty scores that we could then compare to the relative novelty scores for other years, and to the initial novelty score.
5.4 Influence probability
We can determine whether or not it was probable that a song was influential or not based on whether its relative novelty score had increased or decreased in relation to its initial novelty score. Since we evaluate the relative likelihood that a song was influential by calculating the change in both its MIR and lyric relative novelty, we first determined whether the rate at which relative novelty changed was consistent over time. Taking the average change in relative song novelty in the years following its initial release, we found that the rate of change for MIR relative novelty plateaued after 2 years, and the rate of change for lyric relative novelty plateaued after 3 years (see Figure 2 left plots). Because of this, we decided to only consider the relative novelty change that occurred in the 3 years following a song’s release by taking the average of the relative novelty scores for those first three years, and subtracting the song’s initial novelty score. Songs released in 2011 or later were excluded since we did not have data for the full three years following those release years. For example, for Prince’s, ‘When Doves Cry’, the average change in MIR relative novelty was an increase of 0.15, and for lyric relative novelty it was an increase of 0.12. Because the relative novelty increased, this tells us that between 1985 and 1987, the average MIR and lyric features of the Rock genre became more dissimilar to the features seen in ‘When Doves Cry’.
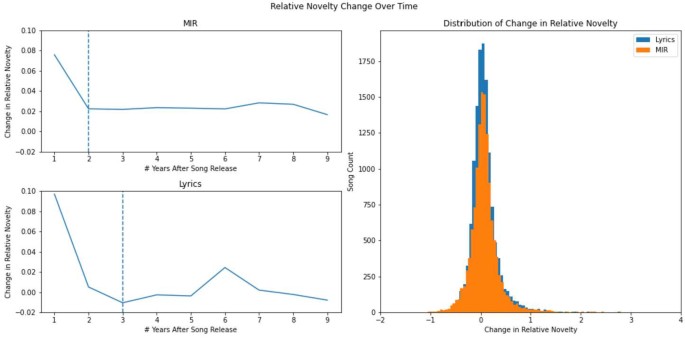
The two plots in the left column show the magnitude of year over year change in the average relative novelty score as songs are compared to later release years. For both MIR relative novelty and lyric relative novelty, we see that the rate of change is steep for the first two years after release, then stabilizes. The vertical lines indicate the inflection points where this occurs, which for MIR relative novelty is after two years, and for lyric relative novelty is after 3 years. The plot in the right column shows the distributions of relative novelty change for MIR novelty, and lyric novelty, which are calculated by taking the average change in relative novelty which occurred in the first three years after the song’s release
5.5 Average feature change over time
In addition to the initial novelty and relative novelty of individual songs, we also consider the magnitude of both MIR and lyric stylistic change over time. Within our data set, this can be understood as changes to the average position of the genre’s feature vectors in feature space over time. Since a snapshot of a genre’s position at a given time is represented by a distribution of song feature vectors, to consider the novelty score within a broader context of the genre’s movement in feature space, we examined the amount of feature variance seen within an individual genre over time for both MIR and lyric features. To perform this analysis, we assigned each song to a decade based on their release years, grouping them into ten-year intervals of 1974-1983, 1984-1993 and so on. Since the MIR and lyric feature vectors for each song provide us with an attribute-based representation of our data, we can compare how distinct the feature distributions of each class are from one another by training a decision tree classifier to predict the temporal class of a given song based on its feature values. Using the training data, a decision tree learns how to partition the feature space to best predict the temporal class of a song. The more distinct the area of feature space that each class inhabits, and the less overlap each has with other classes, the more accurate the decision tree. As a result, a higher accuracy tells us that there is less similarity between the feature distributions of different decades.
For our classifier, we used the random forest classifier model from the scikit-learn library [ 46 ]. A random forest works by fitting multiple decision trees to the data, and averaging results to improve accuracy and avoid overfitting. For our model, we used 500 trees with no depth limit. For individual genres, we then compared the accuracy of the classifier in predicting the temporal class of individual songs when trained using the MIR features versus when trained using the lyric features. For each set of features, a cross-validation was run using a repeated K-fold with 5 splits and 5 repeats, allowing us to generate the distribution of accuracy scores across different train-test splits of the data.
6.1 Relationship between MIR novelty and lyric novelty
In comparing the MIR and lyric novelty score distributions across all years and genres, we found that the MIR novelty distribution had a greater positive kurtosis and a greater positive skew than the lyric novelty distribution (see Fig. 3 top plot and Table 2 ). This tells us that there is a greater range in the above average novelty scores occurring within the MIR distribution. Although we can observe that the median value for the MIR novelty distribution, −0.21, is slightly lower than the median value for the lyric novelty distribution, −0.09, a one-way ANOVA test confirms no significant difference between the MIR novelty distribution and the lyrics novelty distribution ( \(F= 6.11\mathrm{e}{-}30\) , \(p= 1.0\) ). These trends held true when analyzing the novelty distributions within individual genres (Fig. 3 bottom plots and Table 2 ).
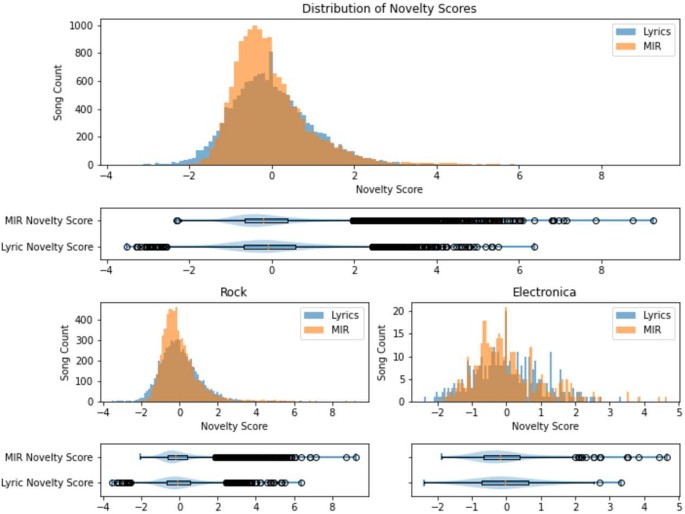
Distribution of MIR novelty scores and lyric novelty scores. Top plot displays the distributions for all songs within the dataset. The bottom two plots display the distributions for all songs within the Rock genre and Electronica genre, respectively
When examining the relationship between MIR novelty and lyric novelty of individual songs, we did not find a significant correlation between the two (Pearson correlation test \(r=-0.01\) , \(p=0.10\) ). They appear to be independent of one another, with no consistent patterns found in the relationship between the MIR novelty score and the lyric novelty score of a given song. As a result, a set of songs with the same MIR novelty score might have a wide variation in their lyric novelty scores, and vice versa.
6.2 Initial success
We incorporated song commercial success data to determine whether these variations in the novelty distributions of the two modalities were indicative of differences in how they impacted the likelihood of a song becoming popular. Using total number of weeks on chart as the metric for song success, we looked at the success of individual songs in relation to their MIR novelty score and the lyric novelty score. For example, Prince’s 1984 song, ‘When Doves Cry’, spent 21 weeks on the chart, and had an MIR novelty score of −0.63, putting it at the 26th percentile, and a lyric novelty score of 0.48, putting it at the 68th percentile. This tells us that the MIR features of the song were less novel when compared to other rock songs in 1984, but that the lyric features were more novel.
We found that similar to previous findings, the most popular songs had a degree of optimal differentiation both for MIR novelty, and for lyric novelty [ 22 , 23 ] (see Fig. 4 top row). We looked at the relationship between novelty and success for each modality separately, and using the Hotelling T2 test, found no statistically significant difference between the joint distribution of total weeks on chart with respect to MIR novelty, and the joint distribution of total weeks on chart with respect to lyrics novelty ( \(F= 3.07\mathrm{e}{-}30\) , \(p=1.0\) ). This was also found to be the case at the genre level as well (Fig. 4 bottom row). Specifically, Hotelling T2 test found no significant difference between the MIR joint distribution and the lyrics joint distribution for either Rock ( \(F= 7.03\mathrm{e}{-}30\) , \(p=1.0\) ) or Electronica ( \(F= 5.44\mathrm{e}{-}31\) , \(p=1.0\) ).
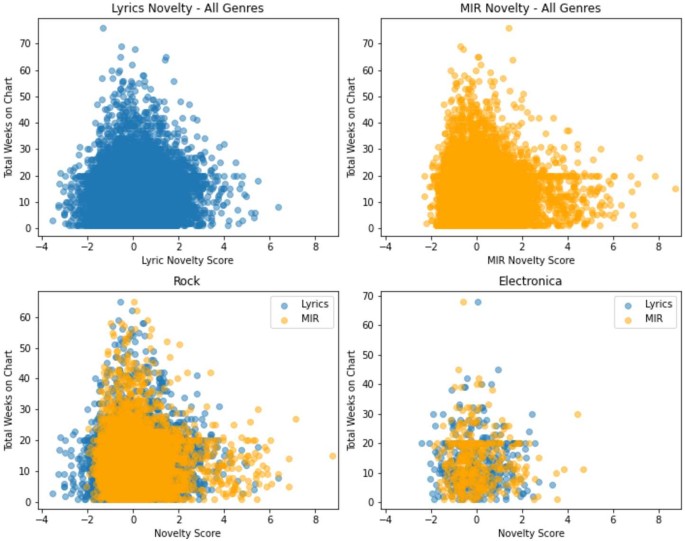
Joint distributions of song novelty scores and total number of weeks the song spent on the chart. Top row shows the joint distributions for all genres. Bottom row shows the overlay of MIR-total weeks joint distribution and lyrics-total weeks joint distribution for Rock, and Electronica. In each distribution we can see that the highest number of total weeks on chart occur within a certain range of novelty scores
Given that the songs with the most success fell into a rather narrow range of novelty values, we performed a Kernel Density Estimation analysis to estimate both the MIR novelty score and lyric novelty score which had the highest probability of being in the top 85th, 90th, and 95th percentile of total weeks on chart. For both the MIR-total weeks joint distribution and lyrics-total weeks joint distribution, the Python library scikit-learn was used to generate a Kernel Density Estimation using a Gaussian mixture model and a bandwidth of 0.3. [ 46 ] The KDE was used to generate probability scores for hypothetical pairings of novelty scores and total weeks on chart, which indicated the likelihood that a song with the given novelty score would be on the chart for the given number of weeks. This was done for 250,000 individual generated data points that were equally distributed across 500 unique values in the range of −1 to 1, which represented novelty values, and across 500 unique values in the range of 20 to 76, which represented the top 85th percentile of total weeks on chart. For each novelty value, we took the summation of the generated probability scores to calculate the relative probability that a song with that amount of novelty would reach anywhere within the top 85th percentile of total weeks on chart. This process was then repeated for the top 90th percentile of total weeks on chart, and the top 95th percentile of total weeks on chart.
For each of these, we can see in Fig. 5 that for both MIR novelty and lyric novelty, the probability of success increases as the novelty score increases, until a certain point at which it peaks and then because to decrease again. The novelty score for this peak value that we have estimated in our analysis indicates the degree of optimal differentiation that is most likely to help them succeed. Below this, the song is likely to be too similar to stand out from other songs, while above this, it starts to diverge too much from what the audience expects. While the novelty scores of a song cannot be used to predict exactly how successful it will be, songs with novelty scores close to our estimates will have a greater chance of achieving high levels of success than songs with novelty scores that are higher or lower.
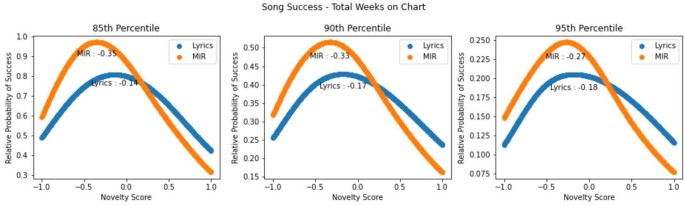
Relative probability of success in reaching the 85th, 90th, and 95th percentile of total weeks on chart across a range of MIR novelty scores, and across a range of lyric novelty scores. Since the relative probability values are a summation of probability estimates of a generated data set, they are dependent on the total number of data points generated for our sample, and should not be treated as an absolute probability value
We found that for both lyrics and MIR, the novelty scores which had the highest probabilities of success for each of these performance tiers was just slightly lower than the mean novelty scores of the population. We also found that across the three total week ranges we tested, the MIR novelty score was consistently slightly lower than the lyric novelty score (see Fig. 5 and Table 3 ). Additionally, as the analysis narrows from the top 85th percentile to only the top 95th percentile, we also see that the MIR novelty score increases, while the lyric novelty score decreases, causing the difference between them to grow smaller.
Given that we did not find any consistent patterns in the relationship between the MIR novelty and lyric novelty of individual songs, we wanted to explore whether different combinations of MIR novelty scores and lyric novelty scores would impact a song’s probability of success. For this, we generated a Kernel Density Estimation for the joint distribution which included both lyric novelty and MIR novelty, along with total weeks on chart. This was then used to calculate the probability scores for 1,000,000 equally distributed generated data points having lyric novelty scores between −1 to 1, MIR novelty scores between −1 to 1, total weeks on chart in the 90th percentile, between 22 to 76. For each unique pair of MIR and lyric novelty scores, we took the summation of the generated probability scores to calculate the relative probability of a song with those scores being in the top 90th percentile of total weeks on chart.
We see in Fig. 6 that the highest relative probability of success occurs when a song is close to the optimal novelty values for both lyrics and MIR. As we move outward from this area where both novelty scores are close to optimal, the radial pattern of the gradient indicates that variance in the probability of success is equally affected by both variance in the MIR novelty score, and variance in the lyric novelty score. Additionally, given that the gradient is roughly equal for points that have the same distance from this optimal center point, this tells us that the proportional relationship of MIR novelty to lyric novelty is not an explanatory variable, but rather it is the combined total distance from the optimal center that impacts a songs probability of success.
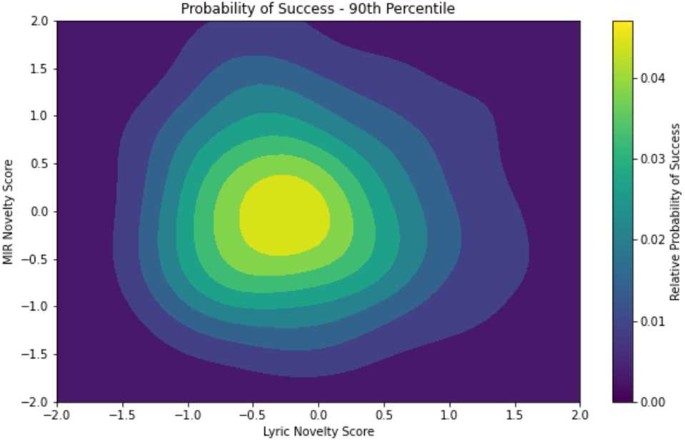
Relative probability of success in reaching the 90th percentile of total weeks on chart for different combinations of MIR and lyric novelty scores. We see that the probability decreases as either score moves away from the value that provides the optimal degree of differentiation within its modality. Since the relative probability values are a summation of probability estimates of a generated data set, they are dependent on the total number of data points generated for our sample, and should not be treated as an absolute probability value
Whether the point lies above or below the optimal value for either novelty score does not change the relationship, which tells us that having a higher than optimal novelty score for one modality can’t be ‘balanced out’ by a lower than optimal novelty score for the other modality. If that were the case, and it were only about reaching an optimal value for the average of the two novelty scores, then we would expect to see, for instance, a song with an MIR novelty score of −0.22, 0.1 higher than optimal, and a lyric novelty score of −0.27, 0.1 less than optimal, to have an equal probability of success as a song with the optimal values of an MIR novelty score of −0.32 and a lyric novelty score of −0.17. In the contour map we see that the MRI novelty score that optimizes success probability does not change for different values of lyric novelty, and vice versa, the lyric novelty score that optimizes success does not change for different values of MIR novelty. However, we also observe that the combined distance of both novelty scores from their respective optimal values will impact a song’s probability of success, indicating that even though a song’s lyric novelty and MIR novelty are independent of one another, their deviations from the optimal values will have an additive effect on the overall perception of song novelty as it relates to optimal differentiation.
6.3 Influence probability
It is worth noting that when looking at the inflection points in Figure 2 that indicate a plateau in the rate of change of relative novelty, the average year over year change in relative novelty for MIR stays positive, meaning that on average, the MIR features of a genre will tend to become less similar to those in previous years as the gap in time increases. The average year over year change in relative novelty for lyrics, however, is for the most part negative, suggesting that song lyrics within a genre tend to be more similar to those of songs released in previous years. When comparing the distribution relative novelty change for both modalities, we see that both follow a normal distribution, with a one-way ANOVA test showing no statistically significant difference between them ( \(F= 0.05\) , \(p= 0.83\) ). However, the distribution of lyric relative novelty change shows a larger positive kurtosis and skew than that of the MIR relative novelty change distribution, which is the opposite of the trend we observed between MIR and lyrics in the initial novelty score distributions (see Fig. 2 right plot and Table 4 ). Additionally, while the difference is not statistically significant, we observe greater variance between the two relative novelty change distributions than we do between the initial MIR and lyric novelty score distributions.
Since a greater decrease in a song’s relative novelty indicates a higher likelihood that the song was influential, scores in the bottom 10th percentile represent high performers for this metric. To determine whether a song’s initial novelty scores had any correlation with how its relative novelty changed over time, we generated a Kernel Density Estimation for the joint distribution between MIR novelty scores and MIR relative novelty change, as well as for the joint distribution between lyric novelty scores and lyric relative novelty change. Using the same procedure as for the initial success KDE, we found that for both lyrics and MIR, the novelty scores that correlated with the highest probabilities of seeing a large decrease in relative novelty were below the average novelty score of the population, and slightly lower than the optimal differentiation novelty values we estimated for initial success (see Fig. 7 ). Again, we see that the optimal MIR novelty score is slightly lower than the optimal lyric novelty score.
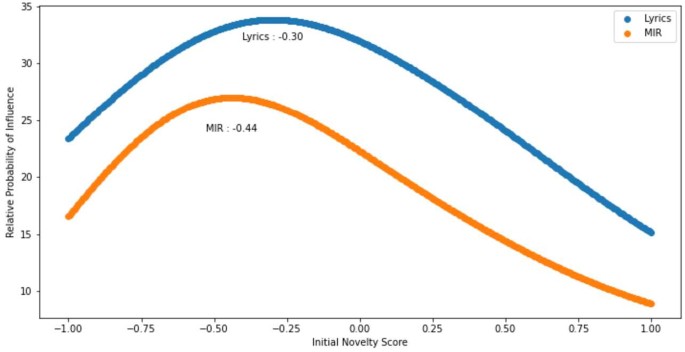
Relative probability of a song being in the bottom 10th percentile for relative novelty change based on initial novelty scores. We see that the probability decreases as either score moves away from the value that provides the optimal degree of differentiation within its modality. Since the relative probability values are a summation of probability estimates of a generated data set, they are dependent on the total number of data points generated for our sample, and should not be treated as an absolute probability value
Because optimal differentiation is about the relationship between cultural artifacts and their contemporaries, the fact that we see a degree of optimal differentiation in the relationship between artifacts released at different points in time is a new finding. While one possible explanation is that the initial popularity of songs that are optimally differentiated makes them more likely to be influential, we did not find any correlation between total number of weeks on chart, and either lyric or MIR relative novelty change. For example, while both the 1998 Janet Jackson song ‘Together Again’ and the 2005 Kelly Clarkson song ‘Since U Been Gone’ were on the chart for a total of 46 weeks, the relative MIR novelty for ‘Together Again’ decreased by −0.21, while the relative MIR novelty for ‘Since U Been Gone’ increased by 0.22. These results suggest that the amount of time a song has spent on the chart cannot be used to predict its likelihood of being influential.
To investigate this, we ran an analysis to examine the relationship between total weeks on chart and influence probability when controlling for limited ranges of novelty scores. Running two analyses, one for MIR novelty and MIR relative novelty change, and one for lyric novelty and lyric relative novelty change, we considered songs that fell within three different ranges of novelty scores; the bottom 10th percentile, the top 10th percentile, and the 10th percentile centered around the novelty score with the highest probability of maximizing total weeks on chart. Within each range, the songs were then grouped by the number of weeks they had spent on the chart. An aggregated influence probability was calculated for each week in the range of 0 to 35 by calculating the percentage of songs in that grouping whose relative novelty had decreased. Additional details for this process can be found in the Appendix .
We found that, relative to the song’s novelty score, the influence probability varied with increases in the amount of time the song spent on the chart. In Fig. 8 we see that regardless of the modality or the novelty grouping, influence probability initially increases with more time spent on chart, then at a certain point, peaks and starts to decrease. For lyric novelty, we see a peak occurs at the same time for all three novelty bins, at roughly 10-15 weeks. Beyond that, both the bottom 10th percentile and the top 10th percentile see a decrease, although the rate of decrease for influence probability appears to be more pronounced for the songs in the top 90th novelty percentile. For lyric novelty in the optimal range, we do see a second peak around 25-30 weeks, however beyond that the influence probability again drops. We see that regardless of the total amount of time on chart, higher lyric novelty scores are correlated with lower influence probability. For MIR novelty, we see in Fig. 8 that the relationship between the influence probability and additional weeks on the chart varies depending on the song’s novelty score. Songs in the bottom 10th percentile see influence probability peak at 10 weeks, and then consistently decrease with additional time on the chart. For songs in the optimal zone, however, there is a consistent increase in influence probability until roughly 26-7 weeks, at which point the influence probability quickly decreases. For songs in the top 90th percentile, we see a steeper increase in influence probability which lasts until roughly 30 weeks before hitting the peak and then decreasing. Here we also see that in contrast to the pattern observed for lyric novelty scores, higher MIR novelty scores are correlated with higher influence probability regardless of the number of weeks spent on the chart. We typically associate increased exposure with greater success, for both short term commercial success, and also for being influential within the creative space. It would seem intuitive that greater exposure would lead to a greater probability of exerting influence, as more people hear and become familiar with the cultural artifact in question. However these results suggest that while that is sometimes the case, there is also an optimal amount of exposure, which can vary depending on the novelty and modality of the attributes being considered.
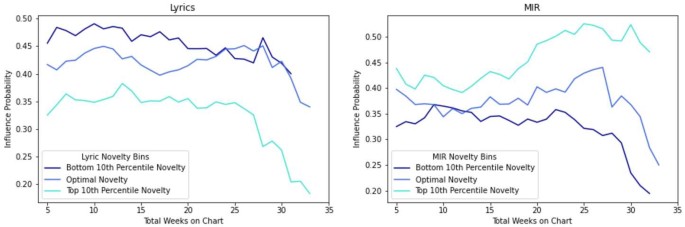
Controlling for initial novelty, the influence probability increases at first with additional weeks on chart, then after a certain amount of time it peaks and begins decreasing. For lyric novelty, the peak occurs at roughly 10 weeks. For MIR novelty, the change in influence probability relative to time on chart varies depending on the initial novelty score. For higher initial novelty scores, we see the peak influence probability occur after a longer period of time on the chart
Although we previously found no correlation between individual songs’ initial lyric novelty and initial MIR novelty, we did find that there was a small but significant correlation ( \(r= 0.22\) , \(p< 0.001\) ) between the average change in lyric relative novelty and the average change in MIR relative novelty (see Fig. 9 ). Additionally, we observed that for songs with low lyric novelty scores, higher MIR novelty scores had a slight positive correlation with influence probability, while for songs with high MIR novelty scores, higher lyric novelty scores also had a slight positive correlation with influence probability. A possible explanation is that these combinations of high and low novelty scores impact how memorable a song is, even if they are more likely to hurt the songs initial success probability. However, it is not clear why we observe these interaction patterns only for songs at the extreme ends of the novelty score ranges, and these findings highlight an avenue for further research into how the attributes of cultural artifacts impact their likelihood of influencing the larger cultural ecosystem.
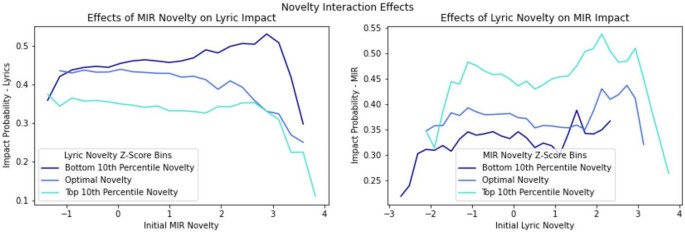
Controlling for initial novelty, we see that different combinations of high and low MIR and lyric novelty scores have different associations with changes in influence probability
6.4 Feature variance over time
In order to delve into why the effect of exposure was so different for lyric novelty than for MIR novelty, we examine the differences in the amount of change over time for the average MIR features compared to the amount of change over time for the average lyric features. In Fig. 10 , we compare the distribution of accuracy scores when using our random forest classifier to predict the temporal class of individual songs using the MIR features versus when using the lyric features. For each of the largest genres, we observed a statistically significant difference ( \(p < 0.001\) ) between the predictive power of the two distributions, with the MIR features consistently resulting in more accurate predictions. Given that the overall accuracy scores are fairly low, with the model trained on lyric features ranging between 42% and 48% accuracy and the model trained on MIR features ranging between 52% and 60% accuracy, this indicates that there is still overlap in the feature distributions of different decades. However, since our analysis demonstrates that there is more predictive power in the MIR features, this tells us that the distributions of MIR features from different decades are more distinct from one another in the feature space than are the distributions of lyric vectors for different decades. Given that we also found that the average year-over-year change in relative novelty for MIR stays positive, indicating that the average distance between old and new songs in MIR feature space is always increasing, we can infer that within the individual genres, there is long-term directionality to the genre’s movement through MIR feature space. In contrast, the average year-over-year change in relative novelty for lyrics is, for the most part, negative, which when taken in conjunction with the lower classification accuracy scores when using lyric features, suggests that there is not a significant amount of directionality to the movement occurring within the lyric feature space.
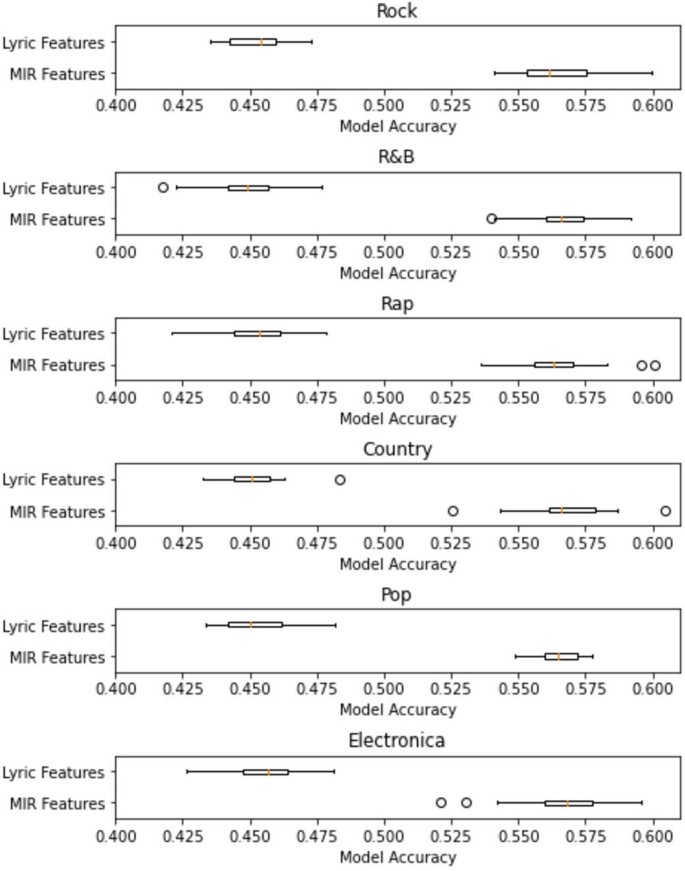
Distributions of accuracies for a random forest classifier when trained on each genre’s MIR and lyric features, respectively. The difference between each pair of distributions is statistically significant ( \(p < 0.001\) ), with MIR features resulting in higher accuracy scores from cross-validation
7 Discussion
By utilizing computational methods to analyze cultural data at scale, this research contributes to our understanding of the relationship between novelty impacts the dynamics of cultural change within the context of the larger cultural ecosystem. Our results highlight the ways in which we perceive and evaluate different degrees of novelty and differentiation. Although the high-level and aggregate nature of our data does not enable us to create a prediction model for identifying hit songs in their unique context, or causally attribute stylistic changes within a genre to the influence of specific songs, our results contribute to understanding overarching patterns of novelty.
7.1 Novelty and music cognition
Our finding that there is no relationship between the lyric and MIR novelty scores of individual songs, and that the optimal novelty scores for each modality are also independent of one another is supported by previous work in music cognition, which finds evidence that music and lyrics are processed independently [ 47 , 48 ]. This explains why the negative impact on success probability of an above optimal novelty score for one modality cannot be mitigated by the song having a below optimal score in the other modality. Our results do not provide any information which would allow us to evaluate the possibility of a causal relationship between the aforementioned music cognition data and the trends we have observed, however the observation of these connections between large scale phenomenon and cognitive processes that occur at the individual level suggest that this could be a productive area of interdisciplinary study. It is possible that research in the field of cognitive science could provide insights into cognitive perceptual processing, which could inform potential avenues of inquiry when investigating cultural trends and evolution.
7.2 Novelty and exposure effects
Although our data is not sufficient to investigate any possible causal relationship between initial novelty and influence probability, we observed that the relationship between a song’s initial novelty score and its influence probability varies relative to how many weeks it has spent on the chart. For songs with higher MIR novelty scores, we see that an increase in time on chart has a positive correlation with influence probability within the time range we analyzed. This potentially explains why the distribution of MIR novelty scores is more heavily right-tailed than the distribution of lyric novelty scores, as the positive impact of increased exposure may cause more variation and range in the MIR novelty scores that end up being successful enough to reach the Billboard Hot 100 chart.
Previous work exploring the impact of repeated exposure to unfamiliar music and subsequent music preferences have found that this additional exposure increases the likelihood that the listeners will enjoy the music when they hear it again [ 49 ]. Additional research has also found that repeated exposure in the context of collective attention to news stories shared online, leads to novelty decay over time [ 50 ]. It is possible, then, that for high-novelty songs, the increased exposure due to more time spent on the chart may decrease the perceived novelty of the song, leading audiences to experience it as being closer to the optimal level of differentiation. For low-novelty artifacts, however, this decrease in perceived novelty could make them seem too familiar, hence we see that the amount of exposure which was beneficial varied depending on the initial novelty scores. For lyric novelty, we saw that increased time on the chart correlated with a decrease in influence probability, regardless of whether the lyric novelty score was lower or higher than optimal. Given that we observed significantly less variance over time for lyric features than we did for MIR features, it is possible that even songs with very high lyric novelty scores are not distinct enough to benefit from higher levels of exposure.
For lyric novelty, we saw that increased time on the chart correlated with a decrease in influence probability, regardless of whether the lyric novelty score was lower or higher than optimal. Given that we observed significantly less variance over time for lyric features than we did for MIR features, it is possible that even songs with very high lyric novelty scores are not distinct enough to benefit from higher levels of exposure. This suggests that when analyzing differences between cultural artifacts and their relationship to various metrics of success, it is important to draw a distinction between differentiation, which measures the amount of variation between the artifact and the other artifacts it is being compared to, and ‘true’ novelty, which would consider the degree to which the artifact is introducing new material into the canon of its domain.
Our findings suggest that it is possible for an artifact to be ‘overexposed’, at which point in time the perception of novelty drops below an optimal level. Our results indicate that the amount of exposure it takes for this to occur is going to vary depending on the initial novelty of the artifact. This is an important consideration when modeling and predicting the dissemination of creative ideas and products, both in theoretical research, and in the development of practical applications, such as recommender systems. Additionally, this highlights the importance of considering the potential effect of social influence on not only the initial popularity of cultural artifacts, but also the longer term evolution of the cultural ecosystem. In Salganik et al’s study on social influence in cultural markets, different social behaviors led to different patterns of success within an artificial music market, demonstrating the significant impact of social influence on what songs become popular [ 51 ]. While the effect of social influence was shown to be largely independent of the specific attributes of the individual songs, by impacting which songs become popular this will also impact the relative amount of exposure those songs will receive. As our analysis suggests that patterns of exposure may potentially impact the likelihood of an artifact exerting stylistic influence, suggests a possible mechanism by which the impact of social influence has a downstream effect on the stylistic evolution of the musical ecosystem.
7.3 Implications for recommender systems
Understanding the degree to which the defining characteristics of musical genres change over time has applications for music recommendation software. There are limitations to traditional approaches of using historical data in predictions [ 52 ], and in order to avoid static behavior it is important for us to be able to identify what are the indicators that can help predict a preference shift [ 40 ]. If we can determine the degree to which more novel outliers indicate the evolution of a subset of music, we can take that into account when tracking an individual’s music preferences, and better predict what they might like as their taste evolves. This is especially relevant for increasing the efficiency of recommender systems incorporating exploratory algorithms, as it can inform more directed exploration, as well as the ideal degree of novelty to incorporate with each round of exploration [ 53 ].
7.4 Limitations
It is important to note that there are many external factors that can affect whether or not a song become successful, which are unfortunately not captured in the scope of this data set. As a result, we cannot draw any inferences about direct causal relationships between novelty and the success metrics we examined. Additionally, our data is lacking important controls that could be correlated with novelty and influence, due to the currently unavailability of such comprehensive data.
As the Hot 100 data contains only a partial view of popular music, and its selection criteria has changed over time, future work could involve gathering a larger data set that would encompass a broader representation of modern music. For the purposes of this analysis, the Hot 100 songs served as a sample of the prevailing mainstream cultural trends in music. However, it is still a small sample of all the potential songs that could be included in the umbrella of modern popular music. Additionally when grouping music by genre, we must acknowledge that genre classifications are inherently subjective. Genre labeling for this data set came from Discogs.com, which provides crowd-sourced data for songs, so the groupings provided do not necessarily represent an objective ground truth [ 54 ].
8 Conclusion
Utilizing MIR data to perform analysis at scale, we compared musical artifacts’ relative novelty over time to identify consistent patterns in the dynamics of cultural change. Our results showed evidence for both optimal differentiation in successful songs, and the conditioning effect of prior artifacts on stylistic change. By bringing in findings from sociology, cognitive science, and musicology to provide further insight into the impact of novelty on modern music evolution, our research provides quantitative methods that will enable media systems to track this organic evolution in a more informed manner.
Availability of data and materials
The code that supports the findings of this study are available at: https://github.com/LINK-NU/Billboard-Hot-100-Music-Novelty . Data available on request.
Abbreviations
Music Information Retrieval
Mauch M, MacCallum RM, Levy M, Leroi AM (2015) The evolution of popular music: USA 1960–2010. R Soc Open Sci 2(5):150081. https://doi.org/10.1098/rsos.150081
Article Google Scholar
WeißC, Mauch M, Dixon S, Müller M (2019) Investigating style evolution of Western classical music: a computational approach. Music Sci 23(4):486–507. https://doi.org/10.1177/1029864918757595
Serrà J, Corral A, Boguñá M, Haro M, Arcos JL (2012) Measuring the evolution of contemporary Western popular music. Sci Rep 2(1):521. https://doi.org/10.1038/srep00521
Bomin SL, Lecointre G, Heyer E (2016) The evolution of musical diversity: the key role of vertical transmission. PLoS ONE 11(3):0151570. https://doi.org/10.1371/journal.pone.0151570
Prockup M, Ehmann AF, Gouyon F, Schmidt EM, Celma O, Kim YE (2015) Modeling genre with the music genome project: comparing human-labeled attributes and audio features. In: Proceedings of the 16th ISMIR conference, Malaga, p 7
Google Scholar
Klimek P, Kreuzbauer R, Thurner S (2019) Fashion and art cycles are driven by counter-dominance signals of elite competition: quantitative evidence from music styles. J R Soc Interface 16(151):20180731. https://doi.org/10.1098/rsif.2018.0731
Magron P, Févotte C (2020) Leveraging the structure of musical preference in content-aware music recommendation. CoRR. arXiv:2010.10276
Moffat D, Ronan D, Reiss JD (2015) An evaluation of audio feature extraction toolboxes. In: Proc. of the 18th int. conference on digital audio effects (DAFx-15), Trondheim, p 7
Friberg A, Schoonderwaldt E, Hedblad A, Fabiani M, Elowsson A (2014) Using perceptually defined music features in music information retrieval. arXiv:1403.7923 [cs]. Accessed 2020-10-06
Bertin-Mahieux T (2013) Large-Scale Pattern Discovery in Music. PhD thesis, Columbia University. https://doi.org/10.7916/D8NC67CT . Accessed 2020-10-06
Lippens S, Martens JP, De Mulder T (2004) A comparison of human and automatic musical genre classification. In: 2004 IEEE international conference on acoustics, speech, and signal processing, vol 4, pp 1520–6149. https://doi.org/10.1109/ICASSP.2004.1326806 . ISSN: 1520-6149
Chapter Google Scholar
Interiano M, Kazemi K, Wang L, Yang J, Yu Z, Komarova NL (2018) Musical trends and predictability of success in contemporary songs in and out of the top charts. R Soc Open Sci 5(5):171274. https://doi.org/10.1098/rsos.171274
Mayerl M, Votter M, Zangerle MME (2020) Comparing lyrics features for genre recognition. In: Proceedings of the 1st workshop on NLP for music and audio (NLP4MusA). Assoc. Comput. Linguistics, Online, pp 73–77. https://www.aclweb.org/anthology/2020.nlp4musa-1.15.pdf
Mayer R, Neumayer R, Rauber A (2008) Combination of audio and lyrics features for genre classification in digital audio collections. In: Proceedings of the 16th ACM international conference on multimedia. MM ’08. Assoc. Comput. Mach., New York, pp 159–168. https://doi.org/10.1145/1459359.1459382
Hu X, Downie JS, Ehmann AF (2009) Lyric text mining in music mood classification. In: Proceedings of the 10th international society for music information retrieval conference. Kobe International Conference Center, Kobe, p 6
Hu X, Downie JS (2010) Improving mood classification in music digital libraries by combining lyrics and audio. In: Proceedings of the 10th annual joint conference on digital libraries. JCDL ’10. Assoc. Comput. Mach., New York, pp 159–168. https://doi.org/10.1145/1816123.1816146
McVicar M, Giorgi BD, Dundar B, Mauch M (2021) Lyric document embeddings for music tagging. In: Proc. of the 15th international symposium on CMMR, p 10
Mikolov T, Chen K, Corrado G, Dean J (2013) Efficient estimation of word representations in vector space. arXiv:1301.3781 [cs]. Accessed 2020-12-08
Le QV, Mikolov T (2014) Distributed representations of sentences and documents. arXiv:1405.4053 [cs]. Accessed 2020-12-08
Whalen R, Lungeanu A, DeChurch L, Contractor N (2020) Patent similarity data and innovation metrics. J Empir Leg Stud 17(3):615–639. https://doi.org/10.1111/jels.12261
Askin N, Mauskapf M (2017) What makes popular culture popular? Product features and optimal differentiation in music. Am Sociol Rev 82(5):910–944. https://doi.org/10.1177/0003122417728662
Askin N, Mauskapf M (2014) Cultural attributes and their influence on consumption patterns in popular music. In: Aiello LM, McFarland D (eds) Social informatics. SocInfo 2014. Lecture notes in computer science, vol 8851. Springer, Cham, pp 508–530. https://doi.org/10.1007/978-3-319-13734-6_36
Berger J, Packard G (2018) Are atypical things more popular? Psychol Sci 29(7):1178–1184. https://doi.org/10.1177/0956797618759465
Anderson A, Maystre L, Anderson I, Mehrotra R, Lalmas M (2020) Algorithmic effects on the diversity of consumption on Spotify. In: Proceedings of the web conference 2020. Assoc. Comput. Mach., New York, pp 2155–2165. https://doi.org/10.1145/3366423.3380281
Laurier C, Grivolla J, Herrera P (2008) Multimodal music mood classification using audio and lyrics. In: 2008 seventh international conference on machine learning and applications, pp 688–693. https://doi.org/10.1109/ICMLA.2008.96
Neumayer R, Rauber A (2007) Integration of text and audio features for genre classification in music information retrieval. In: Amati G, Carpineto C, Romano G (eds) Advances in information retrieval. Lecture notes in computer science, vol 4425. Springer, Berlin, pp 724–727. http://link.springer.com/10.1007/978-3-540-71496-5_78
Saleh B, Abe K, Arora RS, Elgammal A (2016) Toward automated discovery of artistic influence. Multimed Tools Appl 75(7):3565–3591. https://doi.org/10.1007/s11042-014-2193-x
Uzzi B, Mukherjee S, Stringer M, Jones B (2013) Atypical combinations and scientific impact. Science 342(6157):468–472. https://doi.org/10.1126/science.1240474
Li Y, Zhang Y, Capra R (2022) Analyzing information resources that support the creative process. In: ACM SIGIR conference on human information interaction and retrieval. CHIIR, vol ’22. Assoc. Comput. Mach., New York, pp 180–190. https://doi.org/10.1145/3498366.3505817
Liu L, Wang Y, Sinatra R, Giles CL, Song C, Wang D (2018) Hot streaks in artistic, cultural, and scientific careers. Nature 559(7714):396–399. https://doi.org/10.1038/s41586-018-0315-8
Shin H, Kim K, Kogler DF (2022) Scientific collaboration, research funding, and novelty in scientific knowledge. PLoS ONE 17(7):0271678. https://doi.org/10.1371/journal.pone.0271678
Shi F, Foster JG, Evans JA (2015) Weaving the fabric of science: dynamic network models of science’s unfolding structure. Soc Netw 43:73–85. https://doi.org/10.1016/j.socnet.2015.02.006
Miles SA, Rosen DS, Barry S, Grunberg D, Grzywacz N (2021) What to expect when the unexpected becomes expected: harmonic surprise and preference over time in popular music. Front Human Neurosci 15:578644
Sreenivasan S (2013) Quantitative analysis of the evolution of novelty in cinema through crowdsourced keywords. Sci Rep 3(1):2758. https://doi.org/10.1038/srep02758
Jing E, DeDeo S, Ahn Y-Y (2019) Sameness attracts, novelty disturbs, but outliers flourish in fanfiction online. arXiv:1904.07741 [cs]. Accessed 2020-11-20
Park D, Nam J, Park J (2020) Novelty and influence of creative works, and quantifying patterns of advances based on probabilistic references networks. EPJ Data Sci 9(1):1. https://doi.org/10.1140/epjds/s13688-019-0214-8
Liu M, Bu Y, Chen C, Xu J, Li D, Leng Y, Freeman RB, Meyer ET, Yoon W, Sung M, Jeong M, Lee J, Kang J, Min C, Song M, Zhai Y, Ding Y (2022) Pandemics are catalysts of scientific novelty: evidence from COVID-19. J Assoc Inf Sci Technol 73(8):1065–1078. https://doi.org/10.1002/asi.24612
Cheng D, Joachims T, Turnbull D (2020) Exploring acoustic similartiy for novel music recommendation, 7
Zangerle E, Huber R, Vötter M, Yang YH (2019) Hit song prediction: leveraging low- and high-level audio features. In: Proceedings of the 20th international society for music information retrieval conference, ISMIR 2019, pp 319–326. https://doi.org/10.5281/zenodo.3258042
Moore JL, Chen S, Joachims T, Turnbull D (2013) Taste over time: the temporal dynamics of user preferences. In: Proceedings of the 14th international society for music information retrieval conference, ISMIR 2013, p 6
Berlyne DE (1970) Novelty, complexity, and hedonic value. Percept Psychophys 8(5):279–286. https://doi.org/10.3758/BF03212593
Chmiel A, Schubert E (2017) Back to the inverted-U for music preference: a review of the literature. Psychol Music 45(6):886–909. https://doi.org/10.1177/0305735617697507
Chai S, Menon A (2019) Breakthrough recognition: bias against novelty and competition for attention. Res Policy 48(3):733–747. https://doi.org/10.1016/j.respol.2018.11.006
Wang J, Veugelers R, Stephan P (2017) Bias against novelty in science: a cautionary tale for users of bibliometric indicators. Res Policy 46(8):1416–1436
Radim Ř, Sojka P (2010) Gensim: topic modelling for humans. In: Proceedings of the LREC 2010 workshop on new challenges for NLP frameworks. ELRA, Valletta, pp 45–50. https://radimrehurek.com/gensim/models/doc2vec.html
Pedregosa F, Varoquaux G, Gramfort A, Michel V, Thirion B, Grisel O, Blondel M, Prettenhofer P, Weiss R, Dubourg V, Vanderplas J, Passos A, Cournapeau D, Brucher M, Perrot M, Duchesnay E (2011) Scikit-learn: machine learning in python. J Mach Learn Res 12(85):2825–2830
MathSciNet MATH Google Scholar
Besson M, Faïta F, Peretz I, Bonnel A-M, Requin J (1998) Singing in the brain: independence of lyrics and tunes. Psychol Sci 9(6):494–498. https://doi.org/10.1111/1467-9280.00091
Rigoulot S, Armony JL (2016) Early selectivity for vocal and musical sounds: electrophysiological evidence from an adaptation paradigm. Eur J Neurosci 44(10):2786–2794. https://doi.org/10.1111/ejn.13391
Peretz I, Gaudreau D, Bonnel A-M (1998) Exposure effects on music preference and recognition. Mem Cogn 26(5):884–902. https://doi.org/10.3758/BF03201171
Wu F, Huberman BA (2007) Novelty and collective attention. Proc Natl Acad Sci 104(45):17599–17601. https://doi.org/10.1073/pnas.0704916104
Salganik MJ, Dodds PS, Watts DJ (2006) Experimental study of inequality and unpredictability in an artificial cultural market. Science 311(5762):854–856. https://doi.org/10.1126/science.1121066
Jung S-G, Salminen J, Chowdhury SA, Ramirez Robillos D, Jansen BJ (2020) Things change: comparing results using historical data and user testing for evaluating a recommendation task. In: Extended abstracts of the 2020 CHI conference on human factors in computing systems. CHI EA ’20. Assoc. Comput. Mach., New York, pp 1–7. https://doi.org/10.1145/3334480.3382945
Xing Z, Wang X, Wang Y (2014) Enhancing collaborative filtering music recommendation by balancing exploration and exploitation. In: Proceedings of the 15th international society for music information retrieval conference, Taipei
Lorince J, Zorowitz S, Murdock J, Todd PM (2015) The wisdom of the few? “Supertaggers” in collaborative tagging systems. J Web Sci 1:16–32. https://doi.org/10.1561/106.00000002
Download references
Acknowledgements
The authors would like to thank Miriam Alex for her assistance with data collection and cleaning, and would like to thank Michael Mauskapf and Noah Askin for sharing their unique data set.
Author information
Authors and affiliations.
Northwestern University, 2240 Campus Drive, 60208, Evanston, USA
Katherine O’Toole & Emőke-Ágnes Horvát
You can also search for this author in PubMed Google Scholar
Contributions
KO designed the study, ran all data analysis, and drafted the manuscript. EÁH provided feedback on study design and revised and edited the manuscript. All authors read and approved the final manuscript.
Corresponding author
Correspondence to Katherine O’Toole .
Ethics declarations
Competing interests.
The authors declare that they have no competing interests.
1.1 A.1 Influence probability calculations
For the sake of reducing noise, we did not use the overall average of the relative novelty change for the aggregated influence probability value. Instead, two dummy variables were created, one corresponding with lyric relative novelty change, and one with MIR relative novelty change. For each song, if its change in lyric relative novelty was negative, the corresponding dummy variable was assigned a 1, and if positive, a 0. The same was done for MIR relative novelty change. When grouping the songs by novelty range and total weeks on chart, we then took the average of the appropriate dummy variable to calculate the probability that a song with those parameters would have a decrease in relative novelty, indicating a higher likelihood of being influential. Because the distribution of data meant that the size of each sample varied for the different numbers of total weeks on chart, the influence probability over the range of total weeks was calculated using a weighted rolling window average, with a window size of 8. Additionally, because there are a relatively few songs that spend more than 40 weeks on the chart, almost all of which fall into the optimal novelty bin for both modalities, we limited our analysis to the 0-35 week range.
Rights and permissions
Open Access This article is licensed under a Creative Commons Attribution 4.0 International License, which permits use, sharing, adaptation, distribution and reproduction in any medium or format, as long as you give appropriate credit to the original author(s) and the source, provide a link to the Creative Commons licence, and indicate if changes were made. The images or other third party material in this article are included in the article’s Creative Commons licence, unless indicated otherwise in a credit line to the material. If material is not included in the article’s Creative Commons licence and your intended use is not permitted by statutory regulation or exceeds the permitted use, you will need to obtain permission directly from the copyright holder. To view a copy of this licence, visit http://creativecommons.org/licenses/by/4.0/ .
Reprints and permissions
About this article
Cite this article.
O’Toole, K., Horvát, EÁ. Novelty and cultural evolution in modern popular music. EPJ Data Sci. 12 , 3 (2023). https://doi.org/10.1140/epjds/s13688-023-00377-7
Download citation
Received : 15 June 2022
Accepted : 27 January 2023
Published : 10 February 2023
DOI : https://doi.org/10.1140/epjds/s13688-023-00377-7
Share this article
Anyone you share the following link with will be able to read this content:
Sorry, a shareable link is not currently available for this article.
Provided by the Springer Nature SharedIt content-sharing initiative
- Cultural novelty
- Computational methods
- Quantitative analysis
- Computational social science
Thank you for visiting nature.com. You are using a browser version with limited support for CSS. To obtain the best experience, we recommend you use a more up to date browser (or turn off compatibility mode in Internet Explorer). In the meantime, to ensure continued support, we are displaying the site without styles and JavaScript.
- View all journals
- Explore content
- About the journal
- Publish with us
- Sign up for alerts
- Open access
- Published: 26 July 2012
Measuring the Evolution of Contemporary Western Popular Music
- Joan Serrà 1 ,
- Álvaro Corral 2 ,
- Marián Boguñá 3 ,
- Martín Haro 4 &
- Josep Ll. Arcos 1
Scientific Reports volume 2 , Article number: 521 ( 2012 ) Cite this article
104k Accesses
113 Citations
1063 Altmetric
Metrics details
- Applied physics
- Mathematics and computing
- Statistical physics, thermodynamics and nonlinear dynamics
Popular music is a key cultural expression that has captured listeners' attention for ages. Many of the structural regularities underlying musical discourse are yet to be discovered and, accordingly, their historical evolution remains formally unknown. Here we unveil a number of patterns and metrics characterizing the generic usage of primary musical facets such as pitch, timbre and loudness in contemporary western popular music. Many of these patterns and metrics have been consistently stable for a period of more than fifty years. However, we prove important changes or trends related to the restriction of pitch transitions, the homogenization of the timbral palette and the growing loudness levels. This suggests that our perception of the new would be rooted on these changing characteristics. Hence, an old tune could perfectly sound novel and fashionable, provided that it consisted of common harmonic progressions, changed the instrumentation and increased the average loudness.
Similar content being viewed by others
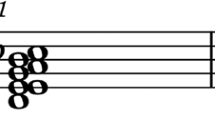
The diachronic development of Debussy’s musical style: a corpus study with Discrete Fourier Transform
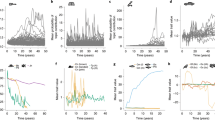
The pace of modern culture
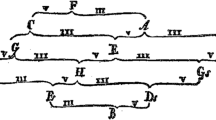
Computational modeling of interval distributions in tonal space reveals paradigmatic stylistic changes in Western music history
Introduction.
Isn't it always the same? This question could be easily posed while listening to the music of any mainstream radio station in a western country. Like language, music is a human universal involving perceptually discrete elements displaying organization 1 . Therefore, contemporary popular music may have a well-established set of underlying patterns and regularities 1 , 2 , 3 , 4 , some of them potentially inherited from the classical tradition 5 , 6 , 7 . Yet, as an incomparable artistic product for conveying emotions 8 , music must incorporate variation over such patterns in order to play upon people's memories and expectations, making it attractive to listeners 3 , 4 , 5 . For the very same reasons, long-term variations of the underlying patterns may also occur across years 9 . Many of these aspects remain formally unknown or lack scientific evidence, specially the latter, which is very often neglected in music-related studies, from musicological analyses to technological applications. The study of patterns and long-term variations in popular music could shed new light on relevant issues concerning its organization, structure and dynamics 10 . More importantly, it addresses valuable questions for the basic understanding of music as one of the main expressions of contemporary culture: Can we identify some of the patterns behind music creation? Do musicians change them over the years? Can we spot differences between new and old music? Is there an ‘evolution’ of musical discourse?
Current technologies for music information processing 11 , 12 provide a unique opportunity to answer the above questions under objective, empirical and quantitative premises. Moreover, akin to recent advances in other cultural assets 13 , they allow for unprecedented large-scale analyses. One of the first publicly-available large-scale collections that has been analyzed by standard music processing technologies is the million song dataset 14 . Among others, the dataset includes the year annotations and audio descriptions of 464,411 distinct music recordings (from 1955 to 2010), which roughly corresponds to more than 1,200 days of continuous listening. Such recordings span a variety of popular genres, including rock, pop, hip hop, metal, or electronic. Explicit descriptions available in the dataset 15 cover three primary and complementary musical facets 2 : loudness, pitch and timbre. Loudness basically correlates with our perception of sound amplitude or volume (notice that we refer to the intrinsic loudness of a recording, not the loudness a listener could manipulate). Pitch roughly corresponds to the harmonic content of the piece, including its chords, melody and tonal arrangements. Timbre accounts for the sound color, texture, or tone quality and can be essentially associated with instrument types, recording techniques and some expressive performance resources. These three music descriptions can be obtained at the temporal resolution of the beat, which is perhaps the most relevant temporal unit in music, specially in western popular music 2 , 4 .
Here we study the music evolution under the aforementioned premises and large-scale resources. By exploiting tools and concepts from statistical physics and complex networks 16 , 17 , 18 , 19 , we unveil a number of statistical patterns and metrics characterizing the general usage of pitch, timbre and loudness in contemporary western popular music. Many of these patterns and metrics remain consistently stable for a period of more than 50 years, which points towards a great degree of conventionalism in the creation and production of this type of music. Yet, we find three important trends in the evolution of musical discourse: the restriction of pitch sequences (with metrics showing less variety in pitch progressions), the homogenization of the timbral palette (with frequent timbres becoming more frequent) and growing average loudness levels (threatening a dynamic richness that has been conserved until today). This suggests that our perception of the new would be essentially rooted on identifying simpler pitch sequences, fashionable timbral mixtures and louder volumes. Hence, an old tune with slightly simpler chord progressions, new instrument sonorities that were in agreement with current tendencies and recorded with modern techniques that allowed for increased loudness levels could be easily perceived as novel, fashionable and groundbreaking.
To identify structural patterns of musical discourse we first need to build a ‘vocabulary’ of musical elements ( Fig. 1 ). To do so, we encode the dataset descriptions by a discretization of their values, yielding what we call music codewords 20 (see Supplementary Information, SI ). In the case of pitch, the descriptions of each song are additionally transposed to an equivalent main tonality, such that all of them are automatically considered within the same tonal context or key. Next, to quantify long-term variations of a vocabulary, we need to obtain samples of it at different periods of time. For that we perform a Monte Carlo sampling in a moving window fashion. In particular, for each year, we sample one million beat-consecutive codewords, considering entire tracks and using a window length of 5 years (the window is centered at the corresponding year such that, for instance, for 1994 we sample one million consecutive beats by choosing full tracks whose year annotation is between 1992 and 1996, both included). This procedure, which is repeated 10 times, guarantees a representative sample with a smooth evolution over the years.
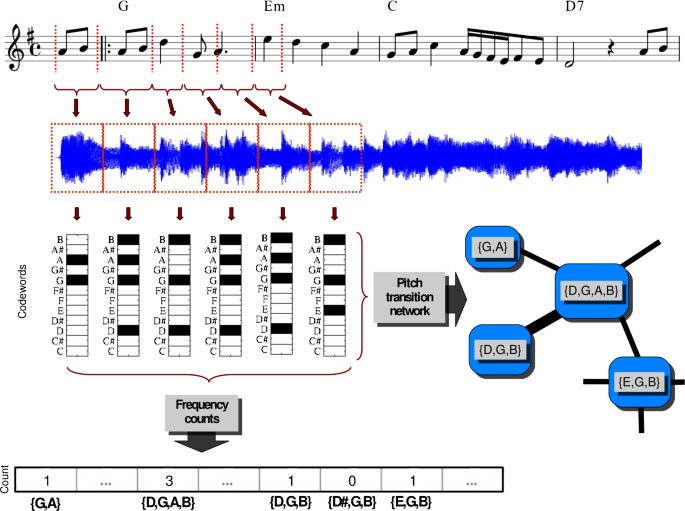
Method schematic summary with pitch data.
The dataset contains the beat-based music descriptions of the audio rendition of a musical piece or score (G, Em and D7 on the top of the staff denote chords). For pitch, these descriptions reflect the harmonic content of the piece 15 and encapsulate all sounding notes of a given time interval into a compact representation 11 , 12 , independently of their articulation (they consist of the 12 pitch class relative energies, where a pitch class is the set of all pitches that are a whole number of octaves apart, e.g. notes C1, C2 and C3 all collapse to pitch class C). All descriptions are encoded into music codewords, using a binary discretization in the case of pitch. Codewords are then used to perform frequency counts and as nodes of a complex network whose links reflect transitions between subsequent codewords.
We first count the frequency of usage of pitch codewords (i.e. the number of times each codeword type appears in a sample). We observe that most used pitch codewords generally correspond to well-known harmonic items 21 , while unused codewords correspond to strange/dissonant pitch combinations ( Fig. 2a ). Sorting the frequency counts in decreasing order provides a very clear pattern behind the data: a power law 17 of the form z ∝ r −α , where z corresponds to the frequency count of a codeword, r denotes its rank (i.e. r = 1 for the most used codeword and so forth) and α is the power law exponent. Specifically, we find that the distribution of codeword frequencies for a given year nicely fits to P ( z ) ∝ ( c + z ) −β for z > z min , where we take z as the random variable 22 , β = 1 + 1/α as the exponent and c as a constant ( Fig. 2b ). A power law indicates that a few codewords are very frequent while the majority are highly infrequent (intuitively, the latter provide the small musical nuances necessary to make a discourse attractive to listeners 3 , 4 , 5 ). Nonetheless, it also states that there is no characteristic frequency nor rank separating most used codewords from largely unused ones (except for the largest rank values due to the finiteness of the vocabulary). Another non-trivial consequence of power-law behavior is that when α ≤ 2, extreme events (i.e. very rare codewords) will certainly show up in a continuous discourse providing the listening time is sufficient and the pre-arranged dictionary of musical elements is big enough.
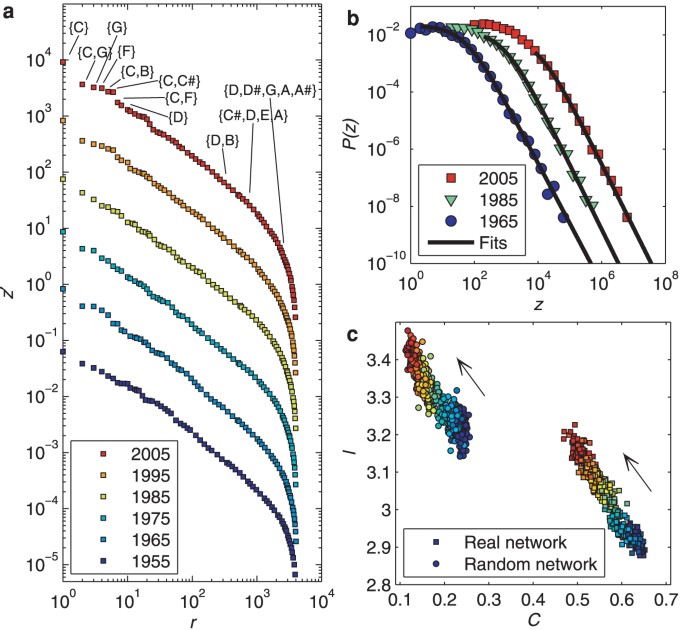
Pitch distributions and networks.

Importantly, we find this power-law behavior to be invariant across years, with practically the same fit parameters. In particular, the exponent β remains close to an average of 2.18 ± 0.06 (corresponding to α around 0.85), which is similar to Zipf's law in linguistic text corpora 23 and contrasts with the exponents found in previous small-scale, symbolic-based music studies 24 , 25 . The slope of the least squares linear regression of β as a function of the year is negligible within statistical significance ( p > 0.05, t-test). This makes a high stability of the distribution of pitch codeword frequencies across more than 50 years of music evident. However, it could well be that, even though the distribution is the same for all years, codeword rankings were changing (e.g. a certain codeword was used frequently in 1963 but became mostly unused by 2005). To assess this possibility we compute the Spearman's rank correlation coefficients 26 for all possible year pairs and find that they are all extremely high, with an average of 0.97 ± 0.02 and a minimum above 0.91. These high correlations indicate that codeword rankings practically do not vary with years.
Codeword frequency distributions provide a generic picture of vocabulary usage. However, they do not account for discourse syntax, as well as a simple selection of words does not necessarily constitute an intelligible sentence. One way to account for syntax is to look at local interactions or transitions between codewords, which define explicit relations that capture most of the underlying regularities of the discourse and that can be directly mapped into a network or graph 18 , 19 . Hence, analogously to language-based analyses 27 , 28 , 29 , we consider the transition networks formed by codeword successions, where each node represents a codeword and each link represents a transition (see SI ). The topology of these networks and common metrics extracted from them can provide us with valuable clues about the evolution of musical discourse.
All the transition networks we obtain are sparse, meaning that the number of links connecting codewords is of the same order of magnitude as the number of codewords. Thus, in general, only a limited number of transitions between codewords is possible. Such constraints would allow for music recognition and enjoyment, since these capacities are grounded in our ability for guessing/learning transitions 3 , 4 , 8 and a non-sparse network would increase the number of possibilities in a way that guessing/learning would become unfeasible. Thinking in terms of originality and creativity, a sparse network means that there are still many ‘composition paths’ to be discovered. However, some of these paths could run into the aforementioned guessing/learning tradeoff 9 . Overall, network sparseness provides a quantitative account of music's delicate balance between predictability and surprise.
In sparse networks, the most fundamental characteristic of a codeword is its degree k , which measures the number of links to other codewords. With pitch networks, this quantity is distributed according to a power law P ( k ) ∝ k −γ for k > k min , with the same fit parameters for all considered years. The exponent γ, which has an average of 2.20±0.06, is similar to many other real complex networks 18 and the median of the degree k is always 4. Nevertheless, we observe important trends in the other considered network metrics, namely the average shortest path length l , the clustering coefficient C and the assortativity with respect to random Γ. Specifically, l slightly increases from 2.9 to 3.2, values comparable to the ones obtained when randomizing the network links. The values of C show a considerable decrease from 0.65 to 0.45 and are much higher than those obtained for the randomized network. Thus, the small-worldness 30 of the networks decreases with years ( Fig. 2c ). This trend implies that the reachability of a pitch codeword becomes more difficult. The number of hops or steps to jump from one codeword to the other (as reflected by l ) tends to increase and, at the same time, the local connectivity of the network (as reflected by C ) tends to decrease. Additionally, Γ is always below 1, which indicates that the networks are always less assortative than random (i.e. well-connected nodes are less likely to be connected among them), a tendency that grows with time if we consider the biggest hubs of the network ( SI ). The latter suggests that there are less direct transitions between ‘referential’ or common codewords. Overall, a joint reduction of the small-worldness and the network assortativity shows a progressive restriction of pitch transitions, with less transition options and more defined paths between codewords.
As opposed to pitch, timbre provides a different picture. Even though the distribution of timbre codeword frequencies is also well-fitted by a power law ( Fig. 3a ), the parameters of this distribution vary across years. In particular, since 1965, β constantly decreases to values approaching 4 ( Fig. 3b ). Although such large values of β would imply that other fits could also be acceptable, the power law provides a simple parameterization to compare the changes over the years (and is not rejected in a likelihood ratio test in front of other alternatives). Smaller values of β indicate less timbral variety: frequent codewords become more frequent and infrequent ones become even less frequent. This evidences a growing homogenization of the global timbral palette. It also points towards a progressive tendency to follow more fashionable, mainstream sonorities. Interestingly, rank correlation coefficients are generally below 0.7, with an average of 0.57 ± 0.15 ( Fig. 3c ). These rather low rank correlations would act as an attenuator of the sensation that contemporary popular music is becoming more homogeneous, timbrically speaking. The fact that frequent timbres of a certain time period become infrequent after some years could mask global homogeneity trends to listeners.
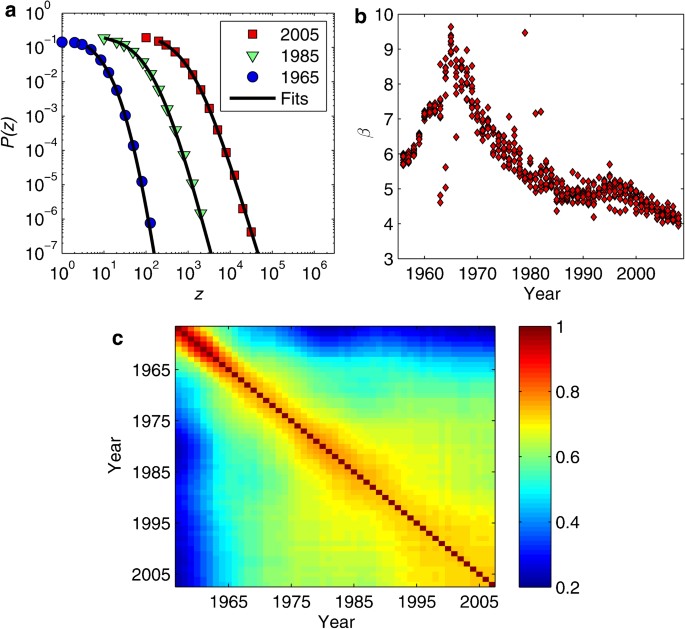
Timbre distributions.
(a) Examples of the density values and fits taking z as the random variable. (b) Fitted exponents β. (c) Spearman's rank correlation coefficients for all possible year pairs.
Compared to timbre codeword frequencies, metrics obtained from timbre transition networks show no substantial variation. Again, similar median degrees (all equal to 8) and degree distributions were observed for all considered years. However, we were not able to achieve a proper fit for the latter ( SI ). Values of Γ are larger than 1 and increasing since 1965. Thus, in contrast to pitch, timbre networks are more assortative than random. The values of l fluctuate around 4.8 and C is always below 0.01. Noticeably, both are close to the values obtained with randomly wired networks. This close to random topology quantitatively demonstrates that, as opposed to language, timbral contrasts (or transitions) are rarely the basis for a musical discourse 1 . This does not regard timbre as a meaningless facet. Global timbre properties, like the aforementioned power law and rankings, are clearly important for music categorization tasks 2 , 11 (one example is genre classification 31 ). Notice however that the evolving characteristics of musical discourse have important implications for artificial or human systems dealing with such tasks. For instance, the homogenization of the timbral palette and general timbral restrictions clearly challenge tasks exploiting this facet. A further example is found with the aforementioned restriction of pitch codeword connectivity, which could hinder song recognition systems (artificial song recognition systems are rooted on pitch codeword-like sequences, cf. 32 ).
Loudness distributions are generally well-fitted by a reversed log-normal function ( Fig. 4a ). Plotting them provides a visual account of the so-called loudness race (or loudness war), a terminology that is used to describe the apparent competition to release recordings with increasing loudness 33 , 34 , perhaps with the aim of catching potential customers' attention in a music broadcast (from our point of view, loudness changes are not only the result of technological developments but, in part, also the result of conscious decisions made by musicians and producers in the musical creation process, cf. 33 ). The empiric median of the loudness values x grows from −22 dB FS to −13 dB FS ( Fig. 4b ), with a least squares linear regression yielding a slope of 0.13 dB/year ( p < 0.01, t-test). In contrast, the absolute difference between the first and third quartiles of x remains constant around 9.5 dB ( Fig. 4c ), with a regression slope that is not statistically significant ( p > 0.05, t-test). This shows that, although music recordings become louder, their absolute dynamic variability has been conserved, understanding dynamic variability as the range between higher and lower loudness passages of a recording 34 . However and perhaps most importantly, one should notice that digital media cannot output signals over 0 dB FS 35 , which severely restricts the possibilities for maintaining the dynamic variability if the median continues to grow.
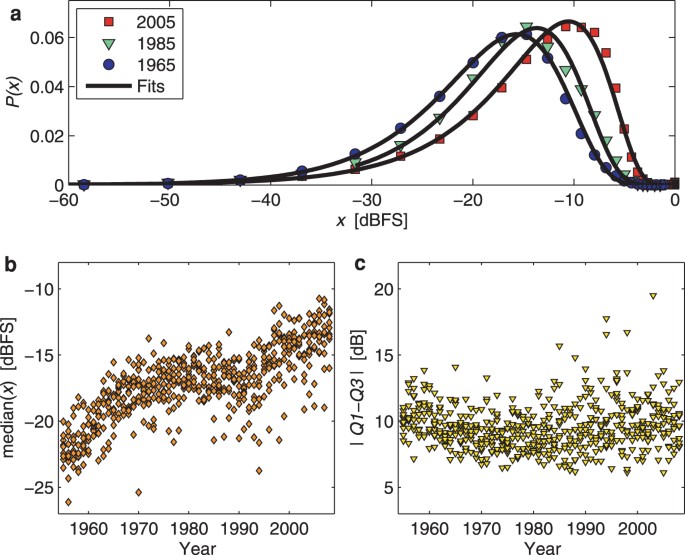
Loudness distributions.
(a) Examples of the density values and fits of the loudness variable x . (b) Empiric distribution medians. (c) Dynamic variability, expressed as absolute loudness differences between the first and third quartiles of x , | Q 1 − Q 3 |.
Finally we look at loudness transition networks, which show comparable degree distributions, a median degree between 13 and 14, values of l between 8 and 10 and a Γ fluctuating around 1.08. Noticeably, l is appreciably beyond the values obtained by randomly wired networks. The values of C have an average of 0.59 ± 0.02, also much above the values obtained by the random networks. These two observations suggest that the network has a one-dimensional character, inferring that no extreme loudness transitions occur (one rarely finds loudness transitions to drive a musical discourse). The very stable metrics obtained for loudness networks imply that, despite the race towards louder music, the topology of loudness transitions is maintained.
Beyond the specific outcomes discussed above, we now focus on the evolution of musical discourse. Much of the gathered evidence points towards an important degree of conventionalism, in the sense of blockage or no-evolution, in the creation and production of contemporary western popular music. Thus, from a global perspective, popular music would have no clear trends and show no considerable changes in more than fifty years. Pitch codeword frequencies are found to be always under the same underlying pattern: a power law with the same exponent and fitting parameters. Moreover, frequency-based rankings of pitch codewords are practically identical and several of the network metrics for pitch, timbre and loudness remain immutable. Frequency distributions for timbre and loudness also fall under a universal pattern: a power law and a reversed log-normal distribution, respectively. However, these distributions' parameters do substantially change with years. In addition, some metrics for pitch networks clearly show a progression. Thus, beyond the global perspective, we observe a number of trends in the evolution of contemporary popular music. These point towards less variety in pitch transitions, towards a consistent homogenization of the timbral palette and towards louder and, in the end, potentially poorer volume dynamics.
Each of us has a perception of what is new and what is not in popular music. According to our findings, this perception should be largely rooted on the simplicity of pitch sequences, the usage of relatively novel timbral mixtures that are in agreement with the current tendencies and the exploitation of modern recording techniques that allow for louder volumes. This brings us to conjecture that an old popular music piece would be perceived as novel by essentially following these guidelines. In fact, it is informally known that a ‘safe’ way for contemporizing popular music tracks is to record a new version of an existing piece with current means, but without altering the main ‘semantics’ of the discourse.
Some of the conclusions reported here have historically remained as conjectures, based on restricted resources, or rather framed under subjective, qualitative and non-systematic premises. With the present work, we gain empirical evidence through a formal, quantitative and systematic analysis of a large-scale music collection. We encourage the development of further historical databases to be able to quantify the major transitions in the history of music and to start looking at more subtle evolving characteristics of particular genres or artists, without forgetting the whole wealth of cultures and music styles present in the world.
Detailed method descriptions are provided in the SI .
Patel, A. D. Music, language and the brain (Oxford University Press, Oxford, UK, 2007).
Ball, P. The music instinct: how music works and why we can't do without it (Bodley Head, London, UK, 2010).
Huron, D. Sweet anticipation: music and the psychology of expectation (MIT Press, Cambridge, USA, 2006).
Honing, H. Musical cognition: a science of listening (Transaction Publishers, Piscataway, USA, 2011).
Levitin, D. J., Chordia, P. & Menon, V. Musical rhythm spectra from Bach to Joplin obey a 1/f power law. Proc. of the National Academy of Sciences of the USA 109, 3716–3720 (2012).
Article CAS ADS Google Scholar
Lerdahl, F. & Jackendoff, R. A generative theory of tonal music (MIT Press, Cambridge, USA, 1983).
Temperley, D. Music and probability (MIT Press, Cambridge, USA, 2007).
Juslin, P. & Sloboda, J. A. Music and emotion: theory and research (Oxford University Press, Oxford, UK, 2001).
Reynolds, R. The evolution of sensibility. Nature 434, 316–319 (2005).
Zanette, D. H. Playing by numbers. Nature 453, 988–989 (2008).
Casey, M. A. et al. Content-based music information retrieval: current directions and future challenges. Proc. of the IEEE 96, 668–696 (2008).
Article Google Scholar
Müller, M., Ellis, D. P. W., Klapuri, A. & Richard, G. Signal processing for music analysis. IEEE Journal of Selected Topics in Signal Processing 5, 1088–1110 (2011).
Article ADS Google Scholar
Michel, J.-B. et al. Quantitative analysis of culture using millions of digitized books. Science 331, 176–182 (2011).
Bertin-Mahieux, T., Ellis, D. P. W., Whitman, B. & Lamere, P. The million song dataset. In: Proc. of the Int. Soc. for Music Information Retrieval Conf. (ISMIR), 591–596 (2011).
Jehan, T. Creating music by listening. Ph.D. thesis, Massachussets Institute of Technology, Cambridge, USA (2005).
Bak, P. How nature works: the science of self-organized criticality (Copernicus, New York, USA, 1996).
Newman, M. E. J. Power laws, Pareto distributions and Zipf's law. Contemporary Physics 46, 323–351 (2005).
Newman, M. E. J. Networks: an introduction (Oxford University Press, Oxford, UK, 2010).
Barrat, A., Barthélemy, M. & Vespignani, A. Dynamical processes on complex networks (Cambridge University Press, Cambridge, UK, 2008).
Haro, M., Serrà, J., Herrera, P. & Corral, A. Zipf's law in short-time timbral codings of speech, music and environmental sound signals. PLoS ONE 7, e33993 (2012).
De Clercq, T. & Temperley, D. A corpus analysis of rock harmony. Popular Music 30, 47–70 (2011).
Adamic, L. A. & Huberman, B. A. Zipf's law and the internet. Glottometrics 3, 143–150 (2002).
Google Scholar
Zipf, G. K. Human behavior and the principle of least effort (Addison-Wesley, Boston, USA, 1949).
Zanette, D. Zipf's law and the creation of musical context. Musicae Scientiae 10, 3–18 (2006).
Beltrán del Río, M., Cocho, G. & Naumis, G. G. Universality in the tail of musical note rank distribution. Physica A 387, 5552–5560 (2008).
Hollander, M. & Wolfe, D. A. Nonparametric statistical methods (Wiley, New York, USA, 1999), 2nd edn.
Sigman, M. & Cecchi, G. A. Global organization of the Wordnet lexicon. Proc. of the National Academy of Sciences of the USA 99, 1742–1747 (2002).
Ferrer i Cancho, R., Solé, R. V. & Köhler, R. Patterns in syntactic dependency networks. Physical Review E 69, 051915 (2004).
Amancio, D. R., Altmann, E. G., Oliveira, O. N. & Costa, L. d. F. Comparing intermittency and network measurements of words and their dependence on authorship. New Journal of Physics 13, 123024 (2011).
Watts, D. J. & Strogatz, S. H. Collective dynamics of ‘small-world’ networks. Nature 393, 440–442 (1998).
Scaringella, N., Zoia, G. & Mlynek, D. Automatic genre classification of music content: a survey. Signal Processing Magazine 23, 133–141 (2006).
Serrà, J., Gómez, E. & Herrera, P. Audio cover song identification and similarity: background, approaches, evaluation and beyond. In Raś Z. W., & Wieczorkowska A. A., (eds.) Advances in Music Information Retrieval , vol. 274 of Studies in Computational Intelligence , chap. 14, 307–332 (Springer, Berlin, Germany, 2010).
Milner, G. Perfecting sound forever: an aural history of recorded music (Faber and Faber, London, UK, 2009).
Deruty, E. ‘Dynamic range’ and the loudness war. Sound on Sound – September 2011 22–24 (2011).
Oppenheim, A. V., Schafer, R. W. & Buck, J. R. Discrete-time signal processing (Prentice-Hall, Upper Saddle River, USA, 1999), 2nd edn.
Download references
Acknowledgements
This work was partially supported by Catalan Government grants 2009-SGR-164 (A.C.), 2009-SGR-1434 (J.S. and J.Ll.A.), 2009-SGR-838 (M.B.) and ICREA Academia Prize 2010 (M.B.), European Comission grant FP7-ICT-2011.1.5-287711 (M.H.), Spanish Government grants FIS2009-09508 (A.C.), FIS2010-21781-C02-02 (M.B.) and TIN2009-13692-C03-01 (J.Ll.A.) and Spanish National Research Council grant JAEDOC069/2010 (J.S.). The authors would like to thank the million song dataset team for making this massive source of data publicly available.
Author information
Authors and affiliations.
Artificial Intelligence Research Institute, Spanish National Research Council (IIIA-CSIC), Bellaterra, Barcelona, Spain
Joan Serrà & Josep Ll. Arcos
Complex Systems Group, Centre de Recerca Matemàtica, Bellaterra, Barcelona, Spain
- Álvaro Corral
Departament de Física Fonamental, Universitat de Barcelona, Barcelona, Spain
Marián Boguñá
Music Technology Group, Universitat Pompeu Fabra, Barcelona, Spain
Martín Haro
You can also search for this author in PubMed Google Scholar
Contributions
J.S., A.C. and M.B. performed research. All authors designed research, discussed results and wrote the paper.
Ethics declarations
Competing interests.
The authors declare no competing financial interests.
Electronic supplementary material
Supplementary Information
Rights and permissions
This work is licensed under a Creative Commons Attribution-NonCommercial-ShareALike 3.0 Unported License. To view a copy of this license, visit http://creativecommons.org/licenses/by-nc-sa/3.0/
Reprints and permissions
About this article
Cite this article.
Serrà, J., Corral, Á., Boguñá, M. et al. Measuring the Evolution of Contemporary Western Popular Music. Sci Rep 2 , 521 (2012). https://doi.org/10.1038/srep00521
Download citation
Received : 11 May 2012
Accepted : 04 July 2012
Published : 26 July 2012
DOI : https://doi.org/10.1038/srep00521
Share this article
Anyone you share the following link with will be able to read this content:
Sorry, a shareable link is not currently available for this article.
Provided by the Springer Nature SharedIt content-sharing initiative
This article is cited by
Trajectories and revolutions in popular melody based on u.s. charts from 1950 to 2023.
- Madeline Hamilton
- Marcus Pearce
Scientific Reports (2024)
Originality, influence, and success: a model of creative style
- Karol Jan Borowiecki
- Caterina Adelaide Mauri
Journal of Cultural Economics (2023)
Magnetoencephalography recordings reveal the spatiotemporal dynamics of recognition memory for complex versus simple auditory sequences
- Gemma Fernández-Rubio
- Elvira Brattico
- Leonardo Bonetti
Communications Biology (2022)
Lognormals, power laws and double power laws in the distribution of frequencies of harmonic codewords from classical music
- Marc Serra-Peralta
Scientific Reports (2022)
- Ben Lambert
- Georgios Kontonatsios
- Armand M. Leroi
Nature Human Behaviour (2020)
By submitting a comment you agree to abide by our Terms and Community Guidelines . If you find something abusive or that does not comply with our terms or guidelines please flag it as inappropriate.
Quick links
- Explore articles by subject
- Guide to authors
- Editorial policies
Sign up for the Nature Briefing: AI and Robotics newsletter — what matters in AI and robotics research, free to your inbox weekly.

Mining Modern Music: The Classification of Popular Songs
- Conference paper
- First Online: 30 October 2021
- Cite this conference paper
- Caitlin Genna 8
Part of the book series: Transactions on Computational Science and Computational Intelligence ((TRACOSCI))
2430 Accesses
1 Citations
The rising popularity of streaming services has made in-depth musical data more accessible than ever before and has created new opportunities for data mining. This project utilizes data from 19,000 songs made available by Spotify. Several data mining algorithms (including J48 Decision Trees, Random Forest, Simple K Means, NaiveBayes, ZeroR, and JRIP) were used to assess the data as a classification task with the target class being popularity. The data was pre-processed and the popularity class was split into two different schemes, both of which were used to train the aforementioned algorithms with the goal of attaining the highest possible classification accuracy. Once reliable models were produced, the best performing algorithms were used in conjunction with association algorithms and Information Gain evaluation in order to assess the importance of features such as key, acousticness, tempo, instrumentalness, etc., in the prediction of the popularity class. Through this lens certain groups of attributes emerged as indicators of what makes a song popular or unpopular, and relationships between the attributes themselves were revealed as well. Overall it was concluded that popular music does in fact have patterns and a formulaic nature, making the “art” of creating music seem more like a science. However, within those patterns enough variation can be seen to account for different genres and musical moods that still persist in this era of pop music, and support the idea that as a modern musical community we still maintain some diversity.
This is a preview of subscription content, log in via an institution to check access.
Access this chapter
Subscribe and save.
- Get 10 units per month
- Download Article/Chapter or eBook
- 1 Unit = 1 Article or 1 Chapter
- Cancel anytime
- Available as PDF
- Read on any device
- Instant download
- Own it forever
- Available as EPUB and PDF
- Compact, lightweight edition
- Dispatched in 3 to 5 business days
- Free shipping worldwide - see info
- Durable hardcover edition
Tax calculation will be finalised at checkout
Purchases are for personal use only
Institutional subscriptions
Similar content being viewed by others
Harmonizing Insights: Python-Based Data Analysis of Spotify's Musical Tapestry
Comparing Audio Features and Playlist Statistics for Music Classification
Electronic Dance Music Sub-genre Classification Using Machine Learning
F. Fibe, I.H. Witten, Weka 3.8 (Hamilton, New Zealand, 1993)
Google Scholar
“Get Audio Features for a Track,” Spotify for Developers
E. Ramirez, 19,000 Spotify Songs (Kaggle, 2019)
J.D.D. Santos, Is my Spotify music boring? An analysis involving music, data, and machine learning. Towards Data Sci (2017)
Download references
Acknowledgments
I thank Dr. Gary Weiss (Fordham University) for sparking my interest in the powerful field of data mining.
Author information
Authors and affiliations.
Fordham University, New York City, NY, USA
Caitlin Genna
You can also search for this author in PubMed Google Scholar
Editor information
Editors and affiliations.
HBS – Hamburg Business School, Institute of Information Systems, University of Hamburg, Hamburg, Hamburg, Germany
Robert Stahlbock
Department of Computer & Information Science, Fordham University, New York, NY, USA
Gary M. Weiss
College of Engineering & Computer Science, University of Michigan-Dearborn, Dearborn, MI, USA
Mahmoud Abou-Nasr
Department of Computer Science, University of Taipei, Taipei City, Taiwan
Cheng-Ying Yang
Department of Computer Science, University of Georgia, Athens, GA, USA
Hamid R. Arabnia
School of Computing and Data Sciences, Wentworth Institute of Technology, Boston, MA, USA
Leonidas Deligiannidis
Rights and permissions
Reprints and permissions
Copyright information
© 2021 Springer Nature Switzerland AG
About this paper
Cite this paper.
Genna, C. (2021). Mining Modern Music: The Classification of Popular Songs. In: Stahlbock, R., Weiss, G.M., Abou-Nasr, M., Yang, CY., Arabnia, H.R., Deligiannidis, L. (eds) Advances in Data Science and Information Engineering. Transactions on Computational Science and Computational Intelligence. Springer, Cham. https://doi.org/10.1007/978-3-030-71704-9_43
Download citation
DOI : https://doi.org/10.1007/978-3-030-71704-9_43
Published : 30 October 2021
Publisher Name : Springer, Cham
Print ISBN : 978-3-030-71703-2
Online ISBN : 978-3-030-71704-9
eBook Packages : Mathematics and Statistics Mathematics and Statistics (R0)
Share this paper
Anyone you share the following link with will be able to read this content:
Sorry, a shareable link is not currently available for this article.
Provided by the Springer Nature SharedIt content-sharing initiative
- Publish with us
Policies and ethics
- Find a journal
- Track your research

An official website of the United States government
The .gov means it’s official. Federal government websites often end in .gov or .mil. Before sharing sensitive information, make sure you’re on a federal government site.
The site is secure. The https:// ensures that you are connecting to the official website and that any information you provide is encrypted and transmitted securely.
- Publications
- Account settings
Preview improvements coming to the PMC website in October 2024. Learn More or Try it out now .
- Advanced Search
- Journal List
- Front Psychol

The psychological functions of music listening
Thomas schäfer.
1 Department of Psychology, Chemnitz University of Technology, Chemnitz, Germany
Peter Sedlmeier
Christine städtler, david huron.
2 School of Music, Cognitive and Systematic Musicology Laboratory, Ohio State University, Columbus, OH, USA
Why do people listen to music? Over the past several decades, scholars have proposed numerous functions that listening to music might fulfill. However, different theoretical approaches, different methods, and different samples have left a heterogeneous picture regarding the number and nature of musical functions. Moreover, there remains no agreement about the underlying dimensions of these functions. Part one of the paper reviews the research contributions that have explicitly referred to musical functions. It is concluded that a comprehensive investigation addressing the basic dimensions underlying the plethora of functions of music listening is warranted. Part two of the paper presents an empirical investigation of hundreds of functions that could be extracted from the reviewed contributions. These functions were distilled to 129 non-redundant functions that were then rated by 834 respondents. Principal component analysis suggested three distinct underlying dimensions: People listen to music to regulate arousal and mood , to achieve self-awareness , and as an expression of social relatedness . The first and second dimensions were judged to be much more important than the third—a result that contrasts with the idea that music has evolved primarily as a means for social cohesion and communication. The implications of these results are discussed in light of theories on the origin and the functionality of music listening and also for the application of musical stimuli in all areas of psychology and for research in music cognition.
Introduction
Music listening is one of the most enigmatic of human behaviors. Most common behaviors have a recognizable utility that can be plausibly traced to the practical motives of survival and procreation. Moreover, in the array of seemingly odd behaviors, few behaviors match music for commandeering so much time, energy, and money. Music listening is one of the most popular leisure activities. Music is a ubiquitous companion to people's everyday lives.
The enthusiasm for music is not a recent development. Recognizably musical activities appear to have been present in every known culture on earth, with ancient roots extending back 250,000 years or more (see Zatorre and Peretz, 2001 ). The ubiquity and antiquity of music has inspired considerable speculation regarding its origin and function.
Throughout history, scholars of various stripes have pondered the nature of music. Philosophers, psychologists, anthropologists, musicologists, and neuroscientists have proposed a number of theories concerning the origin and purpose of music and some have pursued scientific approaches to investigating them (e.g., Fitch, 2006 ; Peretz, 2006 ; Levitin, 2007 ; Schäfer and Sedlmeier, 2010 ).
The origin of music is shrouded in prehistory. There is little physical evidence—like stone carvings or fossilized footprints—that might provide clues to music's past. Necessarily, hypotheses concerning the original functions of music will remain speculative. Nevertheless, there are a number of plausible and interesting conjectures that offer useful starting-points for investigating the functions of music.
A promising approach to the question of music's origins focuses on how music is used—that is, it's various functions. In fact, many scholars have endeavored to enumerate various musical functions (see below). The assumption is that the function(s) that music is presumed to have served in the past would be echoed in at least one of the functions that music serves today. Of course, how music is used today need have no relationship with music's function(s) in the remote past. Nevertheless, evidence from modern listeners might provide useful clues pertinent to theorizing about origins.
In proposing various musical functions, not all scholars have related these functions to music's presumed evolutionary roots. For many scholars, the motivation has been simply to identify the multiple ways in which music is used in everyday lives (e.g., Chamorro-Premuzic and Furnham, 2007 ; Boer, 2009 ; Lonsdale and North, 2011 ; Packer and Ballantyne, 2011 ). Empirical studies of musical functions have been very heterogeneous. Some studies were motivated by questions related to development. Many related to social identity. Others were motivated by cognitive psychology, aesthetics, cultural psychology, or personality psychology. In addition, studies differed according to the target population. While some studies attempted to assemble representative samples of listeners, others explicitly focused on specific populations such as adolescents. Most studies rely on convenient samples of students. Consequently, the existing literature is something of a hodgepodge.
The aim of the present study is to use the extant literature as a point of departure for a fresh re-appraisal of possible musical functions. In Part 1 of our study, we summarize the results of an extensive literature survey concerning the possible functions of music. Specifically, we identified and skimmed hundreds of publications that explicitly suggest various functions, uses, or benefits for music. We provide separate overviews for the empirical literatures and the theoretical literatures. This survey resulted in just over 500 proposed musical functions. We do not refer to each of the identified publications but concentrate on the ones that have identified either more than one single function of music listening or a single unique function that is not captured in any other publication. In Part 2, we present the results of an empirical study whose purpose was to distill—using principal components analysis (PCA)—the many proposed functions of music listening. To anticipate our results, we will see that PCA suggests three main dimensions that can account for much of the shared variance in the proposed musical functions.
Review of the research on the functions of music
Discussions and speculations regarding the functions of music listening can be found in both theoretical literature concerning music as well as in empirical studies of music. Below, we offer a review of both literatures. The contents of the reviews are summarized in Tables TablesA1, A1 , ,A2. A2 . Table TableA1 A1 provides an overview of theoretical proposals regarding musical function, whereas Table TableA2 A2 provides an overview of empirical studies regarding musical function. Together, the two tables provide a broad inventory of potential functions for music.
Theoretical approaches
Many scholars have discussed potential functions of music exclusively from a theoretical point of view. The most prominent of these approaches or theories are the ones that make explicit evolutionary claims. However, there are also other, non-evolutionary approaches such as experimental aesthetics or the uses-and-gratifications approach. Functions of music were derived deductively from these approaches and theories. In addition, in the literature, one commonly finds lists or collections of functions that music can have. Most of these lists are the result of literature searches; in other cases authors provide no clear explanation for how they came up with the functions they list. Given the aim of assembling a comprehensive list, all works are included in our summary.
Functions of music as they derive from specific approaches or theories
Evolutionary approaches. Evolutionary discussions of music can already be found in the writings of Darwin. Darwin discussed some possibilities but felt there was no satisfactory solution to music's origins (Darwin, 1871 , 1872 ). His intellectual heirs have been less cautious. Miller ( 2000 ), for instance, has argued that music making is a reasonable index of biological fitness, and so a manifestation of sexual selection—analogous to the peacock's tail. Anyone who can afford the biological luxury of making music must be strong and healthy. Thus, music would offer an honest social signal of physiological fitness.
Another line of theorizing refers to music as a means of social and emotional communication. For example, Panksepp and Bernatzky ( 2002 , p. 139) argued that
in social creatures like ourselves, whose ancestors lived in arboreal environments where sound was one of the most effective ways to coordinate cohesive group activities, reinforce social bonds, resolve animosities, and to establish stable hierarchies of submission and dominance, there could have been a premium on being able to communicate shades of emotional meaning by the melodic character (prosody) of emitted sounds.
A similar idea is that music contributes to social cohesion and thereby increases the effectiveness of group action. Work and war songs, lullabies, and national anthems have bound together families, groups, or whole nations. Relatedly, music may provide a means to reduce social stress and temper aggression in others. The idea that music may function as a social cement has many proponents (see Huron, 2001 ; Mithen, 2006 ; Bicknell, 2007 ).
A novel evolutionary theory is offered by Falk ( 2004a , b ) who has proposed that music arose from humming or singing intended to maintain infant-mother attachment. Falk's “putting-down-the-baby hypothesis” suggests that mothers would have profited from putting down their infants in order to make their hands free for other activities. Humming or singing consequently arose as a consoling signal indicating caretaker proximity in the absence of physical touch.
Another interesting conjecture relates music to human anxiety related to death, and the consequent quest for meaning. Dissanayake ( 2009 ), for example, has argued that humans have used music to help cope with awareness of life's transitoriness. In a manner similar to religious beliefs about the hereafter or a higher transcendental purpose, music can help assuage human anxiety concerning mortality (see, e.g., Newberg et al., 2001 ). Neurophysiological studies regarding music-induced chills can be interpreted as congruent with this conjecture. For example, music-induced chills produce reduced activity in brain structures associated with anxiety (Blood and Zatorre, 2001 ).
Related ideas stress the role music plays in feelings of transcendence. For example, (Frith, 1996 , p. 275) has noted that: “We all hear the music we like as something special, as something that defies the mundane, takes us “out of ourselves,” puts us somewhere else.” Thus, music may provide a means of escape. The experience of flow states (Nakamura and Csikszentmihalyi, 2009 ), peaks (Maslow, 1968 ), and chills (Panksepp, 1995 ), which are often evoked by music listening, might similarly be interpreted as forms of transcendence or escapism (see also Fachner, 2008 ).
More generally, Schubert ( 2009 ) has argued that the fundamental function of music is its potential to produce pleasure in the listener (and in the performer, as well). All other functions may be considered subordinate to music's pleasure-producing capacity. Relatedly, music might have emerged as a safe form of time-passing—analogous to the sleeping behaviors found among many predators. As humans became more effective hunters, music might have emerged merely as an entertaining and innocuous way to pass time during waking hours (see Huron, 2001 ).
The above theories each stress a single account of music's origins. In addition, there are mixed theories that posit a constellation of several concurrent functions. Anthropological accounts of music often refer to multiple social and cultural benefits arising from music. Merriam ( 1964 ) provides a seminal example. In his book, The anthropology of music , Merriam proposed 10 social functions music can serve (e.g., emotional expression, communication, and symbolic representation). Merriam's work has had a lasting influence among music scholars, but also led many scholars to focus exclusively on the social functions of music. Following in the tradition of Merriam, Dissanayake ( 2006 ) proposed six social functions of ritual music (such as display of resources, control, and channeling of individual aggression, and the facilitation of courtship).
Non-evolutionary approaches. Many scholars have steered clear of evolutionary speculation about music, and have instead focused on the ways in which people use music in their everyday lives today. A prominent approach is the “uses-and-gratifications” approach (e.g., Arnett, 1995 ). This approach focuses on the needs and concerns of the listeners and tries to explain how people actively select and use media such as music to serve these needs and concerns. Arnett ( 1995 ) provides a list of potential uses of music such as entertainment, identity formation, sensation seeking, or culture identification.
Another line of research is “experimental aesthetics” whose proponents investigate the subjective experience of beauty (both artificial or natural), and the ensuing experience of pleasure. For example, in discussing the “recent work in experimental aesthetics,” Bullough ( 1921 ) distinguished several types of listeners and pointed to the fact that music can be used to activate associations, memories, experiences, moods, and emotions.
By way of summary, many musical functions have been proposed in the research literature. Evolutionary speculations have tended to focus on single-source causes such as music as an indicator of biological fitness, music as a means for social and emotional communication, music as social glue, music as a way of facilitating caretaker mobility, music as a means of tempering anxiety about mortality, music as escapism or transcendental meaning, music as a source of pleasure, and music as a means for passing time. Other accounts have posited multiple concurrent functions such as the plethora of social and cultural functions of music found in anthropological writings about music. Non-evolutionary approaches are evident in the uses-and-gratifications approach—which revealed a large number of functions that can be summarized as cognitive, emotional, social, and physiological functions—and the experimental aesthetics approach, whose proposed functions can similarly be summarized as cognitive and emotional functions.
Functions of music as they derive from literature research
As noted, many publications posit musical functions without providing a clear connection to any theory. Most of these works are just collections of functions of music from the literature. Not least, there are also accounts of such collections where it remained unclear how the author(s) came up with the functions contained. Some of these works refer to only one single function of music—most often because this functional aspect was investigated not with the focus on music but with a focus on other psychological phenomena. Yet other works list extensive collections of purported musical functions.
Works that refer to only one single functional aspect of music include possible therapeutic functions for music in clinical settings (Cook, 1986 ; Frohne-Hagemann and Pleß-Adamczyk, 2005 ), the use of music for symbolic exclusion in political terms (Bryson, 1996 ), the syntactic, semantic, and mediatizing use of film music (Maas, 1993 ), and the use of music to manage physiological arousal (Bartlett, 1996 ).
The vast majority of publications identify several possible musical functions, most of which—as stated above—are clearly focused on social aspects. Several comprehensive collections have been assembled, such as those by Baacke ( 1984 ), Gregory ( 1997 ), Ruud ( 1997 ), Roberts and Christenson ( 2001 ), Engh ( 2006 ), and Laiho ( 2004 ). Most of these studies identified a very large number of potential functions of music.
By way of summary, there exists a long tradition of theorizing about the potential functions of music. Although some of these theories have been deduced from a prior theoretical framework, none was the result of empirical testing or exploratory data-gathering. In the ensuing section, we turn to consider empirically-oriented research regarding the number and nature of potential musical functions.
Empirical investigations
A number of studies have approached the functions of music from an empirical perspective. Two main approaches might be distinguished. In the first approach, the research aim is to uncover or document actual musical functioning. That is, the research aims to observe or identify one or more ways in which music is used in daily life. In the second approach, the research goal is to infer the structure or pattern underlying the use of music. That is, the research aims to uncover potential basic or fundamental dimensions implied by the multiple functions of music. This is mostly done using PCA or factor analyses or cluster analyses that reduce a large number of functions to only a few basic dimensions. In some cases, the analyses are run exploratively whereas in other cases, they are run in a confirmatory way, that is—with a predefined number of dimensions. The empirical studies can be categorized according to several criteria (see Table TableA2). A2 ). However, when discussing some of the most important works here, we will separate studies where respondents were asked for the functions of music in open surveys from studies where the authors provided their own collections of functions, based on either literature research or face validity.
Surveys about the functions music can have
A number of studies have attempted to chronicle the broad range of musical functions. Most of these studies employed surveys in which people were asked to identify the ways in which they make use of music in their lives. In some studies, expert interviews were conducted in order to identify possible functions. Table TableA2 A2 provides a summary of all the pertinent studies including their collections of functions and—where applicable—their derived underlying dimensions. We will restrict our ensuing remarks to the largest and most comprehensive studies.
Chamorro-Premuzic and Furnham ( 2007 ) identified 15 functions of music among students and subsequently ran focus groups from which they distilled three distinct dimensions: emotional use, rational use, and background use. Some of the largest surveys have been carried out by Boer ( 2009 ). She interviewed more than a thousand young people in different countries and assembled a comprehensive collection of musical functions. Using factor analysis, she found 10 underlying dimensions: emotion, friends, family, venting, background, dancing, focus, values, politic, and culture. (Lonsdale and North, 2011 , Study 1) pursued a uses-and-gratifications approach. They identified 30 musical uses that could be reduced to six distinct dimensions. In a related study employing a larger sample, the same authors came up with eight distinct dimensions: identity, positive and negative mood management, reminiscing, diversion, arousal, surveillance, and social interaction (Lonsdale and North, 2011 , Study 4). When interviewing older participants, Hays and Minichiello ( 2005 ) qualitatively identified six dimensions: linking, life events, sharing and connecting, wellbeing, therapeutic benefits, escapism, and spirituality.
The various surveys and interview studies clearly diverge with regard to the number of different musical functions. Similarly, the various cluster and factor analyses often end up producing different numbers of distinct dimensions. Nevertheless, the results are often quite similar. On a very broad level, there are four categories that appear consistently: social functions, emotional functions, cognitive or self-related functions, and physiological or arousal-related functions (see also Hargreaves and North, 1999 ; Schäfer and Sedlmeier, 2009 , 2010 ).
Empirical studies using predefined collections of functions of music
Apart from the open-ended surveys and interview methods, a number of studies investigating musical functions begin with researcher-defined collections or even categories/dimensions. Some of these predefined collections or categories/dimensions were simply borrowed from the existing published research, whereas others were derived from specific theoretical perspectives.
Empirical studies on functions of music emerging from specific theoretical approaches. Some of the above mentioned theoretical approaches to the functionality of music have been investigated in empirical studies. Boehnke and Münch ( 2003 ) developed a model of the relationship of adolescents' development, music, and media use. They proposed seven functions of music that relate to the developmental issues of young people (such as peer group integration, physical maturation, or identity development). In two studies with a large number of participants, Lonsdale and North ( 2011 ) applied the model of media gratification (from McQuail et al., 1972 ) and used a collection of 30 functions of music they assembled from literature research and interviews. In both studies, they ran factor analyses—reducing the number of functions to six dimensions and eight dimensions, respectively. Lehmann ( 1994 ) developed a situations-functions-preference model and proposed that music preferences emerge from the successful use of music to serve specific functions for the listener, depending on the current situation. Lehmann identified 68 ways in which people use music, from which he was able to reduce them to 15 music reception strategies (Rezeptionsweisen) such as compensation/escapism, relaxation, and identification. Misenhelter and Kaiser ( 2008 ) adopted Merriam's ( 1964 ) anthropological approach and attempted to identify the functions of music in the context of music education. They surveyed teachers and students and found six basic functions that were quite similar to the ones proposed by Merriam ( 1964 ). Wells and Hakanen ( 1997 ) adopted Zillmann's ( 1988a , b ) mood management theory and identified four types of users regarding the emotional functions of music: mainstream, music lover, indifferent, and heavy rockers.
Empirical studies on functions of music emerging from literature research. A number of studies have made use of predefined musical functions borrowed from the existing research literature. The significance of these functions and/or their potential underlying structure has then been empirically investigated using different samples. As mentioned, not all of those studies tried to assemble an exhaustive collection of musical functions in order to produce a comprehensive picture of the functions of music; but many studies were focused on specific aspects such as the emotional, cognitive, or social functions of music.
Schäfer and Sedlmeier ( 2009 ) collected 17 functions of music from the literature and found functions related to the management of mood and arousal as well as self-related functions to be the ones that people highly ascribe to their favorite music. Tarrant et al. ( 2000 ) used a collection of 10 functions of music from the literature and factor analyzed them resulting in three distinct dimensions of music use: self-related, emotional, and social.
Sun and Lull ( 1986 ) collected 18 functions of music videos and were able to reduce them to four dimensions: social learning, passing time, escapism/mood, and social interaction. Melton and Galician ( 1987 ) identified 15 functions of radio music and music videos; and Greasley and Lamont ( 2011 ) collected 15 functions of music, as well. Ter Bogt et al. ( 2011 ) collected 19 functions of music from the literature and used confirmatory factor analysis to group them into five dimensions. In a clinical study with adolescents, Walker Kennedy ( 2010 ) found 47 functions of music that could be reduced to five dimensions.
By way of summary, extant empirical studies have used either an open approach—trying to capture the variety of musical functions in the course of surveys or questionnaire studies—or predefined collections of functions as they resulted from specific theoretical approaches or from literature research. These different approaches have led to quite heterogeneous collections of possible musical functions—from only few functions posited by a specific hypothesis, to long lists arising from open surveys. Moreover, although the many attempts to distill the functions of music to fewer dimensions have produced some points of agreement, the overall picture remains unclear.
The structure among the functions of music
With each successive study of musical functions, the aggregate list of potential uses has grown longer. Questionnaire studies, in particular, have led to the proliferation of possible ways in which music may be relevant in people's lives. Even if one sidesteps the question of possible evolutionary origins, the multitude of hundreds of proposed functions raises the question of whether these might not be distilled to a smaller set of basic dimensions.
As noted earlier, previous research appears to converge on four dimensions: social functions (such as the expression of one's identity or personality), emotional functions (such as the induction of positive feelings), cogni tive or self-related functions (such as escapism), and arousal-related functions (such as calming down or passing time). These four dimensions might well account for the basic ways in which people use music in their daily lives.
Notice that cluster analysis and PCA/factor analysis presume that the research begins with a range of variables that ultimately capture all of the factors or dimensions pertaining to the phenomenon under consideration. The omission of even a single variable can theoretically lead to incomplete results if that variable proves to share little variance in common with the other variables. For example, in studying the factors that contribute to a person's height, the failure to include a variable related to developmental nutrition will led to deceptive results; one might wrongly conclude that only genetic factors are important. The validity of these analyses depends, in part, on including a sufficient range of variables so that all of the pertinent factors or dimensions are likely to emerge.
Accordingly, we propose to address the question of musical functions anew, starting with the most comprehensive list yet of potential music-related functions. In addition, we will aim to recruit a sample of participants covering all age groups, a wide range of socio-economic backgrounds, and pursue our analysis without biasing the materials to any specific theory.
Fundamental functions of music—a comprehensive empirical study
The large number of functions of music that research has identified during the last decades has raised the question of a potential underlying structure: Are there functions that are more fundamental and are there others that can be subsumed under the fundamental ones? And if so, how many fundamental functions are there? As we have outlined above, many scientists have been in search of basic distinct dimensions among the functions of music. They have used statistical methods that help uncover such dimensions among a large number of variables: factor analyses or cluster analyses.
However, as we have also seen, the approaches and methods have been as different as the various functions suggested. For instance, some scholars have focused exclusively on the social functions of music while others have been interested in only the emotional ones; some used only adolescent participants while others consulted only older people. Thus, these researchers arrived at different categorizations according to their particular approach. To date, there is still no conclusive categorization of the functions of music into distinct dimensions, which makes psychological studies that rely on the use of music and its effects on cognition, emotion, and behavior still difficult (see also Stefanija, 2007 ). Although there exist some theoretically driven claims about what fundamental dimensions there might be (Tarrant et al., 2000 ; Laiho, 2004 ; Schubert, 2009 ; Lonsdale and North, 2011 ), there has been no large-scale empirical study that analyzed the number and nature of distinct dimensions using the broad range of all potential musical functions—known so far—all at once.
We sought to remedy this deficiency by assembling an exhaustive list of the functions of music that have been identified in past research and putting them together in one questionnaire study. Based on the research reviewed in the first part of this study, we identified more than 500 items concerned with musical use or function. Specifically, we assembled an aggregate list of all the questions and statements encountered in the reviewed research that were either theoretically derived or used in empirical studies. Of course, many of the items are similar, analogous, or true duplicates. After eliminating or combining redundant items, we settled on a list of 129 distinct items. All of the items were phrased as statements in the form “I listen to music because … ” The complete list of items is given in Table TableA3, A3 , together with their German versions as used in our study.
Participants were asked to rate how strongly they agreed with each item-statement on a scale from 0 ( not at all ) to 6 ( fully agree ). When responding to items, participants were instructed to think of any style of music and of any situation in which they would listen to music. In order to obtain a sample that was heterogeneous with regard to age and socioeconomic background, we distributed flyers promoting the Internet link to our study in a local electronics superstore. Recruitment of participants was further pursued via some mailing lists of German universities, students from comprehensive schools, and members of a local choir. As an incentive, respondents got the chance to win a tablet computer. A total of 834 people completed the survey. Respondents ranged from 8 to 85 years of age ( M = 26, SD = 10.4, 57% female).
Notice that in carrying out such a survey, we are assuming that participants have relatively accurate introspective access to their own motivations in pursuing particular musical behaviors, and that they are able to accurately recall the appropriate experiences. Of course, there exists considerable empirical research casting doubt on the accuracy of motivational introspection in self-report tasks (e.g., Wilson, 2002 ; Hirstein, 2005 ; Fine, 2006 ). These caveats notwithstanding, in light of the limited options for gathering pertinent empirical data, we nevertheless chose to pursue a survey-based approach.
Principal component analysis revealed three distinct dimensions behind the 129 items (accounting for about 40% of the variance), based on the scree plot. This solution was consistent over age groups and genders. The first dimension (eigenvalue: 15.2%) includes statements about self-related thoughts (e.g., music helps me think about myself), emotions and sentiments (e.g., music conveys feelings), absorption (e.g., music distracts my mind from the outside world), escapism (e.g., music makes me forget about reality), coping (e.g., music makes me believe I'm better able to cope with my worries), solace (e.g., music gives comfort to me when I'm sad), and meaning (e.g., music adds meaning to my life). It appears that this dimension expresses a very private relationship with music listening. Music helps people think about who they are, who they would like to be, and how to cut their own path. We suggest labeling this dimension self-awareness . The second dimension (eigenvalue: 13.7%) includes statements about social bonding and affiliation (e.g., music helps me show that I belong to a given social group; music makes me feel connected to my friends; music tells me how other people think). People can use music to feel close to their friends, to express their identity and values to others, and to gather information about their social environment. We suggest labeling this dimension social relatedness . The third dimension (eigenvalue: 10.2%) includes statements about the use of music as background entertainment and diversion (e.g., music is a great pastime; music can take my mind off things) and as a means to get into a positive mood and regulate one's physiological arousal (e.g., music can make me cheerful; music helps me relax; music makes me more alert). We suggest labeling this dimension arousal and mood regulation . All factor loadings are reported in Table TableA3 A3 .
In order to analyze the relative significance of the three derived dimensions for the listeners, we averaged the ratings for all items contained in each dimension (see Figure Figure1). 1 ). Arousal and mood regulation proved to be the most important dimension of music listening closely followed by self-awareness. These two dimensions appear to represent the two most potent reasons offered by people to explain why they listen to music, whereas social relatedness seems to be a relatively less important reason (ranging below the scale mean). This pattern was consistent across genders, socioeconomic backgrounds, and age groups. All differences between the three dimensions are significant (all p s < 0.001). The reliability indices (Cronbach's α) for the three dimensions are α = 0.97 for the first, α = 0.96 for the second, and α = 0.92 for the third dimension.

The three distinct dimensions emerging from 129 reasons for listening to music . Error bars are 95% confidence intervals. Self-awareness: M = 3.59 ( SE = 0.037); social relatedness: M = 2.01 ( SE = 0.035); arousal and mood regulation: M = 3.78 ( SE = 0.032).
General discussion
Since the earliest writing on the psychology of music, researchers have been concerned with the many ways in which people use music in their lives. In the first part of this paper, we reviewed literature spanning psychological, musicological, biological, and anthropological perspectives on musical function. The picture that emerged from our review was somewhat confusing. Surveying the literature from the past 50 years, we identified more than 500 purported functions for music. From this list, we assembled a somewhat catholic list of 129 non-redundant musical functions. We then tested the verisimilitude of these posited functions by collecting survey responses from a comparatively large sample. PCA revealed just three distinct dimensions: People listen to music to achieve self-awareness , social relatedness , and arousal and mood regulation . We propose calling these the Big Three of music listening.
In part one of our study we noted that several empirical studies suggest grouping musical functions according to four dimensions: cognitive, emotional, social/cultural, and physiological/arousal-related functions. This raises the question of how our three-dimensional result might be reconciled with the earlier work. We propose that there is a rather straightforward interpretation that allows the four-dimensional perspective to be understood within our three-dimensional result. Cognitive functions are captured by the first dimension (self-awareness); social/cultural functions are captured by the second dimensions (social relatedness); physiological/arousal-related functions are captured by the third dimension (arousal and mood regulation); and emotional functions are captured by the first and third dimensions (self-awareness + arousal and mood regulation). Notably—as can be seen with the items in Table TableA3—there A3 —there is a dissociation of emotion-related and mood-related functions. Emotions clearly appear in the first dimension (e.g., music conveys feelings; music can lighten my mood; music helps me better understand my thoughts and emotions), indicating that they might play an important role in achieving self-awareness, probably in terms of identity formation and self-perception, respectively. However, the regulation of moods clearly appears in the third dimension (e.g., music makes me cheerful; music can enhance my mood; I'm less bored when I listen to music), suggesting that moods are not central issues pertaining to identity. Along with the maintenance of a pleasant level of physiological arousal, the maintenance of pleasant moods is an effect of music that might rather be utilized as a “background” strategy, that is, not requiring a deep or aware involvement in the music. The regulation of emotions, on the other side, could be a much more conscious strategy requiring deliberate attention and devotion to the music. Music psychology so far has not made a clear distinction between music-related moods and emotions; and the several conceptions of music-related affect remain contentious (see Hunter and Schellenberg, 2010 ). Our results appear to call for a clearer distinction between moods and emotions in music psychology research.
As noted earlier, a presumed evolutionary origin for music need not be reflected in modern responses to music. Nevertheless, it is plausible that continuities exist between modern responses and possible archaic functions. Hence, the functions apparent in our study may echo possible evolutionary functions. The three functional dimensions found in our study are compatible with nearly all of the ideas about the potential evolutionary origin of music mentioned in the introduction. The idea that music had evolved as a means for establishing and regulating social cohesion and communication is consistent with the second dimension. The idea of music satisfying the basic human concerns of anxiety avoidance and quest for meaning is consistent with the first dimension. And the notion that the basic function of music could have been to produce dissociation and pleasure in the listener is consistent with the third dimension.
In light of claims that music evolved primarily as a means for promoting social cohesion and communication—a position favored by many scholars—the results appear noteworthy. Seemingly, people today hardly listen to music for social reasons, but instead use it principally to relieve boredom, maintain a pleasant mood, and create a comfortable private space. Such a private mode of music listening might simply reflect a Western emphasis on individuality: self-acknowledgement and well-being appear to be more highly valued than social relationships and relatedness (see also Roberts and Foehr, 2008 ; Heye and Lamont, 2010 ).
The results of the present study may be of interest to psychologists who make use of music as a tool or stimulus in their research. The way people usually listen to music outside the laboratory will surely influence how they respond to musical stimuli in psychological experiments. For those researchers who make use of music in psychological studies, some attention should be paid to how music is used in everyday life. The three dimensions uncovered in this study can provide a parsimonious means to identify the value a person sets on each of three different types of music use. It is also conceivable that individual patterns of music use are related to personality traits, a conjecture which may warrant future research.
With regard to music cognition, the present results are especially relevant to studies about aesthetic preferences, style or genre preferences, and musical choice. Recent research suggests that musical functions play an important role in the formation and development of music preferences (e.g., Schäfer and Sedlmeier, 2009 ; Rentfrow et al., 2011 ). It will be one of the future tasks of music cognition research to investigate the dependence of music preference and music choice on the functional use of music in people's lives.
By way of summary, in a self-report study, we found that people appear to listen to music for three major reasons, two of which are substantially more important than the third: music offers a valued companion, helps provide a comfortable level of activation and a positive mood, whereas its social importance may have been overvalued.
Conflict of interest statement
The authors declare that the research was conducted in the absence of any commercial or financial relationships that could be construed as a potential conflict of interest.
Overview of theoretical contributions that have derived, proposed, or addressed more than one function or functional aspect of music listening .

Overview about empirical studies that have identified and/or investigated more than one function or functional aspect of music listening .

In some places, we could only provide exemplary functions because either the total number of functions was too large to be displayed here or not all functions were given in the original publications .
The 129 statements referring to the functions of music exhaustively derived from past research, together with their means, standard deviations, and factor loadings (varimax rotated) .
Because it helps me think about myself. | |||||
[weil ich dann gut über mich nachdenken kann.] | 3.53 | 1.82 | 0.715 | ||
Because it can lead my thoughts to somewhere else. | |||||
[weil ich dann in Gedanken ganz weit weg sein kann.] | 4.21 | 1.70 | 0.671 | ||
Because it makes me believe I am better able to cope with my worries. | |||||
[weil ich dann das Gefühl habe, mit meinen Sorgen besser fertig zu werden.] | 3.37 | 1.87 | 0.668 | ||
Because it helps me better understand my thoughts and emotions. | |||||
[weil sie hilft, meine Gedanken und Gefühle besser zu verstehen.] | 3.02 | 1.85 | 0.667 | ||
Because it helps me think about my identity. | |||||
[weil sie mir hilft, über meine Identität nachzudenken.] | 2.87 | 1.94 | 0.665 | ||
Because it is therapy for my soul. | |||||
[weil sie eine Therapie für meine Seele ist.] | 3.82 | 1.84 | 0.656 | ||
Because it gives comfort to me when I'm sad. | |||||
[weil sie mir Trost spendet, wenn ich traurig bin.] | 4.10 | 1.73 | 0.645 | ||
Because it makes me feel secure. | |||||
[weil ich mich dann geborgen fühle.] | 3.00 | 1.79 | 0.637 | ||
Because it is a means to express myself. | |||||
[weil sie mir eine Möglichkeit bietet, mich selbst auszudrücken] | 3.39 | 1.89 | 0.632 | ||
Because it helps me find my own way. | |||||
[weil sie mir hilft, meinen Weg zu finden.] | 3.07 | 1.85 | 0.625 | ||
Because it mirrors my feelings and moods. | |||||
[weil ich darin meine Gefühle und Stimmungen wiederfinde.] | 4.92 | 1.31 | 0.610 | ||
Because it conveys feelings. | |||||
[weil sie Gefühle transportiert.] | 4.80 | 1.38 | 0.608 | ||
Because it expresses something that cannot be expressed in words. | |||||
[weil sie etwas vermittelt, was sich in Worten nicht ausdrücken lässt.] | 3.82 | 1.91 | 0.602 | ||
Because it helps me learn about myself. | |||||
[weil ich dadurch etwas über mich lernen kann.] | 2.30 | 1.82 | 0.589 | ||
Because it helps me be contemplative. | |||||
[weil sie mir beim Nachdenken hilft.] | 3.65 | 1.82 | 0.572 | ||
Because it helps me escape from my daily routines. | |||||
[weil ich dann aus dem grauen Alltag fliehen kann.] | 3.62 | 1.85 | 0.567 | ||
Because it often induces visual imagery. | |||||
[weil ich dabei oft bildhafte Vorstellungen habe.] | 3.88 | 1.72 | 0.564 | ||
Because it can make me dream. | |||||
[weil ich dabei träumen kann.] | 4.49 | 1.50 | 0.562 | ||
Because it distracts my mind from the outside world. | |||||
[weil sie mich von der “Außenwelt” ablenkt.] | 3.74 | 1.78 | 0.552 | ||
Because it lets me forget the world around me. | |||||
[weil ich dann die Welt um mich herum vergessen kann.] | 4.48 | 1.57 | 0.551 | ||
Because it makes me forget about reality. | |||||
[weil sie mich die Realität vergessen lässt.] | 3.30 | 1.91 | 0.544 | ||
Because it puts fantastic images or stories in my head. | |||||
[weil mir dann tolle Bilder oder Geschichten in den Kopf kommen.] | 3.88 | 1.76 | 0.543 | ||
Because it alleviates my inner tension. | |||||
[weil das die Anspannung in mir verringert.] | 3.85 | 1.55 | 0.542 | ||
Because it helps me reminisce. | |||||
[weil ich dabei in Erinnerungen schwelgen kann.] | 4.24 | 1.64 | 0.532 | ||
Because it gives me the energy I need for the day. | |||||
[weil sie mir Energie für den Tag gibt.] | 4.44 | 1.47 | 0.531 | ||
Because I can recognize myself in the lyrics. | |||||
[weil ich mich in den Texten wiederfinden kann.] | 3.72 | 1.70 | 0.524 | ||
Because it makes me feel somebody else feels the same as I do. | |||||
[weil sie mir das Gefühl gibt, dass jemand anderes dasselbe fühlt wie ich.] | 3.13 | 1.94 | 0.521 | ||
Because it supports my ideas. | |||||
[weil sie meine Ideen unterstützt.] | 2.86 | 1.82 | 0.512 | ||
Because it lets me be the way I am. | |||||
[weil ich dadurch so sein kann, wie ich bin.] | 3.66 | 1.89 | 0.508 | ||
Because it enables me to experiment with different facets of my personality. | |||||
[weil sie mir ermöglicht, mit verschiedenen Seiten meiner Persönlichkeit zu experimentieren.] | 2.52 | 1.94 | 0.508 | ||
Because it calms me. | |||||
[weil sie mich beruhigt.] | 4.32 | 1.37 | 0.501 | ||
Because it adds meaning to my life. | |||||
[weil sie mir Sinn im Leben gibt.] | 2.24 | 2.03 | 0.496 | ||
Because it can reduce my anxiety. | |||||
[weil sie meine Angst reduzieren kann.] | 2.51 | 1.93 | 0.493 | ||
Because it makes me feel that I want to change the world. | |||||
[weil ich dann das Gefühl bekomme, dass ich die Welt verändern möchte.] | 2.34 | 1.96 | 0.489 | ||
Because it is a means of venting my frustration. | |||||
[weil sie eine Möglichkeit bietet, meine Frustration abzuladen.] | 3.82 | 1.82 | 0.488 | ||
Because it can reduce my stress. | |||||
[weil sie meinen Stress reduziert.] | 4.42 | 1.39 | 0.487 | ||
Because it can make me feel less lonely. | |||||
[weil ich mich dann weniger einsam fühle.] | 2.93 | 1.92 | 0.486 | ||
Because I like the bodily changes it evokes (changes of heartbeat, prickling, etc.) | |||||
[weil ich die körperlichen Wirkungen mag (Veränderung des Herzschlags, Kribbeln auf der Haut usw.), die sie auslöst.] | 3.33 | 2.02 | 0.483 | ||
Because it gives me a way to let off steam. | |||||
[weil ich dadurch Dampf ablassen kann.] | 3.82 | 1.83 | 0.479 | ||
Because it can lighten my mood. | |||||
[weil sie meine Gefühle positiv beeinflussen kann.] | 4.86 | 1.22 | 0.473 | ||
Because it gives me pleasure. | |||||
[weil sie Wohlgefallen auslöst.] | 4.54 | 1.49 | 0.473 | ||
Because it reminds me of certain periods of my life or past experiences. | |||||
[weil sie mich an bestimmte Phasen meines Lebens bzw. an vergangene Ereignisse erinnert.] | 4.62 | 1.51 | 0.471 | ||
Because I just enjoy listening to music. | |||||
[weil ich es einfach genieße, Musik zu hören.] | 5.33 | 1.06 | 0.460 | ||
Because it gives me intellectual stimulation. | |||||
[weil es eine intellektuelle Stimulation für mich ist.] | 2.94 | 1.90 | 0.434 | ||
Because it gives me something that is mine alone. | |||||
[weil ich dann etwas für mich alleine habe.] | 2.58 | 2.00 | 0.418 | ||
Because it gives me goose bumps. | |||||
[weil ich dann Gänsehaut bekomme.] | 3.16 | 1.91 | 0.416 | ||
Because it addresses my sense of aesthetics. | |||||
[weil sie meinen Sinn für Ästhetik anspricht.] | 2.94 | 2.04 | 0.386 | ||
Because it reminds me of a particular person. | |||||
[weil sie mich an eine bestimmte Person erinnert.] | 3.39 | 1.88 | 0.379 | ||
Because it makes me feel my body. | |||||
[weil ich dabei meinen Körper spüre.] | 2.43 | 1.89 | 0.376 | ||
Because I can enjoy it as art. | |||||
[weil ich sie als Kunst genießen kann.] | 3.63 | 1.93 | 0.358 | ||
Because I want to play or sing it myself. | |||||
[weil ich sie nachspielen oder nachsingen möchte.] | 3.13 | 1.98 | 0.316 | ||
Because it helps me show that I belong to a given social group. | 1.30 | 1.62 | 0.726 | ||
[weil ich damit zeigen kann, dass ich einer bestimmten sozialen Gruppe angehöre.] | |||||
Because it makes me feel connected to all people who like the same kind of music. | |||||
[weil ich mich dann allen Leuten zugehörig fühle, die solche Musik hören.] | 1.68 | 1.71 | 0.686 | ||
Because it makes me feel connected to my friends. | |||||
[weil sie dazu führt, dass ich mich mit meinen Freunden verbunden fühle.] | 2.02 | 1.73 | 0.671 | ||
Because it provides me useful information for my everyday life. | |||||
[weil ich dadurch nützliche Informationen für das alltägliche Leben sammeln kann.] | 1.67 | 1.65 | 0.665 | ||
Because it is a reason to meet my friends. | |||||
[weil sie einen Grund dafür bietet, mit meinen Freunden zusammen zu sein.] | 1.86 | 1.73 | 0.662 | ||
Because it makes me feel connected to others. | |||||
[weil ich mich durch sie mit anderen verbunden fühle.] | 2.28 | 1.75 | 0.661 | ||
Because it can help me meet other people. | |||||
[weil ich dadurch neue Leute kennenlernen kann.] | 2.15 | 1.78 | 0.661 | ||
Because it helps me form friendships with people who have similar musical taste. | |||||
[weil sie mir hilft, Freundschaften mit Personen zu schließen, die einen ähnlichen Musikgeschmack haben wie ich.] | 2.17 | 1.82 | 0.660 | ||
Because it tells me how other people think. | |||||
[weil ich dann weiß, wie andere Leute denken.] | 1.89 | 1.72 | 0.636 | ||
Because I can learn something about other people. | |||||
[weil ich dadurch etwas über andere lernen kann.] | 2.49 | 1.76 | 0.629 | ||
Because music is a social experience. | |||||
[weil Musik eine Gruppenerfahrung ist.] | 2.00 | 1.77 | 0.628 | ||
Because it helps me develop social values. | |||||
[weil Musik hilft, soziale Werte zu entwickeln.] | 2.44 | 1.80 | 0.622 | ||
Because I would like to identify with a particular music scene. | |||||
[weil ich mich mit einer bestimmten Musikszene identifizieren möchte.] | 1.75 | 1.83 | 0.608 | ||
Because it helps me understand the world better. | |||||
[weil ich dadurch die Welt besser verstehen kann.] | 2.26 | 1.75 | 0.600 | ||
Because it mirrors the history and culture of my country. | |||||
[weil sie die Kultur und die Geschichte meines Landes widerspiegelt.] | 1.15 | 1.58 | 0.588 | ||
Because it can be a means to show political engagement. | |||||
[weil sie ein wichtiges Mittel für mich ist, um politisches Engagement zu zeigen.] | 1.00 | 1.50 | 0.582 | ||
Because it helps me develop my personal values. | |||||
[weil sie mir hilft, meine persönlichen Werte zu entwickeln.] | 2.34 | 1.79 | 0.581 | ||
Because it is a good way to express the uniqueness of our culture. | |||||
[weil das ein gutes Mittel ist, um die Einzigartigkeit unserer Kultur auszudrücken.] | 1.95 | 1.84 | 0.581 | ||
Because I would like to take the artists/musicians as role models. | |||||
[weil ich mir die Künstler/Musiker als Vorbild nehmen möchte.] | 1.83 | 1.83 | 0.575 | ||
Because it is something my friends like to do, as well. | |||||
[weil das etwas ist, was meine Freundinnen und Freunde auch gerne tun.] | 1.71 | 1.69 | 0.575 | ||
Because it makes me feel connected to the world. | |||||
[weil ich mich dann mit der Welt verbunden fühle.] | 2.03 | 1.76 | 0.571 | ||
Because it is something I can talk about with my friends. | |||||
[weil ich dann etwas habe, worüber ich mich mit meinen Freundinnen und Freunden unterhalten kann.] | 2.04 | 1.65 | 0.567 | ||
Because I can be together with my family. | |||||
[weil ich dabei mit meiner Familie zusammen sein kann.] | 1.38 | 1.53 | 0.565 | ||
Because it makes me belong. | |||||
[weil ich somit “dazu gehöre.”] | .88 | 1.32 | 0.560 | ||
Because my best friend and I can enthuse about it together. | |||||
[weil meine beste Freundin/mein bester Freund und ich dann gemeinsam für etwas schwärmen können.] | 1.62 | 1.69 | 0.543 | ||
Because it can express my political attitudes. | |||||
[weil sie meine politischen Überzeugungen ausdrücken kann.] | 1.48 | 1.77 | 0.531 | ||
Because my friends like the same music as I do. | |||||
[weil sie auch meinen Freundinnen und Freunden gefällt.] | 1.76 | 1.65 | 0.523 | ||
Because when listening, I can imagine how the music would sound in a concert. | |||||
[weil ich mir dabei vorstellen kann, wie die Musik wohl im Konzert wäre.] | 2.54 | 1.97 | 0.496 | ||
Because it is related to spirituality. | |||||
[weil sie für mich eng mit Spiritualität verbunden ist.] | 1.17 | 1.71 | 0.483 | ||
Because I learn a lot about the world. | |||||
[weil ich dadurch viel von der Welt erfahre.] | 2.30 | 1.64 | 0.472 | ||
Because I can identify with the musicians or bands. | |||||
[weil ich mich dadurch mit einigen MusikerInnen oder Gruppen so gut identifizieren kann.] | 2.58 | 1.79 | 0.465 | ||
Because it supports my religious faith. | |||||
[weil sie meinen Glauben unterstützt.] | 1.22 | 1.83 | 0.448 | ||
Because it has a supernatural meaning to me. | |||||
[weil sie für mich eine übersinnliche Bedeutung hat.] | 1.18 | 1.75 | 0.446 | ||
Because I want to know what's going on in the music scene. | |||||
[weil ich darüber Bescheid wissen will, was in der Musikszene gerade aktuell ist.] | 1.97 | 1.82 | 0.429 | ||
Because I want to find out something about the music. | |||||
[weil ich etwas über die Musik herausfinden möchte.] | 2.60 | 1.81 | 0.428 | ||
Because it makes me let go of myself when I'm in company. | |||||
[weil sie mir hilft, aus mir herauszugehen, wenn ich in Gesellschaft bin.] | 2.81 | 1.84 | 0.422 | ||
Because it contributes to my health. | |||||
[weil sie zu meiner Gesundheit beiträgt.] | 2.51 | 1.88 | 0.415 | ||
Because it can soothe my physical pain. | |||||
[weil sie meine körperlichen Beschwerden lindern kann.] | 1.95 | 1.82 | 0.412 | ||
Because you can learn something from the music. | |||||
[weil man etwas dabei lernen kann.] | 2.75 | 1.76 | 0.404 | ||
Because I want to be informed about hits and trends. | |||||
[weil ich mich über Hits und Trends informieren will.] | 1.86 | 1.71 | 0.402 | ||
Because it structures my everyday life. | |||||
[weil sie meinem Alltag Struktur gibt.] | 2.24 | 1.85 | 0.397 | ||
Because I can get away from my family. | |||||
[weil ich damit meiner Familie entkommen kann.] | 1.31 | 1.73 | 0.378 | ||
Because it is a means to share my memories with my friends. | |||||
[weil sie eine Möglichkeit bietet, Erinnerungen mit Freunden zu teilen.] | 3.40 | 1.81 | 0.375 | ||
Because it makes me feel sexy. | |||||
[weil ich mich dann sexy fühle.] | 1.68 | 1.87 | 0.372 | ||
Because I can learn about new pieces. | |||||
[weil ich dabei neue Stücke kennenlernen kann.] | 3.50 | 1.86 | 0.369 | ||
Because I'm interested in the musicians and bands. | |||||
[weil ich die MusikerInnen und Gruppen interessant finde.] | 3.86 | 1.66 | 0.312 | ||
Because it is a great pastime. | 3.97 | 1.68 | 0.640 | ||
[weil sie ein prima Zeitvertreib ist.] | |||||
Because it can take my mind off things. | |||||
[weil sie mich ablenken kann.] | 4.52 | 1.45 | 0.627 | ||
Because it prevents me from being bored while I do other things. | |||||
[weil ich dann weniger gelangweilt bin, während ich etwas anderes tue.] | 3.39 | 1.94 | 0.621 | ||
Because it makes time pass markedly faster. | |||||
[weil dann die Zeit deutlich schneller vergeht.] | 3.57 | 1.85 | 0.609 | ||
Because it enables me to kill time. | |||||
[weil ich damit die Zeit totschlagen kann.] | 2.64 | 1.99 | 0.598 | ||
Because I'm less bored then. | |||||
[weil es dann nicht so langweilig ist.] | 3.99 | 1.75 | 0.584 | ||
Because I need it in the background while I do other things. | |||||
[weil ich sie im Hintergrund brauche, während ich etwas anderes tue.] | 3.76 | 1.79 | 0.564 | ||
Because it makes me cheerful. | |||||
[weil ich dann gute Laune bekomme.] | 4.76 | 1.28 | 0.555 | ||
Because it can enhance my mood. | |||||
[weil sie meine Stimmung verbessern kann.] | 5.04 | 1.15 | 0.539 | ||
Because it fills the unpleasant silence when no one speaks. | |||||
[weil Musik die unangenehme Stille füllt, wenn gerade niemand spricht.] | 3.15 | 2.00 | 0.537 | ||
Because it helps me get up in the morning. | |||||
[weil sie mir morgens hilft, wach zu werden.] | 3.75 | 1.94 | 0.532 | ||
Because it helps me relax. | |||||
[weil ich mich dann besser entspannen kann.] | 4.84 | 1.18 | 0.520 | ||
Because it provides diversion. | |||||
[weil sie für mich eine gute Abwechslung bietet.] | 4.24 | 1.44 | 0.511 | ||
Because it puts me in the right mood for going out. | |||||
[weil ich mich damit einstimmen kann, bevor ich ausgehe.] | 3.69 | 2.05 | 0.508 | ||
Because it enhances my drive or my motivation for certain actions. | |||||
[weil sie meinen Antrieb bzw. meine Motivation für bestimmte Tätigkeiten steigert.] | 4.41 | 1.55 | 0.505 | ||
Because it is a good way to entertain myself. | |||||
[weil das eine gute Art ist, mich selbst zu unterhalten.] | 4.15 | 1.56 | 0.492 | ||
Because I take delight in doing so. | |||||
[weil ich dabei Spaß habe.] | 5.10 | 1.15 | 0.491 | ||
Because it makes me more alert. | |||||
[weil ich dann wacher bin.] | 3.32 | 1.72 | 0.477 | ||
Because it makes doing things seem effortless. | |||||
[weil mir dann vieles lockerer von der Hand geht.] | 4.40 | 1.40 | 0.464 | ||
Because it stimulates me. | |||||
[weil sie mich animiert.] | 3.96 | 1.63 | 0.441 | ||
Because I can dance to it. | |||||
[weil ich dazu tanzen kann.] | 3.53 | 2.04 | 0.436 | ||
Because it makes me feel fitter. | |||||
[weil ich mich dann fitter fühle.] | 3.37 | 1.81 | 0.432 | ||
Because it enables me to work off my aggression. | |||||
[weil ich dabei meine Aggressionen abreagieren kann.] | 3.48 | 2.02 | 0.427 | ||
Because it takes my mind off things. | |||||
[weil sie mich auf andere Gedanken bringt.] | 4.82 | 1.27 | 0.421 | ||
Because it provides a pleasant ambience for conversations. | |||||
[weil sie eine angenehme Atmosphäre beim Gespräch schafft.] | 3.17 | 1.72 | 0.416 | ||
Because music just fits into my life. | |||||
[weil Musik einfach gut in mein Leben passt.] | 4.90 | 1.37 | 0.403 | ||
Because it fits my sports. | |||||
[weil es zu meinem Sport passt.] | 2.62 | 2.16 | 0.400 | ||
Because working is easier with music. | |||||
[weil ich dann besser arbeiten kann.] | 3.43 | 1.83 | 0.389 | ||
Because it helps me fall asleep. | |||||
[weil sie mir beim Einschlafen hilft.] | 2.68 | 2.03 | 0.357 | ||
Because I can cuddle with my partner. | |||||
[weil ich mit meinem Partner bzw. meiner Partnerin dabei gut kuscheln kann.] | 2.50 | 1.85 | 0.354 | ||
Because I can sing or hum along. | |||||
[weil ich dabei mitsingen oder mitsummen kann.] | 3.91 | 1.80 | 0.346 | ||
Because I can try out new movements. | |||||
[weil ich dann neue Bewegungen ausprobieren kann.] | 1.83 | 1.83 | 0.337 |
Dimension 1, self-awareness; Dimension 2, social relatedness; Dimension 3, arousal and mood regulation .
- Arnett J. J. (1995). Adolescents' uses of media for self-socialisation . J. Youth Adolesc . 24 , 519–533 [ Google Scholar ]
- Baacke D. (1984). Kommunikations-kultur der Jugend , in Medienpädagogik and Kommunikationskultur. Referate und Texte Nach Dem Ersten “Forum Kommunikationskultur,” ed de Haen I. (Frankfurt am Main: GEP; ), 37–53 [ Google Scholar ]
- Bartlett D. L. (1996). Physiological responses to music and sound stimuli , in Handbook of Music Psychology, 2nd Edn , ed Hodges D. A. (St. Louis, MO: MMB Music; ), 343–385 [ Google Scholar ]
- Bicknell J. (2007). Explaining strong emotional responses to music: sociality and intimacy . J. Conscious. Stud . 14 , 5–23 [ Google Scholar ]
- Blood A. J., Zatorre R. J. (2001). Intensely pleasurable responses to music correlate with activity in brain regions implicated in reward and emotion . Proc. Natl. Acad. Sci. U.S.A 98 , 11818–11823 10.1073/pnas.191355898 [ PMC free article ] [ PubMed ] [ CrossRef ] [ Google Scholar ]
- Boehnke K., Münch T. (2003). Jugendsozialisation und Medien. Helfen Medien und Musik beim Erwachsenwerden? in Neue Medien im Alltag. Nutzung, Vernetzung, Interaktion , eds Keitel E., Boehnke K., Wenz K. (Lengerich: Pabst Science Publishers; ), 203–227 [ Google Scholar ]
- Boer D. (2009). Music Makes the People Come Together: Social Functions of Music Listening for Young People Across Cultures . Department of Psychology. Victoria University of Wellington, Wellington. Available online at: http://researcharchive.vuw.ac.nz/bitstream/handle/10063/1155/thesis.pdf?sequence=1
- Brown J. D., Campbell K., Fischer L. (1986). American adolescents and music videos: why do they watch . Int. Commun. Gaz . 37 , 19–32 10.1177/001654928603700104 [ CrossRef ] [ Google Scholar ]
- Brown S. (2006). How does music work? Toward a pragmatics of musical communication , in Music and Manipulation: On the Social Uses and Social Control of Music , eds Brown S., Volgsten U. (New York, NY: Berghahn Books; ), 1–30 [ Google Scholar ]
- Bryson B. (1996). “Anything but heavy metal”: symbolic exclusion and musical dislikes . Am. Soc. Rev . 61 , 884–899 10.2307/2096459 [ CrossRef ] [ Google Scholar ]
- Bullough E. (1921). Recent work in experimental aesthetics . Br. J. Psychol . 12 , 76–99 [ Google Scholar ]
- Campbell C., Connell S., Beegle A. P. (2007). Adolescents' expressed meanings of music in and out of school . J. Res. Music Educ . 55 , 220–236 [ Google Scholar ]
- Chamorro-Premuzic T., Furnham A. (2007). Personality and music: can traits explain how people use music in everyday life . Br. J. Psychol . 98 , 175–185 10.1348/000712606X111177 [ PubMed ] [ CrossRef ] [ Google Scholar ]
- Coleman J. S. (1961). Psychological effects of the social system , in The Adolescents Society: The Social Life of the Teenager and its Impact on Education , ed Coleman J. S. (Oxford: Free Press of Glencoe; ), 220–243 [ Google Scholar ]
- Cook J. D. (1986). Music as an intervention in the oncology setting . Cancer Nurs . 9 , 23–28 [ PubMed ] [ Google Scholar ]
- Darwin C. (1871). The Descent of Man, and Selection in Relation to Sex . London: John Murray [ Google Scholar ]
- Darwin C. (1872). The Expression of the Emotions in Man and Animals . London: John Murray [ Google Scholar ]
- DeNora T. (1999). Music as a technology of the self . Poetics 27 , 31–56 10.1016/S0304-422X(99)00017-0 [ CrossRef ] [ Google Scholar ]
- Dissanayake E. (2006). Ritual and ritualization: musical means of conveying and shaping emotion in humans and other animals , in Music and Manipulation: On the Social Uses and Social Control of Music , eds Brown S., Volgsten U. (New York, NY: Berghahn Books; ), 31–56 [ Google Scholar ]
- Dissanayake E. (2009). Root, leaf, blossom, or bole: concerning the origin and adaptive function of music , in Communicative Musicality: Exploring the Basis of Human Companionship , eds Malloch S., Trevarthen C. (New York, NY: Oxford University Press; ), 17–30 [ Google Scholar ]
- Engh M. (2006). Popstars als Marke: Identitätsorientiertes Marken-management für die Musikindustrielle Künstlerentwicklung und –Vermarktung . Wiesbaden: Deutscher Universitäts-Verlag [ Google Scholar ]
- Fachner J. (2008). Musik und veränderte Bewusstseinszustände [Music and altered states of consciousness] , in Musikpsychologie. Das neue Handbuch , eds Bruhn H., Kopiez R., Lehmann A. C. (Reinbek: Rowohlt; ), 594–612 [ Google Scholar ]
- Falk D. (2004a). Prelinguistic evolution in early hominins: whence motherese . Behav. Brain Sci . 27 , 491–503 10.1017/S0140525X04000111 [ PubMed ] [ CrossRef ] [ Google Scholar ]
- Falk D. (2004b). The “putting the baby down” hypothesis: bipedalism, babbling, and baby slings . Behav. Brain Sci . 27 , 526–534 10.1017/S0140525X0448011X [ CrossRef ] [ Google Scholar ]
- Fine C. (2006). A Mind of Its Own: How Your Brain Distorts and Deceives . New York, NY: W.W. Norton [ Google Scholar ]
- Fitch W. T. (2006). The biology and evolution of music: a comparative perspective . Cognition 100 , 173–215 10.1016/j.cognition.2005.11.009 [ PubMed ] [ CrossRef ] [ Google Scholar ]
- Frith S. (1996). Performing Rites. On the Value of Popular Music . Oxford: Oxford University Press [ Google Scholar ]
- Frohne-Hagemann I., Pleß-Adamczyk H. (2005). Indikation Musiktherapie bei psychischen Problemen im Kindes- und Jugendalter. Musiktherapeutische Diagnostik und Manual nach ICD-10 . Göttingen: Vandenhoeck and Ruprecht [ Google Scholar ]
- Gantz W., Gartenberg H. M., Pearson M. L., Schiller S. O. (1978). Gratifications and expectations associated with Pop music among adolescents . Pop. Music Soc . 6 , 81–89 10.1080/03007767808591113 [ CrossRef ] [ Google Scholar ]
- Greasley A. E., Lamont A. (2011). Exploring engagement with music in everyday life using experience sampling methodology . Music. Sci . 15 , 45–71 10.1177/1029864910393417 [ CrossRef ] [ Google Scholar ]
- Gregory A. H. (1997). The roles of music in society: the ethnomusicological perspective , in The Social Psychology of Music , eds Hargreaves D. J., North A. C. (New York, NY: Oxford University Press; ), 123–140 [ Google Scholar ]
- Hargreaves D. J., North A. C. (1999). The functions of music in everyday life: redefining the social in music psychology . Psychol. Music 27 , 71–83 10.1177/0305735699271007 [ CrossRef ] [ Google Scholar ]
- Hays T., Minichiello V. (2005). The meaning of music in the lives of older people: a qualitative study . Psychol. Music 33 , 437–451 10.1177/0305735605056160 [ CrossRef ] [ Google Scholar ]
- Heister H.-W. (1993). Stellenwert der Musik im gesellschaftlichen System , in Musikpsychologie. Ein Handbuch , eds Bruhn H, Oerter R., Rösing H. (Reinbek: Rowohlt; ), 103–112 [ Google Scholar ]
- Herbert R. (2011). Everyday Music Listening: Absorption, Dissociation and Trancing . Farnham, Burlington: Ashgate Publishing Limited [ Google Scholar ]
- Heye A., Lamont A. (2010). Mobile listening situations in everyday life: the use of MP3 players while travelling . Music. Sci . 14 , 95–120 [ Google Scholar ]
- Hirstein W. (2005). Brain Fiction: Self-Deception and the Riddle of Confabulation . Cambridge, MA: MIT Press [ Google Scholar ]
- Hunter P. G., Schellenberg E. G. (2010). Music and emotion , in Music Perception , Vol. 36 , eds Jones M. R., Fay R. R., Popper A. N. (New York, NY: Springer; ), 129–164 [ Google Scholar ]
- Huron D. (2001). Is music an evolutionary adaptation? , in The Biological Foundations of Music , eds Zatorre R. J., Peretz I. (New York, NY: New York Academy of Sciences; ), 43–61 [ PubMed ] [ Google Scholar ]
- Juslin P. N., Liljeström S., Västfjäll D., Barradas G., Silva A. (2008). An experience sampling study of emotional reactions to music: listener, music, and situation . Emotion 8 , 668–683 10.1037/a0013505 [ PubMed ] [ CrossRef ] [ Google Scholar ]
- Kapteina H. (2010). Was Geschieht, Wenn Wir Musik Hören. Fragmente Zur Psychologie Des Hörens . Available online at: http://www.musiktherapie.uni-siegen.de/kapteina/material/forschungsgebiete/neu_was_geschieht_wenn_wir_musik_hoeren.pdf
- Laiho S. (2004). The psychological functions of music in adolescence . Nord. J. Music Ther . 13 , 47–63 10.1080/08098130409478097 [ CrossRef ] [ Google Scholar ]
- Larson R. (1995). Secrets in the bedroom: adolescents' private use of media . J. Youth Adolesc . 24 , 535–550 10.1007/BF01537055 [ CrossRef ] [ Google Scholar ]
- Laukka P. (2007). Uses of music and psychological well-being among the elderly . J. Happiness Stud . 8 , 215–241 10.1007/s10902-006-9024-3 [ CrossRef ] [ Google Scholar ]
- Lehmann A. C. (1994). Habituelle Und Situative Rezeptionsweisen Beim Musikhören. Eine Einstellungstheoretische Untersuchung . Frankfurt: Peter Lang [ Google Scholar ]
- Levitin D. J. (2007). Life Soundtrack: The Uses of Music in Everyday Life . Montreal, QC: McGill University; Available online at: http://levitin.mcgill.ca/pdf/LifeSoundtracks.pdf [ Google Scholar ]
- Lonsdale A. J., North A. C. (2011). Why do we listen to music. a uses and gratifications analysis . Br. J. Psychol . 102 , 108–134 10.1348/000712610X506831 [ PubMed ] [ CrossRef ] [ Google Scholar ]
- Maas G. (1993). Filmmusik , in Musikpsychologie. Ein Handbuch , eds Bruhn H., Oerter R., Rösing H. (Reinbek: Rowohlt; ), 203–207 [ Google Scholar ]
- Maslow A. H. (1968). Toward a Psychology of Being . 2nd Edn New York, NY: Van Nostr and Company [ Google Scholar ]
- McQuail D., Blumler J. G., Brown J. (1972). The television audience: a revised perspective , in Sociology of Mass Communication , ed McQuail D. (Middlesex: Penguin; ), 135–165 [ Google Scholar ]
- Melton G. W., Galician M. Lou. (1987). A sociological approach to the pop music phenomenon: radio and music video utilization for expectation, motivation and satisfaction . Pop. Music Soc . 11 , 35–46 10.1080/03007768708591286 [ CrossRef ] [ Google Scholar ]
- Merriam A. P. (1964). The Anthropology of Music . Evanston, IL: Northwestern University Press [ Google Scholar ]
- Miller G. (2000). Evolution of human music through sexual selection , in The Origins of Music , eds Wallin N. L., Merker B., Brown S. (Cambridge: The MIT Press; ), 329–360 [ Google Scholar ]
- Misenhelter D., Kaiser K. (2008). Social functions of music in music education . J. Artistic Creat. Educ . 2 , 61–74 [ Google Scholar ]
- Mithen S. (2006). The Singing Neanderthals: The Origins of Music, Language, Mind, and Body . Cambridge: Harvard University Press [ Google Scholar ]
- Münch T., Bommersheim U., Müller-Bachmann E. (2005). Jugendliches Musikverhalten. Musikinvolvement, Nutzungsmotive und Musikpräferenzen , in Jugendsozialisation und Medien , eds Boehnke K., Münch T. (Lengerich: Pabst Science Publishers; ), 167–199 [ Google Scholar ]
- Nakamura J., Csikszentmihalyi M. (2009). Flow theory and research , in Oxford Handbook of Positive Psychology, 2nd Edn , eds Lopez S. J., Snyder C. R. (New York, NY: Oxford University Press; ), 195–206 [ Google Scholar ]
- Newberg A., D'Aquili E., Rause V. (2001). Why God Won't Go Away: Brain Science and the Biology of Belief . New York, NY: Ballantine Books [ Google Scholar ]
- North A. C., Hargreaves D. J., O'Neill S. A. (2000). The importance of music to adolescents . Br. J. Educ. Psychol . 70 , 255–272 [ PubMed ] [ Google Scholar ]
- Packer J., Ballantyne J. (2011). The impact of music festival attendance on young people's psychological and social well-being . Psychol. Music 39 , 164–181 10.1177/0305735610372611 [ CrossRef ] [ Google Scholar ]
- Panksepp J. (1995). The emotional sources of “chills” induced by music . Music Percept . 13 , 171–207 [ Google Scholar ]
- Panksepp J., Bernatzky G. (2002). Emotional sounds and the brain: the neuro-affective foundations of musical appreciation . Behav. Process . 60 , 133–155 10.1016/S0376-635700080-3 [ PubMed ] [ CrossRef ] [ Google Scholar ]
- Peretz I. (2006). The nature of music from a biological perspective . Cognition 100 , 1–32 10.1016/j.cognition.2005.11.004 [ PubMed ] [ CrossRef ] [ Google Scholar ]
- Rentfrow P. J., Goldberg L. R., Levitin D. J. (2011). The structure of musical preferences: a five-factor model . J. Pers. Soc. Psychol . 100 , 1139–1157 [ PMC free article ] [ PubMed ] [ Google Scholar ]
- Roberts D. F., Christenson P. G. (2001). Popular music in childhood and adolescence , in Handbook of Children and the Media , eds Singer D. G., Singer J. L. (Thousand Oaks, CA: Sage Publications, Inc; ), 395–414 [ Google Scholar ]
- Roberts D. F., Foehr U. G. (2008). Trends in media use . Future Child . 18 , 11–37 [ PubMed ] [ Google Scholar ]
- Roe K. (1985). Swedish youth and music: listening patterns and motivations . Commun. Res . 12 , 353–362 10.1177/009365085012003007 [ CrossRef ] [ Google Scholar ]
- Rösing H. (1993). Musik im Alltag , in Musikpsychologie. Ein Handbuch , eds Bruhn H., Oerter R., Rösing H. (Reinbek: Rowohlt; ), 113–130 [ Google Scholar ]
- Ruud E. (1997). Music and the quality of life . Nord. J. Music Ther . 6 , 86–97 10.1080/08098139709477902 [ CrossRef ] [ Google Scholar ]
- Schäfer T., Sedlmeier P. (2009). From the functions of music to music preference . Psychol. Music 37 , 279–300 10.1177/0305735608097247 [ CrossRef ] [ Google Scholar ]
- Schäfer T., Sedlmeier P. (2010). What makes us like music. Determinants of music preference . Psychol. Aesthe. Creativity Arts 4 , 223–234 10.1037/a0018374 [ CrossRef ] [ Google Scholar ]
- Schubert E. (2009). The fundamental function of music . Music. Sci . 13 , 63–81 10.1177/1029864909013002051 [ CrossRef ] [ Google Scholar ]
- Steele J. R., Brown J. D. (1995). Adolescent room culture: studying the media in the context of everyday life . J. Youth Adolesc . 24 , 551–576 10.1007/BF01537056 [ CrossRef ] [ Google Scholar ]
- Stefanija L. (2007). Functions of music: a survey of research vocabularies . Muzikos funkcijos: tyrimø terminologijos apžvalga. (Lithuanian) 7 , 6–17 [ Google Scholar ]
- Sun S., Lull J. (1986). The adolescent audience for music videos and why they watch . J. Commun . 36 , 115–125 10.1111/j.1460-2466.1986.tb03043.x [ CrossRef ] [ Google Scholar ]
- Tarrant M., North A. C., Hargreaves D. J. (2000). English and American adolescents' reasons for listening to music . Psychol. Music 28 , 166–173 10.1177/0305735600282005 [ CrossRef ] [ Google Scholar ]
- Ter Bogt T. F. M., Mulder J., Raaijmakers Q. A. W., Gabhainn S. N. (2011). Moved by music: a typology of music listeners . Psychol.Music 39 , 147–163 10.1177/0305735610370223 [ CrossRef ] [ Google Scholar ]
- Troldahl V. C., Skolnik R. (1967). The meanings people have for radio today . J. Broadcast . 12 , 57–67 10.1080/08838156709386226 [ CrossRef ] [ Google Scholar ]
- Walker Kennedy S. (2010). An Exploration of Differences in Response to Music Related to Levels of Psychological Health in Adolescents . Toronto, ON: University of Toronto [ Google Scholar ]
- Wells A., Hakanen E. A. (1997). The emotional use of popular music by adolescents , in Mass Media and Society , eds Wells A., Hakanen E. A. (Greenwich: Ablex Publishing Corporation; ), 217–228 [ Google Scholar ]
- Wilson T. D. (2002). Strangers to Ourselves: Discovering the Adaptive Unconscious . Cambridge, MA: Harvard University Press [ Google Scholar ]
- Zatorre R. J., Peretz I. (2001). The Biological Foundations of Music . New York, NY: New York Academy of Sciences [ Google Scholar ]
- Zillmann D. (1988a). Mood management through communication choices . Am. Behav. Sci . 31 , 327–341 [ Google Scholar ]
- Zillmann D. (1988b). Mood management: using entertainment to full advantage , in Communication, Social Cognition, and Affect , eds Donohew L., Sypher H. E., Higgins E. T. (Hillsdale, NJ: Lawrence Erlbaum Associates; ), 147–171 [ Google Scholar ]
American Music--Music in the United States: A Guide to Research and Resources
- Introduction
- Search Strategies
Periodicals on American Music
Popular music, music periodical databases.
- Encyclopedias & Books
- Sound Recordings & Videos
- Key Surveys & Essay Collections
Listed here are some key journals and magazine that deal with solely or frequently with topics related to American music. Follow the title links to full descriptions, which include links to issues available online (fully reproduced in pdf or only available in html full-text).
- American Music Publishes articles on American composers, performers, publishers, institutions, events, the music industry, and the history and analysis of blues, jazz, folk music, and mixed and emerging musical styles. Also includes book and recording reviews, bibliographies, and discographies.
- Journal of the Society for American Music Deals with all aspects of American music and music in the Americas. The journal's broad range of topics includes cultural hierarchy; social, political, economic, gender, and sexuality issues; race and ethnicity; the impact and role of the media; sacred, secular, and popular contexts and traditions; geographical and historical patterns; composers, performers, and audiences; historiography and reception history; problems of research, analysis, criticism, and aesthetics.
- Black Music Research Journal (BMRJ) Official journal of the Center for Black Music Research. Includes articles about the philosophy, aesthetics, history, and criticism of black music.
- Rock's Backpages: The Online Library of Rock Writing This link opens in a new window Full-text, expanding archive of selective interviews, feature articles, reviews, and more on rock and a wide range of popular music (including blues and soul) from the music and mainstream press, updated weekly. The homepage includes links to highlights, audio and video interviews, blogs, and many other features beyond the ultimate archive of rock music writing and journalism. 1950s+
- Bloomsbury Music and Sound (formerly Bloomsbury Popular Music) This link opens in a new window Provides scholarly coverage of modern popular music worldwide, from the early 20th century to the present day, by cross-searching the 14-volume Bloomsbury Encyclopedia of Popular Music of the World; all 120+ volumes of the 33 1/3 book series (providing in-depth analysis of influential albums); an expanding range of scholarly books from Bloomsbury Popular Music Studies; and titles in the new 33 1/3 Global book series.
Use these resources primarily to find print and online articles, reports, notices, reviews, and other writings on the music industry published in music journals, trade publications, and magazines.
- RILM Abstracts of Music Literature with Full Text This link opens in a new window Covers all aspects of music, including historical musicology, ethnomusicology, instruments and voice, dance, and music therapy. If related to music, works in other fields, such as literature, dramatic arts, visual arts, anthropology, sociology, philosophy and physics are included. 1967+
- Music Periodicals Database This link opens in a new window Indexes music journals covering a broad scope of research from performance, theory and composition to music education, jazz and ethnomusicology. Provides selected full text coverage of the most important music journals, and both scholarly and popular publications.
- Music Index with Full Text This link opens in a new window Includes international music periodicals covering classical, popular and world of music. Comprehensively cites articles and news about music, musicians and the music industry as well as book and concert reviews and obituaries. 1970+ more... less... Print volumes covering 1949-1972 are available in Mendel Music Library: Reference (SV) ML 118 M84.
- << Previous: Search Strategies
- Next: Encyclopedias & Books >>
- Last Updated: Aug 5, 2024 10:37 AM
- URL: https://libguides.princeton.edu/american-music
Academia.edu no longer supports Internet Explorer.
To browse Academia.edu and the wider internet faster and more securely, please take a few seconds to upgrade your browser .
- We're Hiring!
- Help Center
Contemporary Music
- Most Cited Papers
- Most Downloaded Papers
- Newest Papers
- Last »
- Music Aesthetics Follow Following
- Musicology Follow Following
- Music Follow Following
- Composition (Music) Follow Following
- Music Theory Follow Following
- Electroacoustic Music Follow Following
- Experimental Music Follow Following
- Musical Composition Follow Following
- Computer Music Follow Following
- Music analysis Follow Following
Enter the email address you signed up with and we'll email you a reset link.
- Academia.edu Journals
- We're Hiring!
- Help Center
- Find new research papers in:
- Health Sciences
- Earth Sciences
- Cognitive Science
- Mathematics
- Computer Science
- Academia ©2024
- Tools and Resources
- Customer Services
- African Literatures
- Asian Literatures
- British and Irish Literatures
- Latin American and Caribbean Literatures
- North American Literatures
- Oceanic Literatures
- Slavic and Eastern European Literatures
- West Asian Literatures, including Middle East
- Western European Literatures
- Ancient Literatures (before 500)
- Middle Ages and Renaissance (500-1600)
- Enlightenment and Early Modern (1600-1800)
- 19th Century (1800-1900)
- 20th and 21st Century (1900-present)
- Children’s Literature
- Cultural Studies
- Film, TV, and Media
- Literary Theory
- Non-Fiction and Life Writing
- Print Culture and Digital Humanities
- Theater and Drama
- Share This Facebook LinkedIn Twitter
Article contents
Shakespeare and music.
- Joseph M. Ortiz Joseph M. Ortiz Department of English, The University of Texas at El Paso
- https://doi.org/10.1093/acrefore/9780190201098.013.1190
- Published online: 29 September 2021
William Shakespeare entertained many ideas about music, some of them conflicting, and he frequently represented these ideas in his plays. Music was a multifaceted art and science in early modern England, and debates over the nature and interpretation of music played out in a variety of contexts: academic, religious, political, commercial, and aesthetic. At the same time, music was a vital part of Shakespeare’s theatrical practice. He made use of his company’s musical resources to include performed music in his plays, and his characters frequently sing and quote popular ballads and songs that would have been recognized by his audiences. The combination of words about music and musical performances gave Shakespeare the opportunity to test various theories of music in complex and original ways. His plays are especially demonstrative of the ways in which certain views of music were connected to other ideological perspectives. Shakespeare’s most modern idea about music is the notion that musical meaning derives from its contexts and conventions rather than from an inherent, universal nature. Taken together, his plays provoke skepticism about unified theories of music. At the same time, they demonstrate that the seeming universality of music makes it an extremely powerful tool for both the polemicist and the dramatist.
- Shakespeare
- performance
- Renaissance drama
You do not currently have access to this article
Please login to access the full content.
Access to the full content requires a subscription
Printed from Oxford Research Encyclopedias, Literature. Under the terms of the licence agreement, an individual user may print out a single article for personal use (for details see Privacy Policy and Legal Notice).
date: 26 August 2024
- Cookie Policy
- Privacy Policy
- Legal Notice
- Accessibility
- [195.158.225.244]
- 195.158.225.244
Character limit 500 /500
Custom Essay, Term Paper & Research paper writing services
- testimonials
Toll Free: +1 (888) 354-4744
Email: [email protected]
Writing custom essays & research papers since 2008
Best 100 music research topics (just updated).
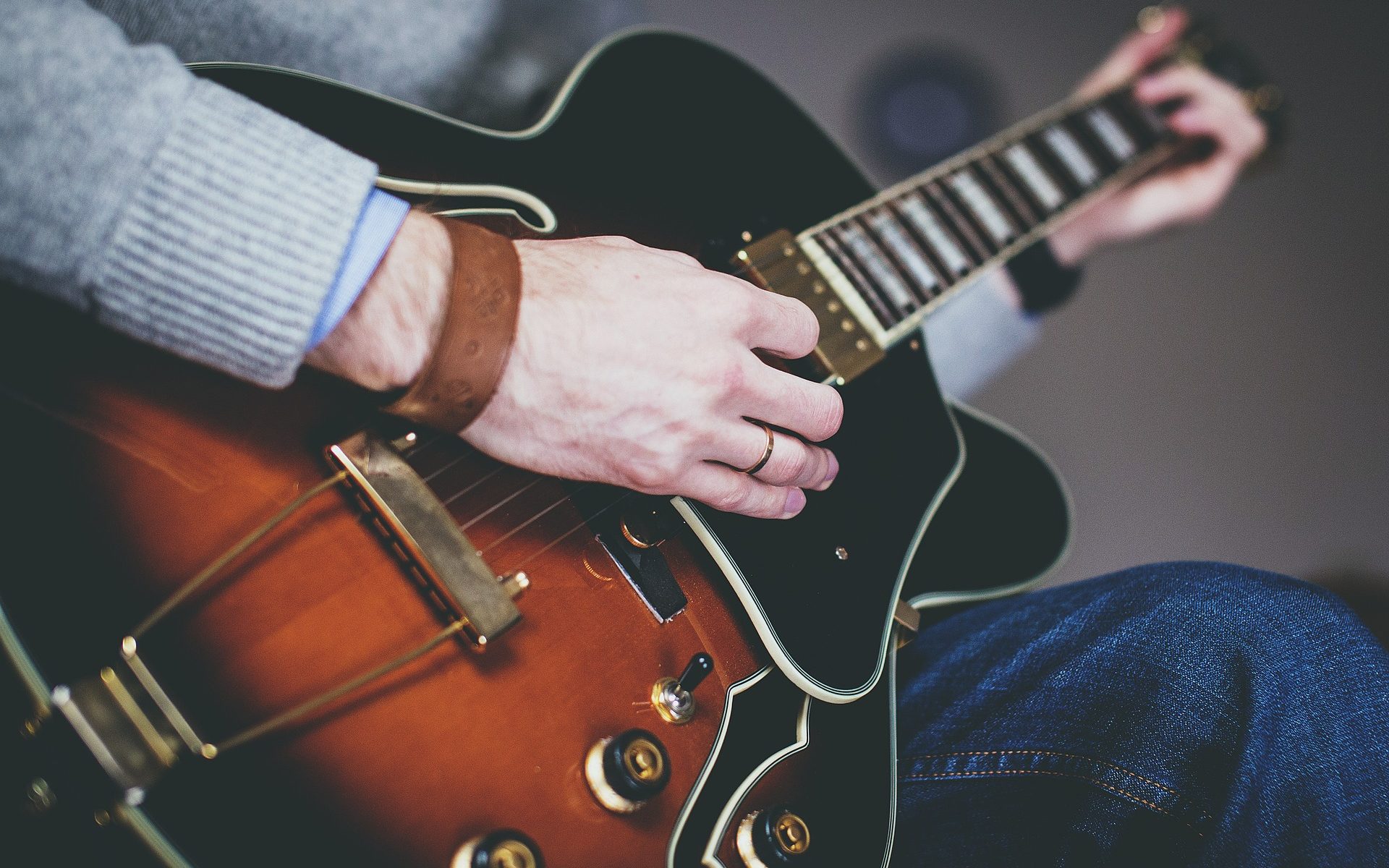
If you are reading this, you are probably looking for the best music research topics for your next essay. Truth be told, choosing the right topic is very important. It can make the difference between a B and an A, or even between an A and an A+. Unfortunately, choosing the best topics is not as simple as you think. Even though the internet is full of music research topics, most of them are plain and, quite frankly, boring.
Your professor wants more than this. Let’s see why you need the most interesting topics and where you can find them. Of course, you are free to use any of our 100 topics for free and even reword them as you see fit. Read on!
Choosing Good Music Research Topics
By now, you are probably wondering why everyone keeps telling you to come up with the best music topics. The truth is that there are many, many benefits to choosing an awesome topic. Here are just some of them, so you can get a better idea of the importance of a great idea:
- Excellent music research paper topics show your professor that you really did your best to get a top grade.
- A good topic is one that you know much about. It should be relatively easy to you to research it and to write about it.
- An awesome topic will pique the interest of your professor and will keep him or her reading. You will often get bonus points for this.
- Great topics make you stand out from your classmates. Your professor will notice you, and the grade will reflect this.
Where Can You Find Decent Music Topics?
Finding amazing music research topics is easier said than done. Yes, the Internet is full of websites that are offering ideas. There are even websites where you can buy bundles of topics. However, the music argumentative essay topics you will get from these websites are not of the highest quality. Most of them are actually quite boring. And remember, you classmates are probably searching for music history research paper topics on the same websites as you do. You want your research topics on music or book review topics to be original, so your professor can have a reason to award your paper some bonus points. The best place to get excellent music topics to write about is this page. The list of ideas is updated frequently, so you can get an original topic for free right now.
Music History Research Topics
Are you looking for the most interesting music history research topics? If you do, just pick one from our list for free:
- How did the Catholic church influence Renaissance music?
- Social issues described in Baroque-period music.
- Analyze the evolution of Romantic-era music.
- How did the Baroque Opera come to be?
- Who invented Medieval music and when?
- Why has western music almost disappear in the last 10 years?
- Analyze the evolution of music in the Classical era.
- Analyzing violin music performance during the Romantic Era.
Music Argument Topics
Are you looking to find an argument and support it? Then you absolutely need to check out our exceptional list of music argument topics:
- Music today is better than music in the 90s.
- The most lucrative career for a musician.
- Music helps you memorize faster.
- The most popular kind of metal music.
- The evolution of blues songs over the last 30 years.
- Music helps children develop faster.
- Hip-hop is a misunderstood music genre.
- Jazz music is not obsolete.
Music Theory Topics
Interested in writing about music theory? Our amazing academic writers have put together a list of music theory topics for you:
- Analyze the most important aspects of modern music.
- Classical music has specific medical applications.
- Hidden symbols in Renaissance-period music.
- The unique features of Baroque-age music.
- Analyze the evolution of music in the Baroque era.
- The best music compositors in the Romantic era.
- Remarkable characteristics of Romantic-age songs.
- The peculiarities of Asian modern music.
Music Industry Topics
Writing about the music industry can be fun and entertaining. Your professor will love it. Pick one of our music industry topics and start writing:
- What do you associate rock music with and why?
- Should the music industry pay songwriters more?
- How does illegal pirating of songs affect the music industry?
- Do music sharing sites help new artists become famous.
- Analyze the evolution of music labels in the US.
- What differentiates a music label from all others?
- Music talent shows and their effects on a musician’s career.
- The difficulties of signing a contract with a major music label in the US.
Research Paper Topics on Music for High School
Are you a high school student? In this case, you will need our research paper topics on music for high school:
- The best compositors of the Baroque Era.
- What differentiates modern music from classical music?
- Notable women in classical music.
- Analyze the evolution of music in the Modern age.
- How was Beethoven’s music influenced by his loss of hearing?
- How would our world be without music?
- Does music cause negative effects on US teens?
Music Thesis Topics
Writing a thesis about music is not easy. In fact, it can be one of the most difficult projects in your academic career. Start right now by choosing one of the best music thesis topics:
- What made a musician stand out in the Baroque Age?
- The most notable musical experiments in the Classical age.
- Comparing Renaissance and Medieval music styles.
- Analyze the evolution of music in the Renaissance age.
- How did royalty in the UK benefit from music in the Renaissance era?
- Discuss a folk song from the Renaissance age.
- Differences between Asian and European classical music.
Music Controversial Topics
Music, like most other disciplines, has plenty of controversial topics you can talk about. Don’t waste any time and pick one of these music controversial topics:
- Does digital music cut the profits of musicians?
- Who owns the intellectual property to a song?
- The difficulties of getting songwriting credit.
- Illegal downloads are changing the music industry.
- Should music education still be included in the curriculum?
- Analyze medieval liturgical music.
- Music should be free for everyone to download and use.
Persuasive Speech Topics About Music
Are you required the write a persuasive speech about music? If you are, you may need a bit of help. Pick one of these persuasive speech topics about music (updated for 2023):
- Music has a significant effect on advertising.
- The changes rap music has brought to the US culture.
- Indie is a term that should not apply to music.
- Metal music should be banned from the US.
- Does listening to music have a great influence on mental health?
- The amazing evolution of music in the Medieval age.
- People should be free to listen to the music they like for free.
- The fashion industry wouldn’t be where it is today without music.
Easy Topics About Music
Perhaps you don’t want to spend 5 or 6 hours writing the research paper . You need an easier topic. Choose one of these easy topics about music and write the essay fast:
- How can one become a symbol of modern music?
- My favorite singer today.
- Which musician from the past would you bring back to life and why?
- Do politics influence modern music?
- Compare and contrast two music genres.
- Analyze the evolution of music in the modern age in the United States.
- The side effects of turning the volume too loud.
- How is classical music used in Disney movies?
Music Education Research Topics
Are you interested in talking about music education? Perhaps you’ll have some suggestions to make after you’ve done the research. Just choose one of the music education research topics below:
- Can E-Learning be applied to music education?
- Can music teachers offer distance learning services?
- The advantages and disadvantages of Zoom music lessons.
- Why are music worksheets so important for high school students?
- How did the Internet change music education?
- Why are modern music studies so important?
- Should we learn more about Asian music in school?
- How can students learn music while respecting COVID19 measures?
Highly Interesting Music Topics
We know you want a top grade on your next music research paper. We advise you to select one of these highly interesting music topics and surprise your professor:
- How did pop music came to existence and why?
- Analyze the history of hip-hop music.
- Compare metal music with classical music.
- Why is rock music so popular in the United Kingdom?
- Which song would best present our species to aliens?
- Compare and contrast Korean and Chinese music.
- Analyze the popular themes of Japanese music.
- The stunning rise of K-pop bands.
Informative Speech Topics About Music
It’s difficult to find good informative speech topics about music these days. If you want to stand out from the rest of your classmates, choose one of our topics:
- Discuss the ideas presented in romantic music.
- What do people who appreciate classic music have in common?
- Analyze the most popular Bach music.
- Describe the role of market music in the Baroque era.
- Analyze the evolution of European music.
- Ways to make classical music popular with teens in the UK.
- Discuss the most popular musical instrument in the Classical age.
Music Essay Topics for College
Are you a college student? If you want an A+ on your next research paper, use one of these music essay topics for college students:
- Does modern music contain medieval themes?
- Analyze a song from the Renaissance age.
- Why is blues music so important for our culture?
- Who invented the blues genre and when?
- Analyze the evolution of American folk music.
- Most popular names in Baroque-age songs.
- Modern interpretations of medieval songs.
- Listening to blues music can lead to depression.
Need some more music history paper topics? Or perhaps you need a list of music related research topics to choose from for your thesis. Our best paper writer can help you in no time. Get in touch with us and we guarantee that we will find the perfect music topic for your needs. You will be well on your way to getting the A+ you need. Give us a try and get an amazing research topic on music in 10 minutes or less!
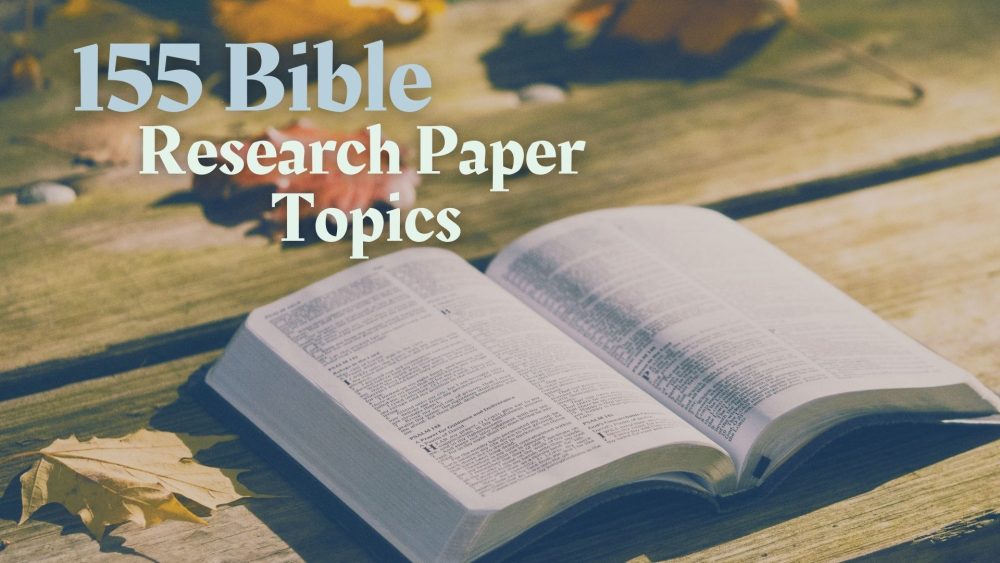
- How It Works
- PhD thesis writing
- Master thesis writing
- Bachelor thesis writing
- Dissertation writing service
- Dissertation abstract writing
- Thesis proposal writing
- Thesis editing service
- Thesis proofreading service
- Thesis formatting service
- Coursework writing service
- Research paper writing service
- Architecture thesis writing
- Computer science thesis writing
- Engineering thesis writing
- History thesis writing
- MBA thesis writing
- Nursing dissertation writing
- Psychology dissertation writing
- Sociology thesis writing
- Statistics dissertation writing
- Buy dissertation online
- Write my dissertation
- Cheap thesis
- Cheap dissertation
- Custom dissertation
- Dissertation help
- Pay for thesis
- Pay for dissertation
- Senior thesis
- Write my thesis
216 Awesome Music Topics That Will Inspire Your Thesis
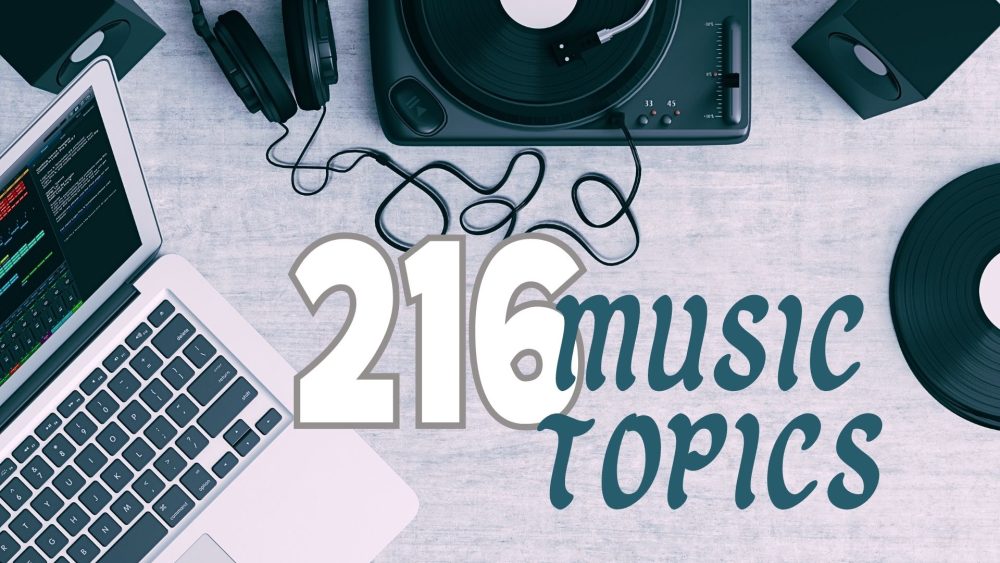
On this page, you will find the ultimate list of 216 brand new, 100% original music topics for high school, college and university students. No, it’s not a trick! You can use any of our topics about music for free and you don’t even have to give us credit. Many of these research topics on music should work great in 2023.
In addition, we have the best step by step guide to writing a research paper right here on this page. Just like the topics, you can read the guide for free. It will help you stay focused on what’s important and ensure you don’t miss any steps. And remember, if you need assistance with your academic writing tasks, our native English-speaking writers are the most reliable on the Internet!
Writing A Research Paper About Music
So, what is music? Music is a form of art that uses sound and rhythm to create an emotional or aesthetic experience. It can be created by combining different elements such as melody, harmony, rhythm and timbre. Music is a universal language that can be found in all cultures and has been an important part of human history for thousands of years. It can evoke emotions, tell stories, and communicate ideas. Music can take many forms, including vocal or instrumental, solo or ensemble, live or recorded, and can be classified into various genres such as rock, pop, classical, jazz, and many more.
But how do you write a research paper about music quickly? Well, we have a great step by step guide for you right here.
Choose a music topic. Select a topic that interests you and that you have enough background knowledge on to research and write about. Conduct research. Use a variety of sources to gather information on your topic, including books, academic journals, online databases, and primary sources such as interviews or musical recordings. Organize your research. Once you have gathered enough information, organize your research into an outline or a mind map to help you visualize how your paper will flow. Write a thesis statement. Your thesis statement should be a concise statement that summarizes the main argument of your paper. Write a rough draft. Begin writing your paper using the information you have gathered and the outline or mind map you created. Focus on creating a clear and coherent argument, and be sure to cite all sources using the appropriate citation style. Help with coursework services can aid you in succeeding with this part. Revise and edit. Once you have completed a rough draft, revise and edit your paper to improve its clarity, organization, and coherence. Check for grammar and spelling errors, and make sure all citations are correct and properly formatted. Create a bibliography or works cited page. Include a list of all sources you used in your research, including books, articles, interviews, and recordings. Finalize your paper. After making all necessary revisions and edits, finalize your paper and ensure that it meets all the requirements set by your instructor or professor. Proofread everything and make sure it’s perfectly written. You don’t want to lose points over some typos, do you?
Easy Research Topics About Music
- The history and evolution of hip-hop culture
- The impact of classical music on modern composers
- The role of music in therapy for mental health
- The cultural significance of jazz in African-American communities
- The influence of traditional folk music on contemporary artists
- The development of electronic music over the past decade
- The use of music in film to enhance storytelling
- The rise of K-pop and its global popularity
- The effects of music on our learning abilities
- The use of music in branding in the fashion industry
- The influence of the Beatles on popular music
- The intersection of music and politics in the 1960s
- The cultural significance of reggae music in Jamaica
- The history and evolution of country music in America
- The impact of music streaming on the music industry
Opinion Essay Music Topics
- Music piracy: Should it be considered a serious crime?
- Should music education be mandatory in schools?
- Is autotune ruining the quality of music?
- Are music awards shows still relevant in today’s industry?
- Should music lyrics be censored for explicit content?
- Is it fair that some musicians earn more money than others?
- Is classical music still relevant in modern society?
- Should music festivals have age restrictions for attendees?
- Is it fair for musicians to be judged on their personal lives?
- Is the current state of the music industry sustainable?
- Should musicians be held accountable for the messages in their lyrics?
- Is the role of the record label still important in the age of digital music?
- Should musicians be able to express their political views in their music?
- Does the use of music in movies and TV shows enhance or detract from the storytelling?
Interesting Music Research Topics
- The impact of music on athletic performance
- The use of music in advertising and consumer behavior
- The role of music in enhancing cognitive abilities
- The effects of music on stress reduction and relaxation
- The cultural significance of music in indigenous communities
- The influence of music on fashion and style trends
- The evolution of protest music and its impact on society
- The effects of music on Alzheimer’s disease
- The intersection of music and technology in the music industry
- The effects of music on emotional intelligence and empathy
- The cultural significance of hip hop music in the African diaspora
- The influence of music on human behavior and decision-making
- The effects of music on physical performance and exercise
- The role of music in promoting social and political activism
Research Paper Topics On Music
- The effects of music on the brain and mental health
- The impact of streaming on the music industry
- The history and evolution of rap music
- The cultural significance of traditional folk music
- The use of music in video games to enhance the gaming experience
- The role of music in religious and spiritual practices
- The effects of music on memory and learning
- The development of rock and roll in America
- The intersection of music and politics in the 21st century
- The cultural significance of country music in the South
- The use of music in autism therapy
- The impact of social media on music promotion and marketing
- The influence of music on the LGBTQ+ community
- The effects of music on social behavior and interaction
Argumentative Essay Topics About Music
- Does music have a negative effect on behavior?
- Is streaming music harming the music industry?
- Can music censorship be justified in certain cases?
- Is cultural appropriation a problem in the music industry?
- Should musicians be held accountable for controversial lyrics?
- Is autotune a helpful tool or a crutch for musicians?
- Should music education be a required part of the curriculum?
- Is the use of explicit lyrics in music harmful?
- Should music festivals be required to have safety measures?
- Does the use of profanity in music undermine its artistic value?
- Can music be used to promote political messages effectively?
- Should musicians be allowed to profit from tragedies?
Current Music Topics To Write About In 2023
- The rise of TikTok and its impact on music promotion
- The effects of the COVID-19 pandemic on UK music
- The use of virtual concerts and live streaming during COVID-19
- The influence of social media on music consumption and trends
- The emergence of new genres and sub-genres in popular music
- Talk about cancel culture in music
- The debate over the use of explicit lyrics in music
- The impact of climate change on music festivals and events
- The use of artificial intelligence in music production and composition
- The influence of music on political and social movements
- The rise of female and non-binary artists in the music industry
- The effects of globalization on the diversity of music around the world
- The role of nostalgia in the popularity of music from past decades
Musical Topics About Famous Musicians
- The life and legacy of Beethoven
- The impact of Elvis Presley on rock and roll
- The career and contributions of Bob Dylan
- The influence of Michael Jackson on pop music
- The musical evolution of Madonna over time
- The enduring appeal of the Rolling Stones
- The career of Prince and his impact on music
- The contributions of David Bowie to pop culture
- The iconic sound of Jimi Hendrix’s guitar
- The impact of Whitney Houston on the music industry
- The life and career of Freddie Mercury of Queen
- The artistry and impact of Joni Mitchell
- The groundbreaking work of Stevie Wonder in R&B
- The musical legacy of the Beatles and their influence on pop music
Music Research Paper Topics For College
- The cultural significance of the accordion in folk music
- The use of sampling in hip-hop and electronic music production
- The evolution of the drum kit in popular music
- The significance of Taylor Swift in contemporary country-pop music
- The effects of drug abuse in the music industry
- The role of music in shaping political movements and protests
- The impact of streaming services on the music industry and artists’ income
- The significance of the Burning Man festival in music and culture
- The emergence and growth of Afrobeat music globally
- The role of musical collaboration in the creation of new music genres
- The use of autotune and other vocal processing tools in pop music
- The effects of social and political issues on rap music lyrics
- The significance of the Coachella Valley Music and Arts Festival in pop culture
- The impact of music on emotional regulation and mental health
Our Controversial Music Topics
- The controversy of the “cancel culture” in US music
- The impact of music piracy on the industry and artists
- The ethical concerns of music sampling without permission
- The controversy surrounding lip-syncing during live performances
- The debate over the authenticity of auto-tune in music
- The controversy surrounding the use of profanity in music
- The debate over the cultural appropriation of music styles
- The controversy surrounding music festivals and their impact on local communities
- The debate over the role of music in promoting violence and aggression
- The controversy surrounding the ownership of an artist’s discography
- The ethical concerns of musicians profiting from songs about tragedies and disasters
Captivating Music Thesis Topics
- The role of music in promoting social justice
- The impact of music streaming on album sales
- The significance of lyrics in contemporary pop music
- The evolution of heavy metal music over time
- The influence of gospel music on rock and roll
- The effects of music education on cognitive development
- The cultural significance of hip-hop music in America
- The role of music in promoting environmental awareness and activism
- The impact of music festivals on local economies
- The evolution of country music and its impact on popular music
- The use of music in advertising and marketing strategies
Classical Music Topic Ideas
- The influence of Baroque music on classical music
- The history and evolution of the symphony orchestra
- The career and legacy of Wolfgang Amadeus Mozart
- The significance of Ludwig van Beethoven’s Ninth Symphony
- The evolution of opera as an art form
- The role of women composers in classical music history
- The impact of the Romantic era on classical music
- The use of program music to tell a story through music
- The significance of the concerto in classical music
- The influence of Johann Sebastian Bach on classical music
- The contributions of Antonio Vivaldi to the concerto form
- The use of counterpoint in classical music composition
- The role of chamber music in classical music history
- The significance of George Frideric Handel’s Messiah in classical music
Interesting Music Topics For High School
- The history and evolution of the piano as a musical instrument
- The significance of Beethoven in classical music
- The impact of Elvis Presley on US music
- The emergence and growth of the hip-hop music genre
- The role of music festivals in contemporary music culture
- The effects of technology on music production and performance
- The influence of social media on music promotion and distribution
- The effects of music on mental health and well-being
- The role of music in popular culture and media
- The impact of musical soundtracks on movies and TV shows
- The use of music therapy for individuals with autism spectrum disorder
- The significance of the Coachella Music Festival in modern music culture
- The cultural significance of the ukulele in Hawaiian culture
Awesome Music Research Questions For 2023
- Should musicians be required to use their platform to promote social justice causes?
- Is music piracy a victimless crime or does it harm the industry?
- Should music venues be required to provide safe spaces for concertgoers?
- Is the Grammy Awards selection process biased towards mainstream artists?
- Should music streaming services pay musicians higher royalties?
- Is it appropriate for music to be used in political campaign advertisements?
- Should music journalists be required to disclose their personal biases in reviews?
- Is it ethical for musicians to profit from songs about tragedies and disasters?
- Should music education be funded equally across all schools and districts?
- Is it fair for record labels to own the rights to an artist’s entire discography?
- Should music festivals have more diverse and inclusive lineups?
- Should musicians be allowed to use drugs and alcohol as part of their creative process?
Fantastic Music Topics For Research
- The evolution of the electric guitar in rock music
- The cultural significance of the sitar in Indian music
- The impact of synthesizers on contemporary music production
- The use of technology in the creation and performance of music
- The influence of Beyoncé on modern pop music
- The significance of Kendrick Lamar in contemporary rap music
- The effects of misogyny and sexism in the rap music industry
- The emergence and growth of K-pop music globally
- The significance of Coachella Music Festival in the music industry
- The history and evolution of the Woodstock Music Festival
- The impact of music festivals on tourism and local economies
- The role of music festivals in shaping music trends and culture
- The effects of music piracy on the music industry
- The impact of social media on the promotion and distribution of music
- The role of music in the Black Lives Matter movement
Catchy Music Related Research Topics
- Is hip-hop culture beneficial or harmful to society?
- Is it ethical to sample music without permission?
- Should music streaming services censor explicit content?
- Is auto-tune a valid musical technique or a crutch?
- Does the music industry unfairly exploit young artists?
- Should radio stations be required to play a certain percentage of local music?
- Is the practice of lip-syncing during live performances acceptable?
- Is music education undervalued and underfunded in schools?
- Does the use of profanity in music contribute to a decline in society?
- Should music venues be held accountable for the safety of concertgoers?
Informative Speech Topics About Music
- The history and evolution of jazz music
- The cultural significance of classical music in Europe
- The origins and development of blues music in America
- The influence of Latin American music on American popular music
- The impact of technology on music production and distribution
- The role of music in expressing emotions and feelings
- The effects of music therapy on mental health and wellbeing
- The cultural significance of traditional music in Africa
- The use of music in films and television to create mood and atmosphere
- The influence of the Beatles on popular music and culture
- The evolution of electronic dance music (EDM)
- The role of music in promoting cultural diversity and unity
- The impact of social media on the music industry and fan culture
Get Quick Research Paper And Thesis Help
Do you need assistance with your research paper or thesis? Getting thesis help has never been easier! Our experts with write you a high quality academic paper in as little as 3 hours, even in the middle of the night. Yes, our customer support department is online 24/7 to take your order and assign it to our best available writer. We can write you a good essay for any class and any course – online or face to face.
Get a cheap thesis writing help about music fast and spend more free time with your friends or family. Everything we send to our customers is unique, 100% written from scratch online. Every student will be satisfied with our work because every professor will be compelled to give our work an A+ rating. What are you waiting for? Get the help you need right now and improve your GPA!
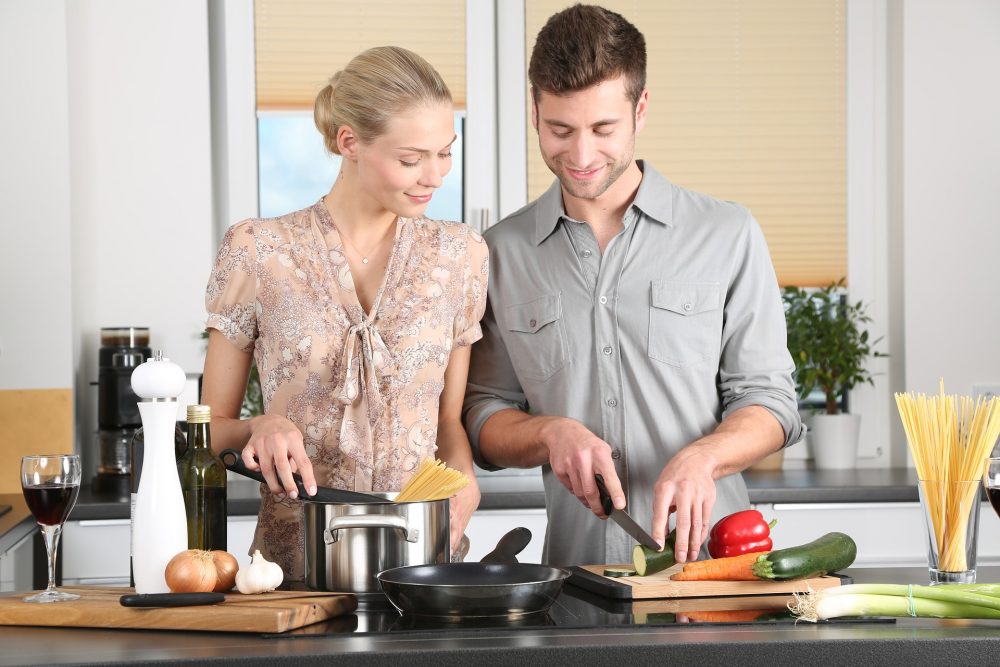
Leave a Reply Cancel reply
Your email address will not be published. Required fields are marked *
Comment * Error message
Name * Error message
Email * Error message
Save my name, email, and website in this browser for the next time I comment.
As Putin continues killing civilians, bombing kindergartens, and threatening WWIII, Ukraine fights for the world's peaceful future.
Ukraine Live Updates
- Paper writing help
- Buy an Essay
- Pay for essay
- Buy Research Paper
- Write My Research Paper
- Research Paper Help
- Custom Research Paper
- Custom Dissertation
- Dissertation Help
- Buy Dissertation
- Dissertation Writer
- Write my Dissertation
- How it works
200 Best Music Research Paper Topics For Students
Music research topics are an excellent opportunity to trace the history of the development of individual genres or entire eras. You can create an essay or research paper with an emphasis on certain stylistic features, or delve deeper into the technical aspects of album making. Also, the research topics in music allow you to learn more about popular composers, musicians, and individual bands. You can find out the history of creating certain songs or finding out the nuances of the breakup of groups.
While music research paper topics seem easy, it still requires a good outline and reliable sources to gather information. The life of many musicians is very busy, so certain topics for music research papers may require a more thorough analysis. For example, you will need to research the biography and all creation stages of famous music industry personalities.
Any research topic about music should be analyzed, and only verified facts added. You should also avoid using emotional coloring and bias. And don't forget about formatting. Any interesting music topics require clear structuring into paragraphs, lists, and subheadings.
By popular genres & styles
Individual styles are especially appreciated in research paper topics on music. You can choose the genre or group that interests you. This will allow you to get additional motivation and focus more on facts. The main challenge in this case is to find authoritative sources.
- The impact of rock and roll on the modern music industry.
- The basic concepts of creating musical songs.
- Rock performers and their popularity in society.
- Reasons for the negative attitude towards the rock vocalist.
- Rock musicians and problems with the law.
- The nuances of alcohol addiction of rock musicians.
- The main features of creating rock songs.
- Musical agitation as the main motive of rock songs.
- The main reasons for making rock songs.
- Symbiosis of rock and classical music.
- Rock performers and popular musicians.
- The analysis of the creative personality on the example of Kurt Cobain.
- The modern musical trend in culture.
- Top 10 most popular metal groups.
- Why has metal music become so popular?
- The mix of traditional music and heavy metal.
- Analysis of lyrical constructions of metal performers.
- The symbiosis of musical instruments in metal music.
- The analysis of seventh chords in the construction of metal songs.
- The influence of metal on other genres.
- The symbiosis of metal and pop music.
- The influence of metal vocalists on American culture.
- The symbiosis of genres as the reason for the creation of metal.
- The modern icons of the metal scene.
- The best metal bands in the last thirty years.
- The analysis of the dynamics of the popularity of metal bands.
- The modern concerts on the example of metal bands
- Female vocalists in pop music.
- The reason for the creation of numerous female musical groups.
- Pop music as a tuning fork of public morality.
- Why is pop music degrading?
- How can pop music be used to improve college grades?
- The nuances of using pop music in contemporary American culture.
- How can pop music be used to improve mood?
- The symbiosis of pop music and rap culture.
- How does contemporary pop music affect young people?
- The study of pop music in the context of the social culture of Harlem.
- The classic examples of pop artists.
- Madonna: the most popular popes of personalities.
- The analysis of the popularity of Britney Spears.
- Pop icons of the past decade.
- Hip-hop as the basis of the movement for social equality.
- The origins of hip-hop and the reasons for its popularization.
- How does hip-hop affect contemporary pop artists?
- The analysis of hip-hop performers on the example of the best vocalists of the decade.
- How does hip-hop allow athletes to train?
- Modern hip-hop and new musical trends.
- The symbiosis of hip-hop and metal music.
- How does hip-hop motivate people for sporting achievements?
- The analysis of hip-hop performers on the example of female vocalists.
- Modern hip-hop and its impact on youth.
- The main aspects of the integration of hip-hop music in the modern community.
- All technical aspects of creating hip-hop music.
- The classic approach to the formation of hip-hop motives.
- The analysis of the structure of hip-hop songs.
- The best hip-hop artists of the last decade.
- The stages of the formation of jazz as a separate musical genre.
- Why is jazz so popular these days?
- The nuances of studying jazz musical combinations.
- How Jess influences the structuring of student learning.
- The nuances of jazz performers in modern America.
- The best American jazz performers.
- Jazz as the most structured musical theory.
- How can you quickly learn to create jazz compositions?
- The influence of jazz on the cultural and political elite of the United States.
- Can jazz replace other musical styles?
- Jazz fusion as an example of musical prowess.
- The technical aspects of creating a pentatonic scale in a jazz style.
- The selection of jazz musicians.
- The development of jazz in the United States.
- The main reasons for the popularization of jazz in modern society.
- Blues and its influence on the development of the music industry.
- The symbiosis of blues and jazz.
- Can the Blues be compared to classical music?
- How do contemporary artists use the Blues in pop music?
- Historical context creation of blues compositions.
- How does the Blues affect rock music?
- Can the Blues help students learn?
- How blues musicians are developing in the USA.
- Can blues be used as a springboard for classical music production?
- The best US blues artists of the last 20 years.
- Blues performers of the last ten years.
- The influence of the blues on the formation of other genres.
- The analysis of the statistical popularity of the blues.
- The critical aspects when creating Blues compositions.
- The selection of blues parties when creating music.
- The influence of classical music on pop culture.
- The classical music and the best composers of the last century.
- Beethoven and his best works.
- How did Mozart influence classical music?
- Is the symbiosis of jazz and classical music possible?
- The structure of making classical music.
- The stages of the formation of classical music in modern society.
- Can you replace pop culture with classical music?
- How does classical music affect the psychological state of people?
- The classical music and symbiosis with opera.
- The basic concept of the analysis of classical music.
- A technical comparison of the mastery of classical composers.
- The choice of classical music for the mood.
- The classical music and its influence on rock culture.
- The main technical aspects of creating a score.
The region is important for those looking for musical topics for research paper. Most genres of European music and some information about composers are open to general use. If your research topic on music is aimed at analyzing the Arab countries, then you will need more time looking for reliable information. The fact is that not all Muslim archives are in the public domain.
Western music
- The features of musical motives of Western music.
- The history of Western music with real examples.
- How has Western music changed over the past two hundred years?
- Is it possible to combine Western music with European motives?
- The features of the use of traditional Western musical instruments.
- How do Western countries use music for meditation?
- Western music in the context of modern society.
- The role of Western music in the life of native people.
- How melodic is oriental music?
- The stages of the formation of Western music in American culture.
European music
- European music and modern trends.
- British pop bands and their worldwide popularity.
- How popular are German pop bands in the USA?
- European music and national musical instruments.
- How does European music affect well-being?
- The analysis of European music with specific examples.
- Top 10 of the greatest European musical groups.
- The analysis of European music on the example of instrumental groups.
- The best pop music performers in Europe.
- How does pop music influence the development of culture?
Asian music
- Asian music: the example of ethnic trends.
- The influence of Asian music on world culture.
- The main musical instruments of Asia.
- Can you compare Asian music with European motives?
- How has Asian music changed over the past hundred years?
- The nuances of creating Asian music.
- How does Asian music influence contemporary cinema?
- The best Asian performers.
- Top 10 Asian vocalists who have conquered the whole world.
- Do national instruments influence the creation of Asian music?
By history periods
You can use music appreciation research paper topics to analyse a specific period in history. Baroque, Renaissance and other eras are especially relevant for research as they allow you to see the history of the development of music. You can concentrate on a specific time period and the most famous composers.
- Medieval music and its influence on the Crusades.
- The major trends in the medieval music industry.
- The influence of kings on the creation of medieval music.
- The main musical instruments in medieval Europe.
- Musical instruments in Central Asia during the Middle Ages.
- What kind of music was popular in the Middle Ages.
- How difficult was the life of a musician in the Middle Ages?
- The analysis of medieval music on modern examples.
- How has contemporary music influenced pop culture?
- Historical aspects of the creation of medieval music.
- The influence of medieval music and culture.
- The rhythmic pattern of medieval music.
- The medieval music during the feast.
- The influence of medieval music on classical music.
- The medieval music channel and musical comparison.
Renaissance
- The dawn of musical culture during the Renaissance.
- The analysis of Renaissance music with specific examples.
- How has Renaissance music influenced contemporary pop culture?
- The analysis of Renaissance music as a constructive masterpiece.
- The nuances of Renaissance music and, most importantly, musical instruments.
- How difficult is it to reproduce Renaissance music in today's environment?
- The analysis of structural compositions of the Renaissance.
- Renaissance music as a tuning fork of public morality.
- How has music changed since the Renaissance?
- Can Renaissance music be used to create a modern instrumental ensemble?
Baroque Age (XVI-XVIII)
- The influence of politicians on the formation of music during the Baroque period.
- How has Baroque influenced contemporary instrumental music?
- The nuances of musical constructions during the Baroque period.
- How has the baroque influenced modern instruments?
- The nuances of the Baroque in the context of the complexity of musical compositions.
- The main effect of the Baroque in contemporary music.
- The historical aspects of the creation of the Baroque as a separate genre of music.
- The influence of the Baroque on the creation of contemporary musical groups.
- The analysis of the structure and musical motives of Baroque in detail.
- Baroque in contemporary music.
- The nuances of creating songs in the Baroque style.
Classical Age (XVIII-XIV)
- The Classical Age of music in modern society.
- How did the Classical Age influence the formation of musical trends?
- The general concept of the Classical Age in instrumental music.
- The nuances of creating music based on the Classical Age.
- How did the Classical Age influence the creation of pop culture?
- The theory of creating musical compositions on the example of the Classical Age.
- The general factors of the Classical Age in instrumental music.
- The main trends and popular instruments of the Classical Age.
- The main musical compositions of the 14th century.
- The main factors in the creation of musical compositions in the 13th century.
Romantic Era (XIV-XX)
- The Romantic Era and its impact on contemporary music.
- The main principles of structuring music into the Romantic Era.
- Features of creating instrumental compositions in the Romantic Era.
- The Romantic Era and modern music trends.
- The main factors influencing the Romantic Era in the music industry.
- Key figures in the music industry and their passion for the Romantic Era.
- How did the Romantic Era form the modern style?
- How has the Romantic Era influenced rhythmic music?
- The Romantic Era in the music industry.
- The main aspects of the formation of the Romantic Era in musical culture.
- Making marching music in the Romantic Era.
- Features of creating musical compositions.
- The technical aspects that influenced modern romantic motives.
Modern Era (XX-XXI)
- Jazz music as a phenomenon of the modern roar the influence of the modern era on instrumental music.
- Technical aspects of hip-hop and Reggae.
- How is contemporary classical music created?
- Can modern music genres be combined to create something new?
- Why is the modern music industry stagnating?
- The aspects of contemporary music.
- How does instrumental music affect culture?
- Contemporary music and technical innovation.
- How is contemporary music created?
- The nuances of creating hip-hop albums.
How To Write On Music Related Research Topics
By choosing topics about music for an essay, you get the opportunity to prepare a detailed paper work with facts, genre nuances and detailed biographies of famous musicians. You need to stick to the formatting and your outline. Find reliable information for music history research topics and talk about the emergence of certain genres.
Music business research topics are especially important, as you need to consider not only the stylistic but also the commercial nuances of the bands. For example, you can prepare detailed data on annual music tours or album sales.
All music appreciation presentation topics require detailed factual focus, which can be difficult for many people. If you are not ready to do it yourself, then we can help you.
Our service will solve your problem with music research topics high school. We also guarantee that you will get a good grade. We will help you organize all the nuances so that your music history paper topics become a reason for pride and high scores.
An Inspiration List
- popular music | Description, History, & Facts | Britannica
- History of music
- Music History from Primary Sources
- Brief History of Music: An Introduction
- How Music and Instruments Began

IMAGES
COMMENTS
Subsequently, musical activities and traditions incrementally evolved throughout modernity (from 250 Kya onwards), global dispersal from Africa (currently thought to be from 60-100 Kya onwards), and the Holocene (from 12 Kya). In this article I provide an overview of recent research and a sketch of music's evolutionary career.
Now published by Routledge, the Journal of Musicological Research is a peer-reviewed quarterly publication with an international circulation. Readership includes professionals, academics, and students of musicology, as well as composers, historians, musicians, and individuals interested in music scholarship. Read full aims and scope.
In response, Fink made a persuasive refutation of the paper's central finding of decreasing musical diversity and the newspaper headlines touting it ("Modern Music too Loud, All Sounds the ...
Contemporary Music Review is an interdisciplinary forum for research in and about music now. Our six issues per year feature articles and essays across a broad spectrum of global contemporary music research as well as reports, bibliographic studies, interviews, scores and translations. Interested guest editors may submit proposals for themed ...
View PDF View EPUB. This journal's title, Contemporary Music Review, implies a special relationship with music history. Rather than foreground genre, idiom, location, period or technique, the adjective 'contemporary' seems to stake out boundaries at the edge of time. Unlike other historical periods, the contemporary needs neither beginning ...
Abstract. In modern societies, cultural change seems ceaseless. The flux of fashion is especially obvious for popular music. While much has been written about the origin and evolution of pop, most claims about its history are anecdotal rather than scientific in nature. To rectify this, we investigate the US Billboard Hot 100 between 1960 and 2010.
Winner-takes-all. Cultural markets often exhibit a highly skewed distribution of success (e.g. Keuschnigg, 2015, Salganik et al., 2006).In the music market in particular, a few hits expand across ...
The ubiquity of digital music consumption has made it possible to extract information about modern music that allows us to perform large scale analysis of stylistic change over time. In order to uncover underlying patterns in cultural evolution, we examine the relationship between the established characteristics of different genres and styles, and the introduction of novel ideas that fuel this ...
Like language, music is a human universal involving perceptually discrete elements displaying organization 1. Therefore, contemporary popular music may have a well-established set of underlying ...
In The Republic III (Plato, ∼360 BC/2006), Plato attributes to music a powerful influence on the personality of its audience.He and his contemporaries, as part of the Greek theory of musical ethos, argued that music can be a very powerful tool for education. They believed that a selection of particular modes in music could possibly reinforce positive human characteristics and inhibit ...
The rising popularity of streaming services has made in-depth musical data more accessible than ever before and has created new opportunities for data mining. This project utilizes data from 19,000 songs made available by Spotify. Several data mining algorithms (including J48 Decision Trees, Random Forest, Simple K Means, NaiveBayes, ZeroR, and ...
Moreover, there remains no agreement about the underlying dimensions of these functions. Part one of the paper reviews the research contributions that have explicitly referred to musical functions. It is concluded that a comprehensive investigation addressing the basic dimensions underlying the plethora of functions of music listening is warranted.
Provides scholarly coverage of modern popular music worldwide, from the early 20th century to the present day, by cross-searching the 14-volume Bloomsbury Encyclopedia of Popular Music of the World; all 120+ volumes of the 33 1/3 book series (providing in-depth analysis of influential albums); an expanding range of scholarly books from Bloomsbury Popular Music Studies; and titles in the new 33 ...
1. Introduction. Music, and, in particular, songs, rarely leave people unmoved. There is something magical about music and scientists have been trying to disentangle the magic and explain what it is that makes us love some music, hate other music and just listen to music [1-3].In particular, it has been noticed that different generations like different types of music.
Structure, Texture, and Influence in the Music of the Mahavishnu Orchestra" (conference paper, 2001) This paper examines parallels between jazz-rock fusion of the late 1960s/early 1970s and well-established jazz practices in the 1950s and 60s, focusing especially on the music of John Coltrane and John McLaughlin's Mahavishnu Orchestra.
For those papers that describe a generation system, we also include: • System name: Only if the paper uses a specific name for the system. • Dataset: Dataset used for training. • Music Representation: The type of music representation used in the paper (symbolic or audio). • Type of generation: Ex Nihilo, inpainting, harmonization, etc. •
Abstract. The essay presents an attempt at characterizing contemporary music's culture by identifying a dialectical tension between "modern" and "postmodern" currents in it. After ...
William Shakespeare entertained many ideas about music, some of them conflicting, and he frequently represented these ideas in his plays. Music was a multifaceted art and science in early modern England, and debates over the nature and interpretation of music played out in a variety of contexts: academic, religious, political, commercial, and ...
The paper synthesises indicative research findings and considers the implications for . ... Journal of Research in Music Education, 47(5), 198-212. Davidson, J.W. & Good, J.M.M. (2002) 'Social ...
Then you absolutely need to check out our exceptional list of music argument topics: Music today is better than music in the 90s. The most lucrative career for a musician. Music helps you memorize faster. The most popular kind of metal music. The evolution of blues songs over the last 30 years. Music helps children develop faster.
Fantastic Music Topics For Research. The evolution of the electric guitar in rock music. The cultural significance of the sitar in Indian music. The impact of synthesizers on contemporary music production. The use of technology in the creation and performance of music. The influence of Beyoncé on modern pop music.
Analysis of lyrical constructions of metal performers. The symbiosis of musical instruments in metal music. The analysis of seventh chords in the construction of metal songs. The influence of metal on other genres. The symbiosis of metal and pop music. The influence of metal vocalists on American culture.